Using a chemiresistor-based alkane sensor to distinguish exhaled breaths of lung cancer patients from subjects with no lung cancer
Introduction
Lung cancer is the most common cancer worldwide, with 1.59 million people estimated to have died from lung cancer in 2012 (1). In spite of the steady increase in survival rate for most cancers, advances have been slow for lung cancer. The 5-year survival rate for lung cancer is merely 18%, as at least half of the cases are diagnosed at an advanced stage when a cure is not possible (2). Therefore, an accurate and timely diagnosis of lung cancer is essential in order to identify patients who are still in the early, treatable stage of the disease.
In recent years, there has been an increasing interest in finding a diagnostic method for lung cancer that is minimally invasive, free of radiation and accurate in detecting lung cancer at its early stage. This is because the National Lung Screening Trial (NLST) (3) conducted on 53,454 study subjects demonstrated that the chest tomography (CT) scan and chest X-ray imaging are associated with very high false-positive results in detecting lung cancer in early stages (96.4% for low-dose CT scan; 94.5% for chest X-ray). Furthermore, one person out of 2,500 people screened by chest imaging will die of radiation-induced cancer (4). To address this issue, previous studies show that using the pattern of endogenous volatile organic compounds (VOCs) in the exhaled breath for the detection of lung cancer is one of the promising solutions. Cellular metabolisms produce VOCs, such as alcohols, alkanes, etc., that will be carried in the bloodstream. Subsequently, the VOCs diffuse into the alveolar air in the blood-gas interface quickly in the lung because of their low solubility in the blood, and they are exhaled out of the lung (5). Therefore, analysing the composition of VOCs in exhaled breath can provide a window into the biochemical process of the body and detect altered metabolism, particularly in cancer cells. This method has a few advantages: it is non-invasive, potentially inexpensive and it is very easy to obtain exhaled breath (6,7). Compared to biomarkers in serum, the breath contains a less complicated mixture of VOCs. Moreover, breath testing provides direct and real-time monitoring, and has the potential of detecting lung cancer when it is still localised as VOCs markers are transmitted to the alveoli to be exhaled at the onset of the disease. Another important advantage is the presence of lung cancer tumour is not masked by other diseases since every disease has its own volatiles fingerprint. Even the VOC signatures in the exhaled breath of patients with different types of cancer such as lung, breast, colorectal and prostate cancers mostly do not overlap (8). For these reasons, in recent years, there has been an increasing interest in the field of analysing the exhaled volatile compounds and exhaled breath condensate (EBC), known as breathomics, in order to find the non-invasive biomarkers for respiratory diseases (9).
Alkane compounds in the breath are reported to be one of the significant biomarkers for lung cancer identification. In 2003, Phillips et al. (10) reported that after gas chromatography-mass spectrometry (GC-MS) analysis of the exhaled breath, a predictive model employing only nine alkane compounds in the exhaled breath was sufficient to give adequate discrimination between lung cancer patients and healthy controls. Analysis of headspace of the in vitro lung cancer cell lines and healthy lung tissues using GC-MS confirmed that the concentration of expired alkane compounds differed between diseased and healthy state (11). The increased level of alkane compounds in the breath from the lung cancer patients is associated with the elevated oxidative stress in the lung neoplasms which promotes the peroxidation of polyunsaturated fatty acids (PUFAs) and produces greater amount of saturated hydrocarbons such as pentane and heptane (12).
Besides lung cancer, it is known that long term cigarette smoking plays a significant role in the pathogenesis of chronic obstructive pulmonary disease (COPD), and several non-invasive oxidative stress biomarkers in the exhaled breath had been investigated to detect the development of COPD (13). Thus far, it is still unknown whether the differentiation between patients with COPD, a population with an increased risk of developing lung cancer, and patients with lung cancer based on the level of alkane compounds is possible. Dragonieri et al. (14) observed that patients with these two different smoking-related diseases have different smellprints and an electronic nose with pattern recognition algorithm can separate the VOCs smellprints. However, in their study, the specific VOCs which differed between COPD patients and lung cancer patients remain unclear since an electronic nose does not give quantitative measure of the concentration of each VOC in the smellprints (15).
The purpose of this study is to investigate the ability of a simple alkane sensor to differentiate the exhaled breath of lung cancer patients, patients with COPD and people without pulmonary disease. We hypothesize that there are significantly different levels of alkanes in the mixture of exhaled VOCs between patients with lung cancer and people without lung cancer.
Methods
Part 1 of the study: fabrication of the chemiresistor-based alkane sensor
Chemiresistor-based alkane sensor
A chemiresistor-based sensor consists of a chemiresistive film made up of organic compounds or semiconductor metal oxides that responds to the presence of VOCs by changing the resistance of the sensor (16,17). By measuring the change in resistance of the sensor, the concentration of the VOCs can be measured indirectly. In our study, we used a monomer composite to fabricate the chemiresistive film because it is cheap, operative under room temperature (17) and it can detect a mixture of alkanes in the presence of saturated water vapour (18). The composite film consisted of conductive carbon particles interspersed in an insulating monomer matrix. On exposure to VOC gases, the VOC diffuses into the monomer composite causing the composite to swell, which then causes the dispersed conductive carbon particles in the composite to move further apart from each other. As a result, the resistance of the sensor changes. The chemical sensor is similar to the widely used commercial e-nose, Cyranose 320, which has shown to have high between-day and within-day reproducibility (19).
