The diagnostic value of vascular architecture in solid solitary pulmonary nodules quantified by dynamic contrast enhanced MRI
Highlight box
Key findings
• Quantitative parameters Ktrans and Ve values of contrast enhanced magnetic resonance imaging (DCE-MRI) showed high diagnostic efficacy for malignant solid solitary pulmonary nodules (SSPNs).
• Quantitative DCE-MRI parameters of SSPNs correlate with some vascular architectural parameters.
• DCE-MRI provides a non-invasive, ionizing radiation-free method for the differential diagnosis of SSPNs.
What is known and what is new?
• Early diagnosis of SSPNs is critical for improving prognosis and enhancing survival.
• Microvessel density, vascular endothelial growth factor and microvascular perfusion index are histological markers reflecting tumor microangiogenesis and vascular normalization.
• DCE-MRI is now clinically used to evaluate microvascular changes in tumors of such as breast, prostate and rectal cancer.
• Our study adds conventional MRI scanning with respiratory gating as well as artifact correction to ensure image quality.
• Differential diagnosis of SSPNs at the level of the vascular microenvironment using quantitative DCE-MRI parameters is helpful.
What is the implication, and what should change now?
• Due to improvements in scanning and post-processing techniques, magnetic resonance technology has been progressively used in the differential diagnosis of lung lesions, assessment of efficacy, and prediction of prognosis.
• Evaluation of SSPNs that are difficult to identify clinically can be attempted by means of DCE-MRI examination.
Introduction
Lung cancer is an important public health problem worldwide, the leading cause of cancer-related deaths globally, and the malignant tumor with the highest morbidity and mortality rate in China (1,2). A study investigating more than 37.5 million cancer patients from 71 countries across a 15-year period [2000–2014] found that survival rates for lung cancer patients in some developed countries have improved by nearly 5% (3); however, the survival rate of Chinese lung cancer patients is still at a low level, and there are large differences between regions. The registry of the National Cancer Center, through large-scale screening and follow-up, found that the 5-year overall survival rate of lung cancer is 19.7%, but the 5-year survival rate of early-stage lung cancer can reach 90% (4). This may be due to the high malignancy of advanced lung cancer, the rich blood supply of the lesion and microvascular invasion increasing the risk of distant metastasis and the complexity of prognosis (5). Survival is significantly shorter in patients presenting with early metastases, and a study published in the Lancet as early as 1992 has shown that microvessel counts and vascular maturation significantly correlate with the progression and prognosis of distant metastases from lung cancer (6).
Microvessel density (MVD) is known to be a common marker for quantifying angiogenesis within tumors (7). In this study on lung adenocarcinoma, MVD expression has been found to correlate with tumor prognosis and can be an important predictor of overall survival and progression-free survival (8). Using immunohistochemical staining, the vascular endothelial cell marker CD31 and the perivascular cell and smooth muscle cell positive reactant smooth muscle actin (SMA) antibodies together quantitatively assess MVD. CD31-MVD is expressed in both mature and immature microvascular endothelial cells, while SMA-MVD is a marker of mature endothelial cells only (9). For a comprehensive assessment of the vascular architecture of solid solitary pulmonary nodules (SSPNs), the clinical basis also includes an assessment of the “vascular normalization” index: microvascular perfusion index (MPI), which represents the proportion of mature blood vessels to vascular endothelial cells (MPI = SMA-MVD/CD31-MVD) (10). Vascular endothelial growth factor (VEGF) contributes to tumor cell infiltration, proliferation and metastasis by promoting tumor neovascularization and altering vascular permeability (11).
Currently, MVD, VEGF, and MPI are quantitative indicators for clinically assessing the vascular architecture of lung nodules, which are closely related to the prognosis and survival of lung cancer patients. However, the detection of the expression of the above indicators relies on postoperative immunohistochemistry, and the method of pathological microangiometry is not only cumbersome, but also does not allow for dynamic and reproducible observation. Preoperative quantification of SSPNs vascular architecture using a method that is rapid, noninvasive, reproducible, and reflective of the full extent of the lesion is clinically important.
Computed tomography (CT) is currently the most commonly used imaging tool for the examination of SSPNs, and differential diagnosis of SSPNs is based on CT morphologic features; however, plain scanning does not provide information on physiologic function, and the smaller the diameter of the lesion, the greater the diagnostic challenge; and the radiation dose of enhancement scanning restricts the wide range of clinical applications (12-14). Dynamic contrast-enhanced magnetic resonance imaging (DCE-MRI) is a non-ionizing radiation, non-invasive examination method, capable of multi-parameter, multi-sequence high soft-tissue resolution imaging. Malignant tumors are usually characterized by greater blood flow and more irregular vascular structures (15). DCE-MRI scanning captures the dynamic changes of vasculature in the tumor region, and its quantitative parameters reflect the tumor vascular characteristics and tissue microenvironment by evaluating the vascular permeability and the distribution of contrast, which is of large scope of application for comprehensive and precise assessment of lung cancer. For example, a two-dimensional imaging histology model based on T2-weighted imaging (T2WI) images has achieved good results in the differential diagnosis of lung nodules (16), with high diagnostic efficacy in the pathologic subtyping of patients with non-small cell carcinoma (17), and can also be used to assess the efficacy of non-surgical therapeutic means in patients with lung cancer (18-20) and to predict the gene mutation status of patients with lung adenocarcinoma (21).
