Value of contrast-enhanced magnetic resonance imaging-T2WI-based radiomic features in distinguishing lung adenocarcinoma from lung squamous cell carcinoma with solid components >8 mm
Highlight box
Key findings
• The contrast-enhanced MRI-T2WI-based radiomic model has a good diagnostic performance in distinguishing lung adenocarcinoma from lung squamous cell carcinoma with solid components >8 mm.
What is known and what is new?
• As an emerging non-invasive examination technology, radiomics has been widely used in oncology. At present, MRI-based radiomics in identifying the pathological subtypes of lung cancer hasn’t ripened yet;
• It is clinically valuable to clarify whether MRI-based radiomics can distinguish the pathological subtypes of lung cancer, the difference in diagnostic performance between MRI-based radiomics and CT radiomics, and whether MRI can replace CT in some circumstances.
What is the implication, and what should change now?
• MRI-based radiomics may replace CT-based radiomics in the differential diagnosis of some pathological subtypes of lung cancer located in special sites. However, prospective clinical studies are warranted to further verify the potential clinical value of the MR-Rad model.
Introduction
In 2020, there were about 19.3 million new cancer cases and nearly 10 million cancer-related deaths worldwide. Among them there were 2.20 million new lung cancer cases (11.4%), making lung cancer the second most common malignancy; in addition, almost 1.78 million people (18.0%) died from lung cancer, and thus lung cancer remains the most common cause of cancer death globally (1). Non-small cell lung cancer (NSCLC) accounts for about 85% of all lung cancer cases. It has a variety of pathological subtypes, the most common of which are adenocarcinoma (Ade) and squamous cell carcinoma (Squ) (2). Different pathological subtypes of lung cancer have different phenotypes and biological features. Although Ade and Squ have similar clinical manifestations and imaging findings, they are dramatically different in pathogenesis, pathological features, treatment regimens, and prognosis. For example, targeted therapy brings high benefits to Ade patients but is typically ineffective for Squ. It can be explained by the fact epidermal growth factor receptor (EGFR) mutations and anaplastic lymphoma kinase (ALK) fusions are 2 major driver genes for targeted therapy of NSCLC, and their expressions are more commonly seen in Ade than in Squ (3). In addition, Squ is more sensitive to chemotherapy. Therefore, the differentiation between Ade and Squ is of great significance for regimen selection and prognostic prediction in lung cancer patients.
At present, lung cancer is often qualitatively diagnosed by clinicians based on conventional imaging examinations, which is highly dependent on clinicians’ personal experience and leaves a possibility of missed diagnosis or misdiagnosis. Pathologic diagnosis is the gold standard for lung cancer diagnosis, which is often based on needle biopsy of lesions or postoperative pathology; however, these techniques are generally invasive and non-reproducible and can cause complications (4).
Non-invasive early diagnostic techniques for lung nodules mainly include liquid biopsy and various imaging examinations. Liquid biopsy techniques for lung cancer include the detection of serum autoantibodies, circulating tumor DNA (ctDNA), RNA exosomes, and traditional tumor markers (5,6). Although there are a variety of liquid biopsy techniques for lung cancer, there is no mature detection system; these detection techniques are also expensive, which limits their clinical application (5,7). In 2012, Dutch researchers Kumar et al. first proposed the concept of radiomics, which has more advantages than traditional image reading techniques (8). Radiomics uses automatic data characterization algorithm to transform medical image analysis from traditional qualitative evaluation to quantitative analysis. It performs mathematical processing on the basis of tests statistic, and the results obtained may personalize the diagnosis, treatment, and prognosis of specific diseases (9-12). As a non-invasive and reproducible technique, radiomics has shed new light on the diagnosis and treatment of tumors. In recent years, many studies have explored the role of radiomics in lung cancer management, and radiomics has been widely used in subtype differentiation, prognostic prediction, phenotyping, and classification of benign and malignant lung nodules (13-17).
Most of the currently available studies on lung cancer radiomics are based on computed tomography (CT) images. Ji et al. showed that by constructing a radiomic model predicting the classification of lung Ade and Squ based on stage-specific positron emission tomography (PET) radiomic, the AUC of it in the validation cohort was 0.886. The radiomic-clinical nomogram integrating radiomic features with independent clinical predictors exhibited more favorable discriminative performance, with AUC of 0.978 in the validation cohort (18). The radiomic model, based on CT constructed by Zhu et al. had an AUC of 0.893 (95% CI: 0.789 to 0.996) in the validation cohort, with a sensitivity of 0.828 and a specificity of 0.900 (19). However, CT and PET/CT are radiotoxic, and CT offers less precise visualization of soft tissue detail than magnetic resonance imaging (MRI). In addition, the tumor tissue function information provided by MRI has certain predictive value for the pathological classification of lung cancer. Clinically, pure ground-glass opacity neoplastic lung nodules are more likely to be Ade, whereas nodules with solid components are difficult to identify. Research has shown that MRI has a sensitivity of 60–75% for solid lung nodules measuring 4–6 mm, 80–99% for solid lung nodules measuring 6–8 mm, and 100% for solid nodules with a diameter of >8 mm (20-24). According to the Lung Imaging Reporting and Data System (Lung-RADS) (23), solid nodules measuring ≥6 mm belong to grade 3 and above nodules that require short-term follow-up or further clinical management. MRI has shown good sensitivity and specificity for solid nodules sized ≥6 mm (i.e., Lung-RADS grade 3 and above nodules) (20). The study conducted by Lacroix et al. identifies the tumor histological type from magnetic resonance (MR) T2-weighted images, with classification performance similar to those reported in PET/CT and in multiphase CT in lung cancers (25). More studies have been carried out on MRI-based radiomics. Tang et al. (26) integrated the radiomics features with the clinical features for the histological subtype stratification in patients with NSCLC, and the accuracy reached 79.2%. Some studies have also used MRI-based radiomics models to evaluate the distant metastasis of lung cancer and the efficacy of subsequent treatments (27-29). There have been some studies of T2WI-based models predicting tumor subtypes with some proven diagnostic value (30,31). However, MRI-based omics in identifying the pathological subtypes of lung cancer has not yet fully matured.
