Machine learning analysis of primary hyperhidrosis for classification of hyperhidrosis type and prediction of compensatory hyperhidrosis
Highlight box
Key findings
• The machine learning algorithm using heart rate variability parameters and basic clinical data can classify types and predict compensatory hyperhidrosis (CH) after sympathicotomy for primary hyperhidrosis with considerable accuracy.
What is known and what is new?
• This is the first study in which machine learning analysis was clinically used in primary hyperhidrosis.
• Multivariate analysis showed the type and expansion of sympathicotomy were significant factors for CH after sympathicotomy.
What is the implication, and what should change now?
• Further large-scale studies are needed to validate the findings and provide management guidelines for primary hyperhidrosis. In addition, an approach integrating machine learning models and readily available clinical data can be used to develop other models in the thoracic surgery field.
Introduction
Primary hyperhidrosis is a frequently encountered disorder in the thoracic surgery field and mostly occurs in focal and symmetric patterns involving one or more sites in the hands, armpits, plantar surface, and craniofacial region (1-3). Because primary hyperhidrosis causes a poor quality of life, patients with severe primary hyperhidrosis refractory to medical treatment choose surgical treatment (1,4). Although this procedure is highly effective for improving symptom, compensatory hyperhidrosis (CH) after sympathectomy is a major issue and reduce satisfaction of the procedure (5,6). Incidence of CH varies among centers, up to 98% although surgical techniques advances (5-7). Numerous studies have been performed on how to prevent CH and the underlying mechanism remains unknown (3,4,7). Primary hyperhidrosis is considered associated with complex autonomic nervous system dysfunction although the pathophysiology remains unclear (2,3,8). Consequently, CH is also assumed associated with autonomic nervous system traits, evidenced by the changes of some autonomic nervous system measurements after sympathectomy (8,9). However, using autonomic nervous system traits to reduce and predict CH has been investigated in only a few studies and analyzing autonomic nervous system traits in patients with primary hyperhidrosis is necessary (8,9). The heart rate variability (HRV) test is performed to evaluate autonomic nervous system traits (8,10). HRV consists of physiologic variations in the time intervals between consecutive heartbeats that help the cardiovascular system to rapidly adapt to sudden challenges to homeostasis (9-11). In previous studies, HRV findings were reported to change after sympathectomy (9,12). In our previous study, HRV was shown to provide useful information regarding the pathophysiology of primary hyperhidrosis and improve preoperative risk stratification of CH (2). The aims of the present study are to investigate the characteristics of primary hyperhidrosis and to predict the degree of CH using HRV findings and basic clinical data. Machine learning analysis was used to determine the hyperhidrosis type and predict the degree of CH. We present this article in accordance with the STROBE reporting checklist (available at https://jtd.amegroups.com/article/view/10.21037/jtd-23-471/rc).
Methods
Study population, eligibility assessment, and surgical techniques
From March 2017 to December 2021, data on consecutive subjects with primary hyperhidrosis (craniofacial vs. palmar) who underwent preoperative HRV tests and sympathicotomy in Uijeongbu Saint Mary’s Hospital were reviewed. Eligibility criteria for the present study were the followings: (I) primary hyperhidrosis involving the craniofacial region or hands; (II) no previous thoracic procedure; (III) no routine intakes of antihypertensive or psychotropic medications; (IV) no alcohol, caffeinated drinks, or smoking 1 day before HRV test; and (V) no obvious cardiac arrythmia. Data on subject characteristics, disease status, operative procedures, and postoperative outcomes were retrospectively collected using medical chart reviews and telephone surveys. The telephone survey using a questionnaire was conduct in July 2022 and included symptom improvement, recurrence, degree of CH, and overall satisfaction after sympathicotomy. Symptom improvement was described as success (significant sweat reduction) or failure (no change or recurrence). The degrees of CH were divided into two levels: low (negligible and mild) and high (moderate and severe). Primary hyperhidrosis involving the hands except craniofacial regions was defined as palmar type. All subjects underwent bilateral endoscopic sympathicotomy with or without the expansion of sympathicotomy (13,14). T2 and T3 sympathicotomy were routinely performed in patients with craniofacial and palmar hyperhidrosis, respectively (1,5). In the expansion sympathicotomy, we expanded the level of sympathicotomy to levels ranging from R5 to R12 by chain ablation to prevent compensatory while avoiding injury to the splanchnic nerves (14). The last follow-up in the outpatient clinic is routinely preformed in one month after sympathectomy.