The aim of this study was to investigate DCE-MRI quantitative perfusion parameters including volume transfer constant (Ktrans), rate constant (Kep), and extravascular extracellular volume fraction (Ve), and to assess their value for application to vascularization of SSPNs. We present this article in accordance with the STARD reporting checklist (available at https://jtd.amegroups.com/article/view/10.21037/jtd-24-1467/rc).
Methods
Sample size estimation
In this study, the sample size was calculated using Ktrans as the primary outcome indicator, and based on previous literature (22), the mean was expected to be 0.12 with a standard deviation of 0.07 for the benign group and 0.21 with a standard deviation of 0.15 for the malignant group. We utilized the “Two independent samples t-test-unequal variance” module in PASS version 15 for the sample size calculation, the ratio of the sample size of the two groups 1:2, take α=0.05, β=0.2, test efficacy of 1 − β =0.8, the use of two-sided test, the calculation of a total of 78 cases need to be to fulfill the requirement, 79 cases were included in this study, which fulfills the requirement.
Ethical statement
The study was conducted in accordance with the Declaration of Helsinki (as revised in 2013). The study was approved by the review board of The First Affiliated Hospital of Guangxi Medical University (No. 2024-E232-01) and informed consent was obtained from all individual participants.
Study cohort
The study cohort flow chart is shown in Figure 1. We prospectively included patients who underwent DCE-MRI of the chest and concurrent surgical treatment at The First Affiliated Hospital of Guangxi Medical University from November 2021 to December 2023, and whose pathological results were obtained. Patients were eligible for enrollment if they met the following criteria: (I) solid isolated pulmonary nodular lesions (≥1 and ≤3 cm) were diagnosed by chest CT at our hospital and underwent chest DCE-MRI enhancement scanning; (II) the lesions had homogeneous density, were generally free of calcification or cavitation, and were not surrounded by satellite foci or pulmonary atelectasis; and (III) they all were operated on within 1 week of the DCE-MRI examination, and the postoperative specimens of the pulmonary lesions were obtained and all imaging data were retained. Subjects were excluded from the study if they (I) had received prior radiotherapy or other therapies for the treatment of lung lesions; (II) had incomplete clinical data; and (III) had not undergone relevant immunohistochemistry. All patients included underwent serological tests using the 7600-120 automatic biochemical analyzer (Hitachi High-Tech, Tokyo, Japan) in our hospital’s clinical laboratory within 2 weeks prior to surgery. Postoperatively, clinical TNM staging and pathological grading were performed.
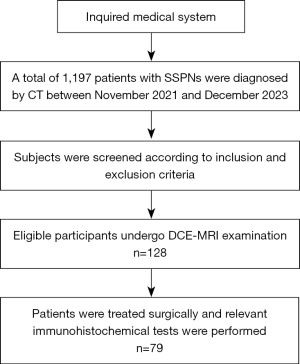
Magnetic resonance imaging (MRI) examination
All patients underwent routine chest MRI plain scanning and dynamic enhancement MRI scanning using a 3.0T MRI scanner (MAGNETOM Verio) equipped with an 8-channel body phase array coil and respiratory gating. All patients were placed in the supine position with the head moved forward, and their respiratory rate and amplitude were matched to the scan as instructed. Routine MRI plain scan included turbo spin echo (TSE) T2WI axial, coronal and dysplasia free breathing with fat suppression, T1-weighted imaging (T1WI) volumetric interpolated breath-hold examination (VIBE) peripheral fat suppression plain and enhanced scans. The DCE-MRI enhancement scan parameters were as follows: repetition time, 4.1 ms; echo time, 1.41 ms; band width, 400 kHz; slice thickness, 3.6 mm; interval between floors, 1.2 mm; field of view, 380 mm × 380 mm; resolution, 320 mm × 224 mm. Gadoteric acid glucosamine injection (15 mL/5.654 g/vial, 0.2 mmol/kg) administered by push-feeding at a venous rate of 2 mL/s. Thirty seconds after injection of the contrast agent, the pulmonary nodal area was scanned for 30 consecutive cycles with a total scan time of 04:58, during which the patient was instructed to maintain shallow and slow breathing.
Imaging analysis
All raw data were transferred to the image perfusion processing software Tissu 4D (Seimens Medical Systems) on the Seimens Syngo Magnetic Resonance Diagnostics post-processing workstation to measure quantitative parameter values. After artefact correction and image screening, the quantitative T1 baseline value before enhancement was automatically obtained by a computer using multi-reverse angle technology. At the same time, with reference to the conventional T1WI and T2WI images, three regions of interest (ROIs) were manually outlined in different areas of the largest horizontal solid portion of the lesion cross-section, avoiding necrosis, cystic degeneration, hemorrhage, etc. as much as possible (Figure 2). The Tofts Two compartment hemodynamic model of the software automatically calculateed quantitative pharmacokinetic parameters in the ROIs, including Ktrans, Kep, and Ve values, which are related to each other by the relationship: Kep = Ktrans/Ve, and the average of three measurements is taken as the final result. To ensure the accuracy of the results, both examiners had 6 years of experience in chest MRI (L.F. and H.D.) and independently measured the relevant parameters.
