New method for delineation of the intersegmental line in a deflated lung
Highlight box
Key findings
• The implementation of the lung deflation simulation algorithm in the three-dimensional (3D) lung image allows for a more accurate understanding of the lines between lung segments.
What is known and what is new?
• Several image-assisted systems have emerged and are being used in real-world clinical practice to provide a more accurate view of intraoperative anatomy in lung surgery. Although these systems are known to allow for efficient and safe surgery, the ability to deflate the lungs has not been implemented.
• The algorithm used in this study includes a function to deflate the lungs, and this is the first report evaluating its usefulness.
What is the implication, and what should change now?
• Improvements of the lung deflation simulation algorithm, and its use in parallel with existing image sup-port systems, could enable the evaluation of intersegmental lines with greater accuracy.
Introduction
Background
Recently, several image-assisted systems have been developed that show more accurate intraoperative anatomy during lung surgery (1,2). By using these systems during surgery, it is now possible to confirm the location of the pulmonary arteriovenous system and bronchioles, identify the location of tumors, and set segment borderlines in segmentectomies, leading to improved surgical safety and reduced stress for the surgeon (3). These image support systems are based on computed tomography (CT) images taken during inspiration. However, in actual surgery, anesthesia is managed by one-lung ventilation, and the lung on the operative side collapses. Because the position of the blood vessels and tumors changes with collapsed lungs, the surgeon must compensate for these changes while performing the surgery, which requires a certain degree of experience.
Rationale and knowledge gap
In the future, the number of segmentectomies, particularly for lung cancer, is expected to increase. In the case of segmentectomy, it is important to accurately identify the intersegmental lines. Intraoperative intravenous administration of indocyanine green is the main method of identifying the intersegmental line, but its disadvantages include the need for specialized equipment and drug administration, as well as the fact that the correct intersegmental line cannot always be determined (4). In addition, the lung is an organ that deforms greatly depending on the amount of air it contains, and the intraoperative lung shape differs greatly from that on the CT image.
Objective
In this study, we developed a lung-deflating algorithm. We created a model that could delineate the intersegmental lines in the deflated state and compared it with the actual surgical field to investigate its usefulness. This novel pulmonary deflation model eliminates the need for drug administration and special equipment. The accuracy of this method was evaluated by comparing it with the commonly used indocyanine green method, which is used for identifying segment borderlines. We present this article in accordance with the STARD reporting checklist (available at https://jtd.amegroups.com/article/view/10.21037/jtd-23-421/rc).
Methods
Patients
Patients who underwent unilateral robot-assisted segmentectomy at Nagoya University Hospital between January 1, 2020, and June 30, 2022 were included in the study. The study was conducted in accordance with the Declaration of Helsinki (as revised in 2013). The study protocol was approved by the Institutional Review Board of Nagoya University School of Medicine (No. 2015-04587082), and written informed consent was obtained from all participants. The segmentectomy procedure consisted of first treating the pulmonary vessels and bronchi of the segment, followed by transvenous administration of indocyanine green and dissection of the lung parenchyma at the border of the fluoresced area. Patients with a history of ipsilateral lung resection, those who had undergone a combined resection of another lung, those who had not undergone contrast-enhanced CT, or those with unclear borders due to intraoperative indocyanine green administration were excluded.
Creation of a three-dimensional (3D) model for segmentectomy
In all cases, a 1-mm thin-slice contrast-enhanced CT was performed before surgery. Our department routinely performs contrast-enhanced CT in patients undergoing pneumonectomy to generate 3D images, unless there are other non-adaptive factors such as low renal function. Using the 3D image analysis system SYNAPSE VINCENT (Fujifilm Medical Co., Ltd., Tokyo, Japan), 3D images of the pulmonary vessels and bronchi were created from the CT images. SYNAPSE VINCENT was kindly loaned free of charge by Fujifilm Medical Co. Ltd. A 3D image was created from the CT images within approximately 5 min. The pulmonary arteries running through the segment to be resected were designated in the 3D image, and the dominant region of the parenchymal lung area was identified (Video 1). In addition, the ribs and spinal column were depicted as reference landmarks so that the angles would match those in the surgical video as closely as possible. Using the lung deflation simulation algorithm on this 3D image, it was possible to deflate the lung to match the intraoperative collapsed lung (Video 2). This system was created by predicting the intraoperative deflated lungs based on the deformable registration of the collapsed lungs. After the analysis of the CT data of the pneumothorax lungs and the intraoperative cone-beam CT data of the deflated and inflated lungs, the algorithm of this system was established by statistical lung modeling based on a complete deformable mesh registration solution for mapping the lung surface and bronchial structures (5,6). This algorithm is based on the fact that the lung deflates during surgery because of deflation, manipulation, or traction.
