Causal relationship between type II diabetes mellitus, metformin, insulin, gliclazide, and esophageal cancer—insights from two-sample Mendelian randomization study and meta-analysis
Highlight box
Key findings
• A genetic predisposition to type II diabetes mellitus (T2DM) is associated with a reduced risk of esophageal cancer (EC).
• Metformin use is linked to a decreased prevalence of EC, while insulin and gliclazide show no significant association.
What is known and what is new?
• Existing knowledge: previous studies have produced mixed results regarding the relationship between T2DM and the risk of EC, with some suggesting potential links to specific medications.
• This study: utilizes a two-sample Mendelian randomization approach combined with meta-analysis to clarify the causal associations between T2DM, its treatments, and the risk of EC.
What is the implication, and what should change now?
• The findings suggest that metformin may offer protective benefits against EC, warranting further investigation into its mechanisms.
Introduction
Diabetes mellitus (DM) constitutes a substantial global health challenge. Over the past three decades, its incidence has witnessed a dramatic surge, ranking ninth among the leading causes of global mortality. Epidemiological evidence reveals that DM affects approximately one in every eleven adults worldwide, with type II DM (T2DM) accounting for 90% of these cases (1). This chronic ailment imposes a substantial burden, both in terms of personal suffering and societal costs.
Esophageal cancer (EC) stands out for its high mortality rate and poor prognosis at the time of diagnosis. Recent secondary analysis of global cancer statistics for 2020 underscores the gravity of the issue, with EC ranking tenth in terms of morbidity and sixth in terms of mortality worldwide (2). Approximately 50,000 deaths worldwide are attributed to EC, representing 5.3% of all global cancer-related fatalities (3). EC has two distinct histological subtypes, and with the increase in esophageal adenocarcinoma (EAC) and the decline in esophageal squamous cell carcinoma (ESCC), the incidence of EAC now accounts for 30% to 50% of cases in Western Europe (4).
Numerous epidemiological observational studies have hinted at a correlation between T2DM and the risk of various cancers, including hepatic, colorectal, and breast cancer (5-8). However, the causal relationship between T2DM and cancer remains enigmatic due to inherent limitations, including potential confounding factors, reverse causality, and various biases in observational studies. Mendelian randomization (MR) study presents an innovative approach utilizing single-nucleotide polymorphisms (SNPs) as instrumental variables (IVs) to determine causal relationships between multiple exposures and outcomes (9). Unlike traditional observational studies, MR minimizes potential confounders and mitigates reverse causality by virtue of the random assortment of genotypes during meiosis (10).
Despite the significance of the topic, relatively few MR studies have explored the causal link between T2DM and EC. Earlier MR investigations have suggested that genetic predisposition to T2DM might decrease the risk of EC (11), a finding inconsistent with some published meta-analyses (12,13). Furthermore, reports have emerged highlighting the potential benefits of metformin in cancer prevention and treatment (14,15). Nonetheless, few studies have delved into the associations between metformin and EC.
Given the high prevalence of T2DM, the substantial mortality associated with EC, and the limited clarity regarding their potential relationship, this study aims to explore the causal association between T2DM and the risk of EC. Additionally, it seeks to evaluate the effects of three commonly prescribed T2DM management drugs—metformin, insulin, and gliclazide—on the risk of developing EC. This investigation is conducted with the goal of providing further insights into the interplay between T2DM, its treatment, and EC risk. We present this article in accordance with the STROBE-MR reporting checklist (available at https://jtd.amegroups.com/article/view/10.21037/jtd-24-1152/rc).