Therefore, based on the contrast-enhanced MRI-T2-weighted imaging (T2WI)-based radiomic features, we constructed prediction models using the quantitative parameters of radiomics for the pathological classification of Ade and Squ with a solid component of >8 mm and investigated the value of this technology in the non-invasive prediction of pathological subtypes of lung cancer. We present the following article in accordance with the TRIPOD reporting checklist (available at https://jtd.amegroups.com/article/view/10.21037/jtd-23-142/rc).
Methods
Patient selection and grouping
In this retrospective study, a total of 71 patients who visited the Department of Thoracic Surgery, The First Affiliated Hospital of Soochow University from January 2020 to September 2021 and underwent chest contrast-enhanced MRI and CT before treatment were enrolled. All cases had definite pathological diagnoses. There were 47 males aged 64.08±7.69 years and 24 females aged 62.08±7.93 years. The pathological types included Ade (n=46) and Squ (n=25).
The inclusion criteria were as follows: (I) undergoing contrast-enhanced MRI and CT before treatment, and the nodules had solid components ≥8 mm; (II) treatment-naïve before the imaging examinations; (III) with pathologically (e.g., exfoliative cytology of pleural fluid, tracheoscopy, puncture biopsy, or surgery) confirmed Ade or Squ; and (IV) with complete clinical data, along with clear MRI and CT images of chest lung cancer lesions before treatment.
The exclusion criteria were as follows: (I) no pulmonary lesion found on MRI; (II) having received any form of treatment (such as radiotherapy and chemotherapy) before completing the imaging examinations; (III) with histopathological types other than Ade and Squ, as confirmed by pathology; (IV) lack of clinical data, or the imaging quality did not meet diagnostic criteria (e.g., obvious metal or motion artifacts, unclear development, etc.); and (V) with a previous history of other malignant tumors in the chest or with other systemic malignant tumors.
All enrolled patients were divided into the Ade group or Squ group according to the pathological results. In addition, according to the needs of model establishment, patients in each group were randomly divided into a training set (n=48) and validation set (n=23) in a ratio of about 7:3. The training set included 16 Squ patients and 32 Ade patients and the validation set included 9 Squ patients and 14 Ade patients.
Materials and methods
The examination was performed using a 3.0 T magnetic resonance scanner (Skyra; Siemens AG, Erlangen, Germany), which used a dedicated 16-channel phased-array coil. Patients underwent breathing training prior to examination to reduce respiratory motion artifacts. The following acquisition parameters were applied: coronal T2WI half-Fourier acquisition single-shot turbo spin echo (HASTE): rotation time (TR), 1,400 ms; echo time (TE), 87 ms; field of view (FOV), 400 mm × 400 mm; FOV, 1.3×1.3; slice thickness, 5 mm; slices, 24. Axial T2WI BLADE [which is also named periodically rotated overlapping parallel lines with enhanced reconstruction (PROPELLER)]: TR, 3,000 ms; TE, 87 ms; FOV, 400 mm × 400 mm; FOV, 1.3×1.3; slice thickness, 5 mm; slices, 24. Axial T1WI three-dimensional volumetric interpolated breath-hold examination (VIBE-3D): TR, 4.11 ms; TE, 1.22 ms; FOV, 420 mm × 420 mm; FOV, 1.3×1.3; slice thickness, 3.5 mm. diffusion-weighted imaging (DWI): b value, 50,800 s/mm2; TR, 5,600 ms; TE, 72 ms; FOV, 400 mm × 400 mm; FOV, 1.6×1.6; slice thickness, 5 mm; and slices, 24. A dose of gadolinium-diethylenetriamine pentaacetic acid (Gd-DTPA) [Magnevist; 0.1 mmol/kg (0.2 mL/kg)] was injected as a quick bolus into the cubital vein at a rate of 3.0 mL/s by using a double-barrel high-pressure syringe. Allergy testing was performed before contrast injection to ensure patient safety. Radiomic features were extracted from chest axial T2WI.