HRV tests
HRV test before sympathicotomy was routinely performed to investigate characteristics of primary hyperhidrosis and analyze the association with postoperative outcomes. The 5-minute HRV test was performed using the SA-3000P model (Medi-core, Seoul, Korea). The HRV test laboratory was soundproof, illuminated by a fluorescent lamp, and maintained at room temperature (25 ℃). Each subject was asked to remove metal accessories and comfortably sit in a chair with electrodes placed on the left ankle and both wrists to acquire HRV measurements. The subject was instructed to breathe normally during the measurement. Time- and frequency-domains in the HRV test were collected (15). Time-domain parameters quantify the amount of HRV observed during test periods (8,10,15). Frequency-domain indices demonstrate how much of a signal energy within component bands with certain frequency bands tend to relate with certain physiological phenomena, such as sympathetic or parasympathetic nervous system activity and their balance (8,10). The following HRV parameters in time- and frequency-domains were gathered. Time-domain parameters included standard deviation of normal-to-normal (SDNN) interval and root mean square of successive differences (RMSSD) of neural network (NN) intervals, and frequency-domain parameters included total power (TP, 0–0.4 Hz), very low frequency (VLF, 0.0033–0.04 Hz), low frequency (LF, 0.04–0.15 Hz), and high frequency (HF, 0.15–0.4 Hz) (15). LF, HF, and LF:HF ratio provide information regarding the autonomic nervous system balance or activity (2,10).
Statistics analysis
Continuous variables are presented as mean ± standard deviation (SD) and categorical variables are presented by number in the group. Statistics evaluation was performed using nonparametric method because the normality assumption was not tenable. Comparisons between the two groups were performed using the Mann-Whitney U test, analysis of covariance, or chi-square test. Multivariate analysis was performed using the binary logistic regression (LR) test (backward method) to investigate independent influencing parameters for CH. The data were evaluated using the Statistical Package of Social Sciences version 22.0 (SPSS, IBM Corp, Armonk, NY, USA). A P value <0.05 was considered statistically significant.
Machine learning analysis
Machine learning algorithms were used to classify the hyperhidrosis type and predict the degree of CH. The training and validation sets were used for model learning and optimal model selection and the performance of this model was evaluated. Five-fold stratified cross-validation was used for training and validation. The performance of all models was compared based on the mean area under the curve (AUC). The performance was evaluated based on AUC, accuracy, precision, F1 score, and recall. Five machine learning algorithms [LR, NN, decision tree (DT), random forest (RF), and support vector machine (SVM)] were used to classify the hyperhidrosis type and predict the degree of CH. Orange® data mining toolbox in Python (Bioinformatics Lab at University of Ljubljana, Slovenia) was used to evaluate the machine learning models (16). This program is an open-source data mining program, and the main advantage is that machine learning models can be tested and evaluated simultaneously (16). The detailed structure for each model includes DT (induce binary tree, minimum number of instances in leave: 2, do not split subsets smaller than 5, limit maximum tree depth: 100, and stop when majority reaches: 95%), RF (number of trees: 10, replicable training, do not split subsets smaller than 5), SVM [cost: 1.0, regression loss epsilon: 0.10, kernel: radial basis function (RBF), optimization numerical tolerance: 0.0010, and optimization iteration limit: 100], LR (regularization type: ridge L2, strength C=1), and NN (neurons in the hidden layers: 100, 50, 20, activation: ReLu, solver: Adam, regularization α=0.0001, and maximum number of iterations: 200, replicable training).
Ethical statements
The study was conducted in accordance with the Declaration of Helsinki (as revised in 2013). Uijeongbu Saint Mary’s Hospital Ethics Committee reviewed and approved the present study (No. UC23RISI0022). The need for informed consent was waived because the present study was retrospective without disclosure of patient information.