Methods
Selection of IVs from exposure data
The study was conducted in accordance with the Declaration of Helsinki (as revised in 2013). Data from genome-wide association studies (GWAS) pertaining to each exposure and EC were sourced from the Integrative Epidemiology Unit (IEU) Open GWAS Project (https://gwas.mrcieu.ac.uk/), a publicly accessible database. In our preliminary MR analysis, we employed SNPs extracted from GWAS data pertaining to the exposure as IVs. The selection of IVs was contingent upon adherence to three fundamental assumptions (16) (Figure 1):
- Relevance to exposure: the IVs must exhibit significant associations with the exposure in question;
- Exclusivity to the exposure-outcome pathway: the IVs should exclusively influence the outcome through their impact on the exposure, ensuring a causal relation between the two;
- Independence from unobserved confounders: the IVs should remain independent to unobserved potential confounding factors that were associated with both the exposure and the outcome.
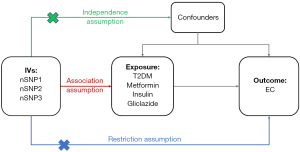
To ensure the robustness of IV selection, a series of quality control procedures were implemented:
- Significance threshold: a stringent significance threshold of P value (P<5 ×108) was applied to identify SNPs reliably associated with the exposures.
- Linkage disequilibrium (LD) analysis: recognizing the critical role of LD in genetic studies, we took measures to mitigate potential confounding arising from LD. A clumping process was employed, where SNPs in LD with each other were excluded. Specifically, a threshold of the LD correlation coefficient (r2) less than 0.001 was set, and SNPs within a genomic distance of less than 10,000 kilobases were removed to ensure the selected IVs were not in LD.
- Minor allele frequency (MAF) threshold: data of the selected SNPs from the outcome trait (EC) summary-level GWAS data were extracted. A MAF threshold of 0.01 was established for SNPs in the outcome data. Proxies were not employed for SNPs that were absent in the outcome data, maintaining the integrity of the selection process.
MR analysis
Following the preparatory steps, we had successfully curated GWAS data for the exposures and the outcome and identified eligible IVs from the pool of SNPs. To proceed with MR study, we harmonized the effects of SNPs on both the outcome and exposure alleles. Subsequently, MR analysis was conducted, encompassing various diagnostic tests.
Methods for MR analysis
Three distinct methods were employed to ascertain the causal estimates between the exposures (T2DM, metformin, insulin, gliclazide) and the outcome (EC).
- The inverse variance weighted (IVW) method: the IVW method, which assumes uncorrelated IVs, provided optimal statistical power for estimating causal effects;
- MR-Egger: a method free from pleiotropic effects, offered unbiased estimates in the presence of pleiotropy;
- The weighted median method: a method robust against outliers. It also yielded unbiased estimates when any one of the three core assumptions was violated (17).
Given the stringent quality control measures applied during IV selection, there were compelling evidence of IV’s independence, enabling the utilization of IVW as the primary method for the primary effect estimate in our MR study (18).
Sensitivity analysis
Cochran’s Q test was employed to quantify potential heterogeneity, while the MR-Egger intercept test was utilized to estimate horizontal pleiotropy among the selected SNPs. A P value below 0.05 was indicative of potential heterogeneity and horizontal pleiotropy, respectively (19). If heterogeneity was detected among the selected IVs, the random effects IVW analysis was applied (20). Leave-one-out plots were also used to evaluate horizontal pleiotropy.
Visualization for the results of MR analysis
Scatter plots were employed to visualize the associations between the exposures and the outcome using the MR methods.
Meta-analysis
A random-effect model of meta-analysis was implemented to evaluate the collective impact of each trait on EC subsequently, predicated on the IVW method. A visual representation of the overall study flow is presented in Figure 2.
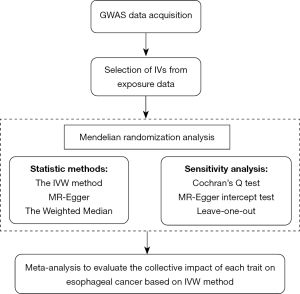
Statistical analysis
The MR analysis was executed utilizing the TwoSampleMR package, and meta-analysis was carried out using the metafor package in R software (version 4.2.3). A significance threshold of P value <0.05 was established, unless otherwise specified.