The CT images were scanned using Toshiba (Tokyo, Japan), Philips (Amsterdam, Netherlands), and Siemens CT scanners. The default values of the scanners included tube voltage 120 kV and tube current 110–240 mA. After the real-time dynamic dose mapper was turned on, the relevant parameters included: collimation, 192 mm × 0.6 mm; TR, 0.25 s; pitch, 0.9; and slice thickness, 5 mm. The patient was asked to take a supine position, with both upper limbs naturally raised. The head was advanced first, and routine chest scan was performed at the end of the deep inspiration. The scan ranged from the thoracic inlet to the level 5 cm below the costophrenic angle. All CT images were reviewed with the lung window [window width, 1,500 Hounsfield units (HU); window level, −500 HU] and the mediastinal window (window width, 400 HU; window level, 45 HU), with the reconstructed slice thickness being 1 mm.
Radiomic data processing and model establishment
The workflow of radiomics mainly included image segmentation, feature extraction, feature selection, and machine learning modeling (32).
Image segmentation
The free and open-source 3D Slicer software (version 4.11.20210226; https://www.slicer.org/) was used to extract radiomics features. After the chest MRI images (in DICOM format) were imported into 3D Slicer, the regions of interest (ROIs) on axial T2WI were manually delineated layer by layer. The delineation went through along the edge of the primary lung cancer lesion, with the adjacent normal tissues and lymph nodes excluded, thus the volume of interest (VOI) was obtained (Figure 1). Finally, the 3D segmentation results that were obtained from the MRI images were saved as .nii image files and exported 1 by 1. On all images, the ROIs were independently segmented by a thoracic surgeon and a radiologist for primary lesions on MRI-T2WI.
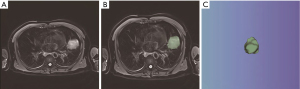
Feature extraction
The radiomic features were extracted by using the SlicerRadiomics, which is an extension for 3D Slicer. The radiomic features included: (I) shape features: shape features describe the geometric aspects of a ROI, such as volume, maximum diameter, and maximum surface area. (II) first-order statistical features: including the average, median, maximum, and minimum values of voxel intensity as well as their skewness (asymmetry), kurtosis (flatness), uniformity, and randomness (entropy). (III) Second-order statistical features: including the so-called texture features such as gray level co-occurrence matrix (GLCM), gray-level dependence matrix (GLDM), gray-level run-length matrix (GLRLM), gray-level size-zone matrix (GLSZM), and neighboring gray tone difference matrix (NGTDM). (IV) Higher-order statistical features were acquired by wavelet transform on images (33-35). The voxel size after resampling was 1 mm × 1 mm × 1 mm.
Feature screening
For the extracted radiomic features, the obtained features were then screened and dimensionally reduced by using the corresponding statistical methods, and finally the radiomic features that were most relevant to lung cancer subtypes were screened from each sequence image. The 5-fold cross-validation was performed for overfitting, so as to improve the stability of the radiomic models.
Machine learning modeling
The imaging features with non-zero coefficients were weighted with the corresponding coefficients and then added linearly to obtain the radiomic scores (Rad-score). The formula used was Rad-score = intercept +βi* Xi, where β represents the coefficient, X represents the feature, and i represents the ordinal. The clinical feature data were combined with Rad-score to construct a combined model based on the results of multivariate logistic regression.
Collection of baseline clinical data and nomogram construction
A further prediction model was established by combining Rad-score and predictive clinical risk factors, the factors included the patient’s sex, age, smoking history. Univariate analysis was performed to select the candidate clinical risk factor and ensure that features for model training were informative and predictive. Subsequently, a multivariable logistic regression algorithm was used to build the nomogram. The study fully complied with the Declaration of Helsinki (as revised in 2013). The study was approved by the Medical Ethics Committee of The First Affiliated Hospital of Soochow University (approval No. LYP2022-507). Informed consent was obtained from all the participants.
Statistical analysis
Statistical analyses were performed using R Studio (version 4.2.0; The R Foundation for Statistical Computing, Vienna, Austria) and SPSS 25.0 (IBM Corp., Armonk, NY, USA). Data were divided into measurement variables and count variables. Normally distributed measurement data were analyzed by using independent sample t-test, and non-normally distributed measurement data by Mann-Whitney U test. The univariate analysis of categorical variables was based on χ2 test, whereas multivariate analysis was based on the binary logistic regression model. The measurement data are presented as mean ± standard deviation (SD), with 0/1 representing the different categories of categorical variables. Accordingly, the relevant variables of Ade and Squ were screened out from the clinical features and imaging features. A P value of <0.05 was considered significantly different and ensured two-sided. The radiomic model was constructed based on the Rad-score, and the nomogram was established by multivariate logistic regression analysis to construct a combined model that combined MRI Rad-score and clinical features. The area under the receiver operating characteristic (ROC) curves (AUC), sensitivity, and specificity were used to evaluate the performance of each model in distinguishing Ade from Squ. Decision curve analysis (DCA) was performed to evaluate the net clinical benefits of the models. The diagnostic performance of these models was compared by using the DeLong’s test.