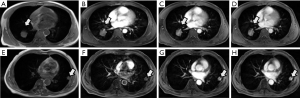
Immunohistochemistry
After surgical excision of the lesions, tissues from nonnecrotic areas were taken as SSPNs pathology tissue specimens and fixed in 10% formalin solution for 24 h. Subsequently, routine dehydration and dewaxing, antigen repair, and other operations were performed, and all specimens were prepared as 4 µm sections. This was done as follows: paraffin embedding was done using a tissue processor, followed by deparaffinization and hydration of the tissue sections, which were fully dried and placed in 0.01 mol/L sodium citrate buffer at pH 6.0 for antigenic repair, after which the sections were washed with phosphate buffered saline (PBS) for 5 min and incubated with 3% H2O2 for 10 min at room temperature. Next, the sections were washed three times with PBS for 5 min each, and then primary antibodies generated against CD31, α-SMA, and VEGF, respectively, were added and incubated overnight in a 4 ℃ refrigerator. On the following day, dropwise addition of the secondary antibody was followed by incubation for 15 min at room temperature. After washing again with PBS dilution, 3,3' diaminobenzidine reagent was added for color development and counterstained with hematoxylin, then dehydrated and sealed. All antibodies and assay kits were provided by Fuzhou Maixin Biotechnology (China).
Immunohistochemistry data interpretation
The resulting sections were connected to a bright-field microscope (model*/BX53+DP80) and imaged at ×40 magnification to observe the homogeneity and consistency of the staining of the entire section. Three hot spots in the section with different fields of view were selected, and three images of each subject were imaged at ×200 magnification to capture the percentage of positive cell staining area and acquire the corresponding microvascular parameter values indirectly by using Image-J software, and the average value was taken as the final measurement. The average value was taken as the final measurement value. MVD was enumerated as reported in the literature by Weidner and Wang and co-labeled by CD31 and SMA antibodies (23,24). CD31-MVD stains the cytomembrane or cytoplasm of vascular endothelial cells with brown granules, which need to be clearly demarcated from adjacent microvessels, and are counted as one microvessel regardless of the presence or absence of erythrocytes, but microvessels with an internal diameter greater than 150 µm are excluded (Figure 3). The hotspot areas selected for CD31 were labeled, and images were acquired in the same field of view on serial SMA-stained sections, and SMA stained for round-like or slit vessels with luminal structures. VEGF-positive cells with staining area less than or equal to 5% were scored as 0, 5–25% were scored as 1, 26–50% were scored as 2, 51–75% were scored as 3, and greater than 75% were scored as 4. The intensity of microscopic staining was 0 for no staining, 1 for light yellow, 2 for brownish yellow, and 3 for tan color. The above two items are multiplied together for the final score, with 0–1 being negative (−), 2–3 being weakly positive (+), 4–6 being moderately positive (++), and 7–12 being strongly positive (+++) (25). All histologic analyses were determined by our hospital pathologist (with more than 10 years of experience).
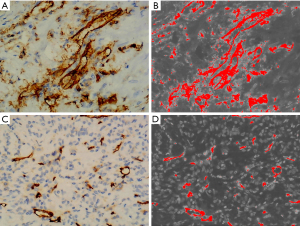
Statistical analysis
Statistical analysis was performed using SPSS 24.0. All data were expressed as the mean ± standard deviation. The Shapiro-Wilk test was used to analyze whether the sample data conformed to normal distribution. The independent samples t-test was used to compare the DCE-MRI quantitative permeability parameters and vascular conformation parameters between subgroups of benign and malignant SSPNs. The diagnostic efficacy of the quantitative parameters for malignant SSPNs was analyzed by plotting the receiver operating characteristic (ROC) curves, and the optimal threshold values of quantitative parameters for predicting malignant SSPNs and the specificity and sensitivity at that threshold were determined. The correlation between DCE-MRI quantitative permeability parameters and angioarchitecture parameters was evaluated by Spearman correlation analysis. A two-sided P<0.05 was determined to be statistically significant.
Results
Patients and characteristics
A total of 79 patients with SSPNs were included in our study, the mean age of the total participants was 50.5±11.5 years, 51 (64.6%) of them were females, with a larger proportion of females (80.0%) in the benign group. The majority of patients never smoked, with 58 (73.4%), 35 (64.8%) and 23 (92.0%) in the three groups, respectively; weight in the normal range was more common, with a total of 30 cases (38.0%); more than half of the patients had lesions in the range of 1–2 cm, with a total of 44 cases (55.7%); the majority of patients with serum carcinoembryonic antigen (CEA) was normal, with a total of 63 (79.7%), of which there were no patients with abnormal CEA values in the benign group; the number of patients with abnormal serum ferritin values were 40 (50.6%), 31 (57.4%) and 9 (36.0%), respectively (Table 1).