Comparison of intersegmental line
From the surgical video, we identified a scene in which the intersegmental line was detected using indocyanine green. The angles of the 3D images were matched to the ribs and spinal column to ensure that the same angles were being viewed. Then the 3D images were deflated to match the lung in the surgical video. The actual intersegmental line in the surgical video was then compared to that created in the deflation simulation algorithm, and the two lines were evaluated by two respiratory surgeons (Okado S, Chen-Yoshikawa TF) on three levels (agreement, partial agreement, and disagreement) to determine if they matched.
Results
Twenty-eight patients underwent robot-assisted segmentectomies during the study period. Twelve patients were excluded (contrast-enhanced CT was not performed in six patients, and the intersegmental line was not clearly delineated by indocyanine green in six other patients). Finally, 16 patients were included (Figure 1). Robot-assisted thoracoscopic surgery was performed in all patients, and no intrapleural adhesions were observed. The median age was 70.5 years, and nine patients were female. Primary lung cancer was the most common diagnosis (14 cases), 13 of which were adenocarcinomas. The most common site of resection was S1–3 of the left lung in seven cases. A history of smoking was observed in 11 patients; however, most patients did not have chronic lung diseases such as emphysema or interstitial pneumonia (Table 1). In all cases, the vessels and bronchi were clearly visualized by SYNAPSE VINCENT, and segment delineation was clearly visible in the surgical movies. A comparison showed agreement between the actual intersegmental line and the 3D image made by the lung deflation simulation algorithm in twelve cases, partial agreement in three cases, and disagreement in one case (Table 2). Partial agreement was observed in two cases of right lung S6 segmentectomy and in one case of left lung S6 segmentectomy, and disagreement was observed in one case of left lung S6 segmentectomy. The concordance rate of the intersegmental lines in the 16 cases studied was 75%.
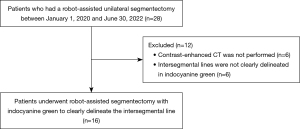
Table 1
Variables | All patients (n=16) |
---|---|
Age, years, median [range] | 70.5 [60–82] |
Male, n (%) | 7 (43.8) |
Diagnosis, n (%) | |
Lung cancer | 14 (87.5) |
Metastasis | 2 (12.5) |
Smoking history (ever), n (%) | 11 (68.8) |
Comorbidity, n (%) | |
Emphysema | 1 (6.3) |
Interstitial pneumonia | 2 (12.5) |
Tumor size, mm, mean [range] | 17.5 [7–31] |
Resected lung segment, n (%) | |
RS6 | 3 (18.8) |
RS7–10 | 2 (12.5) |
RS8 | 1 (6.3) |
LS1–3 | 7 (43.8) |
LS4–5 | 1 (6.3) |
LS6 | 2 (12.5) |
RS6, right lung segment 6; RS7–10, right lung segment 7–10; RS8, right lung segment 8; LS1–3 left lung segment 1–3; LS4–5, left lung segment 4–5; LS6, left lung segment 6.
Table 2
Resected lung segment (n=16) | Agreement | Partial agreement | Disagreement |
---|---|---|---|
RS6 (n=3) | 1 | 2 | 0 |
RS7–10 (n=2) | 2 | 0 | 0 |
RS8 (n=1) | 1 | 0 | 0 |
LS1–3 (n=7) | 7 | 0 | 0 |
LS4–5 (n=1) | 1 | 0 | 0 |
LS6 (n=2) | 0 | 1 | 1 |
RS6, right lung segment 6; RS7–10, right lung segment 7–10; RS8, right lung segment 8; LS1–3 left lung segment 1–3; LS4–5, left lung segment 4–5; LS6, left lung segment 6.