Fabrication of sensor
Tetracosane and carbon powder were used to create the chemiresistive film of the sensor. Tetracosane is a C20 alkane crystalline that has high affinity to alkane gas compounds because of structural similarity while carbon powder endows conductivity to the film. Both the sensor materials were deposited on to the electronic circuit board in a mass ratio of 1:1 by spreading carbon powder on top of the melted tetracosane and subsequently cooling the matrix. Figure 1 shows the prototype of the sensor inside a 125 mL Vitagen® container used as the breath sampling apparatus.
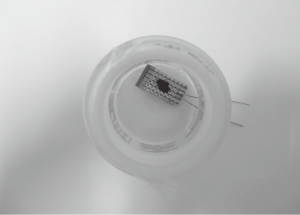
The VOC mixture in human breath contains alkanes and other polar molecules such as alcohol and aldehyde which are in parts per billion (ppb) concentration (20). Santonico et al. (21), who calibrated two commercially available electronic noses with three VOCs that are present in the exhaled breath, stated that it is important to perform instrumental calibrations for gas sensors in terms of sensitivity, resolution and limit of detection to ensure high quality of the data obtained in real clinical practice. To test the sensitivity and specificity of our sensor, the sensors were exposed to different concentrations (10 ppb to 100 ppm) of heptane, ethanal and ethanol, which were prepared using 2 L static dilution bottles and were injected using a gas-tight syringe into the 125 mL bottle at intervals of 3 seconds to simulate normal breathing. The baseline resistance (the resistance value shown before the sensor was exposed to the VOCs) was recorded and the resistance value of the sensor shown in the digital multimeter, which was connected to the electrodes on the sensor, was recorded at intervals of 3 seconds for 7 minutes. The percentage change in baseline resistance to the maximum resistance (known as sensor peak output) and time taken from baseline resistance to maximum resistance (known as time to peak) were calculated.
Figure 2 shows that scatterplot of both the sensor peak output and the time to peak against the concentrations of heptane vapour in logarithm scale. Two Pearson product-moment correlation tests were run individually to determine the relationship between the peak output as well as the time to peak and the concentration of heptane. There were strong, positive correlations between the two dependent variables generated by the sensor and the heptane concentration, both of which were statistically significant (peak output: r=0.978, n=35, P<0.0005; time to peak: r=0.980, n=35, P<0.0005). This is reasonable as there were more analytes so it would generate larger sensor output and would take longer time to reach the peak resistance. The range of detection for alkanes is 20 ppb to 80 ppm, which is considered sufficient to detect the combined concentrations of all alkanes in the exhaled breath (20). The sensitivity of the sensor was a 4.368% change in resistance per log(ppb) for the peak output and 106.1 s per log(ppb) for the time to peak value. It is also found that the sensor was single-use. As expected, the sensor did not respond to ethanol and ethanal because polar ethanol and ethanal molecules would not be absorbed into the non-polar chemiresistive film.
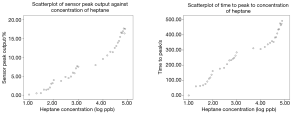
The response of sensor towards water was investigated by dropping water drops onto the sensor. It was found that there was no change in the resistance for the ten sensors tested, demonstrating that the sensor has zero sensitivity to water. The reason is because of the hydrophobicity of both the tetracosane and the carbon powder. Therefore, the saturated level of water vapour in the exhaled breath would not induce sensor response.
Part 2 of the study: clinical testing of the chemiresistor-based alkane sensor
Study design
In this cross-sectional study, patients with newly diagnosed treatment-naïve advanced lung cancer, ex- or current smokers with COPD and healthy never-smokers were asked to exhale through a drinking straw into the breath-in apparatus with the chemiresistor sensor attached to its inside bottom. The sensor peak output (percentage change of baseline resistance measured before exhalation to peak resistance) and the time taken for the baseline resistance to reach peak resistance were measured. The study was approved by the University of Malaya Medical Centre Ethics Committee and was conducted according to the Good Clinical Practice (GCP) Guidelines. All the study subjects gave their written informed consent.
Study population
The study population included a total 37 subjects who were divided into three groups according to their lung health status: patients with non-small cell lung cancer (NSCLC), patients with COPD and non-smokers without known pulmonary disease. All the subjects were adults matched for age 40 to 85 years old. Thirty study subjects (10 lung cancer patients, 10 COPD patients and 10 controls without lung disease) were recruited from the University of Malaya Medical Centre while the other seven were recruited from in KPJ Specialist Hospital, Ipoh and during a lung health screening programme in two other specialist clinics.
The lung cancer group comprised 12 patients with newly diagnosed, histologically confirmed, treatment-naïve NSCLC who were either current, ex- or never smokers. One of the lung cancer patient had pulmonary fibrosis, one had old pulmonary tuberculosis and another had co-existing COPD. The rest did not have any other lung diseases. The cause of pulmonary fibrosis was not studied and the pulmonary tuberculosis was previously treated and remained inactive at the time of testing. Spirometry testing was not performed for all the lung cancer patients. The clinical characteristics of patients with NSCLC are shown in Table 1.
