Screening practices for obstructive sleep apnea in healthy community people: a Chinese community-based study
Highlight box
Key findings
• The use of objective type IV sleep detection equipment to screen a large sample population in the community in a short time is feasible, and the prevalence of high-risk obstructive sleep apnea (OSA) screened was lower than that screened by Stop-Bang.
What is known and what is new?
• The prevalence of OSA is high and awareness is low in the general population. There have been studies using questionnaires to screen for high-risk OSA in the community.
• In this study, we used an objective type IV sleep detection equipment to screen a large sample of community population for high-risk OSA in a short period of time and confirmed its feasibility.
What is the implication, and what should change now?
• It is feasible and necessary to screen the community for OSA at an early stage using objective type IV wearable intelligent sleep monitor.
Introduction
Obstructive sleep apnea (OSA) is a common disorder in which the upper respiratory tract collapses repeatedly during sleep (1), leading to decreased levels of oxygenated hemoglobin and sleep fragmentation. In the general adult population, OSA occurs in 14% of men and 5% of women (2) and has been reported to affect more than 936 million people worldwide, with China having the largest number of cases at 176 million (3). Data has shown that most patients with OSA are undiagnosed and untreated even in developed countries. In developing countries, OSA is poorly understood, and methods of diagnosis and treatment are often not applicable in low-resource settings (4). Owing to the multifactorial nature and social consequences of OSA, the disease is associated with a high economic and social burden. The 2015 report on the Economic Burden of OSA in the US Healthcare System estimated that a poor understanding of the severity of OSA could result in economic losses of $12.4 billion (5). Currently, data on OSA-related medical burden is lacking in China; however, the health damage and economic loss caused by OSA cannot be ignored and must be paid more attention to. Therefore, early detection and treatment of OSA in the general population are important, and early screening for OSA in the community is necessary.
The 1993 Wisconsin Sleep Cohort Study used polysomnography (PSG) to investigate the prevalence and epidemiology of OSA in the adult population (6). PSG is the gold standard for the diagnosis of OSA; however, it is expensive and needs to be performed in a skilled sleep laboratory, resulting in long waiting times and discomfort for patients (7). Subjective screening questionnaires, such as the Epworth Sleepiness Scale (ESS) and the Berlin Questionnaire (BQ), which are easy to use, have low sensitivity and specificity (ESS: 46% and 60%, respectively; BQ: 73% and 44%, respectively), making them unacceptable screening options (8). A type IV wearable intelligent sleep monitor (WISM) may be a strategy to increase OSA screening, particularly in communities with low access to healthcare. Our research team conducted a comparative study between the type IV WISM and PSG at Guangdong Provincial People’s Hospital and found that the sensitivity and specificity of the devices were high (93% and 77%, respectively, when the respiratory disorder index was ≥5; and 92%, and 89%, respectively, when the respiratory disturbance index was ≥15) (9).
This study aimed to screen for OSA in a healthy community population, explore the feasibility of type IV sleep monitoring in screening patients with OSA in a large sample of community residents in China, provide a basis for OSA screening in a healthy population, estimate the prevalence of high-risk OSA, and evaluate its related risk factors. We present this article in accordance with the STROBE reporting checklist (available at https://jtd.amegroups.com/article/view/10.21037/jtd-22-1538/rc).
Methods
Study design
This prospective, multi-point, cross-sectional study was performed on a Chinese population. Participants were recruited from the general population of the community between April 9 and May 18, 2021. A detailed description of participant recruitment was provided in a recently published study by He et al. (10). The study was conducted in accordance with the Declaration of Helsinki (as revised in 2013). This study was approved by the Ethics Committee of Guangdong Provincial People’s Hospital [approval No. GDREC2020221H (R1)]. All the participants provided written informed consent.
Study participants
This study used a multistage stratified cluster sampling to select a representative population. Villages were then selected from counties and communities in urban streets or districts; finally, with the support of the local government, residents of selected villages and communities were invited to participate in the survey. This study was designed and conducted based on a previously published study titled Data Resource Overview: China National Health Survey (11). Inclusion criteria were as follows: (I) community residents aged >18 years who had resided at the study site for >6 months; (II) people who volunteered to participate in the study and signed informed consent; and (III) people who could cooperate with investigators to complete all assessments, including structured questionnaires, sleep monitoring, physical examination, and fasting blood withdrawal. Participants with cognitive impairment, mobility problems, or severe organic disease were excluded.
