The relationship between tumor infiltrating immune cells and the prognosis of patients with lung adenocarcinoma
Highlight box
Key findings
• The abundance of immune cells in LUAD was assessed using the CIBERSORT algorithm.
What is known and what is new?
• There is a close correlation between the tumor-infiltrating immune cells and the prognosis and clinical characteristics of LUAD patients.
• Mast cells and M1 macrophages are key immune-infiltrating cells for the prognosis of LUAD.
What is the implication, and what should change now?
• Only inflammatory factors were detected in the clinical validation; to detect the infiltration of immune cells, further in-depth research is needed.
Introduction
Lung adenocarcinoma (LUAD) is a malignant tumor with the highest mortality rate worldwide. Non-small cell lung carcinoma (NSCLC) accounts for about 85% of all lung carcinoma, and adenocarcinoma is the main pathological type of NSCLC, accounting for about 60% of NSCLC (1). Most LUAD patients are diagnosed at a late stage, and therefore, lose the opportunity for treatment. The World Health Organization reported in 2021 that there were more than 2 million new cases of lung carcinoma and 1.8 million deaths worldwide in 2020, accounting for 11.4% and 18% of all tumor morbidities and deaths, respectively, making it the second largest cancer in the world. Among these, there were 0.82 million new cases as well as 0.71 million deaths of lung carcinoma in China, which are the highest morbidity and mortality rates among all malignant tumors (2,3).
The 5-year survival rate of patients with metastatic advanced NSCLC receiving conventional treatment is less than 5% (4). Recently, important achievements have been made in tumor immunotherapy. The treatment method of blocking the inhibitory receptor programmed cell death-1 (PD-1) on the surface of T lymphocytes has significantly improved both the therapy mode and also the prognosis of carcinoma. Moreover, the 5-year survival rate of patients with advanced lung carcinoma has increased to 16% (5). The PACIFIC study suggested that immune maintenance therapy after radical concurrent chemoradiotherapy for stage III inoperable locally advanced NSCLC can significantly improve the survival of patients, and it has become the standard treatment recommended by the guidelines (6). Also, the application of immune checkpoint inhibitors can achieve sustained and effective remission in lung cancer, melanoma, bladder urothelial carcinoma, and Hodgkin’s lymphoma (7), and patients can survive for a long time. However, PD-1, programmed cell death-ligand 1 (PD-L1) antibody therapy has some limitations, its overall efficiency is only about 20% and is not satisfactory (8).
Tumor-infiltrating immune cells are key factors that hold the balance of the tumor microenvironment (TME), greatly affecting tumor progression and clinical outcomes. Immunotherapy does not benefit all tumor patients. The tumor immune microenvironment before treatment can be divided into three related immune phenotypes according to the degree of its response to immunotherapy, namely immune excluded, immune inflamed, and immune desert. There are a large number of diffuse functional cluster of differentiation CD8+ and CD4+ T cells in the TME of immune inflammation type, and this type of tumor is sensitive to immunotherapy (9,10). The immune-excluded type denotes that the infiltrating lymphocytes in the tumor tissue are distributed in the interstitium of the tumor, and the tumor parenchyma and cancer nests are less infiltrated. The immune desert type signifies that there is no lymphocyte infiltration in the parenchyma and interstitium of the tumor tissue. Both the immune desert and immune excluded types are called non-inflammatory infiltrative tumors, and most tumor patients with these two phenotypes cannot benefit from PD-1/PD-L1 monoclonal antibody (mAbs), highlighting the importance of the immune microenvironment in tumor progression and treatment (11,12). Therefore, clarifying the infiltration and distribution characteristics of immune cells in the immune microenvironment of LUAD cases will help to identify patients with poor prognoses. Furthermore, it will also improve the effective prediction of patient prognosis, ultimately guiding the promotion and implementation of clinical practice and treatment decision-making, which have important clinical application value and significance.