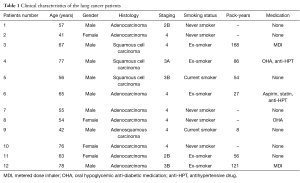
Full table
The COPD group consisted of 12 patients who were current or ex-smokers. The diagnosis of COPD was based on the demonstration of a fixed airflow obstruction with a ratio of post-bronchodilator forced expiratory volume in the first second (FEV1) to forced vital capacity (FVC) of less than 0.7. All COPD patients were on the appropriate inhaled medication. The controls group consisted of 13 subjects, each with a negative history of respiratory symptoms, no history of smoking and absence of any known pulmonary disease. Four subjects in the control group were taking oral hypoglycemic anti-diabetic (OHA) medications. The characteristics of these two groups are shown in Table 2.
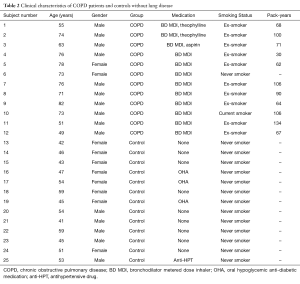
Full table
Breath analysis
The study subjects were not allowed to smoke or take any alcoholic beverage within 12 hours of the breath collection. The baseline resistance of the sensor was recorded before the experiment started. The study subject was then asked to exhale tidally through the drinking straw into the 125 mL bottle containing the chemiresistor sensor 200 times. There was no breath holding during exhalation. The resistance of the sensor during expiration was recorded at intervals of 30 seconds.
Unlike other studies that investigate the diagnostic ability of an electronic nose, the exhaled breath of the subject was immediately transferred to the sensor without passing through any VOC-filter mouthpiece or drying chamber or preconcentrator to remove the water vapour in the exhaled breath.
Statistical analysis
The sensor peak output (percentage change of the baseline resistance to the sensor peak resistance) and the time to peak (time taken for the resistance value to reach the maximum change) were calculated from the data collected. The ability of the sensor to discriminate the exhaled breath between the patients with lung cancer, patients with COPD and control subjects without lung disease was investigated by using one-way multivariate analysis of variance (MANOVA) followed up by post-hoc test on the dependent variables: sensor peak output and time to peak. In preparatory to MANOVA, the data was tested with multiple statistical techniques using IBM SPSS Statistic 24 to ensure that the data met all the assumptions that are required for a one-way MANOVA to produce a valid result, which can be found in a supplementary appendix online. Receiver operator characteristic (ROC) curves were then plotted to analyse the sensitivity and specificity of the sensor in diagnosing lung cancer.
Results
Two anomalous results (one from a COPD patient and one from a healthy control) were removed before MANOVA was carried out. Interestingly, the MANOVA showed a statistically significant difference between the three groups of study subjects on the linear combination of sensor peak output and the time to peak value, Pillai’s Trace =0.393, F=3.909, df = (4, 64), P=0.007, partial η2=0.196. It revealed that different lung health status of the study subjects had significant effect on the time to peak resistance value [F(2, 32) =6.116; P=0.001; partial η2=0.267] and the peak output [F(2, 32) =3.614; P=0.004; partial η2=0.184]. Post-hoc Tukey Honest Statistical Difference (HSD) analysis showed the mean values for time to peak were statistically different between lung cancer patients and control subjects without lung disease (P=0.001) and between lung cancer patients and COPD patients (P=0.006) but not between COPD patients and control subjects without lung disease (P=0.778). Likewise, the mean peak output was statistically different between lung cancer patients and control subjects without lung disease (P=0.007) and between lung cancer patients and COPD patients (P=0.016), but not between COPD patients and control subjects without lung disease (P=0.963).
On average, the sensor peak output for lung cancer patient group (n=12) was 4.45 [95% confidence interval (CI): 2.57–6.33], for the COPD patient group (n=11) was 1.68 (95% CI: 1.00–2.36) and for the control group without lung disease (n=12) was 1.44 (95% CI: 0.54–2.34). For the group of patients with lung cancer, the mean time to peak of the sensor was 205 s (95% CI: 138–272). The time to peak resistance value was 76 s (95% CI: 18–134) for the COPD patients group and 50 s (95% CI: 22.62–77.38) for the group of control subjects.
ROC curve analysis on the three classifiers, namely the combination of both sensor peak output and time to peak, sensor peak output alone and time to peak alone, showed the combination of both independent variables was the best classifier with an area under curve (AUC) of 0.8567 as shown in Figure 3. An AUC of 0.8567 means that the combination of the sensor peak output and the time to peak could provide an excellent discrimination of lung cancer from both COPD patients and people without lung disease. Therefore, the sensor has a potential for clinical application. When a 2.20% sensor peak output and a 90-s time to peak were used as the cutoff value for detection of lung cancer, an optimal diagnostic accuracy was obtained which had a sensitivity of 83.3% and a specificity of 88.0%.
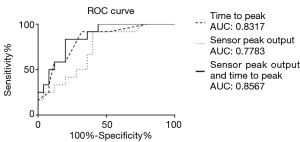
The most striking result to emerge from the study is that chronic tobacco smoking did not affect the diagnostic accuracy of the sensor. The sensitivity and specificity for identifying lung cancer patients from people with a smoking history (n=17) were 85.7% and 80%, respectively while that from the non-smoker population (n=20) were 80% and 93.3%, respectively using the aforementioned threshold.
