Cough frequency monitors: can they discriminate patient from environmental coughs?
Introduction
Cough frequency has long been a parameter of interest in research and clinical practice, for example in studies of excessive coughing and cough suppressants, or where cough is examined as a positive clinical sign of respiratory disease (1-5). Cough frequency measurement could also be of value in the context of neurogenic dysphagia, where the presence of cough might indicate aspiration events and increased pneumonia risk (6).
Objective measurement of cough frequency requires a monitoring system to capture and count cough events. Manual cough counts from sound recordings (cough events counted by a human listener) have been used since the 1950s (7-9). Modern sound-based cough monitors make use of compact digital recording devices that are now available. Automated or semi-automated processes have been developed, which enable a more efficient detection and counting of cough events on sound recordings of many hours’ duration (2,10,11). A potential limitation of sound-based cough monitors is compromise of their accuracy by the potential for environmental coughs (coughs from persons other than the patient) to contaminate the recording (2,10,11).
As part of a stroke trial (12), we had an opportunity to validate a sound-based cough monitor (Leicester Cough Monitor, LCM) (13) in an acute stroke unit environment. There is increasing interest in cough frequency measurements in neurologic patient groups, but devices have not been validated in these patient populations. We considered whether the LCM could discriminate stroke patient cough from environmental cough in an inpatient setting, where environmental coughs (for example from patients who share the same room, visitors or staff) could be registered and result in higher measurements. We conducted a preliminary validation study. The aim was to investigate the accuracy of the cough monitor for discriminating patient cough from environmental cough, in this particular clinical environment.
Methods
Design
Sound-based cough monitor recordings were taken from a cohort of five inpatients (consecutive recruits to the stroke trial). The cough frequency outputs generated by the cough monitor system were compared against live observer count and manual sound-file cough counts, a widely used gold standard to determine the accuracy of cough monitoring systems (14). The sample was a convenience sample, and no formal sample size calculation was conducted. The sample size was largely determined by study resources.
Setting and participants
Acute stroke patients who were inpatients at a large metropolitan acute stroke unit were recruited. To be eligible for study participation, patients had to be alert, oriented and able to follow instructions. Participants occupied beds in multi-occupancy open bays, which connected to the main ward corridor. Participants shared the space with three other patients. All participants gave written informed consent to take part in the study. The study had ethical approval from the United Kingdom National Research Ethics Service (Wandsworth Research Ethics Committee, reference 10/H0803/32).
Clinical characteristics
Patient demographic information and stroke characteristics (stroke lesion site and stroke severity) were described from clinical records, CT and/or MRI head reports and admission National Institutes of Health Stroke Scale (NIHSS) scores (15). Respiratory function was assessed by spirometry according to international clinical standards (16) and using a portable spirometer (SpiroUSB; CareFusion, San Diego, CA, USA). Maximum expiratory (PEmax) and inspiratory (PImax) mouth pressures were measured following international clinical guidelines (17) and using a portable device (MicroRPM; CareFusion). Peak cough flow measurements of maximal volitional cough were made using a calibrated Fleisch pneumotachograph with a face mask (18). Respiratory measurements were conducted at the patient bedside. On completion of the study, the treating clinician was asked to give a description of the cough sound quality.
Cough monitor system
Measurements were made using the LCM, a semi-automated cough frequency measurement system (13,19,20). The LCM consists of a portable sound recording device (Digital Voice Tracer LFH0662, Philips Electronics UK Ltd, Guildford, England), which is worn in a pouch or pocket. The small microphone is clipped onto the patient’s collar or lapel, as close as possible to the anterior neck. A continuous sound recording is made for up to 24 hours. The digital recording is then processed through accompanying computer software, which automatically registers sound patterns typical for cough. During data processing, a human operator listens to examples of identified sound patterns that are presented by the software and confirms whether or not the sound is in fact a cough sound. Sounds sometimes misidentified are, for example, short sharp sounds such as the closing of doors or dropping of objects, or snippets of talk or laughter. The cough count is refined through the feedback provided by the human operator. The software output provides the total number of cough events over the entire time period of recording and average hourly cough frequency.