Questionnaire
The standard questionnaire collected demographic data such as age, sex, educational level, marital status, lifestyle including smoking history, drinking history, and physical exercise, and disease histories such as hypertension, coronary heart disease, diabetes, and dyslipidemia. Hypertension was defined as the use of blood pressure medications and/or systolic or diastolic blood pressure ≥140/90 mmHg (12). Diabetes was defined as fasting blood glucose ≥7 mmol/L or the use of diabetic medications (oral hypoglycemic agents and/or insulin) (13).
The questionnaire was completed by a specialized doctor in the research group through face-to-face interviews. Field supervisors conducted quality control checks to ensure the completeness and authenticity of each questionnaire. The questionnaires were scanned and converted into an electronic format by special software at the end of each day, and the input questionnaire data were checked to verify the accuracy of each input. Participants with snoring and other sleep problems were assessed using a special sleep questionnaire. The Stop-Bang questionnaire was used to evaluate patients with high-risk OSA. A score of ≥3 was considered a high-risk OSA and <3 was considered a low-risk OSA. The Pittsburgh Sleep Quality Scale was used to evaluate sleep quality. A score of ≤5 was considered good sleep quality, and >5 was considered poor sleep quality. The Insomnia Severity Scale was used to evaluate insomnia. A score of ≤7 was considered as no insomnia >7 was considered as insomnia. Daytime sleepiness was assessed using the ESS. A score of ≥9 was considered daytime sleepiness, and <9 was considered no daytime sleepiness. The fatigue severity scale was used to evaluate the fatigue degree.
Type IV WISM
We used a type IV WISM (Chengdu Yunweikang Healthcare Company, China) to screen the community population for OSA. The device uses a photoelectric reflector sensor to measure pulse oxygen saturation based on the absorption characteristics of hemoglobin under infrared and red light, and the raw data are stored in a specific database. Based on physical activity signals, artifact identification, and built-in automated algorithms, the duration of effective monitoring and the number of oxygen saturation decreases (≥3% or ≥4%) were recorded and analyzed, and reports were generated automatically. The recommended areas for monitoring are the palmar muscles of the thenar, and skin areas with thick hair, scars, spots, and other areas that should be avoided. The device can be continuously monitored overnight for 7 h and recycled after disinfection and charging. The main monitoring indicators included the oxygen desaturation index (ODI), mean oxygen saturation, lowest oxygen saturation, and percentage of sleep time with oxygen saturation <90%. The sleep-monitoring device was compared and verified with PSG at the Sleep Center of Guangdong Provincial People’s Hospital. A total of 196 participants were enrolled in the study who underwent both overnight PSG and WISM monitoring to assess the sensitivity and specificity of WISM for the diagnosis of OSA. According to the PSG results, 39 patients (20%) had mild OSA, and 135 (69%) had moderate-to-severe OSA. The ODI represents the number of oxygen saturation decreases by ≥4% divided by the effective monitoring time in hours. When the ODI reached 7.0 times/h, the sensitivity, specificity, and accuracy of OSA with AHI ≥5 times/h were 86%, 91%, and 95%, respectively. Therefore, in this study, ODI ≥7 times/h indicated the presence of OSA.
Anthropometric measurements
Details of height, weight, and blood pressure measurements are described in a previous study (11). Body mass index (BMI) was calculated as weight divided by height squared. A BMI ≥25 kg/m2 was defined as obesity. Trained technicians performed two neck and waist measurements. The neck circumference was measured at the upper edge of the cricothyroid cartilage, and the waist circumference was measured 1 cm above the umbilicus.