Results
The mean follow-up period was 31.7 months (±20.3). A total of 128 Korean ethnicity subjects were included in the study. The mean age of study subjects at sympathicotomy was 30.1 years (±16.7). Subjects were divided into two by the mean age. Among included subjects, 74 (57.8%) were male and 54 (42.2%) were female. Hyperhidrosis types included 46 (35.9%) craniofacial and 82 (64.1%) palmar cases (Figure 1). All subjects underwent the routine two-port thoracoscopic sympathicotomy. The palmar type was significantly more prevalent in younger patients (P<0.001). Symptom improvement after sympathicotomy did not differ based on the type. Low and high degree of CH were observed in 93 (72.7%) and 35 (27.3%) subjects, respectively. The summarized clinical characteristics of the study subjects are shown in Table 1.
Table 1
Variables | Hyperhidrosis | P value | |
---|---|---|---|
Craniofacial type (n=46) | Palmar type (n=82) | ||
Age (year) | 49.89±11.4 | 21.3±8.1 | <0.001 |
Age group | <0.001 | ||
Young group (≤30 years old) | 4 (3.1) | 71 (55.5) | |
Old group (>30 years old) | 42 (32.8) | 11 (8.6) | |
Sex | 0.42 | ||
Male | 21 (16.4) | 53 (41.4) | |
Female | 25 (19.5) | 29 (22.7) | |
Level of sympathicotomy | <0.001 | ||
T2 | 46 (35.9) | 0 (0) | |
T3 | 0 (0) | 82 (64.1) | |
Sweat reduction | 0.056 | ||
Success | 42 (32.8) | 81 (63.3) | |
Failure (no change or recurrence) | 4 (3.1) | 1 (0.8) | |
Degree of compensatory hyperhidrosis | <0.001 | ||
Low | 17 (13.3) | 76 (59.4) | |
High | 29 (22.7) | 6 (4.7) | |
Sympathicotomy techniques | <0.001 | ||
Expansion of sympathicotomy | 25 (19.5) | 77 (60.2) | |
No expansion of sympathicotomy | 21 (16.4) | 5 (3.9) |
Data are presented as mean ± standard deviation or n (%).
HRV assessments of primary hyperhidrosis
Because autonomic nervous system traits are affected by age and sex, the groups were compared using the analysis of covariance test (after controlling for age group and sex) (10,15). Patients with palmar hyperhidrosis had significantly greater SDNN (P=0.030), RMSSD (P=0.004), TP (P=0.041), and LF (P=0.022) than subjects with the craniofacial type. These findings indicate that autonomic nervous system traits differ based on hyperhidrosis type. Autonomic nervous system balance is defined as the ratio of LF to HF assuming LF power is generated by the sympathetic nervus system activity and HF power is generated by the parasympathetic nervous system activity (15). Normal range of ratio of LF to HF is 0.5–2.0 (10,15). The imbalance of autonomic nervous system activity was not different according to types. Sympathetic nervous system predominance was observed in all subjects and both types but was significantly more prevalent in the craniofacial type than in palmar type (P=0.019) (Table 2). Comparisons of HRV parameters according to hyperhidrosis types are shown in Table 2.
Table 2
Variables | Hyperhidrosis type | P value | |
---|---|---|---|
Craniofacial type (n=46) | Palmar type (n=82) | ||
SDNN (ms) | 34.4±2.9 | 4330±1.9 | 0.030 |
RMSSD (ms) | 25.3±3.7 | 40.6±2.5 | 0.004 |
TP (ms2) | 1,105.6±244.8 | 1,815.6±163.4 | 0.041 |
VLF (ms2) | 782.2±170.0 | 829.4±113.5 | 0.843 |
LF (ms2) | 268.4±68.1 | 490.0±45.5 | 0.022 |
HF (ms2) | 247.8±109.7 | 498.7±73.2 | 0.106 |
LF/HF | 1.6±0.4 | 2.2±0.3 | 0.293 |
Autonomic predominance | 0.019 | ||
Sympathetic | 37 (28.9) | 48 (37.5) | |
Parasympathetic | 9 (7.0) | 34 (26.6) | |
Autonomic imbalance | 0.268 | ||
Balanced | 25 (19.5) | 35 (27.3) | |
Imbalanced | 21 (16.4) | 47 (36.7) |
Data are presented as mean ± standard deviation or n (%). SDNN, standard deviation of normal-to-normal; RMSSD, root mean square of successive differences; TP, total power; VLF, very low frequency; LF, low frequency; HF, high frequency.