Data collection
Participants wore the LCM for 15 minutes, while a researcher observed the participant from close proximity ‘live’ for the entire duration of the recording. The researcher conducted a visual live observer count of participant coughs (patient coughs), and of all other coughs which could be heard by the researcher in the environment, including coughs from other patients, staff and visitors within the area (environmental coughs). Recordings were conducted in the late mornings or early afternoons. During all recordings, there were other patients, visitors and/or staff present in the area. To ensure that there were sufficient cough events recorded, the participant and other people in the room were prompted to cough several times during the 15 minutes recording, thereby simulating the conditions that might lead to contamination of cough recordings through environmental coughs.
LCM cough frequency
To obtain the LCM semi-automated cough count, recordings were processed by an operator who was blinded to the visual live observer count. When giving operator input to cough sound recognition, the operator consciously assessed for each sound sample presented by the programme: (I) whether the sound was a cough sound or not (designating sounds that did not resemble cough sounds as ‘non-cough’); (II) if the sound was a cough sound, whether it sounded distant or near on the recording (designating distant sounding coughs as ‘non-cough’); and (III) if the sound was a cough sound, whether it resembled the participant’s cough sound from this input phase (for example, considering whether the participant’s cough sound had particular characteristics, such as a wheezing cough quality, which could assist in distinguishing the test subject’s coughs from coughs generated by other persons; and considering whether the cough came from a person of the opposite sex to the participant). LCM output consisted of cough frequency for the 15-minute recording, as well as a list of timings to the nearest second for all identified cough events.
Manual cough count
Manual cough counts from sound files (considered the gold standard by some investigators) were conducted by a researcher who was blinded to the visual live observer counts and to LCM counts (14). The researcher listened (without visualisation of sound traces) to the same recordings that were processed with the LCM. Reference samples of participants’ typical cough sounds were available to the researcher. Each manually counted cough was identified as either a patient cough or a non-patient cough (environmental cough), based on characteristics of the patient cough reference sample, considering whether the cough came from a person of the opposite sex to the patient, and loudness of the cough (environmental coughs being quieter or sounding ‘distant’ on the recording). The timing of each manually counted cough was noted (to the nearest second).
Data analysis
Cough frequencies obtained from the visual live observer count, from the manual sound file count, and from the LCM count were compared descriptively and by calculating the intra-class correlation coefficient for absolute agreement between the three measurement methods, using a two-way mixed-effects model for individual agreement in SPSS statistical software (IBM SPSS Statistics v22). Timings of identified cough events from the manual count and the LCM were cross-referenced, and agreement between the two was analyzed calculating Spearman’s rho, the proportion of exact agreements, Cohen’s kappa, sensitivity, specificity, positive and negative predictive values, and their respective 95% confidence intervals (CI) (21). Exact CIs (22) were calculated for sensitivity, specificity, and positive and negative predictive values using SPSS statistical software.
Results
Sample
The sample consisted of two women and three men within the first month following acute stroke. Clinical characteristics of the five study participants are given in Table 1. Participants’ cough sound qualities included ‘normal’ explosive cough sounds (patients 3 and 4), as well as weak (patients 1 and 2) and ‘bovine’ (patient 5) cough sound qualities.
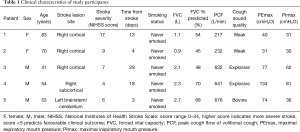
Full table
Cough frequency according to live observer count, manual sound-file count and LCM count
Table 2 presents cough frequencies according to visual live observer count, manual sound file count and LCM count. Live observer counts corroborate manual cough counts of patient and environmental coughs. Discrepancies between observer and manual count ranged from 0–2 coughs (≤8% of manual count).
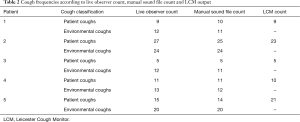
Full table
Cough frequencies obtained from manual count and LCM output correspond well for patients 1–4, with discrepancies ranging from 0–2 coughs (≤10% of manual count). For patient 5 (bovine cough sound), there was a discrepancy of 7 coughs between manual count and LCM output. From the data in Table 2, the intra-class correlation coefficient (95% CI) was 0.94 (0.74, 0.99) for all patients, and 0.98 (0.92, >0.99) only including patients 1–4.