Results
Clinical data
There were significant differences in gender (P<0.001) and smoking history (P<0.001) between Ade group and Squ group, whereas age showed no statistically significant difference (P>0.05) (Table 1).
Table 1
Variables | Squ group (n=25) | Ade group (n=46) | χ2/t | P value |
---|---|---|---|---|
Age (years), mean ± SD | 63.08±9.50 | 63.59±6.77 | 1.94 | 0.795 |
Gender, n (%) | 11.48 | <0.001 | ||
Males | 23 (92.0) | 24 (52.2) | ||
Females | 2 (8.0) | 22 (47.8) | ||
Smoking history, n (%) | 17.12 | <0.001 | ||
No | 4 (16.0) | 31 (67.4) | ||
Current or former smokers | 21 (84.0) | 15 (32.6) |
Ade, adenocarcinoma; Squ, squamous cell carcinoma; SD, standard deviation.
Creation of radiomic models
The radiomic features were extracted from the contrast-enhanced MRI-T2WI images, and 4 radiomic features were finally screened out after dimensionality reduction (Figure 2 and Table 2). The MRI Rad-score was calculated by weighting the coefficients of the selected features with the following formula:
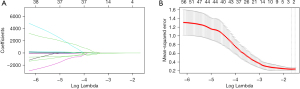
Table 2
Variable | Coefficient |
---|---|
Original_firstorder_Minimum | 0.005834573 |
Wavelet-LLH_ngtdm_Busyness | −0.0000003500894 |
Wavelet-HHL_glszm_SizeZoneNonUniformityNormalized | 0.5322761 |
Wavelet-HHH_firstorder_Minimum | 0.09467841 |
MRI, magnetic resonance imaging; Lasso, least absolute shrinkage and selection operator.
Radiomic features were extracted from the CT images, and ten radiomic features were finally screened out after dimensionality reduction (Figure 3 and Table 3). The CT Rad-score was calculated by weighting the coefficients of the selected features with the following formula:
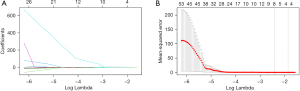
Table 3
Variables | Coefficient |
---|---|
Original_Glcm_Imc2 | 0.4597578 |
Original_Glrlm_SmallDependenceHighGrayLevelEmphasis | −0.000023893 |
Wavelet-LLH_Firstorder_Contrast | −0.000437909 |
Wavelet-LLH_Firstorder_DifferenceVariance | −0.00105647 |
Wavelet-LLH_Firstorder_DependenceNonUniformityNormalized | −0.06892258 |
Wavelet-LLH_Glcm_Autocorrelation | −0.0000272192 |
Wavelet-LHL_Gldm_JointAverage | −0.001207498 |
Wavelet-LHL_Gldm_LargeDependenceLowGrayLevelEmphasis | 0.430877 |
Wavelet-LHL_Glrlm_LargeAreaHighGrayLevelEmphasis | −0.000000000493409 |
Wavelet-LHL_Glrlm_Complexity | −0.00000203789 |
CT, computed tomography; Lasso, least absolute shrinkage and selection operator.
Creation of a combined model
In the multivariate logistic regression, only smoking history alone had a discriminating value (P<0.05). The sensitivity and specificity of the clinical model based on smoking was 75.0% and 93.8%. Multivariate logistic regression was used to combine relevant clinical features or MRI Rad-score to form the nomogram of a combined model (Figure 4). Table 4 lists the components of the combined model and the corresponding feature values. The score of the combined model was calculated as follows: comprehensive diagnostic score (Com-score) = −9.5768 − 6.3930 * Smoking (0/1) + 23.4471 * MRI Rad-score.
Table 4
Feature | Coefficient |
---|---|
Smoking | −6.3930 |
MRI Rad-score | 23.4471 |
MRI, magnetic resonance imaging; Rad-score, radiomic scores.
Diagnostic performance of each model
The distribution of Rad-scores in the training set and validation set in the MR-Rad model is shown in Figure 5A,5B, and the Rad-score significantly differed between Squ group and Ade group (P<0.05). The distribution of Rad-scores in the training set and validation set in the CT-Rad model is shown in Figure 5C,5D, and the Rad-score significantly differed between Squ group and Ade group (P<0.05). The distribution of Com-scores in the training set and validation set in the combined model is shown in Figure 5E,5F, and the Com-score significantly differed between Squ group and Ade group (P<0.05).