Case one: left lung S4–5 segmentectomy
In case 1, a left lung S4–5 segmentectomy was performed, and the 3D image showed a gentle meander on the abdominal side, followed by an inverted U-shaped zonation on the dorsal side, wrapping around the tip of the forceps (Figure 2). The surgical video shows the same inverted U-shaped intersegmental line around the tip of the forceps. The results show that the lung segments can be accurately delineated based on the vascular run; hence, it was determined to be in “agreement”.
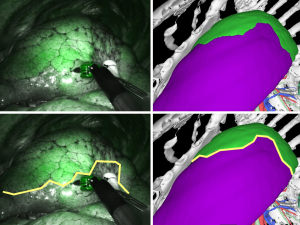
Case two: right lung S6 segmentectomy
In case 2, a patient underwent right lung S6 segmentectomy. The intersegmental lines were in agreement on the dorsal side; however, on the ventral side, the line on the 3D image was meandering and did not match the line that can be seen in the surgical video. Therefore, it was determined to be in “partial agreement” (Figure 3).
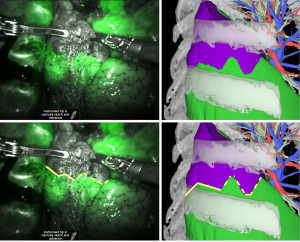
Case three: left lung S6 segmentectomy
In case 3, a patient underwent left lung S6 segmentectomy, and the indocyanine green image showed a large shift of the intersegmental line to the basal segment compared to that in the 3D image (Figure 4). We judged this result to be in “disagreement”.
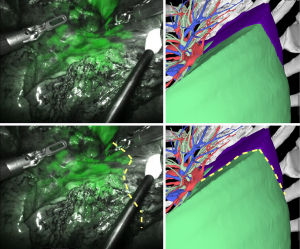
Discussion
This study revealed that the lung intersegmental line delineated with the lung deflation simulation algorithm was generally consistent with those delineated intraoperatively with indocyanine green. This is the first report on segment delineation using deflated 3D images. In this study, 16 patients were examined retrospectively, and the agreement rate for segmental delineation was 75%. The lines did not match at all in only one case. Based on the assumption that indocyanine green is a valid method for delineating intersegmental lines, we can say that the lung deflation simulation algorithm is generally able to delineate reliable lines. In this study, the background ribs and spine were aligned so that the intraoperative images could be compared as accurately as possible with 3D images.
Recently, various imaging techniques have been reported that assist in lung surgery (2,7,8). For example, the Resection Process Map (RPM) reported by Tokuno et al. can show the location of the pulmonary vessels and bronchi in an intraoperative context, thus helping the surgeon understand the anatomy as surgery progresses (9). Currently, several other commercially available 3D imaging systems are available, including AquariusNET (TeraRecon, Inc., San Mateo, CA, USA), Mimics (Materialise Nv Co., Belgium), and Ziostation2 (Ziosoft, Tokyo, Japan). All these systems construct 3D images based on CT images and provide detailed anatomy, but none have the ability to deflate the lungs (10-14). The novelty of this deflation system is that it can deflate the lungs, which is a significant advantage over existing 3D imaging techniques. It also has the potential to replace several conventional methods of identifying intersegmental lines.
In the present study, the delineation of the segments was based on the direction of the pulmonary arteries. The main intraoperative methods for delineating zones are based on the perfusion area of the pulmonary artery using indocyanine green or the line of ventilation after the bronchus has been detached (15,16). Intersegmental pulmonary veins are also located in the intersegmental space, providing clues for identifying the intersegmental line (17). The method which reflects the lung segment more correctly is debatable, but neither method is perfect because conventional way of identification with a ventilation line can be difficult in emphysema patients, and the endoscopic view employed in minimally invasive surgery is not sufficient (18). However, since our department uses indocyanine green to delineate the zones in pulmonary segmentectomy procedures, we also used pulmonary artery delineation in SYNAPSE VINCENT.
However, it is difficult to perfectly determine the intersegmental line. Indocyanine green fluorescence in the operative field is performed at the end of surgery after the pulmonary vessels and bronchi are detached (13). It is difficult to reproduce nonuniformly collapsed lungs using the deflation algorithm; therefore, images with uniformly deflated lungs are used for comparison. It is also difficult to exactly match the angle of the lung parenchyma because of repeated compressions and expansions. Therefore, based on these conditions, a certain degree of error is inevitable in comparisons. However, this is not a factor that makes this technology disadvantageous, as existing image support systems also have similar problems. For example, RPM determines the image angle based on the shape of the entire lung, but as in this report, it is difficult to exactly match the angle (19). Since the determination of the intersegmental line with the lung deflation simulation algorithm alone is still difficult, it is recommended to use in parallel with existing image support systems to evaluate the area boundaries with higher accuracy. Therefore, the use of lung deflation simulation algorithm in parallel with the existing image support systems is considered realistic to evaluate intersegmental lines with higher accuracy.
