Identification of the key transcription factors in esophageal squamous cell carcinoma
Introduction
Esophageal cancer (EC) is a common human malignancy with poor survival. The incidence rate of EC was seventh and sixth in male and female cancer worldwide, respectively. Moreover, EC was usually diagnosed at an advanced stage. It is known that an estimated 455,800 new EC cases and 400,200 deaths occurred in 2012 worldwide (1).
EC is classified as two major subtypes including esophageal adenocarcinoma (EAC) and esophageal squamous cell carcinoma (ESCC) according to histopathology appearance (2). In recent years, the incidence rates of EAC had a markedly decrease in western countries such as the United States, Australia, France and England. On the contrary, the incidence rates of ESCC have been increasing in Asian countries (1). ESCC subtype accounts for more than 90% EC patients in Asian countries such as China and Japan.
Currently, the major etiological factors of ESCC are known as environmental factors, human papillomavirus (HPV) infection and genetic susceptibility. The environmental factors include alcohol consumption and tobacco exposure. The case-control studies show that exposure to secondhand smoking and heavy alcohol intake are risk factors for ESCC (3,4). HPV is one of risk factors of ESCC and HPV status is an independent prognostic factor for survival among patients with ESCC (5). Beyond that, CYP1A1 Val/Val and CYP2E1 c1/c1 genotypes are significantly associated with ESCC risk (6). MiR-34b/c rs4938723 T>C, miR-423 rs6505162 C>A, miR-196a2 rs11614913 and miR-499 rs3746444 polymorphisms are associated with susceptibility to ESCC in Chinese population (7,8).
Transcription factors (TFs) commonly regulating gene expression through binding to specific DNA sequences and involves in tumor cell processes including cell proliferation, apoptosis and migration. It is reported that over-expression of zinc finger E-box binding to homeobox factor 1 promotes aggressive ESCC progression and contributes to the unfavorable prognosis in ESCC (9). SOX2 promotes the EMT of ESCC cells by modulating slug expression through the activation of STAT3/HIF-α signaling, and it suggests that SOX2 promotes the metastasis process of ESCC (10).
In this study, bioinformatics analyses were applied to integrate mRNA expression profiling of ESCC, which were available in the Gene Expression Omnibus (GEO) database. Identification of differentially expressed genes (DEGs) in ESCC and construction of TFs-target genes regulatory network were subjected to screen the key TFs in ESCC. This study aimed to provide valuable information for further pathogenesis mechanism elucidation, and identify cancer biomarkers of diagnosis, prognosis and therapeutic targets in ESCC.
Methods
Microarray data collection
The microarray expression profiles of ESCC were obtained from GEO (http://www.ncbi.nlm.nih.gov/geo/) (11). The inclusion criteria of datasets were as follows: (I) expression profiling of ESCC tumor tissues/cell lines and normal controls were available in the datasets; (II) patients without preoperative treatment before esophagectomy and cell lines without drug stimulation. A total of ten mRNA expression datasets of ESCC were incorporated into our study.
Data preprocessing and identification of DEGs
The raw expression datasets were downloaded and pre-processed to perform background subtraction, quantile normalization and log2 transformation before using moderated t-tests within Bioconductor package Limma (Linear Models for Microarray Data). Subsequently, the Linear Models Limma (12) package for microarray data was used to screen DEGs between ESCC and normal controls. The P values was adjusted to false discovery rate (FDR) through Benjamini and Hochberg method (13). The threshold for the DEGs screening was set as FDR <10−5. Hierarchical cluster analysis of group samples based on expression levels of mRNA were visualized through “pheatmap” package in R language.
Gene ontology (GO) and pathway enrichment analysis of DEGs
The GO annotation analysis has commonly used for functional studies of large-scale transcriptomics data. The Kyoto Encyclopedia of Genes and Genomes (KEGG) pathway database contains biochemistry pathways. Online software of Gorilla and GeneCoDis3 were utilized for GO annotation and KEGG pathway enrichment of DEGs, respectively (14,15). The threshold of GO and KEGG enrichment of DEGs were P<0.001 and FDR <0.05, respectively.