Discussion
Unlike in electronic nose studies where principal component analysis (PCA) is used to interpret the results generated from sensor array, we used MANOVA test to analyse our clinical trial data because only a single alkane sensor was used per breath test, so there was no need for PCA to reduce the dimensionality of the data. Furthermore, MANOVA allows us to test hypotheses regarding the effect of three different groups of study subjects on the sensor peak output and time taken to reach peak resistance. Once the statistical difference between the three groups was established, post-hoc Tukey HSD test showed where the differences between the groups were (22).
Our study demonstrated that the alkane sensor can distinguish the level of alkanes present in the exhaled breath of lung cancer patients from that of controls. Furthermore, it also showed a good discrimination between patients with two different smoking-related lung diseases using the combination of the peak output and the time to peak resistance. From the study result, lung cancer patients had the largest average sensor peak output and time to peak values. These results suggest that the exhaled breath of patients with lung cancer contained a significantly higher level of alkane compounds than that of COPD patients and people without lung disease. Our results are in accordance with findings from recent studies (11,23). A review on chemical pathways for VOCs of lung cancer (12) outlined that endogenous alkanes present in our breath are the products of peroxidation of PUFAs and the mechanism is initiated by reactive oxygen species (ROS) radicals and proceeds by a free radical chain reaction mechanism. As the oxidative stress in lung cancer cell lines is greater (24), there are more ROS such as hydrogen peroxide radicals in the cancerous cells for initiation of PUFA peroxidation so more alkanes are produced. Peng et al. (23) used solid-phase microextraction (SPME)-aided GC-MS to analyse the preconcentrated healthy and lung cancer breath and showed that out of 12 common alkane compounds found in the breath of at least 83% of the patients and in fewer than 83% of the healthy subjects, 10 were elevated for lung cancer patients. Two alkane compounds (2,3,4-trimethylpentane and 2,3-dimethylhexane) were only present in the exhaled breath of lung cancer patients. In vitro studies show that while some alkane compounds elevate, some decrease in the concentration of the headspace of the lung cancer cells compared to that of healthy cell lines (11). The proposed explanation for these findings is that cytochrome p450 mixed oxidase activity is induced during carcinogenesis so there is hydroxylation of some alkane compounds into alcohols (12). However, our results suggest that oxidative stress has a more profound effect than the cytochrome p450 since the concentration of alkane mixture is greater in the exhaled breath of patients with lung cancer compared to people without it.
The onset and development of COPD are associated with several mechanisms such as increased inflammation and autoimmunity that lead to elevated ROS such as superoxide radical and hydrogen peroxide in the lung and continued presence of oxidative stress (25). An interesting study by Poli et al. (26) who used mass spectrometry technology to identify the breath signatures of lung cancer patients and COPD patients showed that the levels of alkanes were statistically different between a group of 36 patients with NSCLC and 25 patients with COPD. Our data suggest that there is a difference of breath alkane composition between lung cancer and COPD and the difference could be because of the different biochemical processes and levels of oxidative stress in the bronchus and lung tissues.
It is surprising that our post-hoc test showed that both the parameters were indistinguishable between the COPD group and the group of subjects without lung disease since Aghdassi et al. (27) had demonstrated that smokers had a higher level of oxidative stress and lipid peroxidation than non-smokers. Nonetheless, both the mean time to peak and sensor peak output for COPD patients were higher compared to that for group of subjects without lung disease. A possible explanation for this might be that the resolution of the sensor was insufficient to pick up the difference in alkane levels in the breath of COPD patients and subjects without lung disease.
Another important finding of our study is that tobacco smoking did not jeopardize the diagnostic accuracy of the sensor. This result is consistent with the finding that the most common breath VOCs associated with tobacco smoking are not alkane compounds (28). Besides, age was not an indirect mediator of the predictive value of the breath alkane in detecting lung cancer albeit oxidative stress is often linked with age. Our study did not detect any evidence to suggest that the magnitude of sensor response varied with age. This could be because the age distribution in the study subjects in our experiment was not wide enough and the sample size was small. Phillips et al. (29) showed that the difference in alkane levels is only apparent when the age difference is over 40 years.
In our study, the patients and the controls were well-characterized by standardized guidelines. Treatment-naïve lung cancer patients were chosen to participate in our study to ensure that drug treatment would not affect the accuracy of the result and secondly, to study the performance of the sensor in diagnosing suspected lung cancer before diagnostic and treatment intervention. For breath analysis, the exhaled breath was not sampled or stored in collection bags for later analysis, instead, the levels of exhaled alkane compounds were measured on-site directly and immediately so the decomposition or the loss of VOCs by diffusion would not take place (30,31). Study subjects exhale 200 times to ensure sufficient period of time for the sensor to reach peak resistance, as our preclinical experiments showed that it could take up to 500 s to generate sensor peak output. Besides, the breath-in apparatus was partially sealed. This allowed the later exhaled breath from the study subject into the container to constantly replace the earlier exhaled breath. In other words, the earlier exhaled air would be ‘forced’ out of the container by the later breaths. Since anatomic dead space air was exhaled for the initial few seconds (32), exhaling for more than 5 minutes would provide sufficient period of time for the sensor to be in contact with and detect alkanes in the air from deeper parts of the airway, which is the alveolar air. However, if the expiratory flow rate of the study subjects was standardised, we could estimate the time (t) needed to wash out the dead space and discard the first t seconds of the exhaled air (32), which would increase the reliability of the data. There was no breath holding during exhalation. It has reported that breath holding would significantly raise the VOCs level due to accumulation of VOCs in the airways (33).