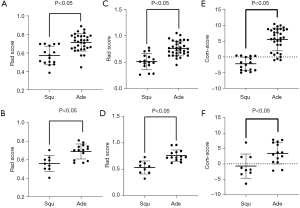
The ROC curve of the training set in the MR-Rad model is shown in Figure 6A, and its AUC was 0.8438 [95% confidence interval (CI): 0.7304–0.9571], with the diagnostic sensitivity and specificity of 90.63% and 68.75%, respectively. The ROC curve of the validation set in the MR-Rad model is shown in Figure 6B, and its AUC was 0.8651 (95% CI: 0.7170–1.000), with the diagnostic sensitivity and specificity of 71.43% and 88.89%, respectively. The ROC curve of the training set in CT-Rad model is shown in Figure 6A, and its AUC was 0.8848 (95% CI: 0.5699–0.9341), with the diagnostic sensitivity and specificity of 87.50% and 81.25%, respectively. The ROC curve of the validation set in CT-Rad model is shown in Figure 6B, and its AUC was 0.9286 (95% CI: 0.4526–0.9605), with the diagnostic sensitivity and specificity of 92.86% and 77.78%, respectively. The ROC curves of the training set and validation set in the combined model are shown in Figure 6A,6B. The AUC of the training set in the combined model was 0.9570 (95% CI: 0.9066–1.000), with the diagnostic sensitivity and specificity of 87.50% and 93.75%, respectively. The AUC of the validation set in the combined model was 0.8016 (95% CI: 0.6135–0.9897), with the diagnostic sensitivity and specificity of 64.29% and 88.89%, respectively.
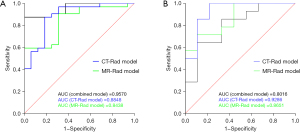
Therefore, all the constructed MR-Rad model, CT-Rad model, and combined model had good diagnostic performance (Table 5).
Table 5
Group | AUC (95% CI) | Sensitivity (%) | Specificity (%) |
---|---|---|---|
Training set | |||
MR-Rad model | 0.8438 (0.7304–0.9571) | 90.63 | 68.75 |
CT-Rad model | 0.8848 (0.5699–0.9341) | 87.50 | 81.25 |
Combined model | 0.9570 (0.9066–1.000) | 87.50 | 93.75 |
Validation set | |||
MR-Rad model | 0.8651 (0.7170–1.000) | 71.43 | 88.89 |
CT-Rad model | 0.9286 (0.4526–0.9605) | 92.86 | 77.78 |
Combined model | 0.8016 (0.6135–0.9897) | 64.29 | 88.89 |
AUC, area under the curve; CI, confidence interval; MR, magnetic resonance; Rad, radiomic; CT, computed tomography.
The calibration curves of the MR-Rad model showed good prediction consistency in training and validation sets (Figure 7). The DCA curves of the radiomic models and the combined model showed that all models had good net survival benefits and thus may be clinically valuable (Figure 8).
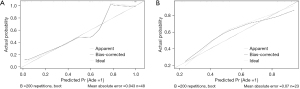
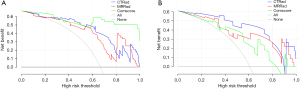
The diagnostic performance of the ROC curves was compared among these models by using the DeLong’s test. In the training set, the diagnostic performance showed no significant difference between the CT-Rad model and the MR-Rad model (P=0.63) but was significantly better in the combined model than in the MR-Rad model (P=0.0247). In the validation set, the diagnostic performance showed no statistically significant difference between the CT-Rad model and the MR-Rad model (P=0.5245); in addition, it was also not significantly different between the combined model and the MR-Rad model (P=0.4807).
Discussion
In our present study, a radiomic model was established based on chest contrast-enhanced MRI-T2WI images to distinguish Ade from Squ with solid components >8 mm. It was found that the AUC of the MR-Rad model was 0.8438 and 0.8651 in the training and validation sets, respectively. It was found that the AUC of the CT-Rad model was 0.8848 and 0.9286 in the training and validation sets, respectively. In contrast, the AUC of the radiomic model based on chest CT images was 0.8848 and 0.9286 in the training and validation sets, respectively. With good degree of fit, consistency, and stability, these models could effectively predict Ade and Squ. The DCA of the radiomic models and the combined model showed that all models had good net survival benefits and thus may be clinically valuable. The diagnostic performance showed no significant difference between the CT-Rad model and the MR-Rad model. The diagnostic performance of the combined model was better than that of the MR-Rad model in the test set; however, no such difference was seen in the validation group. The possible explanation is that the sample size of the validation group was small, and the retrospective study had selection bias. Therefore, we could not draw a conclusion that the combined model was better than the MR-Rad model.
The role of radiomics in the diagnosis of lung cancer has increasingly been recognized in recent years (14,36). In our present study, a total of 4 MRI-based radiomic features were extracted, including 2 first-order features, 1 NGTDM feature, and 1 GLSZM feature, among which the feature SizeZoneNonUniformityNormalized accounted for the largest weighted ratio; the features Minimal and wavelet-HHH_ firstorder_Minimum provided information features about the minimum gray value in the ROI; the feature Busyness was used to calculate the busyness of the image NGTDM, reflecting the difference in average gray value between a pixel and its neighborhood; the SizeZoneNonUniformityNormalized was used to describe the conditional probability density for 2 variables of image brightness, and more uniform image texture corresponds to wider and flatter matrix.
