A discriminant function model as an alternative method to spirometry for COPD screening in primary care settings in China
Abstract
Objective: COPD is often underdiagnosed in a primary care setting where the spirometry is unavailable. This study was aimed to develop a simple, economical and applicable model for COPD screening in those settings.
Methods: First we established a discriminant function model based on Bayes’ Rule by stepwise discriminant analysis, using the data from 243 COPD patients and 112 non-COPD subjects from our COPD survey in urban and rural communities and local primary care settings in Guangdong Province, China. We then used this model to discriminate COPD in additional 150 subjects (50 non-COPD and 100 COPD ones) who had been recruited by the same methods as used to have established the model. All participants completed pre- and post-bronchodilator spirometry and questionnaires. COPD was diagnosed according to the Global Initiative for Chronic Obstructive Lung Disease criteria. The sensitivity and specificity of the discriminant function model was assessed.
Results: The established discriminant function model included nine variables: age, gender, smoking index, body mass index, occupational exposure, living environment, wheezing, cough and dyspnoea. The sensitivity, specificity, positive likelihood ratio, negative likelihood ratio, accuracy and error rate of the function model to discriminate COPD were 89.00%, 82.00%, 4.94, 0.13, 86.66% and 13.34%, respectively. The accuracy and Kappa value of the function model to predict COPD stages were 70% and 0.61 (95% CI, 0.50 to 0.71).
Conclusions: This discriminant function model may be used for COPD screening in primary care settings in China as an alternative option instead of spirometry.
Key words: COPD; Bayes’ Rule; spirometry
Introduction
Chronic obstructive pulmonary disease (COPD) has been predicted to become the fifth leading burden of disease in 2020 (1-3). Nevertheless, COPD is underdiagnosed (4) as most patients did not seek medical attention until they have serious respiratory symptoms. As reported in a recent Chinese population-based study (5), only 35.1% of the patients with “emphysema”, “asthma”, “bronchitis”, or “COPD” were identified by spirometry previously. Even in the U.S., 71.7% of the subjects with mild airflow limitation did not receive an appropriate diagnosis of obstructive lung disease (6).
Although spirometry is a “gold standard” for COPD detection, it is often underused in primary care settings, particularly in China (5) because it requires skills to operate and unfits for some patients. Our previous study (5) reported that only 6.5% of the patients with COPD had ever been tested by spirometry. Thus, it is of great value to develop a simple and economical method which can be used as an alternative option for spirometry to screen COPD and to predict the COPD stage in primary care settings. The aim of this study was to develop a mathematical model which can satisfy the above requirements.
Methods
Design
A total of 505 subjects (343 COPD patients and 162 non-COPD subjects), aged 40 years or over, were recruited from our previous population-based epidemiological study in the communities and local primary care settings in Shaoguan and Liwan, China. The protocol for the present population-based epidemiological survey was published elsewhere (7). The questionnaire and spirometry used for the present study was the same as used for COPD screening among outpatients at local primary care settings in 2008. According to the diagnostic criteria in Global Initiative for Chronic Obstructive Lung Disease (GOLD), COPD was diagnosed by a post-bronchodilator FEV1/FVC ratio <0.7 measured after administration of albuterol. Non-COPD subjects were randomly selected from our previous population-based epidemiological data set using computer. All patients with COPD and Non-COPD subjects selected in this study for analysis except for those with a pre-existing or concomitant non-obstructive lung disease (e.g., pneumonophthisis, bronchiectasia, congestive heart failure, tuberculosis and lung cancer), those with acute respiratory symptoms, unstable hearing disease or other serious diseases, those with disability of walking due to other diseases, those without available data (from pre- and post-bronchodilator spirometric testing and a questionnaire) and those refusals.
The total participants were randomly split into two subsets: Training Set and Validation Set. The Training Set, consisting of 118 COPD patients at stages I-II, 125 COPD patients at stages III-IV and 112 non-COPD subjects, was used to establish the discriminant function model based on Bayes’ Rule. The Validation Set, including 150 subjects who had been randomly selected from the strata of non-COPD, COPD at stage I-II and COPD at stage III-IV, was used to evaluate the sensitivity, specificity and likelihood ratio of the established discriminant function model in COPD screening. The study protocol was approved by the Medical Ethic Committee at Guangzhou Institute of Respiratory Diseases and a written informed consent was given by all participants.