Intersegmental line concordance could not be obtained in some of the patients who underwent lower lobe S6 segmentectomy, and comparison of the 3D image and intraoperative images showed that the function of the deflation simulation algorithm to deflate lungs was not sufficient in the lower lobe, making it difficult to align with the ribs and spinal column, and at the same time, the morphological differences in the deflated lungs were significant. This was thought to have led to the differences in intersegmental lines (20). It is also possible that comorbidities such as emphysema and interstitial pneumonia may affect the degree of lung collapse. However, the number of patients with such comorbidities in this study cohort was small, making statistical examination difficult. If the functionality of the deflation algorithm is expanded in the future, for example, to deflate each lung lobe separately, a higher reliability can be achieved without being affected by lung lobes or comorbidities.
Finally, another issue is the lack of quantitative assessment of the degree of congruence of intersegmental lines. Because the lung is a 3D object, the accuracy of comparing area boundaries with two-dimensional images is limited. For example, quantitative comparisons can be made by measuring the volume of the resection zone or calculating the area ratio of the resection zone in a two-dimensional image. However, it is difficult to calculate the volume of the resection zone from intraoperative images and to project the entire lung on a single screen; therefore, arbitrary settings are involved in the area ratio of the resection zone. Since it is very difficult to evaluate the results quantitatively, the authors subjectively evaluated the results on a three-point scale in this study. It is desirable in the future to include the ability to set specific coordinates and evaluate images from the same angle.
Conclusions
The lung deflation simulation algorithm was developed to allow comparison under conditions more similar to actual lungs than those of existing surgical support imaging systems. Although the concordance rate was 75% in this study, further improvements in the system may make it more useful as a surgical support tool.
Acknowledgments
Funding: This work was partly supported by grants from
Footnote
Reporting Checklist: The authors have completed the STARD reporting checklist. Available at https://jtd.amegroups.com/article/view/10.21037/jtd-23-421/rc
Data Sharing Statement: Available at https://jtd.amegroups.com/article/view/10.21037/jtd-23-421/dss
Peer Review File: Available at https://jtd.amegroups.com/article/view/10.21037/jtd-23-421/prf
Conflicts of Interest: All authors have completed the ICMJE uniform disclosure form (available at https://jtd.amegroups.com/article/view/10.21037/jtd-23-421/coif). TFCY and MN report that SYNAPSE VINCENT was kindly loaned free of charge by Fujifilm Medical Co., Ltd. and financial support for the collaborative work (500,000 JPY) was provided by Fujifilm Medical Co., Ltd. in 2022, respectively. The other authors have no conflicts of interest to declare.
Ethical Statement: The authors are accountable for all aspects of the work in ensuring that questions related to the accuracy or integrity of any part of the work are appropriately investigated and resolved. The study was conducted in accordance with the Declaration of Helsinki (as revised in 2013). The study protocol was approved by the Institutional Review Board of Nagoya University School of Medicine (No. 2015-04587082), and written informed consent was obtained from all participants.
Open Access Statement: This is an Open Access article distributed in accordance with the Creative Commons Attribution-NonCommercial-NoDerivs 4.0 International License (CC BY-NC-ND 4.0), which permits the non-commercial replication and distribution of the article with the strict proviso that no changes or edits are made and the original work is properly cited (including links to both the formal publication through the relevant DOI and the license). See: https://creativecommons.org/licenses/by-nc-nd/4.0/.