Construction of TFs-target genes regulatory network
TRANSFAC (http://www.gene-regulation.com/pub/databases.html) provides data of eukaryotic TFs, experimentally-proven binding sites of TFs and consensus binding sequences (16). Data of position weight matrix (PMW), binding sites motif of TFs were downloaded from TRANSFAC and used for identification of TFs and target-genes. The identified TFs and target-genes were used for regulatory network construction. The regulatory network was visualized by Cytoscape (http://cytoscape.org/) (17). Nodes represented TFs or target-genes and solid line represented correlation between TF and target-gene.
qRT-PCR verification of DEGs and TFs in ESCC
Five pairs of ESCC tumor and adjacent non-tumor tissues were obtained from five Chinese patients underwent surgery in Department of Thoracic Surgery, The Fourth Hospital of Hebei Medical University. The detail information of subjects was shown in Table S1. Our study was approved by the Ethics Committee of The Fourth Hospital of Hebei Medical University (No. 2015MEC073). All of patients signed the consent form and our study compiled with the Declaration of Helsinki.
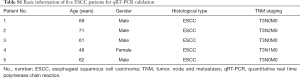
Full table
Total RNA of fresh five paired ESCC tumor and adjacent non-tumor specimens were extracted using TRIzol reagent (Invitrogen, Carlsbad, CA, USA). The SuperScript III Reverse Transcription Kit (Invitrogen, Carlsbad, CA, USA) was used to synthesize the cDNA. qRT-PCR reactions were performed using Power SYBR Green PCR Master Mix (Applied Biosystems, Foster City, CA, USA) on the Applied Biosystems 7500 (Applied Biosystems, Foster City, CA, USA). β-actin was used as internal control for mRNA detected. The relative expression of DEGs was calculated through the comparative Ct methods (18). The PCR primers were used as Table S2 shown.
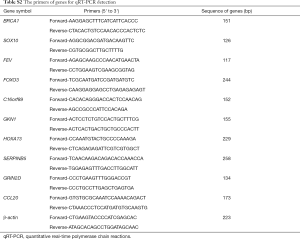
Full table
Stage and survival analysis of TFs in ESCC
To better research the clinical relevance of these TFs in ESCC. We downloaded an illumina HiSeq RNA-Seq data from The Cancer Genome Atlas (TCGA) which consisted of 94 ESCC samples. This TCGA data were used to evaluate the correlation between survival and the expression of TFs in ESCC. In addition, the correlation between stage and the expression of TFs in ESCC were evaluated based on this TCGA data as well.
Results
DEGs in ESCC
We collected ten mRNA expressions profiling including 238 ESCC cases and 142 normal controls (Table 1) from GEO database. With a cut-off value of FDR <10−5, a total of 1,248 DEGs including 663 up- and 585 down-regulated genes were identified in ESCC compared to normal controls. The top 10 up- and down-regulated genes were listed in Table 2. The most up- and down-regulated gene was CST1 and PGA4 in ESCC, respectively. The full list of DEGs was shown in http://jtd.amegroups.com/public/addition/jtd/supp-jtd.2017.12.27.pdf. The top 200 up- and down-regulated DEGs in ESCC compared with normal controls were subjected to heatmap analyses. As Figure 1 shown, the difference of expression pattern of DEGs between ESCC and normal controls was notable.