Table 1
Characteristics | All patients (n=79) | Malignant patients (n=54) | Benign patients (n=25) |
---|---|---|---|
Age (years) | 50.5±11.5 [18–66] | 52.6±8.8 [35–66] | 45.5± 15.0 [18–66] |
Gender | |||
Male | 28 (35.4) | 23 (42.6) | 5 (20.0) |
Female | 51 (64.6) | 31 (57.4) | 20 (80.0) |
Smoking status | |||
Current/former | 21 (26.6) | 19 (35.2) | 2 (8.0) |
Never | 58 (73.4) | 35 (64.8) | 23 (92.0) |
CEA (ng/mL) | |||
Increased (>5) | 16 (20.3) | 16 (29.6) | 0 (0.0) |
Normal (≤5) | 63 (79.7) | 38 (70.4) | 25 (10.0) |
SF (ng/mL) | |||
Increased (>204) | 40 (50.6) | 31 (57.4) | 9 (36.0) |
Normal (4.63–204) | 39 (49.4) | 23 (42.6) | 16 (64.0) |
Lesion size (cm) | |||
>0 and ≤1 | 0 (0.0) | 0 (0.0) | 0 (0.0) |
>1 and ≤2 | 44 (55.7) | 28 (51.9) | 16 (64.0) |
>2 and ≤3 | 35 (44.3) | 26 (48.1) | 9 (36.0) |
BMI category (kg/m2) | |||
Underweight (<18.5) | 9 (11.4) | 5 (9.3) | 4 (16.0) |
Normal (18.5–22.9) | 30 (38.0) | 21 (38.9) | 9 (36.0) |
Overweight (23.0–24.9) | 19 (24.1) | 14 (25.9) | 5 (20.0) |
Obese (≥25) | 21 (26.6) | 14 (25.9) | 7 (28.0) |
The values are presented as mean ± standard deviation [range] or n (%). CEA, carcinoembryonic antigen; SF, serum ferritin; BMI, body mass index.
Comparison of patients’ quantitative permeability parameters and vascular conformation parameters based on different nature of SSPNs
Quantitative permeability parameters (Ktrans, Kep, and Ve values) were higher in the malignant group than in the benign group, with significant differences in Ktrans and Ve values when comparing the two groups (P<0.001) and no statistically significant difference in Kep values (P=0.28). The vascular construct parameter variables SMA-MVD (P=0.01) and MPI (P<0.001) were statistically significant for the comparison between the benign and malignant SSPNs, and CD31-MVD and VEGF were not significantly different between the two groups (Table 2).
Table 2
Parameters | Malignant patients | Benign patients | t value | P value |
---|---|---|---|---|
Ktrans | 0.257±0.039 | 0.056±0.004 | −5.171 | <0.001 |
Kep | 0.413±0.044 | 0.354±0.031 | −1.087 | 0.28 |
Ve | 0.370±0.029 | 0.184±0.015 | −5.670 | <0.001 |
SMA-MVD | 0.009±0.002 | 0.051±0.010 | 4.143 | 0.01 |
CD31-MVD | 0.091±0.008 | 0.056±0.013 | −1.631 | 0.18 |
VEGF | 0.078±0.011 | 0.052±0.016 | −1.323 | 0.22 |
MPI | 0.135±0.010 | 0.745±0.026 | 18.141 | <0.001 |
Patients | 54 | 25 | – | – |
Values are presented as mean ± standard deviation. Ktrans, volume transfer constant; Kep, rate constant; Ve, extravascular extracellular volume fraction; SMA-MVD, smooth muscle actin microvessel density; CD31-MVD, CD31 microvessel density; VEGF, vascular endothelial growth factor; MPI, microvascular perfusion index.
Patient ROC curve analysis
In the ROC curves of DCE-MRI quantitative parameters for the diagnosis of malignant lung nodules, the area under the curve (AUC) for the Ktrans value was 0.813, with a threshold at diagnosis >0.066/min, and the sensitivity and specificity were 96.3% and 56.0%, respectively; the AUC for the Kep value was 0.510, with a threshold at diagnosis ≤0.292/min, and the sensitivity and specificity were 31.5% and 92.0%, respectively; the AUC for the Ve value was 0.759, with a threshold at diagnosis of >0.212/min, and the sensitivity and specificity were 68.0% and 81.5%, respectively (Table 3, Figure 4).
Table 3
DCE-MRI quantitative parameters | AUC (95% CI) | Diagnostic threshold | Sensitivity (%) | Specificity (%) |
---|---|---|---|---|
Ktrans (/min) | 0.813 (0.709–0.892) | >0.066 | 96.3 | 56.0 |
Kep (/min) | 0.510 (0.395–0.624) | ≤0.292 | 31.5 | 92.0 |
Ve | 0.759 (0.650–0.848) | >0.212 | 68.0 | 81.5 |
DCE-MRI, dynamic contrast-enhanced magnetic resonance imaging; AUC, area under the curve; CI, confidence interval; Ktrans, volume transport constant; Kep, rate constant; Ve, plasma volume fraction.