References
- Kadomatsu Y, Nakao M, Ueno H, et al. A novel system applying artificial intelligence in the identification of air leak sites. JTCVS Tech 2022;15:181-91. [Crossref] [PubMed]
- Chen-Yoshikawa TF, Fukui T, Nakamura S, et al. Current trends in thoracic surgery. Nagoya J Med Sci 2020;82:161-74. [PubMed]
- Chen-Yoshikawa TF, Date H. Update on three-dimensional image reconstruction for preoperative simulation in thoracic surgery. J Thorac Dis 2016;8:S295-301. [PubMed]
- Ishizawa T, McCulloch P, Stassen L, et al. Assessing the development status of intraoperative fluorescence imaging for anatomy visualisation, using the IDEAL framework. BMJ Surg Interv Health Technol 2022;4:e000156. [Crossref] [PubMed]
- Nakao M, Kobayashi K, Tokuno J, et al. Deformation analysis of surface and bronchial structures in intraoperative pneumothorax using deformable mesh registration. Med Image Anal 2021;73:102181. [Crossref] [PubMed]
- Yamamoto U, Nakao M, Ohzeki M, et al. Kernel-based framework to estimate deformations of pneumothorax lung using relative position of anatomical land-marks. Expert Systems with Applications 2021;183:115288. [Crossref]
- Fukuhara K, Akashi A, Nakane S, et al. Preoperative assessment of the pulmonary artery by three-dimensional computed tomography before video-assisted thoracic surgery lobectomy. Eur J Cardiothorac Surg 2008;34:875-7. [Crossref] [PubMed]
- Sekine Y, Itoh T, Toyoda T, et al. Precise Anatomical Sublobar Resection Using a 3D Medical Image Analyzer and Fluorescence-Guided Surgery With Transbronchial Instillation of Indocyanine Green. Semin Thorac Cardiovasc Surg 2019;31:595-602. [Crossref] [PubMed]
- Tokuno J, Chen-Yoshikawa TF, Nakao M, et al. Resection Process Map: A novel dynamic simulation system for pulmonary resection. J Thorac Cardiovasc Surg 2020;159:1130-8. [Crossref] [PubMed]
- Yokoyama Y, Sato M, Omasa M, et al. Three-dimensional imaging for thoracoscopic resection of complex lung anomalies. Surg Case Rep 2017;3:106. [Crossref] [PubMed]
- Kato H, Oizumi H, Suzuki J, et al. Thoracoscopic anatomical lung segmentectomy using 3D computed tomography simulation without tumour markings for non-palpable and non-visualized small lung nodules. Interact Cardiovasc Thorac Surg 2017;25:434-41. [Crossref] [PubMed]
- Wu Z, Huang Z, Qin Y, et al. Progress in three-dimensional computed tomography reconstruction in anatomic pulmonary segmentectomy. Thorac Cancer 2022;13:1881-7. [Crossref] [PubMed]
- Yao F, Wang J, Yao J, et al. Three-dimensional image reconstruction with free open-source OsiriX software in video-assisted thoracoscopic lobectomy and segmentectomy. Int J Surg 2017;39:16-22. [Crossref] [PubMed]
- Misaki N, Chang SS, Gotoh M, et al. A novel method for determining adjacent lung segments with infrared thoracoscopy. J Thorac Cardiovasc Surg 2009;138:613-8. [Crossref] [PubMed]
- Okada M, Mimura T, Ikegaki J, et al. A novel video-assisted anatomic segmentectomy technique: selective segmental inflation via bronchofiberoptic jet followed by cautery cutting. J Thorac Cardiovasc Surg 2007;133:753-8. [Crossref] [PubMed]
- Mimae T, Miyata Y, Kumada T, et al. The intersegmental pulmonary vein is not always located on the intersegmental plane of the lung: Evaluation with 3-dimensional volume-rendering image reconstruction. JTCVS Tech 2022;16:132-8. [Crossref] [PubMed]
- Nakamura S, Goto M, Chen-Yoshikawa TF. Fluorescence-guided thoracic surgery. J Vis Surg 2021;7:18. [Crossref]
- Igai H, Matsuura N, Kamiyoshihara M. Preoperative Simulation of Thoracoscopic Segmentectomy. Ann Thorac Surg 2022;114:e295-7. [Crossref] [PubMed]
- Tokuno J, Chen-Yoshikawa TF, Nakao M, et al. Creation of a video library for education and virtual simulation of anatomical lung resection. Interact Cardiovasc Thorac Surg 2022;34:808-13. [Crossref] [PubMed]
- Nakao M, Tokuno J, Chen-Yoshikawa T, et al. Surface deformation analysis of collapsed lungs using model-based shape matching. Int J Comput Assist Radiol Surg 2019;14:1763-74. [Crossref] [PubMed]