HRV evaluations with postoperative outcomes after sympathicotomy
Among 128 subjects, 123 (96.1%) improved and 5 (3.9%) showed no change or experienced recurrence. Significant difference was not observed in HRV parameters based on symptom improvement after sympathicotomy (Table 3). High-degree CH in the craniofacial and palmar type were 29 (63.0%) and 6 (7.3%), respectively. High-degree CH after sympathicotomy was developed more often in the craniofacial type (P<0.001). Low degree of CH had significantly greater RMSSD (P=0.047) and high degree of CH showed more sympathetic predominance (P=0.006). However, autonomic nervous system imbalance did not differ based on degree of CH (Table 4). Univariate analysis showed RMSSD and autonomic nervous system predominance based on HRV parameters were associated with developing CH. Based on basic clinical data available, developing CH after sympathicotomy was also associated with hyperhidrosis type and body mass index (BMI). In our previous study, expansion of sympathicotomy was shown associated with developing CH (14). Therefore, age group, sex, BMI, expansion of sympathicotomy, hyperhidrosis type, RMSSD, and autonomic nervous system predominance were included as covariates in multivariate analysis which was performed using a binary LR test (backward method); palmar type and expansion of sympathicotomy were significant influencing factors for CH after sympathicotomy (P=0.001 and P=0.028, respectively; Table 5).
Table 3
Variables | Symptom improvement | P value | |
---|---|---|---|
Failure (n=5) | Success (n=123) | ||
SDNN (ms) | 36.2±6.8 | 40.3±1.3 | 0.554 |
RMSSD (ms) | 35.9±8.9 | 35.1±1.7 | 0.927 |
TP (ms2) | 1,281.2±575.1 | 1,571.8±111.2 | 0.622 |
VLF (ms2) | 646.8±392.8 | 820.4±75.9 | 0.613 |
LF (ms2) | 401.4±160.8 | 410.7±31.1 | 0.955 |
HF (ms2) | 392.3±256.3 | 409.2±49.5 | 0.949 |
LF/HF | 2.4±1.0 | 2.0±0.2 | 0.703 |
Autonomic predominance | 0.663 | ||
Sympathetic | 4 (3.1) | 81 (63.3) | |
Parasympathetic | 1 (0.8) | 42 (32.8) | |
Autonomic imbalance | 0.370 | ||
Balanced | 1 (0.8) | 59 (46.1) | |
Imbalanced | 4 (3.1) | 64 (50.0) |
Data are presented as mean ± standard deviation or n (%). SDNN, standard deviation of normal-to-normal; RMSSD, root mean square of successive differences; TP, total power; VLF, very low frequency; LF, low frequency; HF, high frequency.
Table 4
Variables | Compensatory hyperhidrosis | P value | |
---|---|---|---|
Low degree (n=93) | High degree (n=35) | ||
SDNN (ms) | 41.7±1.5 | 36.0±2.6 | 0.071 |
RMSSD (ms) | 37.4±2.0 | 29.2±3.4 | 0.047 |
TP (ms2) | 1,658.0±130.3 | 1,301.2±221.6 | 0.183 |
VLF (ms2) | 804.1±89.7 | 834.5±152.4 | 0.869 |
LF (ms2) | 434.4±36.5 | 346.3±62.0 | 0.239 |
HF (ms2) | 464.5±57.7 | 259.8±98.2 | 0.085 |
LF/HF | 2.0±0.2 | 2.0±0.4 | 0.998 |
Autonomic predominance | 0.006 | ||
Sympathetic | 55 (43.0) | 30 (23.4) | |
Parasympathetic | 38 (29.7) | 5 (3.9) | |
Autonomic imbalance | 0.556 | ||
Balanced | 42 (32.8) | 18 (14.1) | |
Imbalanced | 51 (39.8) | 17 (13.3) |
Data are presented as mean ± standard deviation or n (%). SDNN, standard deviation of normal-to-normal; RMSSD, root mean square of successive differences; TP, total power; VLF, very low frequency; LF, low frequency; HF, high frequency.