Results
The selected SNPs as IVs
On account of the rigorous quality controls, all independent SNPs in each GWAS dataset were identified for use as effective IVs. The details of selected SNPs are presented in Table 1.
Table 1
Traits | Dataset | Data source | Population | Sample size | Number of SNPs |
---|---|---|---|---|---|
T2DM | finn-b-E4_DM2 | FinnGen | European | 215,654 | 16,380,440 |
finn-b-T2D | FinnGen | European | 211,766 | 16,380,433 | |
finn-b-E4_DM2_STRICT | FinnGen | European | 212,351 | 16,380,434 | |
finn-b-T2D_WIDE | FinnGen | European | 202,056 | 16,380,418 | |
ebi-a-GCST005413 | UK Biobank | European | 70,100 | 14,277,791 | |
ebi-a-GCST006867 | UK Biobank | European | 62,892 | 5,030,727 | |
ebi-a-GCST007515 | UK Biobank | European | 298,957 | 190,486 | |
Metformin | ukb-b-14609 | MRC-IEU | European | 462,933 | 9,851,867 |
ukb-a-159 | Neale Lab | European | 337,159 | 10,894,596 | |
Insulin | ukb-b-14070 | MRC-IEU | European | 249,710 | 9,851,867 |
ukb-a-491 | Neale Lab | European | 154,702 | 10,894,596 | |
Gliclazide | ukb-b-8602 | MRC-IEU | European | 462,933 | 9,851,867 |
ukb-a-139 | Neale Lab | European | 337,159 | 10,894,596 | |
EC | ieu-b-4960 | UK Biobank | European | 372,756 | 8,970,465 |
SNP, single-nucleotide polymorphism; T2DM, type II diabetes mellitus; UK, United Kingdom; MRC-IEU, Medical Research Council Integrative Epidemiology Unit; EC, esophageal cancer.
The heterogeneity test and pleiotropy test
Cochran’s Q test indicated the absence of heterogeneity among the selected SNPs in all GWAS datasets, apart from the GWAS dataset “ebi-a-GCST006867” (Q-statistics =137.2297, P=0.01). Consequently, we specifically applied the random-effects IVW as the primary method in the MR analysis of the data in “ebi-a-GCST006867”, while employing the fixed-effects IVW for the remaining datasets.
The MR-Egger intercept test did not unveil significant pleiotropy in any of the MR analyses. Heterogeneity and the MR-Egger intercept test results for each trait concerning EC are presented in Table 2.
Table 2
Traits | Dataset | SNPs | Heterogeneity test | Pleiotropy test | |||
---|---|---|---|---|---|---|---|
Cochran Q-statistics | P | MR-Egger intercept | P | ||||
T2DM | finn-b-E4_DM2 | 52 | 49.718 | 0.52 | −2.470E−05 | 0.52 | |
finn-b-T2D | 46 | 37.014 | 0.80 | 1.408E−05 | 0.74 | ||
finn-b-E4_DM2_STRICT | 49 | 50.341 | 0.38 | 4.277E−05 | 0.94 | ||
finn-b-T2D_WIDE | 24 | 19.979 | 0.64 | 6.346E−05 | 0.30 | ||
ebi-a-GCST005413 | 15 | 19.305 | 0.15 | 6.838E−05 | 0.48 | ||
ebi-a-GCST006867 | 103 | 137.230 | 0.01 | 2.733E−05 | 0.36 | ||
ebi-a-GCST007515 | 64 | 69.501 | 0.27 | 2.366E−05 | 0.51 | ||
Metformin | ukb-b-14609 | 43 | 46.887 | 0.28 | 3.199E−05 | 0.52 | |
ukb-a-159 | 21 | 24.212 | 0.23 | 2.517E−05 | 0.72 | ||
Insulin | ukb-b-14070 | 4 | 1.821 | 0.61 | 1.268E−05 | 0.50 | |
ukb-a-491 | 7 | 6.170 | 0.40 | 1.322E−05 | 0.93 | ||
Gliclazide | ukb-b-8602 | 8 | 11.776 | 0.11 | 1.215E−05 | 0.29 | |
ukb-a-139 | 4 | 2.333 | 0.51 | 8.751E−05 | 0.54 |
MR, Mendelian randomization; EC, esophageal cancer; SNP, single-nucleotide polymorphism; T2DM, type II diabetes mellitus.