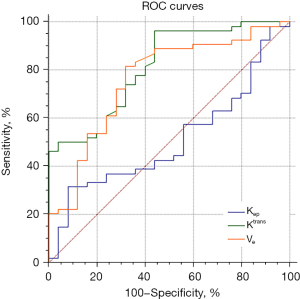
Relationship between quantitative DCE-MRI parameters and vascular architectural parameters
There was a strong positive correlation between Ktrans values and CD31-MVD and VEGF, with correlation coefficients of 0.857 (P<0.001) and 0.851 (P<0.001), respectively; there was a strong negative correlation between Ktrans values and MPI, with a correlation coefficient of −0.779 (P=0.001), which was not statistically different from SMA-MVD. There was also a strong positive correlation between Kep values and CD31-MVD and VEGF, with correlation coefficients of 0.830 (P<0.001) and 0.859 (P<0.001), respectively; there was a significant negative correlation between Kep values and MPI, with a correlation coefficient of −0.864 (P<0.001), which was not significantly different from SMA-MVD. Ve values were strongly positively correlated with CD31-MVD and VEGF, with correlation coefficients of 0.798 (P<0.001) and 0.764 (P<0.001), respectively, and were not statistically significant with either SMA-MVD or MPI (Table 4).
Table 4
DCE-MRI quantitative parameters | SMA-MVD | CD31-MVD | VEGF | MPI |
---|---|---|---|---|
Ktrans (/min) | ||||
r | 0.063 | 0.857 | 0.851 | −0.779 |
P value | 0.79 | <0.001 | <0.001 | 0.001 |
Kep (/min) | ||||
r | −0.041 | 0.830 | 0.859 | −0.864 |
P value | 0.86 | <0.001 | <0.001 | <0.001 |
Ve | ||||
r | 0.138 | 0.798 | 0.764 | −0.446 |
P value | 0.56 | <0.001 | <0.001 | 0.10 |
DCE-MRI, dynamic contrast enhanced magnetic resonance imaging; SMA-MVD, smooth muscle actin microvessel density; CD31-MVD, CD31 microvessel density; VEGF, vascular endothelial growth factor; MPI, microvascular perfusion index; Ktrans, volume transfer constant; Kep, rate constant; Ve, extravascular extracellular volume fraction.
Discussion
Solid tumors rely on neovascularization for growth and metastasis, and the development of SSPNs is also closely related to neovascularization (15). Malignant SSPNs have a haphazard vascular distribution with a lack of muscularis propria resulting in increased permeability and an increased proportion of immature vessels; inflammatory nodules have increased blood flow, but the basement membrane is intact and the increase is in mature vessels; and benign SSPNs are slow-growing and usually have a lack of blood supply. Pathologists have demonstrated that SMA-MVD and MPI reflect the amount and percentage of mature blood vessels, and in our study, malignant SSPNs showed less expression of SMA-MVD and MPI than benign ones, with significant differences in intergroup comparisons, which correlates with the biological characteristics of the lesions, suggesting that cellular proliferation is more active in malignant nodules, and that the neovascularization network formed is predominantly composed of immature vessels. The principle of DCE-MRI imaging is based on the change of vascular permeability in the lesion area, and the change of the concentration of contrast agent in the blood vessels and tissues, which leads to the difference in the measured values of quantitative parameters, indirectly reflecting the angiogenesis, vascular permeability and vascular perfusion (26). Ktrans reflects the vascular permeability of the tissue, Ktrans value increases with the degree of damage to the vascular endothelial cells, Ve value reflects the degree of necrosis of the tissue, and Kep is affected by both, and all three reflect the microcirculatory perfusion of the diseased tissue (27). Currently, DCE-MRI is mainly applied to differential diagnosis, staging and prognosis of breast, prostate and rectum in clinical practice. Ma et al. (28) scholars found that Ktrans and Kep values are significant in identifying the nature of breast occupancy using DCE-MRI, and can also provide a reference for grading invasive ductal carcinoma. Ocak et al (29) confirmed that benign prostate tumors have lower Ktrans and Kep values than malignant tumors. Bloch et al. (30) and Jager et al. (31) reached similar conclusions. Yao et al. (32) found that Ktrans and Kep were statistically significantly different between normal intestinal wall and rectal cancer, and that Ktrans could be used as a prognostic indicator for rectal cancer. DCE-MRI is now also being progressively used in the diagnosis of benign and malignant lung nodules (33). Kumar et al. (34) studied the prediction of malignant SSPNs by DWI and DCE-MRI and found that the Ktrans values had higher diagnostic accuracy and sensitivity. Ktrans and Kep values also have significant advantages over PET/CT parameters for determining the nature of lung lesions (35). Our results showed that Ktrans, Kep, and Ve values were higher in patients with malignant than benign SSPNs, with significant differences between the Ktrans and Ve groups, and the sensitivity and specificity of the diagnosis were higher, which is consistent with the conclusions of most scholars. Although the Kep value has a tendency to increase with the increase in the malignancy of the nodule, it is not statistically significant, the reason for this phenomenon may be related to the small sample size of the two groups, and the number of imbalance, need to increase the sample size for further analysis.