In this study, we aimed to create a predictive model for the prognosis of LUAD patients based on the tumor-infiltrating immune cell subsets and distribution by applying COX regression and correlation analyses. An effective prognostic prediction of LUAD patients can then be achieved, and the characteristics of immune infiltrating cells on the patient prognosis can be evaluated. We present the following article in accordance with the TRIPOD reporting checklist (available at https://jtd.amegroups.com/article/view/10.21037/jtd-22-1837/rc).
Methods
Data source
The clinical characteristics and transcriptome data of tumor tissues and paracancerous tissues of LUAD patients were obtained from TCGA database (https://cancergenome.nih.gov/). The clinical characteristics of patients included their demographic information, survival endpoints, as well as the histological type and stage of primary and new tumors. The quality control of the transcriptome data was performed using the “limma” R package RDocumentation limma (version 3.28.14).
We downloaded the gene expressions of LUAD tissues (GSE31210) from the Gene Expression Omnibus (GEO) database (http://www.ncbi.nlm.nih.gov/geo/) for verification of TCGA results.
CIBERSORT standardized data processing
We used the CIBERSORT algorithm to quantify the tumor-infiltrating immune cells. The CIBERSORT algorithm scores each immune cell subtype using the signature of a specific marker gene and calculates the ratio of immune cell subtypes based on the score. To improve the reliability of the findings, the CIBERSORT algorithm applied Monte Carlo sampling to obtain the deconvolution values of each sample. After removing data with P>0.05, we uploaded the normalized genetic data to the CIBERSORT online tool, which ran 100 aligned default signature matrixes.
Clinical samples collection
A total of 15 LUAD tissues and adjacent tissues (control group) surgically resected at the oncology department of Liaoning Cancer Hospital from January 2019 to January 2020 were selected, including five female positive cases and 10 male positive cases. The average age of the included cases was 47.89±12.23 years old. Also, none of the patients received radiotherapy, chemotherapy, immunotherapy, or other adjuvant therapies before surgery. All tissue cases were stored at −80 ℃ in a refrigerator for future use. The study was conducted in accordance with the Declaration of Helsinki (as revised in 2013). The study was approved by the Ethics Board of Liaoning Cancer Hospital (No. 20221064) and informed consent was taken from all the patients.
Enzyme-linked immunosorbent assay (ELISA)
After homogenizing the frozen LUAD and paracancerous tissues, the levels of interleukin 8 (IL-8), interferon-γ (IFN-γ), tumor necrosis factor-α (TNF-α), and IL-6 were tested by ELISA (Elabscience, Wuhan, China: E-EL-H0109c, E-EL-H0108c, E-EL-H6156, E-EL-H6008). According to the guidebook, the cells were found in a microplate reader (Thermo Scientific, USA) at a 450 nm wavelength of absorbance, and the curve was drawn to calculate the relative concentration of the above-mentioned markers.
Statistical analysis
All analyses in the current study were performed using the R 3.6.2 software (https://www.r-project.org). The independent t-test was applied to compare continuous variables with a normal distribution between the groups, and the Mann-Whitney U test was employed to compare continuous variables with a skewed distribution between the groups. A Kaplan-Meier (K-M) curve was utilized to evaluate the effect of immune cell infiltration on overall survival. The effect of individual variables on survival was analyzed using univariate regression models. The specificity and sensitivity of the recurrence prediction model were assessed by time-dependent ROC curves. The least absolute shrinkage and selection operator (LASSO) Cox regression model was used to determine the independent influencing factors related to survival. The Cox analysis method was applied to construct a linear regression graph using the regression coefficients. P<0.05 was considered statistically significant.