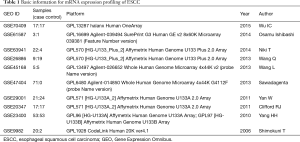
Full table
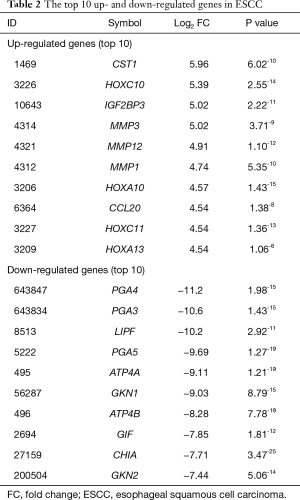
Full table
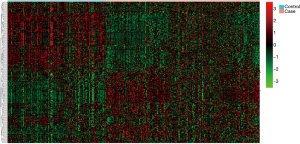
Due to the restriction of GEO, only little clinical information of samples of these ten datasets can be obtained by GEO. The information of gender and age of samples can be obtained from only four datasets (GSE70409, GSE61587, GSE45168 and GSE47404), which accounts for 31.5% (n=119) of all samples. The information of TNM staging or histological differentiation of samples can be obtained from only two datasets (GSE45168 and GSE47404), which accounts for 21.4% (n=81) of all samples. Hence, it is difficult to analyze the confounding effects of age, gender, TNM staging, and histological differentiation on expression of TFs and DEGs in ESCC. Considering the race of ESCC samples in these ten datasets, 57.2% (n=135) ESCC samples were obtained from Asian (China and Japan), 42.4% ESCC samples were obtained from Non-Asian (USA and Germany). Considering the race of control samples in these ten datasets, 19.7% (n=28) samples were obtained from Asian (China and Japan), 79.6% (n=113) samples were obtained from Non-Asian (USA and Germany).
GO annotation of DEGs in ESCC
To understand the biological roles of DEGs in ESSC, GO analysis were performed. The threshold of GO terms was P value <0.001. The system process (GO:0003008) was the most significant enrichment of biological process; substrate-specific transmembrane transporter activity (GO:0022891) was the highest enrichment term of molecular function; and membrane part (GO:0044425) was the highest enrichment term of cellular component, as Table 3 shown.
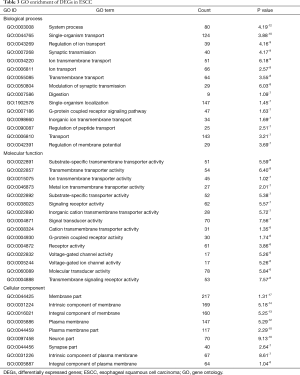
Full table
KEGG pathway enrichment of DEGs in ESCC
KEGG enrichment analysis was performed to obtain the signaling pathways of DEGs in ESCC. The threshold was FDR <0.05. The significantly enriched signaling pathways consisted of cell cycle, DNA replication and oocyte meiosis, as Table 4 shown.
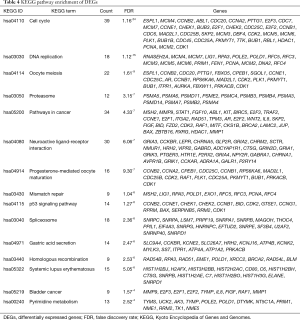
Full table
Construction of TFs-genes regulatory network
TF-gene pairs were selected based on the TRANSFAC database and a transcriptional regulatory network was constructed. Total of 26 TFs including 15 up- and 11 down-regulated genes (Table S3), and corresponding target-genes were identified in the DEGs of ESCC. TFs and targets-genes were carried out to construct regulatory network by Cytoscape software, as Figure 2 shown. The network was consisted of 631 nodes and 882 edges between 21 TFs and 610 target-genes. The TFs had high connectivity with target-genes including BRCA1 (degree =92), SOX10 (degree =89), NFIC (degree =82), ARID3A (degree =82) and ZNF354C (degree =79), as Figure 2 and Table S4 shown.