VEGF and CD31-MVD are frequently used to evaluate the level of microvascular development in tumor tissues. The degree of expression of VEGF levels not only reflects the malignancy of the tumor, but also the proliferation and metastasis of the tumor (36). The quantitative parameters Ktrans, Kep and Ve values in our study were closely and significantly correlated with CD31-MVD and VEGF expression. Preda et al. (37) scholars used DCE-MRI to assess quantitative parameter values before and after treatment in a rat soft tissue sarcoma model study, and concluded that Ktrans values can reflect tumor microvascular changes. The study by Meyer et al. (38) similarly concluded that Ktrans values can quantify changes in tumor tissue perfusion. There are a variety of factors in the vasculature that can cause tumor microangiogenesis, and although VEGF is one of the main factors, it is not the only reference index; secondly, after injecting contrast agent, magnetic resonance scanning acquisition of T1WI sequences can be affected by the physicochemical factors of the contrast agent. Although DCE-MRI quantitative permeability parameters do not fully reflect VEGF expression, they still reflect tumor vascular architecture to some extent. The results of this study showed that the MVD values of patients with malignant SSPNs with strongly positive VEGF expression were significantly higher than those with weakly positive or negative VEGF expression, and increased progressively with the increase of VEGF expression, which was the same as the results of the study by Tateishi et al. (39).
It has been well documented that MPI expression level is closely related to the maturity of blood vessels in tumors, and can be used as a direct reflection of the degree of maturity of microvessels, and when its value is larger, it represents a larger proportion of mature blood vessels (40-43). The results of this study confirmed a significant negative correlation between Ktrans and Kep values and tumor MPI expression, suggesting that the mature blood vessels of the lesion gradually decreased with the increase of Ktrans and Kep values. It has also been demonstrated (44,45) that irregular, tortuous, and leaky blood vessels within malignant tumors lead to reduced vascular maturation, in keeping with the views herein. Ve values did not correlate with the vascular maturity parameters SMA-MVD and MPI, and according to the results of the study by Tofts et al. (27), it was concluded that Ve measurements are unstable and may be altered by the effects of peri-lesion edema.
However, there are some limitations in this study. First, the number of patient cases collected was small, the malignant group was significantly larger than the benign group, and the unbalanced grouping may lead to biased results; second, we could not guarantee that the region of interest measured by MRI was exactly the same as the region examined by pathology; therefore, we outlined the ROIs as broadly as possible in order to ensure that the region examined by pathology was included.
Conclusions
Our results showed that there were significant differences in Ktrans, Ve, SMA-MVD and MPI between benign and malignant SSPNs, and that Ktrans and Ve values were highly specific and sensitive for the diagnosis of malignant SSPNs. In addition, some of the permeability parameters were closely correlated with the expression of vascular architectural parameters, providing an objective, noninvasive, and reproducible scientific basis for the assessment of the SSPNs microcirculation.
Acknowledgments
We thank Dr. Chenhui Li, a Siemens engineer, for developing and modifying the MRI scanning protocol for this study.
Funding: This study was supported by
Footnote
Reporting Checklist: The authors have completed the STARD reporting checklist. Available at https://jtd.amegroups.com/article/view/10.21037/jtd-24-1467/rc
Data Sharing Statement: Available at https://jtd.amegroups.com/article/view/10.21037/jtd-24-1467/dss
Peer Review File: Available at https://jtd.amegroups.com/article/view/10.21037/jtd-24-1467/prf
Conflicts of Interest: All authors have completed the ICMJE uniform disclosure form (available at https://jtd.amegroups.com/article/view/10.21037/jtd-24-1467/coif). The authors have no conflicts of interest to declare.
Ethical Statement: The authors are accountable for all aspects of the work in ensuring that questions related to the accuracy or integrity of any part of the work are appropriately investigated and resolved. The study was conducted in accordance with the Declaration of Helsinki (as revised in 2013). The study was approved by the review board of The First Affiliated Hospital of Guangxi Medical University (No. 2024-E232-01) and informed consent was obtained from all individual participants.
Open Access Statement: This is an Open Access article distributed in accordance with the Creative Commons Attribution-NonCommercial-NoDerivs 4.0 International License (CC BY-NC-ND 4.0), which permits the non-commercial replication and distribution of the article with the strict proviso that no changes or edits are made and the original work is properly cited (including links to both the formal publication through the relevant DOI and the license). See: https://creativecommons.org/licenses/by-nc-nd/4.0/.