Results
Distribution characteristics of the tumor infiltrating immune cells in LUAD
The clinicopathological characteristics of the 522 LUAD samples from TCGA are summarized in Table 1. To explore whether immune cell abundance is related to LUAD, we used the CIBERSORT algorithm to assess the proportions of 22 tumor-infiltrating immune cells in the LUAD tissues. The findings revealed that CD8 T cells, M2 macrophages, CD4 memory resting T cells, and M0 macrophages were significantly overexpressed in both the LUAD tumor tissues and adjacent normal tissues, and may play important roles in LUAD (Figure 1A,1B). We also found a strong link between each type of tumor-infiltrating immune cells by Pearson’s correlation coefficient (Figure 1C). In addition, the Wilcoxon rank-sum test showed that Regulatory cells (Tregs), naive B cells, CD4 memory resting T cells, plasma cells, CD4 memory activated T cells, follicular helper T cells, resting natural killer cell (NK) cells, monocytes, M0 macrophages, resting mast cells, eosinophils, and neutrophils (P<0.001), together with naive CD4 T cells, M1 macrophages, gamma delta T cells (P=0.0042) had markedly different immune cell compositions between the LUAD cancerous and paracancerous tissues (Figure 1D).
Table 1
Index | Level | N |
---|---|---|
Age, years | ≤50 | 38 |
>50 | 465 | |
Unknown | 19 | |
Gender | Female | 280 |
Male | 242 | |
Stage | Stage I | 279 |
Stage II | 124 | |
Stage III | 85 | |
Stage IV | 26 | |
Unknown | 8 | |
T | T1 | 172 |
T2 | 281 | |
T3 | 47 | |
T4 | 19 | |
TX | 3 | |
M | M0 | 353 |
M1 | 25 | |
MX | 140 | |
Unknown | 4 | |
N | N0 | 335 |
N1 | 98 | |
N2 | 75 | |
N3 | 2 | |
NX | 11 | |
Unknown | 1 |
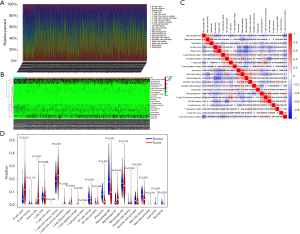
Immune risk score for the prognosis of LUAD
We incorporated all of the important cells into the LASSO Cox model and then created a Cox model to ensure that the multivariate model was not overfitted. Figure 2A shows the patient survival status, immune risk score, and distribution of seven immune cells in LUAD-positive cases, respectively. Each patient was given a risk score according to the model, and the patients were separated into low- and high-risk groups based on the median risk score. The prognostic value of all tumor-infiltrating immune cells was confirmed through K-M survival analysis and Cox proportional hazards models. The ROC curve showed good specificity and sensitivity of the risk model in predicting survival risk (area under curve, AUC =0.848, Figure 2B). The K-M curve also showed a poor prognosis in the high-risk group (Figure 2C). We then used a nomogram to illustrate the impact of seven important immune cells on the 1-, 3-, and 5-year overall survival (Figure 2D). A calibration curve was used to estimate the discriminability and accuracy of the nomogram (Figure 2E).
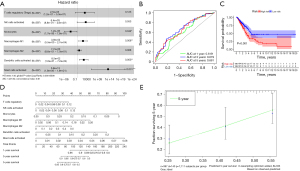
Characteristics of the immune infiltrating cells under different clinical features
We then detected the correlation between seven important immune cells and various clinicopathological indicators, including age, gender, pathological type, and tumor-node-metastasis (TNM) stage. The results revealed significant associations between T staging and naive B cells (P<0.001), activated NK cells (P=0.031), neutrophils (P=0.014), M2 macrophages (P=0.022); N staging and plasma cells (P=0.008), M0 macrophages (P=0.018); stage and M0 macrophages (P=0.008); M stage and activated dendritic cells (P=0.028); CD4 memory resting T cells (P=0.012); gender and activated NK cells (P=0.0042); and gender and CD4 memory resting T cells (P=0.018), plasma cells (P=0.043), and follicular helper T cells (P=0.0065) (Figure 3).