The causality between T2DM and EC
Among the GWAS data related to T2DM, only four datasets—“finn-b-E4_DM2” [odds ratio (OR), 0.999528; 95% confidence interval (CI): 0.999187–0.99987; P=0.007], “finn-b-T2D” (OR, 0.999503; 95% CI: 0.999154–0.999852; P=0.005), “finn-b-E4_DM2_STRICT” (OR, 0.999506; 95% CI: 0.999153–0.999860; P=0.006), and “finn-b-T2D_WIDE” (OR, 0.999554; 95% CI: 0.999142–0.999967; P=0.03) exhibited statistical significance. Effect estimates (β-values) computed via the three methods for T2DM datasets were consistent indirection (Table 3). The consistent trends were reflected visually in scatter plots among the three methods (Figure 3). The meta-analysis of causal estimates derived from IVW can be seen in Figure 4. It underscored a significant causal link between T2DM and EC, with T2DM being associated with a reduced incidence of EC (OR, 0.999612; 95% CI: 0.999468–0.999756; P=0.01; I2=0%).
Table 3
Traits | GWAS.ID | Method | β-value | OR | 95% CI | P | |
---|---|---|---|---|---|---|---|
LCI | UCI | ||||||
T2DM | finn-b-E4_DM2 | MR-Egger | −2.560E−04 | 0.999744 | 0.999011 | 1.000476 | 0.50 |
Weighted median | −4.800E−04 | 0.999520 | 0.998890 | 1.000151 | 0.14 | ||
IVW | −4.720E−04 | 0.999528 | 0.999187 | 0.999870 | 0.007 | ||
finn-b-T2D | MR-Egger | −6.171E−04 | 0.999383 | 0.998609 | 1.000158 | 0.13 | |
Weighted median | −4.772E−04 | 0.999523 | 0.998909 | 1.000137 | 0.12 | ||
IVW | −4.976E−04 | 0.999503 | 0.999154 | 0.999852 | 0.005 | ||
finn-b-E4_DM2_STRICT | MR-Egger | −2.560E−04 | 0.999476 | 0.998673 | 1.000281 | 0.21 | |
Weighted median | −4.800E−04 | 0.999520 | 0.998911 | 1.000130 | 0.12 | ||
IVW | −4.720E−04 | 0.999506 | 0.999153 | 0.999860 | 0.006 | ||
finn-b-T2D_WIDE | MR-Egger | −9.160E−04 | 0.999084 | 0.998128 | 1.000042 | 0.07 | |
Weighted median | −5.120E−04 | 0.999488 | 0.998865 | 1.000111 | 0.11 | ||
IVW | −4.460E−04 | 0.999554 | 0.999142 | 0.999967 | 0.03 | ||
ebi-a-GCST005413 | MR-Egger | −7.340E−04 | 0.999265 | 0.998115 | 1.000417 | 0.23 | |
Weighted median | −5.250E−04 | 0.999475 | 0.998945 | 1.000005 | 0.050 | ||
IVW | −3.390E−04 | 0.999660 | 0.999211 | 1.000109 | 0.14 | ||
ebi-a-GCST006867 | MR-Egger | −3.997E−04 | 0.999600 | 0.998806 | 1.000396 | 0.33 | |
Weighted median | −4.446E−04 | 0.999556 | 0.998996 | 1.000115 | 0.12 | ||
IVW | −6.937E−04 | 0.999931 | 0.999570 | 1.000292 | 0.71 | ||
ebi-a-GCST007515 | MR-Egger | −6.790E−04 | 0.999322 | 0.998288 | 1.000356 | 0.20 | |
Weighted median | −5.300E−04 | 0.999470 | 0.998705 | 1.000236 | 0.18 | ||
