Epidemiology dynamic of common respiratory virus in spring, 2018–2023 in Guangdong Province, China
Respiratory pathogens represent a substantial concern within the domain of public health, given their capacity to induce severe illnesses and even fatal outcomes (1). Recent years have witnessed the emergence of previously unknown infectious agents affecting the respiratory tract. Notably, the global repercussions of the coronavirus disease 2019 (COVID-19) pandemic serve as a striking example, bringing about profound economic and societal impacts (2,3). Consequently, it becomes imperative to undertake vigilant monitoring and meticulous analysis of the prevalence of conventional respiratory pathogens. This process is fundamental in shaping effective strategies for prevention and treatment. Within the scope of this study, we concentrate on examining the dataset pertaining to the detection of respiratory pathogens. Specifically, our focus is on data from spring, recognized for their propensity for respiratory illness incidence, spanning from 2018 to 2023 in Guangdong Province, China. Our objective is to scrutinize the variation in positive detection rates of respiratory pathogens during the months of April through June across these years. Given that the months of April to June consistently fall within the high-incidence period for respiratory diseases in southern China (4,5), we extracted data for these months each year for monitoring purposes. The insights derived from this analysis are intended to provide valuable guidance for informed decision-making within the realm of public health.
From January 2018 to June 2023, KingMed Diagnostics (KMD) conducted an extensive collection of 589,086 respiratory samples across 331 healthcare facilities, including hospitals, maternal and child health care centers, and community health service centers in Guangdong Province, China. These samples encompassed a range of specimen types, such as nasal and pharyngeal swabs, bronchoalveolar lavage fluid, oral secretions, sputum, pleural or peritoneal fluid, and were subjected to comprehensive testing for various respiratory pathogens. The pathogens under investigation included adenovirus (ADV), influenza A virus (IFA), influenza B virus (IFB), human metapneumovirus (HMPV), parainfluenza virus 1/2/3 (PIV1/2/3), rhinovirus (RHV), and respiratory syncytial virus (RSV). Participants in this study encompassed individuals from diverse age groups, including newborns, infants, children, adolescents, adults, and the elderly.
According to our research findings, we have identified some trends in pathogen detection (Figure 1). Firstly, the overall positive detection rate reached its highest level (19.4%) in 2019 and dropped to its lowest in 2022 (7.7%). This may be related to the implementation of public health measures during the COVID-19 pandemic (6) and the interactions between pathogens (7). Secondly, in the period from April to June of 2018, the positive detection rate for RSV was the highest, reaching 33.3%, while in 2019, ADV had the highest positive detection rate at 32.7%. With the introduction of testing for IFA, IFB, and PIV1/2/3 in 2021, we observed different trends. In that year, the positive detection rate for RHV was the highest at 21.5%, followed by IFA at 21.3% in 2022.
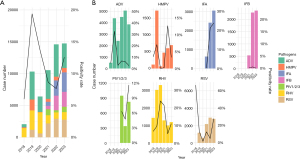
Among the various pathogen detection projects, there was a noticeable decrease in positive detection rates in 2020. Over time, except for PIV1/2/3, ADV, IFA, RHV, and RSV showed varying degrees of increasing trends, possibly reflecting seasonal variations (8) in pathogen prevalence and changes in population immunity (9). Particularly noteworthy is the peak in HMPV detection in 2022, which may be related to the seasonal prevalence of this pathogen. Additionally, IFA detection rates showed a continuous upward trend, warranting close attention in future monitoring data. These results contribute to a better understanding of pathogen trends and provide important information for epidemic prevention and control.
Overall, these findings provide valuable insights into pathogen epidemiology, guiding efforts in disease prevention and control. This research contributes to our understanding of pathogen trends and offers critical information for public health interventions and strategies. Future studies should continue to monitor and analyze these trends to adapt and refine our approaches in combating infectious diseases.
Acknowledgments
None.
Funding: This work was supported by
Footnote
Provenance and Peer Review: This article was commissioned by the editorial office, Journal of Thoracic Disease, for the series “Thoracic Diseases and Big Data”. The article has undergone external peer review.
Peer Review File: Available at https://jtd.amegroups.com/article/view/10.21037/jtd-23-1638/prf
Conflicts of Interest: All authors have completed the ICMJE uniform disclosure form (available at https://jtd.amegroups.com/article/view/10.21037/jtd-23-1638/coif). The series “Thoracic Diseases and Big Data” was commissioned by the editorial office without any funding or sponsorship. Z.Y. served as the unpaid Guest Editor of the series. Y.L. is employed by Guangzhou KingMed Center for Clinical Laboratory Co., Ltd. The authors have no other conflicts of interest to declare.
Ethical Statement: The authors are accountable for all aspects of the work in ensuring that questions related to the accuracy or integrity of any part of the work are appropriately investigated and resolved. The study was conducted in accordance with the Declaration of Helsinki (as revised in 2013). The study was approved by the ethics committee of KingMed Diagnostics (No. GZKM-2019-24) and informed consent was taken from all the patients.
Open Access Statement: This is an Open Access article distributed in accordance with the Creative Commons Attribution-NonCommercial-NoDerivs 4.0 International License (CC BY-NC-ND 4.0), which permits the non-commercial replication and distribution of the article with the strict proviso that no changes or edits are made and the original work is properly cited (including links to both the formal publication through the relevant DOI and the license). See: https://creativecommons.org/licenses/by-nc-nd/4.0/.
References
- Jin X, Ren J, Li R, et al. Global burden of upper respiratory infections in 204 countries and territories, from 1990 to 2019. EClinicalMedicine 2021;37:100986. [Crossref] [PubMed]
- Gebru AA, Birhanu T, Wendimu E, et al. Global burden of COVID-19: Situational analyis and review. Hum Antibodies 2021;29:139-48. [Crossref] [PubMed]
- Chen X, Yan X, Sun K, et al. Estimation of disease burden and clinical severity of COVID-19 caused by Omicron BA.2 in Shanghai, February-June 2022. Emerg Microbes Infect 2022;11:2800-7. [Crossref] [PubMed]
- Li ZJ, Zhang HY, Ren LL, et al. Etiological and epidemiological features of acute respiratory infections in China. Nat Commun 2021;12:5026. [Crossref] [PubMed]
- Zhang Y, Qiao L, Yao J, et al. Epidemiological and clinical characteristics of respiratory viruses in 4403 pediatric patients from multiple hospitals in Guangdong, China. BMC Pediatr 2021;21:284. [Crossref] [PubMed]
- Rehfuess EA, Movsisyan A, Pfadenhauer LM, et al. Public health and social measures during health emergencies such as the COVID-19 pandemic: An initial framework to conceptualize and classify measures. Influenza Other Respir Viruses 2023;17:e13110. [Crossref] [PubMed]
- Weidmann MD, Berry GJ, Green DA, et al. Prevalence and Clinical Disease Severity of Respiratory Coinfections During the Coronavirus Disease 2019 Pandemic. Advances in Molecular Pathology 2022;5:73-84.
- He Y, Liu WJ, Jia N, et al. Viral respiratory infections in a rapidly changing climate: the need to prepare for the next pandemic. EBioMedicine 2023;93:104593. [Crossref] [PubMed]
- Chow EJ, Uyeki TM, Chu HY. The effects of the COVID-19 pandemic on community respiratory virus activity. Nat Rev Microbiol 2023;21:195-210. [Crossref] [PubMed]
- Liang J, Wang Y, Liu Y, et al. Epidemiology dynamic of the common respiratory virus in winter-spring, 2018-2023 in Guangdong province, China. J Thorac Dis 2023;15:7165-7. [Crossref] [PubMed]