Tidal breathing, which was the breathing manoeuvre used in this study, was considered the most reliable methodology (31). The study subjects participating in our study were encouraged to ventilate normally and naturally in the study, and breath samples were obtained from a series exhalation. However, there could be occurrence of irregular breathing patterns and random fluctuations in breathing frequency and intensity could occur, which means controlling their expiratory flow rate was impossible (31). Since several studies have pointed out that expiratory flow rate can alter the level of VOCs in the exhaled breath of people without lung cancer (33,34), the effect of different expiratory flow rates on the diagnostic accuracy of the sensor should be further studied and the diagnostic accuracy of controlled flow rate should be compared with tidal breathing.
In our study, we deliberately did not use a VOC-filter to reduce the complexity of the breath collection and analysis system. If a VOC-filter was used, VOC-filtered room air would be needed to set the baseline resistance of the sensor (14,33). Our preclinical experiments showed that concentrations of alkanes in ppb range of concentration, that is the concentration of alkanes in the exhaled breath (20), would still trigger sensor response even if VOC-present room air was used to set the baseline resistance. In fact, a few studies deliberately did not use a VOC-filter for their breath tests and still found the electronic noses could identify the breath-prints accurately (35-37). Our patients were studied in a typical air-conditioned clinical environment, similar to the study by Chapman et al. (35). However, the usage of VOC-filter would potentially increase the diagnostic accuracy as inhalation through a VOC-filter prior to collection could prevent contamination by ambient air and eliminate the potential confounding effect of collection in different environments (36).
This study has several limitations. First, we could not determine the exact concentration of exhaled alkane compounds. Even though the sensor was largely water-resistant, it was possible that the condensation of water (30) on the sensor could become a barrier between the non-soluble exhaled alkanes molecules and certain areas of the composite film. This could have led to less alkane molecules being absorbed into the composite film and induce smaller change in resistance in comparison to preclinical calibration result for same concentration for alkane, where no condensation had occurred. Although this would hypothetically reduce the limit of detection and sensitivity of the sensor, our clinical study showed that the sensor was still able to detect the alkanes in the exhaled breath. Besides, different alkane molecules may induce different magnitudes of change in resistance of the sensor. Secondly, the exact composition of the alkane mixture in the exhaled breath is unknown since the sensor assessed how the mixture of all the endogenous alkane compounds varies between the study groups. In other words, the sensor was unable to investigate the qualitative difference of the alkanes exhaled by the three groups of study subjects. Both the limitations can be overcome by conducting GC-MS and incorporating a drying chamber in the equipment. In spite of the implicit limitations, assessing the level of alkane mixture in exhaled breath suffices in diagnostic assessment.
Although the sensor yielded promising results in diagnosing lung cancer patients with high accuracy, we do not know how it will perform in the diagnosis of early lung cancer because 10 out of 12 our patients with NSCLC were in advanced stages (stage III or IV) of the disease. Further study with a larger sample size of all three groups of study subjects and a mix of patients with early and advanced stage lung cancer is warranted to confirm the results of this study. Besides the sensitivity of the sensor, the ability of the alkane sensor in distinguishing the different histological subtypes of NSCLC should be studied with a larger sample size. Another limitation with the small sample size was that we could not split the study subjects into training and testing datasets. Leopold et al. (38) outlined the necessity of including an external validation set, which is an independent cohort of study subjects that is separated from training cohort by either time or place, to determine the true diagnostic ability of an electronic nose.
In future recruitment of study subjects, population with pneumonia, pulmonary fibrosis, asthma and tuberculosis should be included. Denholm et al. (39) found that apart from chronic bronchitis and emphysema, which are grouped as COPD, a positive association was observed between lung cancer and pneumonia diagnosed 2 years or less before lung cancer, while Li et al. (40) reported high prevalence rates of lung cancer in patients with idiopathic pulmonary fibrosis (IPF), which suggested that IPF would increase the risk of lung cancer. Alkane is not a breath biomarker characteristics of pneumonia (41,42), whereas the concentration of exhaled ethane was found to be higher in the patients with IPF compared to the healthy subjects (43). On the other hand, although there is no independent association between lung cancer and smoking-related respiratory diseases, namely tuberculosis and asthma (39,44), alkanes are associated with oxidative stress resulting from infection by Mycobacterium tuberculosis as well as airway inflammation by asthma (45,46).
The clinical importance of this study is the alkane sensor is inexpensive and any medical personnel can operate it and interpret the results generated. Additionally, it can detect lung cancer from raw untreated exhaled breath. It overcomes the cost, complexity and standardisation problems that are associated with the clinical use of electronic noses. To attain maximum discrimination of patients from controls, laboratory-grade instruments have to be used which are expensive and too complicated for medical personnel and industry technicians to operate and interpret the data generated (47). Standardisation of breath sample collection and analysis methods for electronic nose is also needed, which may be difficult to achieve and this remains the key challenge of full-scale introduction of breath tests into clinical practice (48).