Table 5
Variables | P value | Relative risk | 95% confidence interval |
---|---|---|---|
Old-age group | 0.116 | 0.144 | 0.013–1.617 |
Palmar type | 0.001 | 0.015 | 0.001–0.171 |
Parasympathetic dominance | 0.061 | 0.283 | 0.076–1.060 |
Expansion of sympathicotomy | 0.028 | 0.258 | 0.077–0.862 |
Classification of hyperhidrosis type using the machine learning algorithms
Five machine learning algorithms (LR, NN, DT, RF, and SVM) were used to classify the hyperhidrosis type. Age group, sex, BMI, and HRV parameters (SDNN, RMSSD, TP, VLF, LF, HF, and LF:FH ratio) were included as variables in the machine learning algorithms. The NN model outperformed the other classifying models for the training datasets. The performance scores of the RF model were 0.972 AUC, 0.961 accuracy, 0.961 F1 score, 0.961 precision, and 0.961 recall (Figure 2).
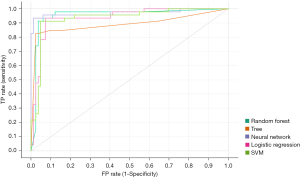
Prediction of degree of CH using machine learning model
The above five machine learning algorithms were used to predict the degree of CH after sympathicotomy. Age group, sex, BMI, expansion of sympathicotomy, hyperhidrosis type, and HRV parameters (SDNN, RMSSD, TP, VLF, LF, HF, and LF:FH ratio) were included as variables in the machine learning algorithms. The RF model outperformed the other predicting models for the training datasets. The performance scores of the RF model were 0.914 AUC, 0.852 accuracy, 0.853 F1 score, 0.856 precision, and 0.852 recall (Figure 3).
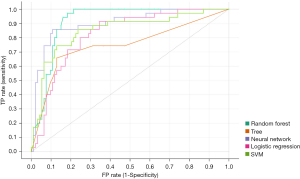
Discussion
Autonomic nervous system activity and balance are associated with the pathophysiology of primary hyperhidrosis and studies have been performed on autonomic nervous system attributes to increase understanding of primary hyperhidrosis (2,8). However, the association between autonomic nervous system attributes and primary hyperhidrosis remains unclear. The HRV test is clinically available to evaluate autonomic nervous system attributes (9,10). HRV is a physiologic phenomenon and indicates neurocardiac function generated by neurocardiac interactions and controlled by the autonomic nervous system (11,15). HRV is clinically used as predictor of certain conditions, such as mortality after cardiac disease and a prognostic factor for diverse diseases (10,17). The use of HRV for primary hyperhidrosis has been investigated in previous studies and changes in HRV after sympathectomy were reported, indicating the possible association of autonomic nervous system attributes with primary hyperhidrosis (8,9,13). However, HRV findings have minimal clinical usefulness in the field of primary hyperhidrosis.
CH is considered an important issue for sympathicotomy in patients with primary hyperhidrosis (3,5,6). Risk factors for CH and feasible techniques to prevent CH have been suggested in numerous studies (3,5,7,17). However, the results were inconclusive and other novel solutions are needed (2,18). We hypothesized that HRV findings provide novel parameters to prevent CH and previously published the possible association of HRV parameters with CH (2). The accurate prediction of CH is crucial for preventing CH and the prediction modality should provide the influencing factors and predictive value for CH development (19). Therefore, the machine learning algorithms were used in the present study to provide numerical probability value for CH after sympathicotomy. Machine learning has been successfully used to make accurate decisions and predictions using diverse and large amount of data (16,20). Machine learning algorithms create a model based on sample data to make decisions or predictions (16,20). As technologies develop, the use of machine learning in medical fields can improve the diagnostic accuracy and personalized treatment and provide new solutions to unsolved issues (16,20).
Similar to our previous study, the association of autonomic nervous system traits with hyperhidrosis type and CH after sympathicotomy based on HRV measurements was investigated in the present study (2). Novel parameters are needed for machine learning algorithms and HRV parameters are useful for analysis when using machine learning algorithms. In the present study, models using machine learning algorithms were used to classify hyperhidrosis type and predict CH after sympathicotomy. HRV parameters and clinical data considered associated with primary hyperhidrosis and CH after sympathicotomy were included as variables in the machine learning algorithms. In the present study, the model performance for type classification were 0.972 AUC, 0.961 accuracy, 0.961 F1 score, 0.961 precision, and 0.961 recall. In addition, the model performance for CH prediction were 0.914 AUC, 0.852 accuracy, 0.853 F1 score, 0.856 precision, and 0.852 recall. The classification model for hyperhidrosis type and the prediction model for CH after sympathicotomy yielded excellent results, which could be useful in clinical practice. To the best of our knowledge, this is the first study on CH after sympathicotomy in which machine learning algorithms were used. Further studies are needed to validate the findings. The approach of integrating machine learning models and readily available clinical data can be used to develop other models in the field of primary hyperhidrosis.