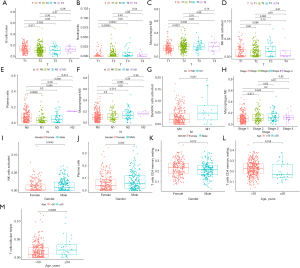
Validation of the immune risk score using the GEO dataset
The immune risk score derived from TCGA dataset was validated using the GSE31210 dataset from the GEO database, comprising 246 LUAD samples (including 20 normal samples and 226 cancer samples). The composition of tumor-infiltrating immune cells in LUAD specimen is shown in Figure 4A. Also, a strong link between each type of tumor-infiltrating immune cells was ascertained using Pearson’s correlation coefficient (Figure 4B). Four important immune cells were obtained using univariate COX regression, and an immune risk score model was constructed using the LASSO Cox model of these immune cells (Figure 4C). The patient survival status, immune risk score, and distribution of the four immune cells in patients with LUAD are shown in Figure 4D,4E, respectively. The risk lines and risk scatterplots show the distribution of risk scores for all LUAD patients. Likewise, in the validation dataset, high-risk patients also exhibited poorer prognosis (P<0.001). The ROC curves (AUC for 1-year survival: 0.628; AUC for 3-year survival: 0.659; and AUC for 5-year survival: 0.709) confirmed that the multivariate Cox regression model was reliable (Figure 4F,4G).
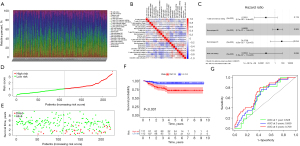
Clinical experiment verified that inflammatory factors are highly expressed in LUAD tissue
In this study, 15 tumor and paracancerous tissues were from LUAD patients, and the respective expressions of IFN-γ, TNF-α, IL-8, and IL-6 in the tissues were detected with ELISA. The results showed that the expressions of IFN-γ, TNF-α, IL-8, and IL-6 were significantly higher in the tumor tissue than those in the adjacent tissue (Figure 5, P<0.05).
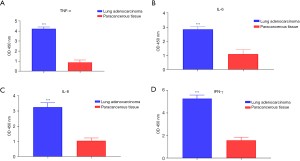
Discussion
In this study, using TCGA and GEO databases, we demonstrated that the prognosis of LUAD could be predicted with the characteristics of tumor immune cell infiltration. In addition, we found a significant variation in the distribution of immune cells in LUAD patients with various clinical characteristics. We identified seven important tumor-infiltrating immune cells through TCGA database analysis (activated mast cells, regulatory T cells, M1–M2 macrophages, monocytes, activated dendritic cells, and activated NK cells) and four important tumor-infiltrating immune cells (M1 macrophages, M0 macrophages, resting mast cells, and CD4 memory resting T cells) via GEO database analysis. The two databases jointly showed that mast cells and M1 macrophages are key immune-infiltrating cells for the prognosis of LUAD.
Macrophages are an important component of non-specific immunity in the human body and can be divided into two types according to their different functions in the tumor immune microenvironment, namely, the M1 and M2 types. Previous research has shown that tumor-associated macrophages (TAMs) are related to tumor progression, angiogenesis, survival, and the suppression of anti-tumor immunity (13). Macrophages are highly plastic and can respond variedly to different signals from their environment. For example, IFN-γ and Toll-like receptor ligands can activate macrophages to clear pathogens and, in some cases, tumor cells. Myeloid cells can also regulate adaptive immunity by modulating the composition, activation, and anti-tumor function of tumor-infiltrating lymphocytes (14). M1 macrophages are classically activated macrophages that can act as antigen-presenting cells that present tumor-associated antigens to effector T cells. Additionally, they can also secrete immune stimulatory factors to promote the proliferation of NK cells as well as T cells to exert a stronger anti-tumor effect. M2 macrophages, which can replace activated macrophages, have the effect of promoting tumor formation and development. M1 macrophages can be converted to M2 under the stimulation of IL-10, TGF-β, etc. (15,16). Previous studies have suggested that tumor-infiltrating macrophages are related to poor outcomes in lung cancer (17-19). However, other studies have revealed the positive role of tumor-infiltrating macrophages in lung cancer was independent risk factor for NSCLC progression (20-22). The function of macrophages in the prognosis of lung cancer is still controversial, and further investigation is required to clarify this issue. In this study, LUAD immune cell analysis showed that M1 macrophages were strongly related to the prognosis of LUAD patients.