Conclusions
This study has demonstrated for the first time that a simple alkane sensor can distinguish the exhaled breath of lung cancer patients from that of COPD patients and people with no lung disease without the need for prior treatment of the breath. The clinical study demonstrated that the sensor could achieve a sensitivity of 83.3% and a specificity of 88.0% in diagnosing lung cancer by using a 2.20% sensor peak output and a 90-s time to peak value as threshold values. The concentration of alkane mixture was higher in the exhaled breath of lung cancer patients than that of subjects without lung cancer. Future studies should assess the accuracy of the alkane sensor in detecting lung cancer at its early stage and the ability of the sensor in identifying histological subtypes of lung cancer.
Acknowledgements
We appreciated the help from Mr. Leaw Ah See for allowing access to the chemistry laboratory of Chung Hua High School, Seremban to fabricate the alkane sensors and to prepare the VOCs at different concentrations for the performance testing of the sensors.
Footnote
Conflicts of Interest: The authors have no conflicts of interest to declare.
Ethical Statement: The study was approved by the University of Malaya Medical Centre Ethics Committee (approval number: 20146-289) and was conducted according to the Good Clinical Practice (GCP) Guidelines. All the study subjects gave their written informed consent.
Assumptions of multivariate analysis of variance (MANOVA)
The main objective of using MANOVA is to determine if the response variables are altered by the observer’s manipulation of the independent variables. There are nine assumptions (49,50) to be met by the data before they undergo MANOVA so that the analysis can produce valid result. The data which were sensor peak output and time to peak resistance collected from the clinical study were checked if they “passed” all the assumptions. All the nine assumptions followed by pre-MANOVA assumptions analysis results are shown below:
- The dependent variables used for MANOVA should be continuous data. All the sensor peak output and the time to peak values were continuous data.
- The independent variables should consist of two or more categorical independent groups. In this study, groups of lung cancer patients, COPD patients and controls without lung disease were the three categorical independent variables. They were categorical data and each group was independent of the other two.
- The observations must be independent, meaning that there must be different participants in each group with no participants being in more than one group. Our data fitted the independence of observations.
- There are more cases in each group of independent variables than the number of dependent variables. In this study, we were analysing two dependent variables while the three groups had 12 or 13 study subjects, so the sample size was adequate.
- There are no multivariate or univariate outliers. We used cumulative chi-squared test of Mahalanobis distance with a degree of freedom of two to check for multivariate outliers for the combination values of sensor peak output and time to peak; none of the multivariate values in the raw data was considered anomalous as all the p-values of the Mahalanobis distance were greater than 0.001. Boxplots were used to detect univariate outliers in the raw data and we found two data which were shown as unfilled circles in Figure S1, were outliers. They were removed from the data used for further assumption-analysis and MANOVA.
- There is multivariate normality. Shapiro-Wilk test of normality was used to analyse our data with P<0.05 as criterion and it showed that our data did not reject the null hypothesis, meaning the data were normally distributed. The P-significance values for the time to peak and the peak output on the three groups were greater than 0.05.
- There is a linear relationship between each pair of dependent variables for each group of the independent variable. Scatterplot matrix in Figure S2 shows that there was a general pattern of top left passing through central of origin to bottom right in all the three scatterplot diagrams, illustrating the linear relationship between the sensor peak output and the time to peak in all the three groups of study subjects.
- There is a homogeneity of variance-covariance matrices. Box’s M test of equality of covariance checks the homogeneity of covariance across the groups using P<0.001 as criterion while Levine’s Test of Equality of Error Variances tests if the variances of each variable are equal across the groups using P<0.05 as criterion. If insignificant P values are obtained from both tests, then the assumption is not violated. We obtained a Box’s M (18.962) with P=0.008, indicating that there was no significant difference between the covariance matrices. However, in the Levine’s Test, both the sensor peak output data (P=0.006) and the time to peak data (P=0.002) violated the assumption of homogeneity of variances. Hence, Pillai’s Trace test in the MANOVA had to be used instead of Wilk’s Lambda test as the former is very robust and not highly linked to the assumptions about the normality of the distribution of the data.
- There is no multicollinearity, which means the dependent variables should have a low to moderate correlation among themselves. The Pearson correlation coefficient between the sensor peak output and the time to peak was 0.735. The correlation value was considered moderate so there was no multicollinearity in our data.