IVW | −3.630E−04 | 0.999637 | 0.999181 | 1.000094 | 0.12 | ||
Metformin | ukb-b-14609 | MR-Egger | −1.920E−02 | 0.98094 | 0.94896 | 1.01401 | 0.26 |
Weighted median | −1.610E−02 | 0.98404 | 0.96341 | 1.00512 | 0.14 | ||
IVW | −9.270E−03 | 0.99077 | 0.97794 | 1.00377 | 0.16 | ||
ukb-a-159 | MR-Egger | −2.030E−02 | 0.97991 | 0.94318 | 1.01807 | 0.31 | |
Weighted median | −1.640E−02 | 0.98378 | 0.96188 | 1.00618 | 0.16 | ||
IVW | −1.380E−02 | 0.98627 | 0.97075 | 1.00204 | 0.09 | ||
Insulin | ukb-b-14070 | MR-Egger | −1.084E−03 | 0.98922 | 0.93526 | 1.04629 | 0.74 |
Weighted median | 8.925E−04 | 1.00089 | 0.97219 | 1.03044 | 0.95 | ||
IVW | 9.759E−03 | 1.00981 | 0.98373 | 1.03657 | 0.47 | ||
ukb-a-491 | MR-Egger | −8.600E−03 | 0.99143 | 0.94666 | 1.03833 | 0.73 | |
Weighted median | −2.760E−04 | 1.00028 | 0.97825 | 1.02279 | 0.98 | ||
IVW | −6.500E−03 | 0.99353 | 0.97671 | 1.01063 | 0.46 | ||
Gliclazide | ukb-b-8602 | MR-Egger | −7.330E−02 | 0.92931 | 0.82157 | 1.05118 | 0.29 |
Weighted median | −2.900E−02 | 0.97145 | 0.91661 | 1.02956 | 0.33 | ||
IVW | −9.520E−03 | 0.99052 | 0.93318 | 1.05138 | 0.75 | ||
ukb-a-139 | MR-Egger | −6.150E−02 | 0.92931 | 0.84593 | 1.04539 | 0.37 | |
Weighted median | −3.210E−02 | 0.97145 | 0.91705 | 1.02255 | 0.25 | ||
IVW | −2.660E−02 | 0.99052 | 0.92706 | 1.02286 | 0.29 |
MR, Mendelian randomization; T2DM, type II diabetes mellitus; GWAS, genome-wide association studies; OR, odds ratio; CI, confidence interval; LCI, lower 95% CI; UCI, upper 95% CI; IVW, inverse variance weighted.
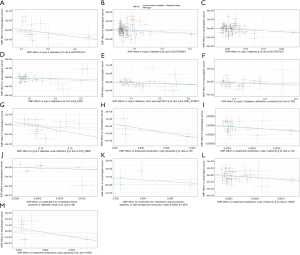
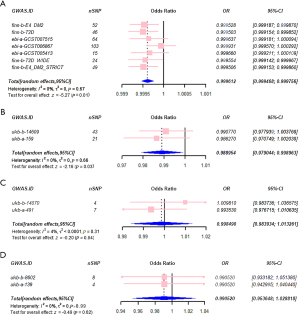
The causality between drug uses and EC
Among the outcomes of MR analysis, the CIs for each dataset pertaining to drug use encompassed the value of 1, and the associated P values exceeded 0.05. Results derived from the weighted median and MR-Egger methods did not provide support for causality between any of the traits and EC, with all P values exceeding 0.05 in MR analyses conducted using these two methods. β-values were consistent in direction, except for the dataset “ukb-b-14070” (Table 3). Scatter plots visually illustrating the MR analyses revealed consistent trends, except for “ukb-b-14070” (Figure 3). The meta-analysis of causal estimates derived from IVW shows metformin was causally related to a reduced incidence of EC (OR, 0.988954; 95% CI: 0.979044–0.998963; P=0.03; I2=0%), while the insulin and gliclazide are insignificant related to EC (Figure 4).