Questionnaire
The questionnaire used in this study was a revised form of the international BOLD study (8) and incorporated parts of the questionnaire was the same as used in our previous study in China (9). The questionnaire covered demographic data, respiratory symptoms/disease, comorbidities, health care use, activity limitation, nutritional status, potential risk factors for COPD, the Medical Research Council Dyspnoea Scale and health status (10). Occupational exposure was defined as exposure to any of noxious agents (dusts, chemicals, and gases) in any of the places where the subject had ever worked for at least 1 year. Smoking index was calculated by the pack number of smoking cigarettes each day multiplied years of smoking. The patients who had suffered from bovillae, pertussis or other respiratory infection in childhood were regarded as having a childhood infection history. Family history refers to a history of COPD in the family members like mother, father, brother or sister of the patient.
Spirometry
Spirometry was performed according to the American Thoracic Society (ATS) criteria (11) and ERS recommendations (12). Each spirometer was calibrated daily with a volume variation of less than 3% by a 3-L syringe. Spirometry operators had been well trained and accredited before the survey. The testing was repeated until three reproducible, acceptable results were obtained and the best FEV1, FVC, and FEV1/FVC ratios were recorded. Subjects with airflow limitations, which was defined as an FEV1/FVC ratio <70%, underwent post-bronchodilator testing at 15 to 20 minutes after inhaling a dose of 400 mcg of salbutamol (Ventolin; GlaxoSmithKline, Middlesex, UK) through a 500-mL spacer. An increase in FEV1 >12% and >200 mL from baseline was considered positive.
Quality control
Our quality control standard was reported previously (4). All interviewers had been well trained and accredited before the study began. A pre-investigation was conducted. Each completed questionnaire and spirometry report was verified. All questionnaire data were coded and entered into a standardised Excel database (Microsoft, Redmond, WA) by two independent investigators, with computer programs checking for out-of-range values and logic mistakes.
Statistical analysis
First, a discriminant function model based on Bayes’ Rule was established by stepwise discriminant analysis using the data from the Training Set. Our initial discriminant factors included the following variables reportedly associated with COPD: gender, index of smoking, body mass index, family history of COPD, educational history, child infection history, dyspnoea scale, occupational exposure, living environment, cooking fuels, wheezing, cough and cough with production (9,13-38). By stepwise discriminant analysis, the statistically significant variables were entered into the final discriminant function model and a retrospective discrimination was conducted among those individuals in the Training Set. Using the established model, the individuals in the Validation Set was then discriminated and the sensitivity, specificity and likelihood ratios of the model were assessed. We additionally evaluated the effect of the established model on discrimination of the COPD severity. All analyses were performed using the SAS version 9.1 software (SAS Institute, Cary, NC).
Results
The COPD group included 215 males and 28 females, with ages ranging from 40 to 86 yrs. A total of 112 non-COPD individuals were recruited as a control group, including 51 females and 61 males, 40 to 79 yrs of age. A total of 84% of the study population were current smokers. Approximately 59% of the study population come from rural. Further characteristics of the enrolled subjects are given in Table 1.
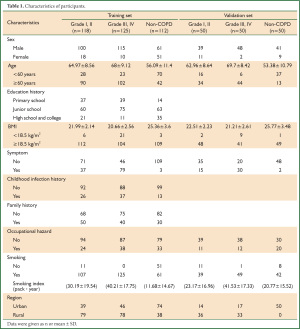
Full table
Of the variables considered, nine were determined as significant discriminatory factors: age, gender, index of smoking, body mass index, occupational exposure, living environment, wheezing, cough and dyspnoea scale (Table 2).
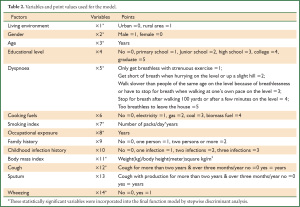
Full table
Therefore, given an individual’s values of x1, x2, x3, x5, x7, x8, x11, x12 and x14 from the questionnaire, we could calculate the values of Y0, Y1 and Y2 and then calculate their health status according to the highest values among Y0, Y1 and Y2 (based on Bayes’ rule). We retrospectively discriminated individuals in the Training Set using this model. As a result, the model had a sensitivity of 93.83%, a specificity of 89.29%, a positive likelihood ratio of 9.23, and a negative likelihood ratio of 0.07, an accuracy of 92.4% and an error rate of 7.6% in retrospective discrimination. Next, 150 individuals were discriminated by the functions of sensitivity, specificity, positive likelihood ratio, negative likelihood ratio, accuracy and error rates of 89.00%, 82.00%, 4.94, 0.13, 86.66% and 13.34%, respectively (Table 3). The discriminant function model resulted in an accuracy of 74.09% and a Kappa value of 0.70 (95% CI, 0.65 to 0.76) for retrospective prediction of COPD stage, as well as an accuracy of 70% and Kappa value of 0.61 (95% CI, 0.50 to 0.71) (Table 4).