The principle of radiomics is that radiomic features can reflect the heterogeneity of tumor cells at the cellular level, and the latter determines the malignancy of a specific tumor. Thus, radiomics can be used to establish descriptive or predictive models to assist the clinical diagnosis of tumors (32,37). Ren et al. and Tang et al. found that the radiomic model constructed based on CT alone had a sensitivity of 69.29%, a specificity of 85.25%, and an AUC of 0.794 in predicting lung cancer subtypes. The combined model based on clinical features, CT/PET radiomics, and tumor markers had a sensitivity of 96.28%, a specificity of 95%, and an AUC of 0.932 (38,39). In our current study, the MR-Rad model was basically the same as the CT-Rad model in terms of sensitivity, specificity, and clinical utility in differentiating lung cancer subtypes. CT is currently the most important examination for lung cancer screening and follow-up. In recent years, chest MRI has increasingly been applied in clinical practice thanks to the upgrading of MRI equipment and the advances in scanning technologies such as respiratory triggering, electrocardiogram (ECG) gating, and parallel acquisition. As another non-invasive imaging mode, MRI is superior to CT in the following aspects. First, unlike CT scan, MRI does not use ionizing radiation and is considered a safer procedure. It has been reported that there was a 5.5% increase in lung cancer risk attributable to annual CT-related radiation exposure in 5–70-year-old current or former smokers (40). Such a risk may be even higher in patients requiring repeated CT scans to determine whether their tumors are benign or malignant. Second, MRI has a high soft tissue resolution and therefore can clearly display the relationships between a tumor and its surrounding soft tissues such as blood vessels and lymph nodes. MRI can distinguish different types of tissues based on the water content and therefore has a better performance than CT in soft tissue imaging. For central lung cancer, for example, MRI can satisfactorily display the compression of bronchi and blood vessels by the tumor, especially the vascular invasion. When lung cancer is located near the mediastinum, MRI can show whether the cancer is adjacent to or infiltrating the mediastinum. When lung cancer is complicated by atelectasis, CT cannot distinguish between the mass and the atelectasis, whereas MRI can show that there is a border between them and can reveal the size and extent of the mass. For superior sulcus tumors, MRI can display tumor involvement in the subclavian artery and brachial plexus; due to partial volumetric effects and bone artifacts, CT is suboptimal at this site. MRI also has certain advantages in the identification of enlarged mediastinal lymph nodes. Third, MRI provides richer functional information on tumor tissue; compared with histopathology and genetic testing, MRI can not only overcome sampling bias but also eliminate the pain and potential complications caused by biopsy or surgery. However, MRI can be inferior to CT in the following aspects: first, MRI has poorer spatial resolution than CT and is more susceptible to the effects of breath and heartbeat. Second, MRI is slow and requires longer examination time. Third, MRI is more expensive than CT, which limits its application in multiple follow-up observations and comparisons. Fourth, MRI is not feasible for patients with metallic clips after a vascular surgery, pacemaker users, or those who are critically ill and need to be monitored by ventilators or ECG. Whether MRI can completely replace CT for lung cancer screening needs to be further investigated. However, it is generally believed that MRI may replace CT in differentiating lung cancer at special sites such as central lung cancer and tumors at the base/apex of the lung.
Our current study had some limitations: first, selection bias might have existed due to the retrospective design of our current study. Second, our study was a small-sample single-center study, and its results need to be further validated in multi-center and large-sample studies. Third, the ROIs were manually drawn by physicians and there was a lack of standardized image acquisition process (41,42). Fourth, the stability of the features might be affected by the differences in machine models, scanning parameters, physiological motion, and other factors (43). Fifth, except for age, gender, and smoking history, no other clinical factors were included in this study.
Conclusions
In summary, contrast-enhanced MRI-T2WI-based radiomic model has a good diagnostic performance and can be used in distinguishing Ade from Squ with solid components >8 mm. MRI may replace CT in the differential diagnosis of some pathological subtypes of lung cancer located in special sites, which may facilitate the development of more tailored treatment protocols.
Acknowledgments
Funding: None.
Footnote
Reporting Checklist: The authors have completed the TRIPOD reporting checklist. Available at https://jtd.amegroups.com/article/view/10.21037/jtd-23-142/rc
Data Sharing Statement: Available at https://jtd.amegroups.com/article/view/10.21037/jtd-23-142/dss
Peer Review File: Available at https://jtd.amegroups.com/article/view/10.21037/jtd-23-142/prf
Conflicts of Interest: All authors have completed the ICMJE uniform disclosure form (available at https://jtd.amegroups.com/article/view/10.21037/jtd-23-142/coif). The authors have no conflicts of interest to declare.
Ethical Statement: The authors are accountable for all aspects of the work in ensuring that questions related to the accuracy or integrity of any part of the work are appropriately investigated and resolved. The study fully complied with the Declaration of Helsinki (as revised in 2013). This study was approved by the Medical Ethics Committee of The First Affiliated Hospital of Soochow University (approval No. LYP2022-507). Informed consent was obtained from all the participants.
Open Access Statement: This is an Open Access article distributed in accordance with the Creative Commons Attribution-NonCommercial-NoDerivs 4.0 International License (CC BY-NC-ND 4.0), which permits the non-commercial replication and distribution of the article with the strict proviso that no changes or edits are made and the original work is properly cited (including links to both the formal publication through the relevant DOI and the license). See: https://creativecommons.org/licenses/by-nc-nd/4.0/.