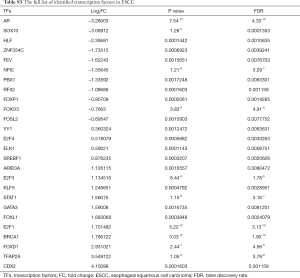
Full table
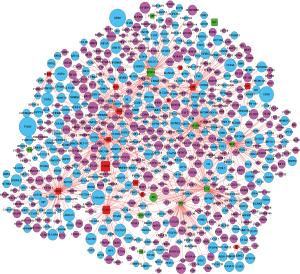
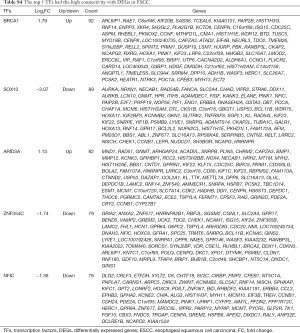
Full table
qRT-PCR verification of DEGs and TFs in ESCC
To validate the microarray analysis data, a number of TFs and top 20 up- and down-regulated DEGs were used to perform qRT-PCR verification. The expression levels of DEGs and TFs including BRCA1, SOX10, FEV, C16orf89, HOXA13, SERPINB5, GRIN2D and CCL20 were detected by qRT-PCR in five paired ESCC tumor and adjacent non-tumor tissues derived from Chinese patients with ESCC. As shown in Figure 3A, BRCA1 was significant up-regulated in ESCC. SOX10, had the up-regulation tendency in ESCC (Figure 3B). FEV had the down-regulation tendency in ESCC (Figure 3C). C16orf89 was significantly down-regulated in ESCC (Figure 3D). HOXA13, SERPINB5, CRIN2D and CCL20 had the up-regulation tendency in ESCC (Figure 3E,F,G,H), respectively. Although most of the qRT-PCR results were not statistically significant, the regulation trends of candidate DEGs and TFs in our qRT-PCR were generally consistent with our integrated analysis.
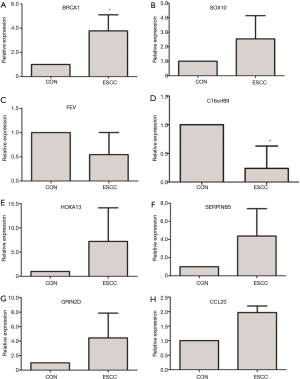
Stage and survival analysis of selected TFs in ESCC
Due to lack of clinical information in GEO, we downloaded an illumina HiSeq RNA-Seq data that consisted of 94 ESCC samples from TCGA. Based on this illumina HiSeq RNA-Seq data, the correlation between survival and the expression levels of the 26 TFs were evaluated. Among which, high expression of SREBF1 (P=0.0427) and TFAP2A (P=0.0422) were significantly correlated with the longer overall survival time of patients with ESCC (Figure 4). The correlation between stage and the expression levels of eight TFs including BRCA1, SOX10, FEV, ARID3A, ZNF354C, NFIC, TFAP2A and SREBF1 were evaluated. The expression of these eight TFs in stage I–IV of patients with ESCC in TCGA were displayed in Figure 5 which shown the association between the expression of TFs and the severity of ESCC.
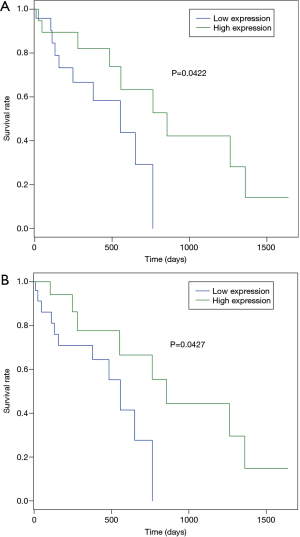
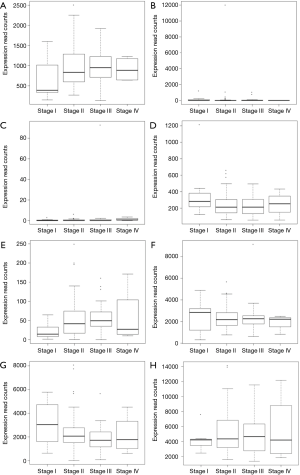
Discussion
Due to the differences of samples and platforms in various microarray studies, integrated analysis of various microarray datasets could obtain more accurate disease-related regulators with a larger sample size than an individual microarray. Our previous study has identified key DEGs in ESCC by integrated analysis of five microarray datasets from GEO (19). With GEO database updated, we identified DEGs in ESCC by integrated analysis of ten microarray datasets with larger sample size in this study. Moreover, the key TFs in ESCC were further identified based these ESCC-related DEGs.