Blood parameters
Blood samples were collected from each participant after an 8-h overnight fast to assess lipid profile parameters [total cholesterol, triglycerides, low-density lipoprotein cholesterol, and high-density lipoprotein cholesterol (HDL-C)], fasting blood glucose, and high-sensitivity C-reactive protein. Details regarding the collection, transportation, handling, storage, and analysis of blood samples are described in a previous study (11). Home sleep apnea test (HSAT) comparison verification
Among all the people screened for the type IV equipment, 305 participants from Chenghai and Jinping Districts of Shantou City were selected and subjected to a type III HSAT to compare the prevalence and consistency of OSA diagnosed using type IV equipment and HSAT sleep monitoring devices, to further verify the accuracy of type IV equipment screening for OSA in healthy community populations.
Statistical analyses
Statistical analysis was performed using SPSS (version 23.0; IBM Corp., Armonk, NY, USA). Normality test was conducted on continuous variables, and the level of α=0.10 was considered that the population obeyed normal distribution. Descriptive statistics were used, with continuous variables expressed as mean ± standard deviation, and categorical variables as frequency and percentage. Student’s t-test was used for continuous variables with normal distribution, and one-way analysis of variance (ANOVA) was used for categorical variables. Logistic regression analysis was used to identify significant independent risk factors for OSA in high-risk groups. The statistical test was two-sided, and statistical significance was set at P<0.05.
Pearson’s correlation was used to analyze the correlation between ODI measured using type IV equipment and AHI measured using HSAT. The consistency of the type IV equipment and HSAT results was determined using the Bland-Altman method.
Results
A total of 5,838 participants were recruited in 2 months, and 3,650 met the inclusion criteria of the study. Their mean age was 53.81±12.71 years (Table 1). The prevalence of OSA in the community screening was 30.7% (1,119/3,650), and that of high-risk OSA was 42.8% (1,563/3,650), according to the Stop-Bang questionnaire.
Table 1
Characteristics | Mean ± SD or n (%) |
---|---|
Region | |
Coastal | 2,279 (62.4) |
Mountain | 1,371 (37.6) |
Gender | |
Male | 1,098 (30.1) |
Female | 2,552 (69.9) |
Age (years) | 53.81±12.71 |
BMI (kg/m2) | 23.60±3.33 |
Neck circumference (cm) | 34.01±3.66 |
Waist circumference (cm) | 82.01±10.21 |
Marital status | |
Never married/divorced/widowed | 459 (12.6) |
Married | 3,191 (87.4) |
Educational level | |
Primary school and below | 831 (22.8) |
Middle school | 902 (24.7) |
High school | 1,042 (28.5) |
College degree and above | 875 (24.0) |
Smoking status | |
Never-smokers/former smokers | 3,168 (86.8) |
Current smokers | 482 (13.2) |
Alcohol use | |
Never-drinker/former drinkers | 3,114 (85.3) |
Current drinkers | 536 (14.7) |
Drinking tea | |
Never-drinkers/former drinkers | 894 (24.5) |
Current drinkers | 2,756 (75.5) |
Exercise | |
5–7 d/week | 1,917 (52.5) |
3–4 d/week | 391 (10.7) |
1–2 d/week | 413 (11.3) |
≤3 d/month | 328 (9.0) |
Never | 601 (16.5) |
Hypertension | |
Yes | 675 (18.5) |
No/unknown | 2,975 (81.5) |
Diabetes | |
Yes | 319 (8.7) |
No/unknown | 3,331 (91.3) |
Dyslipidemia | |
Yes | 1,678 (46.0) |
No | 1,972 (54.0) |
Head and neck neoplasm | |
Yes | 81 (2.2) |
No | 3,569 (97.8) |
LDL-C (mmol/L) | 3.24±0.91 |
HDL-C (mmol/L) | 1.41±0.39 |
Total cholesterol (mmol/L) | 5.61±1.12 |
Triglycerides (mmol/L) | 1.53±1.19 |
Creatinine (mmol/L) | 70.14±20.44 |
Lipoprotein (mmol/L) | 108.20±154.17 |
Uric acid (μmol/L) | 362.73±93.38 |
SD, standard deviation; BMI, body mass index; LDL-C, low-density lipoprotein cholesterol; HDL-C, high-density lipoprotein cholesterol.