Discussion
In this study, our objective was to investigate the potential causal effects of T2DM, metformin, insulin, and gliclazide use on the risk of EC. We employed a combination of two-sample MR and meta-analysis to address this research question. The findings of our study indicated that the genetic liability for T2DM might be associated with a reduced risk of EC. Additionally, the use of metformin was found to have a causal effect on a decreased risk of EC, while there was no evidence of a causal correlation between insulin or gliclazide use and EC. Previous studies exploring the relationship between T2DM and EC have yielded inconsistent results. Some meta-analyses have reported a positive correlation between T2DM and EC (12,13), but these findings may be less reliable due to potential confounding factors, reverse causal effects, and heterogeneity among the included studies. Conversely, a large cohort study of black and white U.S. veterans demonstrated a decreased risk of EC among individuals with DM (21). Our study supports the conclusion that T2DM has a causal effect on a decreased risk of EC, although it is important to note that the protective effect of T2DM against EC is limited. These discrepancies emphasize the need for further research to investigate the effect of T2DM on EC.
Regarding the effect of drug on EC, our findings suggest that metformin has a protective role, while insulin and gliclazide do not. Numerous clinical studies have been conducted over the years to explore the potential impact of metformin on cancer risk. Our results align with previous cohort studies based on population-based cohort analyses (21,22), strengthening the evidence of a causal relationship between metformin use and a decreased risk of EC. However, the specific mechanism by which metformin influences the development of EC remains unclear, despite extensive studies investigating its potential biological mechanisms. Metformin may improve insulin resistance and modulate hyperinsulinemia through intricate cellular signaling pathways (23,24), such as interrupting insulin binding to the insulin receptor or the insulin-like growth factor-1 receptor and activating the 5'-adenosine monophosphate-activated protein kinase (AMPK) pathway. Our study provides supporting evidence for future research on the potential value of metformin use in the prevention and treatment of EC.
There are several strengths to our study. First, we employed a two-sample MR approach, which allows for causal inference using genetic instruments, minimizing the impact of unmeasured confounding and reverse causality compared to traditional observational studies. Second, we conducted a meta-analysis based on the causal estimates of IVW, which increased the sample size and enabled a more accurate estimation of causality. Third, all the GWAS data used in our study were derived from individuals of European ancestry, which reduces the influence of population stratification. Fourth, we implemented a series of quality control measures, including setting thresholds for the selection of IVs, conducting sensitivity analyses, and assessing heterogeneity, to minimize bias and enhance the robustness of our results.
However, there are some limitations in this study that should be acknowledged. First, our analyses relied solely on summary GWAS data from individuals of European ancestry, which limits the generalizability of our findings to other racial and ethnic groups. Therefore, caution should be exercised when applying our results to populations of different ancestries. Second, it is possible that there was data overlap in the aggregated data used in the MR study. Unfortunately, we did not have measures to estimate the extent of data overlap in our study. Third, each MR method employed in our study has its own strengths and limitations, leading to inconsistent results among the three methods used. While we detected heterogeneity and pleiotropy in the selected SNPs to support the use of IVW as the primary approach, these factors may still introduce some degree of uncertainty into our results. Fourth, some GWAS datasets included a relatively small number of selected SNPs, but we addressed this limitation by conducting a meta-analysis to compensate for the shortage of SNPs in certain traits. Finally, EC can be classified into two main histopathological subtypes: ESCC and EAC. However, there are few GWAS cohorts that focus exclusively on either subtype. The GWAS cohort used in this study for EC is based on a European population, where the incidence of EAC ranges from 30% to 50% (4). Therefore, we consider that the results from this cohort reflect both subtypes Previous observation studies have shown that individuals with diabetes have a moderately increased risk of EC, with a stronger association observed for EAC (5,25,26). To improve the design of our study, future investigations can consider conducting a subtype analysis to determine the specific causal effects of exposures on ESCC and EAC, respectively.