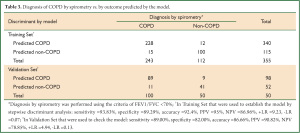
Full table
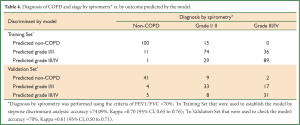
Full table
Discussion
Our study tentatively developed a discriminant function model consisting of nine variables which can be applied to screen COPD as an alternative option in areas where spirometry is unavailable. To our knowledge, no previous study used a discriminant function model to screen for COPD, though the same way has been used in the diagnosis of other diseases (39).
The present studies demonstrated clearly that spirometry underused in primary care settings not only in China but also in other developing countries. Previous studies developed questionnaires as a diagnostic scoring system of COPD and used them to identify persons who are likely to have COPD among specific risk groups (40-42).
We devised a discriminant function model to diagnose COPD according to the patient’s answers to some simple questions in the questionnaire. The model was demonstrated in the present study to have such high sensitivity (>89.00%) and specificity (>82.00%) that it can be used in primitive care settings in China, especially in the rural areas, where spirometry is unavailable. A doctor could easily diagnose COPD using our patient questionnaire and software-based calculations by the model. Compared with spirometry, the short screening questionnaire of nine variables is much simpler, easier and economical. It seems to be a more sensitive method to screen COPD than the scoring system of the COPD diagnostic questionnaires which were reported to have sensitivities of 54% to 82% and specificities of 58% to 88% (42). In addition, our discriminant function model can also be used to predict the stage of COPD, with an accuracy of about 70%.
It is well known that the discriminatory effects of a mathematic model depend on the variables selected. We selected initially 14 variables probably associated with COPD (see Table 2) according to the published literature (9-36) to detect the risk factors of COPD by stepwise screening. At last, nine variables were identified as discriminatory factors and were devised to a discriminant function model to screen COPD by some simple questions. In our discriminant function model, both smoking and BMI are the most significant discriminatory factors, which is consistent with literature. It is well-known that smoking is considered the most important risk factor in the development of COPD. In China, about two-thirds (61.4%) of the patients with COPD, 81.8% among the male patients and 24.0% among the female patients, were smokers; 13.2% of the smokers had COPD and the risk for COPD increased with the number of cigarettes consumed. In Korea, 88% of the male patients with COPD were smokers, and 36% of the adult smokers (45 years of age or older) who had smoked at least 20 cigarettes/day were diagnosed with COPD. Since BMI is another most important risk factor for COPD, those with a BMI of less than 18.5 kg/m2 may have a COPD prevalence of as high as 21.0% and there is a negative correlation between BMI and COPD prevalence. However, some risk factors, such as use of cooking fuels, sputum production, childhood infection, educational level, and familial history, were removed from the model of ours, mainly because the regions involved in the present investigation are highly correlated with the usage of cooking fuels and educational level is strongly correlated with both region and age.
Some limitations should be noted in this study. First, the sample size of 355 participants in this study may be insufficient for characterization of an entire population. Secondly, not enough women were recruited for the present study, possibly because the morbidity of COPD in women is lower than in men. Thirdly, we identified patients with COPD according to the GOLD criteria which might have led to overdiagnosis of COPD in older people. In addition, as the discriminant function model was only used as a screening tool rather than as a diagnostic criterion, the COPD patients identified by the model should have been confirmed by the spirometry. At last, although the discriminant function model was developed from the data of the subjects in Guangdong, the nine variables associated with COPD were generalised from the data of the subjects beyond Guangdong. The discrepancy may have had an unknown influence on the efficacy of the model.
In conclusion, the discriminant function model reported here is a first attempt of its kind to develop an alternative method for the COPD screening in Chinese settings. We believe that it may help diagnosis early enough a great number of COPD patients who may not be diagnosed otherwise and the early diagnosis can allow them to have a timely medical treatment.