Feasibility of screening pathways
The screening roadmap is shown in Figure 1. Five communities in Shantou and Meizhou were selected. The screening time in each community was 5–6 days, and 200 people were screened daily. The community residents who had made the appointment in advance first underwent face-to-face demographic information collection and health questionnaire survey and then completed a series of physical examinations, such as height, weight, blood pressure, body fat measurement, biochemical examination, bone mineral density, and lung function. Residents who were willing to complete the sleep questionnaire were asked face-to-face by the researchers. Residents with high-risk OSA symptoms, such as snoring and daytime sleepiness, were advised to undergo overnight type IV equipment monitoring and return it to the designated collection point the next day.
Screening for OSA with type IV equipment in the five communities is shown in Table 2. OSA screening with type IV equipment in the healthy community population was feasible because 99.79% of devices were returned. The total recovery rate of WISM was 99.37%, and the equipment recovery rates of Chenghai District in Shantou City, Jinping District in Shantou City, Nanao County in Shantou City, Meijiang District in Meizhou City, and Jiaoling County in Meizhou City were 100%, 99.63%, 99.79%, 98.34%, and 99.09%, respectively. The total screening success rate was 89.63%, and the screening success rates of Chenghai District, Shantou Jinping District, Shantou Nanao County, Meijiang District of Meizhou City, and Jiaoling County of Meizhou City were 93.96%, 87.83%, 88.94%, 90.12%, and 86.60%, respectively.
Table 2
Site | Issue | Refuse to check | Actual distribution | Recycle | Valid data | Recovery rate (%) | Screening success rate (%) |
---|---|---|---|---|---|---|---|
Chenghai District | 930 | 19 | 911 | 911 | 856 | 100 | 93.96 |
Jinping District | 838 | 38 | 800 | 797 | 700 | 99.63 | 87.83 |
Nanao County | 944 | 11 | 933 | 931 | 828 | 99.79 | 88.94 |
Meijiang District | 917 | 11 | 906 | 891 | 803 | 98.34 | 90.12 |
Jiaoling County | 809 | 41 | 768 | 761 | 659 | 99.09 | 86.60 |
Total | 4,438 | 120 | 4,318 | 4,291 | 3,846 | 99.37 | 89.63 |
OSA, obstructive sleep apnoea.
A total of 4,438 Type IV equipment were distributed in the five survey communities, and 2.70% (n=120) of the participants opted out of the inspection in the field. Of the remaining 4,318 participants, 0.21% (n=9) lost the device and 0.42% (n=18) failed to retrieve the data due to the device. Of the 4,291 participants enrolled, 3,846 (89.63%) reported valid data, 238 (5.55%) reported insufficient valid data due to a monitoring time of <7 h, and 207 (4.82%) did not wear the device on the night of monitoring. Demographic characteristics and risk factors of the two groups.
According to an ODI of ≥7, patients were divided into OSA and non-OSA groups. Table 3 summarizes the clinical characteristics of the study participants in the OSA and non-OSA groups. The prevalence of high-risk OSA was 42.8% (1,563/3,650) using the Stop-Bang questionnaire and 30.7% (1,119/3,650) using type IV equipment. The prevalence of OSA using the Stop-Bang questionnaire was higher than that according to type IV equipment (P<0.01) (Table 4). The male ratio in OSA group was 44.4% (497/1,119) significantly higher than that in non-OSA group was 23.7% (601/2,531) (P<0.01). There was no significant difference in the ratio of residents living in coastal areas and mountain areas between OSA group and non-OSA group (P=0.473).