Conclusions
The findings of our research corroborate the hypothesis that a causal relationship exists between T2DM and a diminished incidence of EC. A causative linkage between metformin uses and reduced EC risk has been validated. However, no causal associations were observed for both insulin and gliclazide in relation to EC risk. Future population-based and mechanistic studies are imperative to further elucidate the intricate interplay between these multiple exposures and EC.
Acknowledgments
The authors express their gratitude to all the members affiliated with the Key Laboratory of Ministry of Education for Gastrointestinal Cancer of Fujian Medical University.
Funding: This work was supported by
Footnote
Reporting Checklist: The authors have completed the STROBE-MR reporting checklist. Available at https://jtd.amegroups.com/article/view/10.21037/jtd-24-1152/rc
Peer Review File: Available at https://jtd.amegroups.com/article/view/10.21037/jtd-24-1152/prf
Conflicts of Interest: All authors have completed the ICMJE uniform disclosure form (available at https://jtd.amegroups.com/article/view/10.21037/jtd-24-1152/coif). The authors have no conflicts of interest to declare.
Ethical Statement: The authors are accountable for all aspects of the work in ensuring that questions related to the accuracy or integrity of any part of the work are appropriately investigated and resolved. The study was conducted in accordance with the Declaration of Helsinki (as revised in 2013).
Open Access Statement: This is an Open Access article distributed in accordance with the Creative Commons Attribution-NonCommercial-NoDerivs 4.0 International License (CC BY-NC-ND 4.0), which permits the non-commercial replication and distribution of the article with the strict proviso that no changes or edits are made and the original work is properly cited (including links to both the formal publication through the relevant DOI and the license). See: https://creativecommons.org/licenses/by-nc-nd/4.0/.
References
- Zheng Y, Ley SH, Hu FB. Global aetiology and epidemiology of type 2 diabetes mellitus and its complications. Nat Rev Endocrinol 2018;14:88-98. [Crossref] [PubMed]
- Cao W, Chen HD, Yu YW, et al. Changing profiles of cancer burden worldwide and in China: a secondary analysis of the global cancer statistics 2020. Chin Med J (Engl) 2021;134:783-91. [Crossref] [PubMed]
- Sung H, Ferlay J, Siegel RL, et al. Global Cancer Statistics 2020: GLOBOCAN Estimates of Incidence and Mortality Worldwide for 36 Cancers in 185 Countries. CA Cancer J Clin 2021;71:209-49. [Crossref] [PubMed]
- Shikata K, Ninomiya T, Kiyohara Y. Diabetes mellitus and cancer risk: review of the epidemiological evidence. Cancer Sci 2013;104:9-14. [Crossref] [PubMed]
- Huang W, Ren H, Ben Q, et al. Risk of esophageal cancer in diabetes mellitus: a meta-analysis of observational studies. Cancer Causes Control 2012;23:263-72. [Crossref] [PubMed]
- Pang Y, Kartsonaki C, Turnbull I, et al. Diabetes, Plasma Glucose, and Incidence of Fatty Liver, Cirrhosis, and Liver Cancer: A Prospective Study of 0.5 Million People. Hepatology 2018;68:1308-18. [Crossref] [PubMed]
- Larsson SC, Orsini N, Wolk A. Diabetes mellitus and risk of colorectal cancer: a meta-analysis. J Natl Cancer Inst 2005;97:1679-87. [Crossref] [PubMed]