In the TFs-target genes regulatory network, BRCA1, SOX10, ARID3A, ZNF354C and NFIC had the high connectivity with DEGs, which regulated 92, 89 and 82, 79 and 78 DEGs, respectively.
BRCA1 was significantly up-regulated in ESCC compared to paired non-tumor tissues. BRCA1 locates on chromosome 17 and encodes breast cancer 1. BRCA1 is commonly known as mutations in this gene contribute to inherited breast cancers and ovarian cancers. In addition to, it is reported that high expression of BRCA1 is responsible for chemotherapy resistance in ESCC treatment through experimental study in vitro and clinical observation. Low expression of BRCA1 correlates with response rate of patients in advanced ESCC treated with cisplatin-/docetaxel-based chemoradiotherapy (20). Dasatinib enhances cisplatin sensitivity in human ESCC cells through suppression of PI3K/AKT and STAT3 pathways, and suppression of cisplatin-resistant molecules including BRCA1 and ERCC1 (21). In our study, BRCA1 regulated C16orf89, which was significantly down-regulated in ESCC through microarray analysis and qRT-PCR verification (Figure 3D). BRCA1 might be potential biomarkers for prognostic evaluation of chemotherapy treatment in ESCC patients.
SOX10 encodes SRY-box 10, a member of the RY-related HMG (high mobility group)-box family of TFs. This family is shared the highly conserved HMG sequences similarities. Our study demonstrated that SOX10 regulated two of top 20 up-regulated DEGs including HOXA13 and SERPINB5. The expression of these two DEGs had the up-regulated tendency in ESCC (Figure 3E,F) and SERPINB5 was significantly enriched in p53 signaling pathway (Table 4). SOX10 is broadly expressed in human normal adult tissues and fetal tissues. Previous studies present that SOX10 is frequently silenced by promoter CpG methylation in colorectal cancer, gastric cancer and ESCC cell lines (22-24). The expression status of SOX10 in ESCC in the report is consistent with our study that SOX10 was down-regulated in ESCC compared to normal controls through bioinformatics analysis and qRT-PCR validation. The molecular function of SOX10 in ESCC has been elucidated through experimental in vitro. Decreased mRNA expression of SOX10 inhibits cell growth and metastasis of ESCC via suppressing the Wnt/β-catenin pathway (22). The experiment in vivo of biological function of SOX10 in ESCC need to be further explored.
NFIC encodes nuclear factor I/C (CCAAT-binding TF), belongs to CTF/NF-I family. NFIC was down-regulated and regulated 78DEGs in the TFs-genes regulatory network of ESCC (Figure 2). The bioinformatics analysis of gene expression profiling reveals NFIC was dysregulated in gastric cancer and might contribute to the pathogenesis (25). NFI-C2, a dominating NFIC protein, it is lost during mammary tumor progression and is almost invariably absent from lymph node metastases. Enhanced expression of NFI-C2 abolishes tumorigenicity and inhibits EMT and invasiveness of breast cancer through regulating the expression of FOxF1 in vitro and in nude mice (26). In addition to, NFIC suppresses EMT, migration, and invasion in breast cancer cells through regulating the expression level of E-cadherin and KLF4 (27). To our knowledge, NFIC was a novel TF, which have not been reported to be related to ESCC before. NFIC was a key TF in the regulatory network (Figure 2). Based on the abovementioned, NFIC might play essential roles in the carcinogenesis and development of ESCC. The explicit biological functions of NFIC in ESCC need to be further elucidated through experimental exploration in vitro and in vivo.