Acknowledgements
Funding: This study was supported by the National Key Technology R&D Program of the 12th National Five-year Development Plan (2012BAI05B01). The funding providers, however, had no influence on the study design, collection, analysis, interpretation of the data, writing of the report and the decision to submit the report for publication.
Contributors: JC collected the data, monitored data collection, planned the statistical analysis, analyzed the data, and drafted and revised the manuscript. YZ and JT collected the data and revised the manuscript. XW planned the statistical analysis and revised the manuscript. JZ monitored data collection and revised the manuscript. PR and NZ initiated and designed the project, monitored data collection, drafted and revised the manuscript. PR and JC are guarantors.
Competing interests: all authors have completed the Unified Competing Interest Form at www.icmje.org/coi_disclosure.pdf (available on request from the corresponding author), and declared herein no support from any institution for the submitted work, no financial relationship with any institution that might have an interest in the submitted work in the previous 3 years and no other relationship or activity that could appear to have influenced the submitted work.
Ethical approval: the study protocol was approved by Medical Ethic Committee at Guangzhou Institute of Respiratory Diseases and a written informed consent was given by all participants.
References
- Pauwels RA, Buist AS, Calverley PM, et al. Global strategy for the diagnosis, management, and prevention of chronic obstructive pulmonary disease. NHLBI/WHO Global Initiative for Chronic Obstructive Lung Disease (GOLD) Workshop summary. Am J Respir Crit Care Med 2001;163:1256-76.
- Murray CJ, Lopez AD. Alternative projections of mortality and disability by cause 1990-2020: Global Burden of Disease Study. Lancet 1997;349:1498-504.
- Murray CJ, Lopez AD. Evidence-based health policy--lessons from the Global Burden of Disease Study. Science 1996;274:740-3.
- Pauwels RA, Rabe KF. Burden and clinical features of chronic obstructive pulmonary disease (COPD). Lancet 2004;364:613-20.
- Zhong N, Wang C, Yao W, et al. Prevalence of chronic obstructive pulmonary disease in China: a large, population-based survey. Am J Respir Crit Care Med 2007;176:753-60.
- Mannino DM, Gagnon RC, Petty TL, et al. Obstructive lung disease and low lung function in adults in the United States: data from the National Health and Nutrition Examination Survey, 1988-1994. Arch Intern Med 2000;160:1683-9.
- Liu S, Zhou Y, Wang X, et al. Biomass fuels are the probable risk factor for chronic obstructive pulmonary disease in rural South China. Thorax 2007;62:889-97.
- Buist AS, Vollmer WM, Sullivan SD, et al. The Burden of Obstructive Lung Disease Initiative (BOLD): rationale and design. COPD 2005;2:277-83.
- Cheng X, Li J, Zhang Z. Analysis of basic data of the study on prevention and treatment of COPD and chronic cor pulmonale. Zhonghua Jie He He Hu Xi Za Zhi 1998;21:749-52.
- Mannino DM, Buist AS. Global burden of COPD: risk factors, prevalence, and future trends. Lancet 2007;370:765-73.
- Standardization of Spirometry, 1994 Update. American Thoracic Society. Am J Respir Crit Care Med 1995;152:1107-36.
- Miller MR, Hankinson J, Brusasco V, et al. Standardisation of spirometry. Eur Respir J 2005;26:319-38.
- Kim DS, Kim YS, Jung KS, et al. Prevalence of chronic obstructive pulmonary disease in Korea: a population-based spirometry survey. Am J Respir Crit Care Med 2005;172:842-7.
- Tzanakis N, Anagnostopoulou U, Filaditaki V, et al. Prevalence of COPD in Greece. Chest 2004;125:892-900.
- Eisner MD, Balmes J, Yelin EH, et al. Directly measured secondhand smoke exposure and COPD health outcomes. BMC Pulm Med 2006;6:12.
- Eisner MD, Balmes J, Katz PP, et al. Lifetime environmental tobacco smoke exposure and the risk of chronic obstructive pulmonary disease. Environ Health 2005;4:7.
- Weinmann S, Vollmer WM, Breen V, et al. COPD and occupational exposures: a case-control study. J Occup Environ Med 2008;50:561-9.
- Bakke PS, Baste V, Hanoa R, et al. Prevalence of obstructive lung disease in a general population: relation to occupational title and exposure to some airborne agents. Thorax 1991;46:863-70.