The clinical relevance of the present study has several limitations. First, the present study was retrospectively performed in a single center. Second, the HRV test is influenced by various physiologic factors, diseases, and cardiac conditions, that may cause statistical errors (10,15). Third, the number of subjects in the dataset was relatively small. Strict eligible criteria were used to obtain data of good quality. Hyperhidrosis type cannot be strictly defined into two types (craniofacial vs. palmar) because it is diverse and may involve only one site or a few sites simultaneously. However, the heterogeneity in hyperhidrosis type may reflect the real-world situation, and a trained machine learning model with this data may be more appropriate for clinical practice. Sympathicotomy represents a small portion of the thoracic surgery field and knowledge regarding hyperhidrosis is valuable because the complications of a benign condition can have an intractable effect on patients.
Conclusions
The results of the present study showed the machine learning algorithms using HRV parameters and basic clinical data can classify types and predict CH after sympathicotomy for primary hyperhidrosis with considerable accuracy. This machine learning algorithm may be convenient and useful for selecting treatment modality and improvement of sympathicotomy outcomes. Further large-scale studies are needed to validate the findings and provide management guidelines for primary hyperhidrosis. In addition, an approach integrating machine learning models and readily available clinical data can be used to develop other models in the thoracic surgery field.
Acknowledgments
Funding: None.
Footnote
Reporting Checklist: The authors have completed the STROBE reporting checklist. Available at https://jtd.amegroups.com/article/view/10.21037/jtd-23-471/rc
Data Sharing Statement: Available at https://jtd.amegroups.com/article/view/10.21037/jtd-23-471/dss
Peer Review File: Available at https://jtd.amegroups.com/article/view/10.21037/jtd-23-471/prf
Conflicts of Interest: All authors have completed the ICMJE uniform disclosure form (available at https://jtd.amegroups.com/article/view/10.21037/jtd-23-471/coif). The authors have no conflicts of interest to declare.
Ethical Statement: The authors are accountable for all aspects of the work in ensuring that questions related to the accuracy or integrity of any part of the work are appropriately investigated and resolved. The study was conducted in accordance with the Declaration of Helsinki (as revised in 2013). Uijeongbu Saint Mary’s Hospital Ethics Committee reviewed and approved the present study (No. UC23RISI0022). The need for informed consent was waived because the present study was retrospective without disclosure of patient information.
Open Access Statement: This is an Open Access article distributed in accordance with the Creative Commons Attribution-NonCommercial-NoDerivs 4.0 International License (CC BY-NC-ND 4.0), which permits the non-commercial replication and distribution of the article with the strict proviso that no changes or edits are made and the original work is properly cited (including links to both the formal publication through the relevant DOI and the license). See: https://creativecommons.org/licenses/by-nc-nd/4.0/.