References
- Sung H, Ferlay J, Siegel RL, et al. Global Cancer Statistics 2020: GLOBOCAN Estimates of Incidence and Mortality Worldwide for 36 Cancers in 185 Countries. CA Cancer J Clin 2021;71:209-49. [Crossref] [PubMed]
- Simonetti A, Mariotto A, Krapcho M, et al. Improved population-based probability of developing cancer when direct estimates of the cancer-free population are available. Lifetime Data Anal 2012;18:284-301. [Crossref] [PubMed]
- Zhan C, Yan L, Wang L, et al. Identification of immunohistochemical markers for distinguishing lung adenocarcinoma from squamous cell carcinoma. J Thorac Dis 2015;7:1398-405. [Crossref] [PubMed]
- Liu Y, Kim J, Balagurunathan Y, et al. Radiomic Features Are Associated With EGFR Mutation Status in Lung Adenocarcinomas. Clin Lung Cancer 2016;17:441-448.e6. [Crossref] [PubMed]
- Santarpia M, Liguori A, D'Aveni A, et al. Liquid biopsy for lung cancer early detection. J Thorac Dis 2018;10:S882-97. [Crossref] [PubMed]
- Liang W, Zhao Y, Huang W, et al. Liquid biopsy for early stage lung cancer. J Thorac Dis 2018;10:S876-81. [Crossref] [PubMed]
- Cecchini MJ, Yi ES. Liquid biopsy is a valuable tool in the diagnosis and management of lung cancer. J Thorac Dis 2020;12:7048-56. [Crossref] [PubMed]
- Kumar V, Gu Y, Basu S, et al. Radiomics: the process and the challenges. Magn Reson Imaging 2012;30:1234-48. [Crossref] [PubMed]
- Tomaszewski MR, Gillies RJ. The Biological Meaning of Radiomic Features. Radiology 2021;298:505-16. [Crossref] [PubMed]
- Lambin P, Rios-Velazquez E, Leijenaar R, et al. Radiomics: extracting more information from medical images using advanced feature analysis. Eur J Cancer 2012;48:441-6. [Crossref] [PubMed]
- Lambin P, Leijenaar RTH, Deist TM, et al. Radiomics: the bridge between medical imaging and personalized medicine. Nat Rev Clin Oncol 2017;14:749-62. [Crossref] [PubMed]
- Wu G, Jochems A, Refaee T, et al. Structural and functional radiomics for lung cancer. Eur J Nucl Med Mol Imaging 2021;48:3961-74. [Crossref] [PubMed]
- Parmar C, Grossmann P, Bussink J, et al. Machine Learning methods for Quantitative Radiomic Biomarkers. Sci Rep 2015;5:13087. [Crossref] [PubMed]
- Avanzo M, Stancanello J, Pirrone G, et al. Radiomics and deep learning in lung cancer. Strahlenther Onkol 2020;196:879-87. [Crossref] [PubMed]
- Beig N, Khorrami M, Alilou M, et al. Perinodular and Intranodular Radiomic Features on Lung CT Images Distinguish Adenocarcinomas from Granulomas. Radiology 2019;290:783-92. [Crossref] [PubMed]
- Thawani R, McLane M, Beig N, et al. Radiomics and radiogenomics in lung cancer: A review for the clinician. Lung Cancer 2018;115:34-41. [Crossref] [PubMed]
- Tunali I, Gillies RJ, Schabath MB. Application of Radiomics and Artificial Intelligence for Lung Cancer Precision Medicine. Cold Spring Harb Perspect Med 2021;11:a039537. [Crossref] [PubMed]
- Ji Y, Qiu Q, Fu J, et al. Stage-Specific PET Radiomic Prediction Model for the Histological Subtype Classification of Non-Small-Cell Lung Cancer. Cancer Manag Res 2021;13:307-17. [Crossref] [PubMed]
- Zhu X, Dong D, Chen Z, et al. Radiomic signature as a diagnostic factor for histologic subtype classification of non-small cell lung cancer. Eur Radiol 2018;28:2772-8. [Crossref] [PubMed]
- Meier-Schroers M, Homsi R, Gieseke J, et al. Lung cancer screening with MRI: Evaluation of MRI for lung cancer screening by comparison of LDCT- and MRI-derived Lung-RADS categories in the first two screening rounds. Eur Radiol 2019;29:898-905. [Crossref] [PubMed]
- Meier-Schroers M, Homsi R, Schild HH, et al. Lung cancer screening with MRI: characterization of nodules with different non-enhanced MRI sequences. Acta Radiol 2019;60:168-76. [Crossref] [PubMed]
- Dewes P, Frellesen C, Al-Butmeh F, et al. Comparative evaluation of non-contrast CAIPIRINHA-VIBE 3T-MRI and multidetector CT for detection of pulmonary nodules: In vivo evaluation of diagnostic accuracy and image quality. Eur J Radiol 2016;85:193-8. [Crossref] [PubMed]
- Clark TJ, Flood TF, Maximin ST, et al. Lung CT Screening Reporting and Data System Speed and Accuracy Are Increased With the Use of a Semiautomated Computer Application. J Am Coll Radiol 2015;12:1301-6. [Crossref] [PubMed]