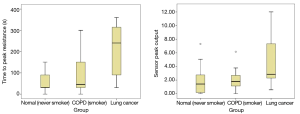
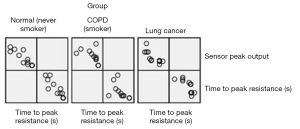
References
- Cancer Research UK [Internet]. United Kingdom: Cancer Research UK [updated 2015 July; cited 2016 May 12]. Lung Cancer Key Statistics; [about 3 screens]. Available online: http://www.cancerresearchuk.org/health-professional/cancer-statistics/statistics-by-cancer-type/lung-cancer
- Siegel RL, Miller KD, Jemal A. Cancer statistics, 2015. CA Cancer J Clin 2015;65:5-29. [Crossref] [PubMed]
- National Lung Screening Trial Research Team, Aberle DR, Adams AM, et al. Reduced lung-cancer mortality with low-dose computed tomographic screening. N Engl J Med 2011;365:395-409. [Crossref] [PubMed]
- Bach PB, Mirkin JN, Oliver TK, et al. Benefits and harms of CT screening for lung cancer: a systematic review. JAMA 2012;307:2418-29. [Crossref] [PubMed]
- Cao W, Duan Y. Breath analysis: potential for clinical diagnosis and exposure assessment. Clin Chem 2006;52:800-11. [Crossref] [PubMed]
- Dent AG, Sutedja TG, Zimmerman PV. Exhaled breath analysis for lung cancer. J Thorac Dis 2013;5 Suppl 5:S540-50. [PubMed]
- Dummer J, Storer M, Swanney M, et al. Analysis of biogenic volatile organic compounds in human health and disease. TrAC Trends Anal Chem 2011;30:960-7. [Crossref]
- Peng G, Hakim M, Broza YY, et al. Detection of lung, breast, colorectal, and prostate cancers from exhaled breath using a single array of nanosensors. Br J Cancer 2010;103:542-51. [Crossref] [PubMed]
- Santini G, Mores N, Penas A, et al. Electronic Nose and Exhaled Breath NMR-based Metabolomics Applications in Airways Disease. Curr Top Med Chem 2016;16:1610-30. [Crossref] [PubMed]
- Phillips M, Cataneo RN, Cummin AR, et al. Detection of lung cancer with volatile markers in the breath. Chest 2003;123:2115-23. [Crossref] [PubMed]
- Filipiak W, Sponring A, Filipiak A, et al. TD-GC-MS analysis of volatile metabolites of human lung cancer and normal cells in vitro. Cancer Epidemiol Biomarkers Prev 2010;19:182-95. [Crossref] [PubMed]
- Hakim M, Broza YY, Barash O, et al. Volatile organic compounds of lung cancer and possible biochemical pathways. Chem Rev 2012;112:5949-66. [Crossref] [PubMed]
- Malerba M, Montuschi P. Non-invasive biomarkers of lung inflammation in smoking subjects. Curr Med Chem 2012;19:187-96. [Crossref] [PubMed]
- Dragonieri S, Annema JT, Schot R, et al. An electronic nose in the discrimination of patients with non-small cell lung cancer and COPD. Lung Cancer 2009;64:166-70. [Crossref] [PubMed]
- Montuschi P, Mores N, Trové A, et al. The electronic nose in respiratory medicine. Respiration 2013;85:72-84. [Crossref] [PubMed]
- Bănică FG. Resistive Gas Sensors (Chemiresistors). In: Fogg AG. editor. Chemical Sensors and Biosensors: Fundamentals and Applications. 1st ed. United Kingdom: John Wiley & Sons, Ltd, 2012:246-56.
- Arshak K, Moore E, Lyons G, et al. A review of gas sensors employed in electronic nose applications. Sensor Rev 2004;24:181-98. [Crossref]
- Folinsky A. Lung Cancer Detection Using an Electronic Nose [Internet]. California Institute of Technology; 2005 March [cited on 2016 May 12]. Available online: http://web.mit.edu/~kilroi/Public/text/ifprop.pdf
- Bofan M, Mores N, Baron M, et al. Within-day and between-day repeatability of measurements with an electronic nose in patients with COPD. J Breath Res 2013;7:017103. [Crossref] [PubMed]
- Buszewski B, Ligor T, Jezierski T, et al. Identification of volatile lung cancer markers by gas chromatography-mass spectrometry: comparison with discrimination by canines. Anal Bioanal Chem 2012;404:141-6. [Crossref] [PubMed]
- Santonico M, Pennazza G, Capuano R, et al. Electronic noses calibration procedure in the context of a multicentre medical study. Sens Actuators B Chem 2012;173:555-61. [Crossref]
- Laerd Statistics [Internet]. United Kingdom: Laerd Statistics; [Publication date unknown; cited 2016 May 12]. One-way ANOVA (cont...); [about 1 screen]. Available online: https://statistics.laerd.com/statistical-guides/one-way-anova-statistical-guide-4.php
- Peng G, Tisch U, Adams O, et al. Diagnosing lung cancer in exhaled breath using gold nanoparticles. Nat Nanotechnol 2009;4:669-73. [Crossref] [PubMed]
- Esme H, Cemek M, Sezer M, et al. High levels of oxidative stress in patients with advanced lung cancer. Respirology 2008;13:112-6. [Crossref] [PubMed]
- Kirkham PA, Barnes PJ. Oxidative stress in COPD. Chest 2013;144:266-73. [Crossref] [PubMed]
- Poli D, Carbognani P, Corradi M, et al. Exhaled volatile organic compounds in patients with non-small cell lung cancer: cross sectional and nested short-term follow-up study. Respir Res 2005;6:71. [Crossref] [PubMed]
- Aghdassi E, Allard JP. Breath alkanes as a marker of oxidative stress in different clinical conditions. Free Radic Biol Med 2000;28:880-6. [Crossref] [PubMed]