- Liao S, Li J, Wei W, et al. Association between diabetes mellitus and breast cancer risk: a meta-analysis of the literature. Asian Pac J Cancer Prev 2011;12:1061-5. [PubMed]
- Davey Smith G, Hemani G. Mendelian randomization: genetic anchors for causal inference in epidemiological studies. Hum Mol Genet 2014;23:R89-98. [Crossref] [PubMed]
- Aikens RC, Zhao W, Saleheen D, et al. Systolic Blood Pressure and Risk of Type 2 Diabetes: A Mendelian Randomization Study. Diabetes 2017;66:543-50. [Crossref] [PubMed]
- Yuan S, Kar S, Carter P, et al. Is Type 2 Diabetes Causally Associated With Cancer Risk? Evidence From a Two-Sample Mendelian Randomization Study. Diabetes 2020;69:1588-96. [Crossref] [PubMed]
- Xu B, Zhou X, Li X, et al. Diabetes mellitus carries a risk of esophageal cancer: A meta-analysis. Medicine (Baltimore) 2017;96:e7944. [Crossref] [PubMed]
- Zhou R, Huang C, Luo Z, et al. The Association between the Risk of Esophageal Cancer and Type 2 Diabetes Mellitus: An Updated Meta-Analysis. Biomed Res Int 2022;2022:8129771. [Crossref] [PubMed]
- Morales DR, Morris AD. Metformin in cancer treatment and prevention. Annu Rev Med 2015;66:17-29. [Crossref] [PubMed]
- Quinn BJ, Kitagawa H, Memmott RM, et al. Repositioning metformin for cancer prevention and treatment. Trends Endocrinol Metab 2013;24:469-80. [Crossref] [PubMed]
- Lin L, Luo P, Yang M, et al. Causal relationship between osteoporosis and osteoarthritis: A two-sample Mendelian randomized study. Front Endocrinol (Lausanne) 2022;13:1011246. [Crossref] [PubMed]
- Zhao SS, Holmes MV, Zheng J, et al. The impact of education inequality on rheumatoid arthritis risk is mediated by smoking and body mass index: Mendelian randomization study. Rheumatology (Oxford) 2022;61:2167-75. [Crossref] [PubMed]
- Luo Q, Chen J, Qin L, et al. Psoriasis may increase the risk of lung cancer: a two-sample Mendelian randomization study. J Eur Acad Dermatol Venereol 2022;36:2113-9. [Crossref] [PubMed]
- Huang S, Huang F, Mei C, et al. Systemic lupus erythematosus and the risk of cardiovascular diseases: A two-sample Mendelian randomization study. Front Cardiovasc Med 2022;9:896499. [Crossref] [PubMed]
- Yuan S, Kim JH, Xu P, et al. Causal association between celiac disease and inflammatory bowel disease: A two-sample bidirectional Mendelian randomization study. Front Immunol 2022;13:1057253. [Crossref] [PubMed]
- Wang QL, Santoni G, Ness-Jensen E, et al. Association Between Metformin Use and Risk of Esophageal Squamous Cell Carcinoma in a Population-Based Cohort Study. Am J Gastroenterol 2020;115:73-8. [Crossref] [PubMed]
- Tseng CH. Metformin and esophageal cancer risk in Taiwanese patients with type 2 diabetes mellitus. Oncotarget 2017;8:18802-10. [Crossref] [PubMed]
- Joo MK, Park JJ, Chun HJ. Additional Benefits of Routine Drugs on Gastrointestinal Cancer: Statins, Metformin, and Proton Pump Inhibitors. Dig Dis 2018;36:1-14. [Crossref] [PubMed]
- Luo Z, Zang M, Guo W. AMPK as a metabolic tumor suppressor: control of metabolism and cell growth. Future Oncol 2010;6:457-70. [Crossref] [PubMed]
- Zhang J, Wu H, Wang R. Metabolic syndrome and esophageal cancer risk: a systematic review and meta‑analysis. Diabetol Metab Syndr 2021;13:8. [Crossref] [PubMed]
- Dixon JL, Copeland LA, Zeber JE, et al. Association between diabetes and esophageal cancer, independent of obesity, in the United States Veterans Affairs population. Dis Esophagus 2016;29:747-51. [Crossref] [PubMed]