References
- Fibla Alfara JJ, Molins López-Rodó L, Hernández Ferrández J, et al. Effectiveness of bilateral clipping of the thoracic sympathetic chain for the treatment of severe palmar and/or axillary hyperhidrosis and facial flushing. Cir Esp 2019;97:196-202. (Engl Ed). [Crossref] [PubMed]
- Jeong SC, Kim JJ, Kim YH, et al. Heart rate variability as a potential diagnostic tool to predict compensatory hyperhidrosis after sympathectomy in patients with primary focal hyperhidrosis. J Thorac Dis 2020;12:6789-96. [Crossref] [PubMed]
- Raveglia F, Orlandi R, Guttadauro A, et al. How to Prevent, Reduce, and Treat Severe Post Sympathetic Chain Compensatory Hyperhidrosis: 2021 State of the Art. Front Surg 2021;8:814916. [Crossref] [PubMed]
- Yazbek G, Ishy A, Alexandrino da Silva MF, et al. Evaluation of compensatory hyperhidrosis after sympathectomy: The use of an objective method. Ann Vasc Surg 2021;77:25-30. [Crossref] [PubMed]
- Kara M, Kose S, Cayirci CE, et al. Can we predict the compensatory hyperhidrosis following a thoracic sympathectomy? Indian J Thorac Cardiovasc Surg 2019;35:190-5. [Crossref] [PubMed]
- Dogru MV, Sezen CB, Girgin O, et al. Is there any relationship between quality of life and the level of sympathectomy in primary palmar hyperhidrosis? Single-center experience. Gen Thorac Cardiovasc Surg 2020;68:273-9. [Crossref] [PubMed]
- Vasconcelos CFM, Aguiar WS, Cordeiro GG, et al. Modified R5-R8 Thoracic Sympathectomy for Severe Compensatory Hyperhidrosis. Ann Thorac Surg 2021;111:e57-9. [Crossref] [PubMed]
- Niwa ASM, Gregório ML, Leão LEV, et al. Heart Rate Variability Assessment and Its Application for Autonomic Function Evaluation in Patients with Hyperhidrosis. Eur Neurol 2020;83:293-300. [Crossref] [PubMed]
- Fiorelli A, Messina G, Chiodini P, et al. Cardiac Autonomic Changes After Thoracic Sympathectomy: A Prospective, Randomized Study. Ann Thorac Surg 2017;103:216-24. [Crossref] [PubMed]
- Cheshire WP, Freeman R, Gibbons CH, et al. Electrodiagnostic assessment of the autonomic nervous system: A consensus statement endorsed by the American Autonomic Society, American Academy of Neurology, and the International Federation of Clinical Neurophysiology. Clin Neurophysiol 2021;132:666-82. [Crossref] [PubMed]
- Apelt-Glitz K, Alken FA, Jungen C, et al. Respiratory and heart rate dynamics during peripheral chemoreceptor deactivation compared to targeted sympathetic and sympathetic/parasympathetic (co-)activation. Auton Neurosci 2022;241:103009. [Crossref] [PubMed]
- Lee ACH, Tung R, Ferguson MK. Thoracoscopic sympathectomy decreases disease burden in patients with medically refractory ventricular arrhythmias. Interact Cardiovasc Thorac Surg 2022;34:783-90. [Crossref] [PubMed]
- Moon DH, Kang DY, Lee HS, et al. To avoid compensatory hyperhidrosis after sympathetic surgery for craniofacial hyperhidrosis. J Thorac Dis 2020;12:2529-35. [Crossref] [PubMed]
- Han JW, Kim JJ, Kim YH, et al. New sympathicotomy for prevention of severe compensatory hyperhidrosis in patients with primary hyperhidrosis. J Thorac Dis 2020;12:765-72. [Crossref] [PubMed]
- Shaffer F, Ginsberg JP. An Overview of Heart Rate Variability Metrics and Norms. Front Public Health 2017;5:258. [Crossref] [PubMed]
- Demsar J, Curk T, Erjavec A, et al. Orange: Data Mining Toolbox in Python. Journal of Machine Learning Research 2013;14:2349-53.
- Castiglioni P, Merati G, Parati G, et al. Sample, Fuzzy and Distribution Entropies of Heart Rate Variability: What Do They Tell Us on Cardiovascular Complexity? Entropy (Basel) 2023;25:281. [Crossref] [PubMed]
- Woo W, Kim BJ, Kang DY, et al. Patient experience and prognostic factors of compensatory hyperhidrosis and recurrence after endoscopic thoracic sympathicotomy. Surg Endosc 2022;36:8340-8. [Crossref] [PubMed]
- Lee J, Jeong JY, Suh JH, et al. Thoracoscopic sympathetic block to predict compensatory hyperhidrosis in primary hyperhidrosis. J Thorac Dis 2021;13:3509-17. [Crossref] [PubMed]
- Beck KS, Gil B, Na SJ, et al. DeepCUBIT: Predicting Lymphovascular Invasion or Pathological Lymph Node Involvement of Clinical T1 Stage Non-Small Cell Lung Cancer on Chest CT Scan Using Deep Cubical Nodule Transfer Learning Algorithm. Front Oncol 2021;11:661244. [Crossref] [PubMed]