FEV was one of down-regulated TFs in ESCC (Figure 3C). C16orf89 and GIN2D were top 20 down-regulated and top 20 up-regulated DEGs of ESCC in our study. Both of them were targeted by FEV. C16orf89, a common putative target of BRCA1 and FEV, is significantly down-regulated (Figure 3D) and GIN2D had the up-regulated tendency (Figure 3G) in ESCC; CCL20 was the top 10 up-regulated DEGs and had the up-regulated tendency in ESCC compared to adjacent non-tumor tissues. In a word, the results of qRT-PCR verification of DEGs and TFs were consistent with our microarray analysis; it suggested that our integrated analysis was acceptable.
The other two TFs, ARID3A and ZNF354C, was identified as up- and down-regulated in ESCC, respectively. ARID3A, encodes AT-rich interaction domain 3A, is a member of the ARID family of DNA-binding proteins. Previous study indicates that ARID3A is dysregulated in colorectal cancer (CRC) and might be potentially prognostic biomarker of CRC (28). In addition, ARID3A is over-expressed in stomach cancer (29). ZNF354C encodes zinc finger protein 354C. ZNF354C is a key TF in gastric cardia adenocarcinoma (GCA) that dysregulated genes in GCA compared with adjacent normal tissues tend to be bound by ZNF354C (30). ARID3A and ZNF354C were novel TFs, which have not been reported to be related to ESCC before. The expression status of ARID3A and ZNF354C need to be validated through large sample size of ESCC specimens; the molecular functions need to be explored through experimental observation.
Taken together, these five TFs (BRCA1, SOX10, ARID3A, ZNF354C and NFIC) mentioned above were identified key TFs in ESCC. To better research the molecular mechanism of these five key TFs in ESCC. We obtained the common targeted genes of these five key TFs including CDC25C, MCM6, ABL1, CHEK1, CCNB1, MCM7, TTK, CDK2, CDC20 and BUB1B (underlined genes in Tables S4 and 4). Moreover, all these ten genes were significantly enriched in cell cycle pathway based on the KEGG enrichment analysis. Deregulation of the cell cycle is one of the most frequent alterations during tumor development. Cancer originates from the deregulation of regulators of cell cycle (31). We speculated that these five key TFs may play important roles in the development in ESCC by modulating the cell cycle of ESCC cancer cells through regulating these ten cell cycle-related DEGs. Further research was needed to explore the precise roles of these TFs and DEGs in ESCC.
After evaluating the correlation between TFs and survival in patients with ESCC, we found that high expression of SREBF1 and TFAP2A were significantly correlated with the longer overall survival time of patients with ESCC. These two TFs may also play important roles in ESCC which may be potential prognostic biomarkers of ESCC.
In conclusion, our integrated analysis identified key TFs in ESCC which provides clues for exploring the mechanism of ESCC. However, there are limitations in our study. Firstly, the small size of the clinical samples for qRT-PCR validation was small. Secondly, only little clinical information of samples of these ten datasets used in our study can be obtained by GEO. Hence, it is difficult to analyze the confounding effects of age, gender, TNM staging, and histological differentiation on gene expression in ESCC. Thirdly, there were radical differences of samples used between our integrated analysis and qRT-PCR results. Fourthly, whether these TF-gene sets are only specific to ESCC or just related to esophageal cancer or cancer was uncertain. All these four limitations may be the reasons why most of qRT-PCR results were not significant. Lastly, the five TFs of FEV, ARID3A, ZNF354C, SREBF1 and TFAP2A were identified as the novel TFs in ESCC, but the biological functions of the three TFs were not explored in our work. Thus, the large sample size of ESCC specimens will be collected and validated through qRT-PCR verification; the confounding effects of clinical characteristics such as age, gender, histological differentiation and race on gene expression in ESCC need to be analyzed in future research; further investigation of these TF-gene sets in EAC and other cancers was needed to identify the specificity of these TF-gene sets in ESCC, further studies are needed to investigate the precise role of TFs and target-genes in ESCC in the future work as well.