For patient 5, we also processed a 24-hour recording (recorded on the same day as the 15-minute validation recording) using the same operator input as described in our methods. LCM 24-hour cough frequency was 280 coughs, or 11.7 coughs per hour. We verified each sound event that was designated by the LCM as a cough by listening to the corresponding section of the sound recording. Out of 280 designated cough events, only 3 (1%) were coughs likely to be from persons other than the patient.
Agreement between manual sound file count and LCM count
Agreement between the manual sound file count and the LCM count according to the timings of identified cough events is presented in Table 3 (individual patients) and Table 4 (entire sample). For individual patients, manual sound file count and LCM count were highly correlated, with Spearman’s rho of 1.0 (P<0.01) for coughs classified as patient coughs, and 0.95 (P=0.014) for coughs classified as non-patient (environmental) coughs. For the entire sample, the proportion of exact agreements was 0.92, and kappa was 0.84 (95% CI: 0.75, 0.93). LCM sensitivity was 0.94 (95% CI: 0.84, 0.98), specificity was 0.91 (95% CI: 0.82, 0.96), positive predictive value was 0.90 (95% CI: 0.79, 0.95) and negative predictive value was 0.95 (95% CI: 0.86, 0.98).
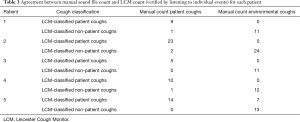
Full table

Full table
Discussion
This preliminary validation study of the LCM system has shown good agreement between LCM semi-automated cough counts and the gold-standard manual cough count, both for the detection of patient cough events and for the discrimination of patient and environmental coughs. Sensitivity of ≥0.90 has been recommended for cough monitoring to be clinically useful (2). With a sensitivity of 0.94 in our study, LCM performance is consistent with other cough monitor systems (2,23-26). However, it has to be acknowledged that the performance of systems may vary according to patient population and the specific circumstances of application, for example the duration of recording and the environment. Our data also lend support to the validity of the LCM system for application in patient groups with neurological impairment, where the cough sound might present with a weak or bovine sound quality as opposed to the normal characteristic explosive cough sound. Our analysis highlights that even the weak cough sounds of patients 1 and 2 were accurately assessed by the system.
We describe a validation method through cross-referencing of cough events according to their timings on the sound recording. This allows a one-to-one comparison of each sound event that is designated and counted as a patient cough by the LCM system against the coughs identified by manual sound file count. We conducted manual counts from sound as opposed to video recordings. Using video (capturing the patient’s face) may provide an additional layer of visual verification of manual counts, although both methods are considered equally valid (14,27). In our study we used the visual live observer count to corroborate manual sound file counts, in particular with respect to the ratio of patient to environmental coughs. The small discrepancies between these two methods are best explained by the difficulty of maintaining attention to the entire environment throughout the period of live observation.
In our study, LCM cough counts from patient 5 differed considerably from the manual count, over-counting by seven coughs. Cross-referenced timings of cough events showed that this was due to non-patient coughs, which were counted as patient coughs by the LCM during a sequence when a number of interchanging patient and non-patient coughs occurred very closely together and partly overlapped; and also distinct non-patient coughs, which occurred in isolation, which were counted as patient coughs by the LCM. This patient’s cough sound had a bovine quality, in accord with their clinical presentation with brainstem and cerebellar stroke. ‘Bovine’ cough describes a non-explosive or hollow sound quality of cough related to vocal cord weakness or dysfunction, typically caused by peripheral damage to the vagus nerve or the relevant central nervous pathways (28,29). We considered that the LCM software algorithm may require learning from a longer sound recording to fine-tune the automated detection algorithm for this particular cough sound quality. To investigate this possibility, we processed a 24-hour recording from the same patient (recorded on the same day as the 15-minute validation recording) using the same operator input as described in our methods. LCM incorrectly classified only 1% of environmental coughs as patient coughs.