References
- Zheng RS, Chen R, Han BF, et al. Cancer incidence and mortality in China, 2022. Zhonghua Zhong Liu Za Zhi 2024;46:221-31. [Crossref] [PubMed]
- Sung H, Ferlay J, Siegel RL, et al. Global Cancer Statistics 2020: GLOBOCAN Estimates of Incidence and Mortality Worldwide for 36 Cancers in 185 Countries. CA Cancer J Clin 2021;71:209-49. [Crossref] [PubMed]
- Allemani C, Matsuda T, Di Carlo V, et al. Global surveillance of trends in cancer survival 2000-14 (CONCORD-3): analysis of individual records for 37 513 025 patients diagnosed with one of 18 cancers from 322 population-based registries in 71 countries. Lancet 2018;391:1023-75. [Crossref] [PubMed]
- Zeng H, Chen W, Zheng R, et al. Changing cancer survival in China during 2003-15: a pooled analysis of 17 population-based cancer registries. Lancet Glob Health 2018;6:e555-67. [Crossref] [PubMed]
- Nie P, Yang G, Wang N, et al. Correction to: Additional value of metabolic parameters to PET/CT-based radiomics nomogram in predicting lymphovascular invasion and outcome in lung adenocarcinoma. Eur J Nucl Med Mol Imaging 2021;48:325-7. [Crossref] [PubMed]
- Macchiarini P, Fontanini G, Hardin MJ, et al. Relation of neovascularisation to metastasis of non-small-cell lung cancer. Lancet 1992;340:145-6. [Crossref] [PubMed]
- Kimura Y, Morohashi S, Yoshizawa T, et al. Clinicopathological significance of vascular endothelial growth factor, thymidine phosphorylase and microvessel density in colorectal cancer. Mol Med Rep 2016;13:1551-7. [Crossref] [PubMed]
- Chen Y, Sun Y, Cui Y, et al. High CTHRC1 expression may be closely associated with angiogenesis and indicates poor prognosis in lung adenocarcinoma patients. Cancer Cell Int 2019;19:318. [Crossref] [PubMed]
- Poblet E, Gonzalez-Palacios F, Jimenez FJ. Different immunoreactivity of endothelial markers in well and poorly differentiated areas of angiosarcomas. Virchows Arch 1996;428:217-21. [Crossref] [PubMed]
- Eberhard A, Kahlert S, Goede V, et al. Heterogeneity of angiogenesis and blood vessel maturation in human tumors: implications for antiangiogenic tumor therapies. Cancer Res 2000;60:1388-93.
- Mukhopadhyay D, Datta K. Multiple regulatory pathways of vascular permeability factor/vascular endothelial growth factor (VPF/VEGF) expression in tumors. Semin Cancer Biol 2004;14:123-30. [Crossref] [PubMed]
- He XQ, Huang XT, Luo TY, et al. The differential computed tomography features between small benign and malignant solid solitary pulmonary nodules with different sizes. Quant Imaging Med Surg 2024;14:1348-58. [Crossref] [PubMed]
- Liu Z, Ran H, Yu X, et al. Immunocyte count combined with CT features for distinguishing pulmonary tuberculoma from malignancy among non-calcified solitary pulmonary solid nodules. J Thorac Dis 2023;15:386-98. [Crossref] [PubMed]
- Franchi P, Procaccini L, Mincuzzi E. Role of computed tomography in the diagnosis of solitary pulmonary nodule with solid component: a narrative review. AME Surg J 2022;2:29.
- Folkman J. Tumor angiogenesis: therapeutic implications. N Engl J Med 1971;285:1182-6. [Crossref] [PubMed]
- Wan Q, Zhou J, Xia X, et al. Diagnostic Performance of 2D and 3D T2WI-Based Radiomics Features With Machine Learning Algorithms to Distinguish Solid Solitary Pulmonary Lesion. Front Oncol 2021;11:683587. [Crossref] [PubMed]
- Tang X, Xu X, Han Z, et al. Elaboration of a multimodal MRI-based radiomics signature for the preoperative prediction of the histological subtype in patients with non-small-cell lung cancer. Biomed Eng Online 2020;19:5. [Crossref] [PubMed]
- Wu L, Li J, Fu C, et al. Chemotherapy response of pancreatic cancer by diffusion-weighted imaging (DWI) and intravoxel incoherent motion DWI (IVIM-DWI) in an orthotopic mouse model. MAGMA 2019;32:501-9. [Crossref] [PubMed]
- Shi C, Liu D, Xiao Z, et al. Monitoring Tumor Response to Antivascular Therapy Using Non-Contrast Intravoxel Incoherent Motion Diffusion-Weighted MRI. Cancer Res 2017;77:3491-501. [Crossref] [PubMed]
- Yuan Z, Niu XM, Liu XM, et al. Use of diffusion-weighted magnetic resonance imaging (DW-MRI) to predict early response to anti-tumor therapy in advanced non-small cell lung cancer (NSCLC): a comparison of intravoxel incoherent motion-derived parameters and apparent diffusion coefficient. Transl Lung Cancer Res 2021;10:3671-81. [Crossref] [PubMed]
- Wang Y, Wan Q, Xia X, et al. Value of radiomics model based on multi-parametric magnetic resonance imaging in predicting epidermal growth factor receptor mutation status in patients with lung adenocarcinoma. J Thorac Dis 2021;13:3497-508. [Crossref] [PubMed]
- Feng H, Shi G, Liu H, et al. Free-breathing radial volumetric interpolated breath-hold examination sequence and dynamic contrast-enhanced MRI combined with diffusion-weighted imaging for assessment of solitary pulmonary nodules. Magn Reson Imaging 2021;75:100-6. [Crossref] [PubMed]
- Weidner N. Current pathologic methods for measuring intratumoral microvessel density within breast carcinoma and other solid tumors. Breast Cancer Res Treat 1995;36:169-80. [Crossref] [PubMed]
- Wang HC, Wang BD, Chen MS, et al. An Underlying Pathological Mechanism of Meningiomas with Intratumoral Hemorrhage: Undifferentiated Microvessels. World Neurosurg 2016;94:319-27. [Crossref] [PubMed]
- Wu X, Mao X, Huang Y, et al. Detection of proteins associated with the pyroptosis signaling pathway in breast cancer tissues and their significance. Int J Clin Exp Pathol 2020;13:1408-14.