- Herth FJ, Hoffmann H, Heussel CP, et al. Lung cancer screening--update 2014. Pneumologie 2014;68:781-3. [Crossref] [PubMed]
- Lacroix M, Frouin F, Dirand AS, et al. Correction for Magnetic Field Inhomogeneities and Normalization of Voxel Values Are Needed to Better Reveal the Potential of MR Radiomic Features in Lung Cancer. Front Oncol 2020;10:43. [Crossref] [PubMed]
- Tang X, Xu X, Han Z, et al. Elaboration of a multimodal MRI-based radiomics signature for the preoperative prediction of the histological subtype in patients with non-small-cell lung cancer. Biomed Eng Online 2020;19:5. [Crossref] [PubMed]
- Galldiks N, Kocher M, Ceccon G, et al. Imaging challenges of immunotherapy and targeted therapy in patients with brain metastases: response, progression, and pseudoprogression. Neuro Oncol 2020;22:17-30. [Crossref] [PubMed]
- Lang N, Zhang Y, Zhang E, et al. Differentiation of spinal metastases originated from lung and other cancers using radiomics and deep learning based on DCE-MRI. Magn Reson Imaging 2019;64:4-12. [Crossref] [PubMed]
- Mahon RN, Hugo GD, Weiss E. Repeatability of texture features derived from magnetic resonance and computed tomography imaging and use in predictive models for non-small cell lung cancer outcome. Phys Med Biol 2019; Epub ahead of print. [Crossref]
- Wang W, Cao K, Jin S, et al. Differentiation of renal cell carcinoma subtypes through MRI-based radiomics analysis. Eur Radiol 2020;30:5738-47. [Crossref] [PubMed]
- Tang X, Bai G, Wang H, et al. Elaboration of Multiparametric MRI-Based Radiomics Signature for the Preoperative Quantitative Identification of the Histological Grade in Patients With Non-Small-Cell Lung Cancer. J Magn Reson Imaging 2022;56:579-89. [Crossref] [PubMed]
- Mayerhoefer ME, Materka A, Langs G, et al. Introduction to Radiomics. J Nucl Med 2020;61:488-95. [Crossref] [PubMed]
- Ergen B, Baykara M. Texture based feature extraction methods for content based medical image retrieval systems. Biomed Mater Eng 2014;24:3055-62. [Crossref] [PubMed]
- Alic L, Niessen WJ, Veenland JF. Quantification of heterogeneity as a biomarker in tumor imaging: a systematic review. PLoS One 2014;9:e110300. [Crossref] [PubMed]
- Lubner MG, Smith AD, Sandrasegaran K, et al. CT Texture Analysis: Definitions, Applications, Biologic Correlates, and Challenges. Radiographics 2017;37:1483-503. [Crossref] [PubMed]
- Kirienko M, Sollini M, Corbetta M, et al. Radiomics and gene expression profile to characterise the disease and predict outcome in patients with lung cancer. Eur J Nucl Med Mol Imaging 2021;48:3643-55. [Crossref] [PubMed]
- Sun Y, Li C, Jin L, et al. Radiomics for lung adenocarcinoma manifesting as pure ground-glass nodules: invasive prediction. Eur Radiol 2020;30:3650-9. [Crossref] [PubMed]
- Ren C, Zhang J, Qi M, et al. Machine learning based on clinico-biological features integrated (18)F-FDG PET/CT radiomics for distinguishing squamous cell carcinoma from adenocarcinoma of lung. Eur J Nucl Med Mol Imaging 2021;48:1538-49. [Crossref] [PubMed]
- Tang X, Liang J, Xiang B, et al. Positron Emission Tomography/Magnetic Resonance Imaging Radiomics in Predicting Lung Adenocarcinoma and Squamous Cell Carcinoma. Front Oncol 2022;12:803824. [Crossref] [PubMed]
- Brenner DJ. Radiation risks potentially associated with low-dose CT screening of adult smokers for lung cancer. Radiology 2004;231:440-5. [Crossref] [PubMed]
- Polan DF, Brady SL, Kaufman RA. Tissue segmentation of computed tomography images using a Random Forest algorithm: a feasibility study. Phys Med Biol 2016;61:6553-69. [Crossref] [PubMed]
- Pavic M, Bogowicz M, Würms X, et al. Influence of inter-observer delineation variability on radiomics stability in different tumor sites. Acta Oncol 2018;57:1070-4. [Crossref] [PubMed]
- He L, Huang Y, Ma Z, et al. Effects of contrast-enhancement, reconstruction slice thickness and convolution kernel on the diagnostic performance of radiomics signature in solitary pulmonary nodule. Sci Rep 2016;6:34921. [Crossref] [PubMed]
(English Language Editor: J. Jones)