Alternative to sound-based cough monitor systems, some devices record cough signals directly from the chest wall, for example using a contact microphone, accelerometer or electromyography (14,30). Cough frequency may be obtained from these signals directly; or these signals may be used in conjunction with sound recordings, one serving to verify the other. The addition of a throat-mounted accelerometer, for example, has been shown to improve the performance of a sound-based cough detection system (31). A disadvantage of these methods for application in an acute stroke unit setting may be that sensors applied to the chest wall under clothing can become displaced, in particular during the frequent moving and handling of patients by staff that takes place during nursing and rehabilitation activities on a stroke unit. When recording over many hours, a system such as the LCM may be of advantage, as the position of the microphone is evident to members of staff and also easily adjusted or corrected, whereas displaced sensors under clothing may go unnoticed for a long time, leading to loss of signal, and may also require specific knowledge for correct re-positioning (for example when using electromyography over a particular muscle).
The possibility of objective cough frequency measurement in neurologic patient groups and in acute ward settings may lead to new insights about cough frequency in dysphagia and aspiration, an aspect that has attracted some interest in the past, but has been restricted by the limitations of data collection through subjective reporting of coughing episodes (6,32). Potential areas for future research could be the correlation between dysphagia severity, reflex cough sensitivity and cough frequency; or the temporary distribution of coughs in relation to the time of ingestion of food and drink.
We acknowledge that the present study is limited by the small number of patients and the relatively short duration of recordings. Taking into consideration that manual cough analysis is a very labour intensive method, our data make a relevant contribution to validating the cough monitor. Nevertheless, we view our results as preliminary, and studies with larger samples are required to yield greater statistical precision.
Conclusions
The semi-automated cough monitor examined in this study demonstrated good ability to detect and discriminate patient cough from environmental cough. The device may provide an appropriate method for measuring cough frequency in an acute stroke unit environment. Further validation studies using recordings of longer duration are recommended to describe the level of accuracy with greater precision and inform interpretation of cough frequency measurements in this clinical setting.
Acknowledgements
Funding: This article presents independent research, part-funded by the National Institute for Health Research (NIHR), Research for Patient Benefit Programme (Grant Reference PB-PG-0408-16096).
Footnote
Conflicts of Interest: SS Birring is developer of the LCM system. The other authors have no conflicts of interest to declare.
Disclaimer: The views expressed are those of the authors and not necessarily those of the NHS, the NIHR or the Department of Health.
References
- Birring SS. Controversies in the evaluation and management of chronic cough. Am J Respir Crit Care Med 2011;183:708-15. [Crossref] [PubMed]
- Smith J, Woodcock A. New developments in the objective assessment of cough. Lung 2008;186 Suppl 1:S48-54. [Crossref] [PubMed]
- Brignall K, Jayaraman B, Birring SS. Quality of life and psychosocial aspects of cough. Lung 2008;186 Suppl 1:S55-8. [Crossref] [PubMed]
- Raj AA, Birring SS. Clinical assessment of chronic cough severity. Pulm Pharmacol Ther 2007;20:334-7. [Crossref] [PubMed]
- Birring SS, Matos S, Patel RB, et al. Cough frequency, cough sensitivity and health status in patients with chronic cough. Respir Med 2006;100:1105-9. [Crossref] [PubMed]
- Smith Hammond CA, Goldstein LB. Cough and aspiration of food and liquids due to oral-pharyngeal dysphagia: ACCP evidence-based clinical practice guidelines. Chest 2006;129:154S-168S. [Crossref] [PubMed]