Mast cells are a kind of innate immune cell. Circulating mast cells induce the growth and metastasis of various tumors. For example, mast cells can promote the metastasis and growth of LUAD cells by promoting tumor angiogenesis, autocrine local hormonal mediators, and secreting various growth factors (23-25). The products of their degranulation process can promote the metastasis of cervical cancer cells (26), and the histamine released by mast cells is related to the degree of malignant transformation in colorectal cancer (27). According to a previous investigation, intratumoral mast cells were found to be predominant in NSCLC stromal cells and correlated with survival in NSCLC (28). The study showed that mast cells in humans have two phenotypes: MCT and MCTC; MCTs are mainly located in the mucosal epithelium and lamina propria, while MCTCs are primarily located in the skin and connective tissue. Both intratumoral phenotypes promote survival in NSCLC, indicating that mast cells may be involved in anti-tumor immunity. Mast cells can also promote the proliferation of T lymphocytes by secreting TNF-α, while T lymphocytes that promote growth through TNF-α can promote the proliferation of mast cells through a positive feedback mechanism, and both exert an anti-tumor effect (29). In addition, mast cells can destroy the tumor matrix by releasing proteases from degranulation, destroying the extracellular matrix and cell structure of the tumor and thereby inhibiting tumor growth. In this study, immune cell analysis of LUAD showed that mast cells were closely associated with the prognosis of LUAD, and were a significant factor affecting the prognosis of LUAD patients.
In conclusion, we demonstrated the characteristics of immune cell infiltration in LUAD tumor tissue through TCGA database analysis and revealed the function of 22 types of infiltrating immune cells in terms of the clinical characteristics and prognosis of LUAD. These findings provide clues for the exploration of the mechanism of carcinogenesis and development, the selection of biomarkers for the prediction of immunotherapy effects, and the selection of therapeutic targets for LUAD.
This investigation also has limitations that should be noted. First, the results were obtained via a bioinformatics analysis, which still requires external verification through basic experiments on clinical samples. Second, some clinical data obtained from TCGA lacked age, classification, stage, and other characteristics. In addition, the number of LUAD samples in each group was unevenly distributed, meaning that this research is unable to comprehensively reveal the relationship between the immune infiltration characteristics and clinical characteristics. Finally, only inflammatory factors were detected in the clinical validation; to detect the infiltration of immune cells, further in-depth research is needed.
Conclusions
In summary, this investigation revealed the function of 22 types of infiltrating immune cells in terms of the clinical characteristics and prognosis of LUAD. What’s more, the risk score model based on TCGA and GEO designed in this study can be applied in clinical practice of LUAD.
Acknowledgments
Funding: None.
Footnote
Reporting Checklist: The authors have completed the TRIPOD reporting checklist. Available at https://jtd.amegroups.com/article/view/10.21037/jtd-22-1837/rc
Data Sharing Statement: Available at https://jtd.amegroups.com/article/view/10.21037/jtd-22-1837/dss
Conflicts of Interest: All authors have completed the ICMJE uniform disclosure form (available at https://jtd.amegroups.com/article/view/10.21037/jtd-22-1837/coif). The authors have no conflicts of interest to declare.
Ethical Statement: The authors are accountable for all aspects of the work in ensuring that questions related to the accuracy or integrity of any part of the work are appropriately investigated and resolved. The study was conducted in accordance with the Declaration of Helsinki (as revised in 2013). The study was approved by the ethics board of Liaoning Cancer Hospital (No. 20221064) and informed consent was taken from all the patients.