- Gordon SM, Wallace LA, Brinkman MC, et al. Volatile organic compounds as breath biomarkers for active and passive smoking. Environ Health Perspect 2002;110:689-98. [Crossref] [PubMed]
- Phillips M, Cataneo RN, Greenberg J, et al. Effect of age on the breath methylated alkane contour, a display of apparent new markers of oxidative stress. J Lab Clin Med 2000;136:243-9. [Crossref] [PubMed]
- Lourenço C, Turner C. Breath analysis in disease diagnosis: methodological considerations and applications. Metabolites 2014;4:465-98. [Crossref] [PubMed]
- Alonso M, Sanchez JM. Analytical challenges in breath analysis and its application to exposure monitoring. TrAC Trends Anal Chem 2013;44:78-89. [Crossref]
- Bikov A, Paschalaki K, Logan-Sinclair R, et al. Standardised exhaled breath collection for the measurement of exhaled volatile organic compounds by proton transfer reaction mass spectrometry. BMC Pulm Med 2013;13:43. [Crossref] [PubMed]
- Bikov A, Hernadi M, Korosi BZ, et al. Expiratory flow rate, breath hold and anatomic dead space influence electronic nose ability to detect lung cancer. BMC Pulm Med 2014;14:202. [Crossref] [PubMed]
- Lärstad MA, Torén K, Bake B, et al. Determination of ethane, pentane and isoprene in exhaled air--effects of breath-holding, flow rate and purified air. Acta Physiol (Oxf) 2007;189:87-98. [Crossref] [PubMed]
- Chapman EA, Thomas PS, Stone E, et al. A breath test for malignant mesothelioma using an electronic nose. Eur Respir J 2012;40:448-54. [Crossref] [PubMed]
- Cheng ZJ, Warwick G, Yates DH, et al. An electronic nose in the discrimination of breath from smokers and non-smokers: a model for toxin exposure. J Breath Res 2009;3:036003. [Crossref] [PubMed]
- Wang XR, Lizier JT, Berna AZ, et al. Human breath-print identification by E-nose, using information-theoretic feature selection prior to classification. Sens Actuators B Chem 2015;217:165-74. [Crossref]
- Leopold JH, Bos LD, Sterk PJ, et al. Comparison of classification methods in breath analysis by electronic nose. J Breath Res 2015;9:046002. [Crossref] [PubMed]
- Denholm R, Schüz J, Straif K, et al. Is previous respiratory disease a risk factor for lung cancer? Am J Respir Crit Care Med 2014;190:549-59. [Crossref] [PubMed]
- Li J, Yang M, Li P, et al. Idiopathic pulmonary fibrosis will increase the risk of lung cancer. Chin Med J (Engl) 2014;127:3142-9. [PubMed]
- Filipiak W, Sponring A, Baur MM, et al. Characterization of volatile metabolites taken up by or released from Streptococcus pneumoniae and Haemophilus influenzae by using GC-MS. Microbiology 2012;158:3044-53. [Crossref] [PubMed]
- Preti G, Thaler E, Hanson CW, et al. Volatile compounds characteristic of sinus-related bacteria and infected sinus mucus: analysis by solid-phase microextraction and gas chromatography-mass spectrometry. J Chromatogr B Analyt Technol Biomed Life Sci 2009;877:2011-8. [Crossref] [PubMed]
- Kanoh S, Kobayashi H, Motoyoshi K. Exhaled ethane: an in vivo biomarker of lipid peroxidation in interstitial lung diseases. Chest 2005;128:2387-92. [Crossref] [PubMed]
- Rosenberger A, Bickeböller H, McCormack V, et al. Asthma and lung cancer risk: a systematic investigation by the International Lung Cancer Consortium. Carcinogenesis 2012;33:587-97. [Crossref] [PubMed]
- Rufo JC, Madureira J, Fernandes EO, et al. Volatile organic compounds in asthma diagnosis: a systematic review and meta-analysis. Allergy 2016;71:175-88. [Crossref] [PubMed]
- Sethi S, Nanda R, Chakraborty T. Clinical application of volatile organic compound analysis for detecting infectious diseases. Clin Microbiol Rev 2013;26:462-75. [Crossref] [PubMed]
- Wilson AD. Future Applications of Electronic-Nose Technologies in Healthcare and Biomedicine [Internet]. In: Akyar I. editor. Wide Spectra of Quality Control. InTech; 2011 [cited 2016 May 12]. Available online: http://www.intechopen.com/books/wide-spectra-of-quality-control/future-applications-of-electronic-nose-technologies-in-healthcare-and-biomedicine
- Wilson AD. Advances in electronic-nose technologies for the detection of volatile biomarker metabolites in the human breath. Metabolites 2015;5:140-63. [Crossref] [PubMed]
- Northern Arizona University Welcome to Oak. Interpreting the one-way MANOVA [Internet]. United States: Microsoft Enterprise Networked Systems Administration group at Northern Arizona University Information Technology Services department; [Publication date unknown]; [cited 2016 Apr 22]. Available online: http://oak.ucc.nau.edu/rh232/courses/EPS625/Handouts/Interpreting%20the%20One-way%20MANOVA.pdf
- Laerd Statistics [Internet]. United Kingdom: Laerd Statistics; [Publication date unknown; cited 2016 Apr 22]. One-way MANOVA in SPSS statistics; [about 2 screens]. Available online: https://statistics.laerd.com/spss-tutorials/one-way-manova-using-spss-statistics.php