- Renovanz HD, Heissler K. Tussometry. I. Review of methods. Wien Med Wochenschr Suppl 1979;58:1-7. [PubMed]
- Loudon RG, Brown LC. Cough frequency in patients with respiratory disease. Am Rev Respir Dis 1967;96:1137-43. [PubMed]
- Woolf CR, Rosenberg A. Objective assessment of cough suppressants under clinical conditions using a tape recorder system. Thorax 1964;19:125-30. [Crossref] [PubMed]
- Birring SS, Spinou A. How best to measure cough clinically. Curr Opin Pharmacol 2015;22:37-40. [Crossref] [PubMed]
- Leconte S, Ferrant D, Dory V, et al. Validated methods of cough assessment: a systematic review of the literature. Respiration 2011;81:161-74. [Crossref] [PubMed]
- Kulnik ST, Birring SS, Moxham J, et al. Does respiratory muscle training improve cough flow in acute stroke? Pilot randomized controlled trial. Stroke 2015;46:447-53. [Crossref] [PubMed]
- Birring SS, Fleming T, Matos S, et al. The Leicester Cough Monitor: preliminary validation of an automated cough detection system in chronic cough. Eur Respir J 2008;31:1013-8. [Crossref] [PubMed]
- Morice AH, Fontana GA, Belvisi MG, et al. ERS guidelines on the assessment of cough. Eur Respir J 2007;29:1256-76. [Crossref] [PubMed]
- NIH Stroke Scale, 1999. Available online: http://www.nihstrokescale.org/
- Miller MR, Hankinson J, Brusasco V, et al. Standardisation of spirometry. Eur Respir J 2005;26:319-38. [Crossref] [PubMed]
- American Thoracic Society/European Respiratory Society. ATS/ERS Statement on respiratory muscle testing. Am J Respir Crit Care Med 2002;166:518-624. [Crossref] [PubMed]
- Kulnik ST, Rafferty GF, Birring SS, et al. A pilot study of respiratory muscle training to improve cough effectiveness and reduce the incidence of pneumonia in acute stroke: study protocol for a randomized controlled trial. Trials 2014;15:123. [Crossref] [PubMed]
- Matos S, Birring SS, Pavord ID, et al. Detection of cough signals in continuous audio recordings using hidden Markov models. IEEE Trans Biomed Eng 2006;53:1078-83. [Crossref] [PubMed]
- Matos S, Birring SS, Pavord ID, et al. An automated system for 24-h monitoring of cough frequency: the leicester cough monitor. IEEE Trans Biomed Eng 2007;54:1472-9. [Crossref] [PubMed]
- Altman DG. Practical Statistics for Medical Research. Boca Raton: Chapman & Hall/CRC, 1991.
- Deeks JJ, Altman DG. Sensitivity and specificity and their confidence intervals cannot exceed 100%. BMJ 1999;318:193-4. [Crossref] [PubMed]
- Sterling M, Rhee H, Bocko M. Automated Cough Assessment on a Mobile Platform. J Med Eng 2014;2014. pii: 951621.
- Turner RD, Bothamley GH. How to count coughs? Counting by ear, the effect of visual data and the evaluation of an automated cough monitor. Respir Med 2014;108:1808-15. [Crossref] [PubMed]
- Barry SJ, Dane AD, Morice AH, et al. The automatic recognition and counting of cough. Cough 2006;2:8. [Crossref] [PubMed]
- Coyle MA, Keenan DB, Henderson LS, et al. Evaluation of an ambulatory system for the quantification of cough frequency in patients with chronic obstructive pulmonary disease. Cough 2005;1:3. [Crossref] [PubMed]
- Smith JA, Earis JE, Woodcock AA. Establishing a gold standard for manual cough counting: video versus digital audio recordings. Cough 2006;2:6. [Crossref] [PubMed]
- Hughes TA, Wiles CM. Neurogenic dysphagia: the role of the neurologist. J Neurol Neurosurg Psychiatry 1998;64:569-72. [Crossref] [PubMed]
- Korpás J, Sadlonová J, Vrabec M. Analysis of the cough sound: an overview. Pulm Pharmacol 1996;9:261-8. [Crossref] [PubMed]
- Spinou A, Birring SS. An update on measurement and monitoring of cough: what are the important study endpoints? J Thorac Dis 2014;6:S728-34. [PubMed]
- Fan J, Comina G, Gilman R, et al. Cough monitoring for pulmonary tuberculosis using combined microphone/accelerometer measurements. J Acoust Soc Am 2014;135:2268. [Crossref]
- Hadjikoutis S, Eccles R, Wiles CM. Coughing and choking in motor neuron disease. J Neurol Neurosurg Psychiatry 2000;68:601-4. [Crossref] [PubMed]