Open Access Statement: This is an Open Access article distributed in accordance with the Creative Commons Attribution-NonCommercial-NoDerivs 4.0 International License (CC BY-NC-ND 4.0), which permits the non-commercial replication and distribution of the article with the strict proviso that no changes or edits are made and the original work is properly cited (including links to both the formal publication through the relevant DOI and the license). See: https://creativecommons.org/licenses/by-nc-nd/4.0/.
References
- Zhong Z, Wang J, Han Q, et al. XBP1 impacts lung adenocarcinoma progression by promoting plasma cell adaptation to the tumor microenvironment. Front Genet 2022;13:969536. [Crossref] [PubMed]
- Ma T, Gu J, Wen H, et al. BIRC5 Modulates PD-L1 Expression and Immune Infiltration in Lung Adenocarcinoma. J Cancer 2022;13:3140-50. [Crossref] [PubMed]
- Luo D, Feng W, Ma Y, et al. Identification and validation of a novel prognostic model of inflammation-related gene signature of lung adenocarcinoma. Sci Rep 2022;12:14729. [Crossref] [PubMed]
- Shen Y, Hou N, Han F, et al. Comprehensive Analysis of Tumor Immune Microenvironment and Prognosis of m6A-Related IncRNAs in Lung Adenocarcinoma. Crit Rev Eukaryot Gene Expr 2022;32:77-91. [Crossref] [PubMed]
- Wang Y, Shen L, Li G, et al. Upregulation of XIAP promotes lung adenocarcinoma brain metastasis by modulating ceRNA network. Front Oncol 2022;12:946253. [Crossref] [PubMed]
- Liu X, Jia Y, Wang Z, et al. A pan-cancer analysis reveals the genetic alterations and immunotherapy of Piezo2 in human cancer. Front Genet 2022;13:918977. [Crossref] [PubMed]
- Qu R, Ye F, Hu S, et al. Distinct cellular immune profiles in lung adenocarcinoma manifesting as pure ground glass opacity versus solid nodules. J Cancer Res Clin Oncol 2022; Epub ahead of print. [Crossref]
- Dong Y, Li Y, Yao Y, et al. A novel defined m7G regulator signature to investigate the association between molecular characterization and clinical significance in lung adenocarcinoma. Front Oncol 2022;12:897323. [Crossref] [PubMed]
- Akhave N, Zhang J, Bayley E, et al. Immunogenomic profiling of lung adenocarcinoma reveals poorly differentiated tumors are associated with an immunogenic tumor microenvironment. Lung Cancer 2022;172:19-28. [Crossref] [PubMed]
- Guo L, Yi J, Liu M, et al. The prognostic landscape of tumor-infiltrating immune cells in lung squamous cell carcinoma. Ann Transl Med 2021;9:799. [Crossref] [PubMed]
- Wu L, Zhong Y, Yu X, et al. Selective poly adenylation predicts the efficacy of immunotherapy in patients with lung adenocarcinoma by multiple omics research. Anticancer Drugs 2022;33:943-59. [Crossref] [PubMed]
- Yin XQ, Yin XH, Yu YQ, et al. Genomic Landscape of RTK/RAS Pathway and Tumor Immune Infiltration as Prognostic Indicator of Lung Adenocarcinoma. Front Oncol 2022;12:924239. [Crossref] [PubMed]
- Wang B, Law A, Regan T, et al. Systematic comparison of ranking aggregation methods for gene lists in experimental results. Bioinformatics 2022;38:4927-33. [Crossref] [PubMed]
- Wu J, Liu X, Wu J, et al. CXCL12 derived from CD248-expressing cancer-associated fibroblasts mediates M2-polarized macrophages to promote nonsmall cell lung cancer progression. Biochim Biophys Acta Mol Basis Dis 2022;1868:166521. [Crossref] [PubMed]