- Humerfelt S, Eide GE, Gulsvik A. Association of years of occupational quartz exposure with spirometric airflow limitation in Norwegian men aged 30-46 years. Thorax 1998;53:649-55.
- Meijer E, Kromhout H, Heederik D. Respiratory effects of exposure to low levels of concrete dust containing crystalline silica. Am J Ind Med 2001;40:133-40.
- Hnizdo E, Murray J, Davison A. Correlation between autopsy findings for chronic obstructive airways disease and in-life disability in South African gold miners. Int Arch Occup Environ Health 2000;73:235-44.
- Peabody JW, Riddell TJ, Smith KR, et al. Indoor air pollution in rural China: cooking fuels, stoves, and health status. Arch Environ Occup Health 2005;60:86-95.
- Viegi G, Simoni M, Scognamiglio A, et al. Indoor air pollution and airway disease. Int J Tuberc Lung Dis 2004;8:1401-15.
- Huttner H, Beyer M, Bargon J. Charcoal smoke causes bronchial anthracosis and COPD. Med Klin (Munich) 2007;102:59-63.
- Ekici A, Ekici M, Kurtipek E, et al. Obstructive airway diseases in women exposed to biomass smoke. Environ Res 2005;99:93-8.
- Warren CP. The nature and causes of chronic obstructive pulmonary disease: a historical perspective. The Christie Lecture 2007, Chicago, USA. Can Respir J 2009;16:13-20.
- Arbex MA, de Souza Conceição GM, Cendon SP, et al. Urban air pollution and chronic obstructive pulmonary disease-related emergency department visits. J Epidemiol Community Health 2009;63:777-83.
- Sauerzapf V, Jones AP, Cross J. Environmental factors and hospitalisation for chronic obstructive pulmonary disease in a rural county of England. J Epidemiol Community Health 2009;63:324-8.
- Zanobetti A, Bind MA, Schwartz J. Particulate air pollution and survival in a COPD cohort. Environ Health 2008;7:48.
- Zhou Y, Wang C, Yao W, et al. COPD in Chinese nonsmokers. Eur Respir J 2009;33:509-18.
- Fuhrman C, Delmas MC, pour le groupe épidémiologie et recherche clinique de la SPLF. Epidemiology of chronic obstructive pulmonary disease in France. Rev Mal Respir 2010;27:160-8.
- Kazerouni N, Alverson CJ, Redd SC, et al. Sex differences in COPD and lung cancer mortality trends--United States, 1968-1999. J Womens Health (Larchmt) 2004;13:17-23.
- Stojkovic J, Stevcevska G. Quality of life, forced expiratory volume in one second and body mass index in patients with COPD, during therapy for controlling the disease. Prilozi 2009;30:129-42.
- Montes de Oca M, Tálamo C, Perez-Padilla R, et al. Chronic obstructive pulmonary disease and body mass index in five Latin America cities: the PLATINO study. Respir Med 2008;102:642-50.
- Pérez-Padilla R, Regalado J, Vedal S, et al. Exposure to biomass smoke and chronic airway disease in Mexican women. A case-control study. Am J Respir Crit Care Med 1996;154:701-6.
- Oxman AD, Muir DC, Shannon HS, et al. Occupational dust exposure and chronic obstructive pulmonary disease. A systematic overview of the evidence. Am Rev Respir Dis 1993;148:38-48.
- Künzli N, Kaiser R, Rapp R, et al. Air pollution in Switzerland--quantification of health effects using epidemiologic data. Schweiz Med Wochenschr 1997;127:1361-70.
- Stang P, Lydick E, Silberman C, et al. The prevalence of COPD: using smoking rates to estimate disease frequency in the general population. Chest 2000;117:354S-9S.
- Le Goff JM, Lavayssière L, Rouëssé J, et al. Nonlinear discriminant analysis and prognostic factor classification in node-negative primary breast cancer using probabilistic neural networks. Anticancer Res 2000;20:2213-8.
- Price DB, Tinkelman DG, Halbert RJ, et al. Symptom-based questionnaire for identifying COPD in smokers. Respiration 2006;73:285-95.
- Tinkelman DG, Price DB, Nordyke RJ, et al. Symptom-based questionnaire for differentiating COPD and asthma. Respiration 2006;73:296-305.
- Price DB, Tinkelman DG, Nordyke RJ, et al. Scoring system and clinical application of COPD diagnostic questionnaires. Chest 2006;129:1531-9.