Acknowledgements
Funding: The work was supported by Major Medical Scientific Research Subject of Hebei Province (zd2013044) and Medical Application Tracking Project of Hebei Province (GL201637).
Footnote
Conflicts of Interest: The authors have no conflicts of interest to declare.
Ethical Statement: Our study was approved by the Ethics Committee of The Fourth Hospital of Hebei Medical University (No. 2015MEC073). All of patients signed the consent form and our study compiled with the Declaration of Helsinki.
References
- Siegel R, Naishadham D, Jemal A. Cancer statistics, 2012. CA Cancer J Clin 2012;62:10-29. [Crossref] [PubMed]
- Rustgi AK, El-Serag HB. Esophageal Carcinoma. New Engl J Med 2014;371:2499-509. [Crossref] [PubMed]
- Kumagai N, Wakai T, Akazawa K, et al. Heavy alcohol intake is a risk factor for esophageal squamous cell carcinoma among middle-aged men: A case-control and simulation study. Mol Clin Oncol 2013;1:811-6. [Crossref] [PubMed]
- Rafiq R, Shah IA, Bhat GA, et al. Secondhand Smoking and the Risk of Esophageal Squamous Cell Carcinoma in a High Incidence Region, Kashmir, India: A Case-control–observational Study. Medicine (Baltimore) 2016;95:e2340. [Crossref] [PubMed]
- Cao F, Han H, Zhang F, et al. HPV infection in esophageal squamous cell carcinoma and its relationship to the prognosis of patients in northern China. Scientific World Journal 2014;2014:804738. [PubMed]
- Bhat GA, Shah IA, Makhdoomi MA, et al. CYP1A1 and CYP2E1 genotypes and risk of esophageal squamous cell carcinoma in a high-incidence region, Kashmir. Tumour Biol 2014;35:5323-30. [Crossref] [PubMed]
- Yin J, Wang X, Zheng L, et al. Hsa-miR-34b/c rs4938723 T>C and hsa-miR-423 rs6505162 C>A polymorphisms are associated with the risk of esophageal cancer in a Chinese population. PLoS One 2013;8:e80570. [Crossref] [PubMed]
- Shen F, Chen J, Guo S, et al. Genetic variants in miR-196a2 and miR-499 are associated with susceptibility to esophageal squamous cell carcinoma in Chinese Han population. Tumour Biol 2016;37:4777-84. [Crossref] [PubMed]
- Yang X, Wang Q, Dai W, et al. Overexpression of zinc finger E-box binding homeobox factor 1 promotes tumor invasiveness and confers unfavorable prognosis in esophageal squamous cell carcinoma. Tumour Biol 2014;35:11977-84. [Crossref] [PubMed]
- Gao H, Teng C, Huang W, et al. SOX2 Promotes the Epithelial to Mesenchymal Transition of Esophageal Squamous Cells by Modulating Slug Expression through the Activation of STAT3/HIF-α Signaling. Int J Mol Sci 2015;16:21643-57. [Crossref] [PubMed]
- Edgar R, Domrachev M, Lash AE. Gene Expression Omnibus: NCBI gene expression and hybridization array data repository. Nucleic Acids Res 2002;30:207-10. [Crossref] [PubMed]
- Smyth GK. Limma: linear models for microarray data. In: Gentleman R, Carey VJ, Huber W, et al. editiors. Bioinformatics and computational biology solutions using R and Bioconductor. Berlin: Springer, 2005:397-420.
- Benjamini Y, Hochberg Y. Controlling the false discovery rate: a practical and powerful approach to multiple testing. J R Stat Soc Series B Stat Methodol 1995;57:289-300.