- Fu JL, Hao HF, Wang S, et al. Marsdenia tenacissima extract disturbs the interaction between tumor-associated macrophages and non-small cell lung cancer cells by targeting HDGF. J Ethnopharmacol 2022;298:115607. [Crossref] [PubMed]
- Gao Y, Stein MM, Kase M, et al. Comparison of the tumor immune microenvironment and checkpoint blockade biomarkers between stage III and IV non-small cell lung cancer. Cancer Immunol Immunother 2023;72:339-50. [Crossref] [PubMed]
- Park JV, Chandra R, Cai L, et al. Tumor Cells Modulate Macrophage Phenotype in a Novel In Vitro Co-Culture Model of the NSCLC Tumor Microenvironment. J Thorac Oncol 2022;17:1178-91. [Crossref] [PubMed]
- Saito Y, Fujiwara Y, Shinchi Y, et al. Classification of PD-L1 expression in various cancers and macrophages based on immunohistocytological analysis. Cancer Sci 2022;113:3255-66. [Crossref] [PubMed]
- Chen Y, Hong C, Qu J, et al. Knockdown of lncRNA PCAT6 suppresses the growth of non-small cell lung cancer cells by inhibiting macrophages M2 polarization via miR-326/KLF1 axis. Bioengineered 2022;13:12834-46. [Crossref] [PubMed]
- Gomez-Roca C, Cassier P, Zamarin D, et al. Anti-CSF-1R emactuzumab in combination with anti-PD-L1 atezolizumab in advanced solid tumor patients naïve or experienced for immune checkpoint blockade. J Immunother Cancer 2022;10:e004076. [Crossref] [PubMed]
- Wu XR, Peng HX, He M, et al. Macrophages-based immune-related risk score model for relapse prediction in stage I-III non-small cell lung cancer assessed by multiplex immunofluorescence. Transl Lung Cancer Res 2022;11:523-42. [Crossref] [PubMed]
- Liu J, Meng Z, Xu T, et al. A SIRPαFc Fusion Protein Conjugated With the Collagen-Binding Domain for Targeted Immunotherapy of Non-Small Cell Lung Cancer. Front Immunol 2022;13:845217. [Crossref] [PubMed]
- Leveque E, Rouch A, Syrykh C, et al. Phenotypic and Histological Distribution Analysis Identify Mast Cell Heterogeneity in Non-Small Cell Lung Cancer. Cancers (Basel) 2022;14:1394. [Crossref] [PubMed]
- Shefler I, Salamon P, Zitman-Gal T, et al. Tumor-Derived Extracellular Vesicles Induce CCL18 Production by Mast Cells: A Possible Link to Angiogenesis. Cells 2022;11:353. [Crossref] [PubMed]
- Tian YL, Fu SB, Li B, et al. Mechanism of Fuzheng Kang'ai Formula Regulating Tumor Microenvironment in Non-Small Cell Lung Cancer. Chin J Integr Med 2022;28:425-33. [Crossref] [PubMed]
- Ma G, Wang C, Lv B, et al. Proteinase-activated receptor-2 enhances Bcl2-like protein-12 expression in lung cancer cells to suppress p53 expression. Arch Med Sci 2019;15:1147-53. [Crossref] [PubMed]
- Shikotra A, Ohri CM, Green RH, et al. Mast cell phenotype, TNFα expression and degranulation status in non-small cell lung cancer. Sci Rep 2016;6:38352. [Crossref] [PubMed]
- Stoyanov E, Uddin M, Mankuta D, et al. Mast cells and histamine enhance the proliferation of non-small cell lung cancer cells. Lung Cancer 2012;75:38-44. [Crossref] [PubMed]
- Ohri CM, Shikotra A, Green RH, et al. Chemokine receptor expression in tumour islets and stroma in non-small cell lung cancer. BMC Cancer 2010;10:172. [Crossref] [PubMed]
(English Language Editor: A. Kassem)