- Hittmair K, Eckersberger F, Klepetko W, et al. Evaluation of solitary pulmonary nodules with dynamic contrast-enhanced MR imaging--a promising technique. Magn Reson Imaging 1995;13:923-33. [Crossref] [PubMed]
- Tofts PS. Modeling tracer kinetics in dynamic Gd-DTPA MR imaging. J Magn Reson Imaging 1997;7:91-101. [Crossref] [PubMed]
- Ma ZS, Wang DW, Sun XB, et al. Quantitative analysis of 3-Tesla magnetic resonance imaging in the differential diagnosis of breast lesions. Exp Ther Med 2015;9:913-8. [Crossref] [PubMed]
- Ocak I, Bernardo M, Metzger G, et al. Dynamic contrast-enhanced MRI of prostate cancer at 3 T: a study of pharmacokinetic parameters. AJR Am J Roentgenol 2007;189:849. [Crossref] [PubMed]
- Bloch BN, Lenkinski RE, Rofsky NM. The role of magnetic resonance imaging (MRI) in prostate cancer imaging and staging at 1.5 and 3 Tesla: the Beth Israel Deaconess Medical Center (BIDMC) approach. Cancer Biomark 2008;4:251-62. [Crossref] [PubMed]
- Jager GJ, Ruijter ET, van de Kaa CA, et al. Dynamic TurboFLASH subtraction technique for contrast-enhanced MR imaging of the prostate: correlation with histopathologic results. Radiology 1997;203:645-52. [Crossref] [PubMed]
- Yao WW, Zhang H, Ding B, et al. Rectal cancer: 3D dynamic contrast-enhanced MRI; correlation with microvascular density and clinicopathological features. Radiol Med 2011;116:366-74. [Crossref] [PubMed]
- Liu H, Chen R, Tong C, et al. MRI versus CT for the detection of pulmonary nodules: A meta-analysis. Medicine (Baltimore) 2021;100:e27270. [Crossref] [PubMed]
- Kumar N, Sharma M, Aggarwal N, et al. Role of Various DW MRI and DCE MRI Parameters as Predictors of Malignancy in Solid Pulmonary Lesions. Can Assoc Radiol J 2021;72:525-32. [Crossref] [PubMed]
- Feng F, Qiang F, Shen A, et al. Dynamic contrast-enhanced MRI versus (18)F-FDG PET/CT: Which is better in differentiation between malignant and benign solitary pulmonary nodules? Chin J Cancer Res 2018;30:21-30. [Crossref] [PubMed]
- Bekes I, Friedl TW, Köhler T, et al. Does VEGF facilitate local tumor growth and spread into the abdominal cavity by suppressing endothelial cell adhesion, thus increasing vascular peritoneal permeability followed by ascites production in ovarian cancer? Mol Cancer 2016;15:13. [Crossref] [PubMed]
- Preda A, Wielopolski PA, Ten Hagen TL, et al. Dynamic contrast-enhanced MRI using macromolecular contrast media for monitoring the response to isolated limb perfusion in experimental soft-tissue sarcomas. MAGMA 2004;17:296-302. [Crossref] [PubMed]
- Meyer JM, Perlewitz KS, Hayden JB, et al. Phase I trial of preoperative chemoradiation plus sorafenib for high-risk extremity soft tissue sarcomas with dynamic contrast-enhanced MRI correlates. Clin Cancer Res 2013;19:6902-11. [Crossref] [PubMed]
- Tateishi U, Nishihara H, Watanabe S, et al. Tumor angiogenesis and dynamic CT in lung adenocarcinoma: radiologic-pathologic correlation. J Comput Assist Tomogr 2001;25:23-7. [Crossref] [PubMed]
- Lindahl P, Johansson BR, Levéen P, et al. Pericyte loss and microaneurysm formation in PDGF-B-deficient mice. Science 1997;277:242-5. [Crossref] [PubMed]
- Barron L, Gharib SA, Duffield JS. Lung Pericytes and Resident Fibroblasts: Busy Multitaskers. Am J Pathol 2016;186:2519-31. [Crossref] [PubMed]
- Benjamin LE, Golijanin D, Itin A, et al. Selective ablation of immature blood vessels in established human tumors follows vascular endothelial growth factor withdrawal. J Clin Invest 1999;103:159-65. [Crossref] [PubMed]
- Lindahl P, Hellström M, Kalén M, et al. Endothelial-perivascular cell signaling in vascular development: lessons from knockout mice. Curr Opin Lipidol 1998;9:407-11. [Crossref] [PubMed]
- Konerding MA, Miodonski AJ, Lametschwandtner A. Microvascular corrosion casting in the study of tumor vascularity: a review. Scanning Microsc 1995;9:1233-43; discussion 1243-4.
- Dvorak HF. Tumors: wounds that do not heal. Similarities between tumor stroma generation and wound healing. N Engl J Med 1986;315:1650-9.