- Eden E, Navon R, Steinfeld I, et al. GOrilla: a tool for discovery and visualization of enriched GO terms in ranked gene lists. BMC Bioinformatics 2009;10:48. [Crossref] [PubMed]
- Carmona-Saez P, Chagoyen M, Tirado F, et al. GENECODIS: a web-based tool for finding significant concurrent annotations in gene lists. Genome Biol 2007;8:R3. [Crossref] [PubMed]
- Matys V, Fricke E, Geffers R, et al. TRANSFAC: transcriptional regulation, from patterns to profiles. Nucleic Acids Res 2003;31:374-8. [Crossref] [PubMed]
- Shannon P, Markiel A, Ozier O, et al. Cytoscape: a software environment for integrated models of biomolecular interaction networks. Genome Res 2003;13:2498-504. [Crossref] [PubMed]
- Schmittgen TD, Livak KJ. Analyzing real-time PCR data by the comparative C(T) method. Nat Protoc 2008;3:1101-8. [Crossref] [PubMed]
- Su P, Wen S, Zhang Y, et al. Identification of the Key Genes and Pathways in Esophageal Carcinoma. Gastroenterol Res Pract 2016;2016:2968106. [PubMed]
- Gao Y, Zhu J, Zhang X, et al. BRCA1 mRNA expression as a predictive and prognostic marker in advanced esophageal squamous cell carcinoma treated with cisplatin-or docetaxel-based chemotherapy/chemoradiotherapy. PLoS One 2013;8:e52589. [Crossref] [PubMed]
- Chen J, Lan T, Zhang W, et al. Dasatinib enhances cisplatin sensitivity in human esophageal squamous cell carcinoma (ESCC) cells via suppression of PI3K/AKT and Stat3 pathways. Arch Biochem Biophys 2015;575:38-45. [Crossref] [PubMed]
- Tong X, Li L, Li X, et al. SOX10, a novel HMG-box-containing tumor suppressor, inhibits growth and metastasis of digestive cancers by suppressing the Wnt/β-catenin pathway. Oncotarget 2014;5:10571-83. [Crossref] [PubMed]
- Ying J, Li H, Yu J, et al. WNT5A exhibits tumor-suppressive activity through antagonizing the Wnt/β-catenin signaling, and is frequently methylated in colorectal cancer. Clin Cancer Res 2008;14:55-61. [Crossref] [PubMed]
- Wang J, Xia Y, Li L, et al. Double restriction-enzyme digestion improves the coverage and accuracy of genome-wide CpG methylation profiling by reduced representation bisulfite sequencing. BMC Genomics 2013;14:11. [Crossref] [PubMed]
- Jiang HB, Yang TJ, Lu P, et al. Gene expression profiling of gastric cancer. Eur Rev Med Pharmacol Sci 2014;18:2109-15. [PubMed]
- Nilsson J, Helou K, Kovács A, et al. Nuclear Janus-activated kinase 2/nuclear factor 1-C2 suppresses tumorigenesis and epithelial-to-mesenchymal transition by repressing Forkhead box F1. Cancer Res 2010;70:2020-9. [Crossref] [PubMed]
- Lee HK, Lee DS, Park JC. Nuclear factor I-C regulates E-cadherin via control of KLF4 in breast cancer. BMC Cancer 2015;15:113. [Crossref] [PubMed]
- Song M, Kim H, Kim WK, et al. High expression of AT-rich interactive domain 3A (ARID3A) is associated with good prognosis in colorectal carcinoma. Ann Surg Oncol 2014;21 Suppl 4:S481-9. [Crossref] [PubMed]
- Samyesudhas SJ, Roy L, Cowden Dahl KD. Differential expression of ARID3B in normal adult tissue and carcinomas. Gene 2014;543:174-80. [Crossref] [PubMed]
- Ju F, Lu L, Zhao QY, et al. Systematic analysis of gene expression and molecular interactions in cardiac and non-cardiac gastric carcinomas. Hepatogastroenterology 2014;61:1835-42. [PubMed]
- Park MT, Lee SJ. Cell cycle and cancer. J Biochem Mol Biol 2003;36:60-5. [PubMed]