Table 3
Characteristics | OSA (N=1,119, 30.7%) | Non-OSA (N=2,531, 69.3%) | P value |
---|---|---|---|
Region | 0.473 | ||
Coastal | 689 (61.6) | 1,590 (62.8) | |
Mountain | 430 (38.4) | 941 (37.2) | |
Gender | <0.01 | ||
Male | 497 (44.4) | 601 (23.7) | |
Female | 622 (55.6) | 1,930 (76.3) | |
Age (years), mean ± SD | 56.55±13.00 | 52.60±12.39 | <0.01 |
BMI (kg/m2), mean ± SD | 24.84±3.52 | 23.06±3.08 | <0.01 |
Neck circumference (cm), mean ± SD | 35.46±3.74 | 33.37±3.43 | <0.01 |
Waist circumference (cm), mean ± SD | 86.27±10.49 | 80.13±9.50 | <0.01 |
Marital status | 0.169 | ||
Never married/divorced/widowed | 128 (11.4) | 331 (13.1) | |
Married | 991 (88.6) | 2,200 (86.9) | |
Educational level | 0.764 | ||
Primary school and below | 263 (23.5) | 568 (22.4) | |
Middle school | 265 (23.7) | 637 (25.2) | |
High school | 319 (28.5) | 723 (28.6) | |
College degree and above | 272 (24.3) | 603 (23.8) | |
Smoking status | <0.01 | ||
Never-smokers/former smokers | 932 (83.3) | 2,236 (88.3) | |
Current smokers | 187 (16.7) | 295 (11.7) | |
Alcohol use | <0.01 | ||
Never-drinker/former drinkers | 895 (80.0) | 2,219 (87.7) | |
Current drinkers | 224 (20.0) | 312 (12.3) | |
Drinking tea | <0.01 | ||
Never-drinkers/former drinkers | 239 (21.4) | 655 (25.9) | |
Current drinkers | 880 (78.6) | 1,876 (74.1) | |
Exercise | 0.002 | ||
5–7 d/week | 640 (57.2) | 1,277 (50.5) | |
3–4 d/week | 108 (9.7) | 283 (11.2) | |
1–2 d/week | 115 (10.3) | 298 (11.8) | |
≤3 d/month | 79 (7.1) | 249 (9.8) | |
Never | 177 (15.8) | 424 (16.8) | |
Hypertension | <0.01 | ||
Yes | 294 (26.3) | 381 (15.1) | |
No/unknown | 825 (73.7) | 2,150 (84.9) | |
Diabetes | 0.097 | ||
Yes | 109 (9.7) | 210 (8.3) | |
No/unknown | 1,010 (90.3) | 2,321 (91.7) | |
Dyslipidemia | <0.01 | ||
Yes | 577 (51.6) | 1,101 (43.5) | |
No | 542 (48.4) | 1,430 (56.5) | |
Head and neck neoplasm | 0.209 | ||
Yes | 21 (1.9) | 60 (2.4) | |
No | 1,098 (98.1) | 2,471 (97.6) | |
LDL-C (mmol/L), mean ± SD | 3.27±0.88 | 3.23±0.92 | 0.185 |
HDL-C (mmol/L), mean ± SD | 1.33±0.38 | 1.45±0.39 | <0.01 |
Total cholesterol (mmol/L), mean ± SD | 5.61±1.08 | 5.60±1.14 | 0.803 |
Triglycerides (mmol/L), mean ± SD | 1.67±1.27 | 1.46±1.15 | <0.01 |
Creatinine (mmol/L), mean ± SD | 75.31±23.41 | 67.85±18.53 | <0.01 |
Lipoprotein (mmol/L), mean ± SD | 108.25±151.56 | 108.18±155.35 | 0.99 |
Uric acid (μmol/L), mean ± SD | 388.11±97.83 | 351.51±89.09 | <0.01 |
Blood oxygen parameters | |||
Lowest SpO2 during sleep (%), mean ± SD | 82.22±5.34 | 89.34±3.56 | <0.01 |
Mean SpO2 during sleep (%), mean ± SD | 95.52±2.03 | 96.96±1.07 | <0.01 |
Maximum oxygen reduction time (s), mean ± SD | 45.23±11.30 | 34.83±9.58 | <0.01 |
OSA, obstructive sleep apnoea; SD, standard deviation; BMI, body mass index; LDL-C, low-density lipoprotein cholesterol; HDL-C, high-density lipoprotein cholesterol.
Table 4
Group | Low-risk OSA (Stop-Bang <3) | High-risk OSA (Stop-Bang ≥3) | Total | P |
---|---|---|---|---|
ODI <7 | 1,658 | 873 | 2,531 (69.3%) | – |
ODI ≥7 | 429 | 690 | 1,119 (30.7%) | – |
Total | 2,087 (57.2%) | 1,563 (42.8%) | 3,650 | <0.01 |
OSA, obstructive sleep apnoea; ODI, oxygen desaturation index.
There were 1,119 patients (30.7%) in the OSA group and 2,531 patients (69.3%) in the non-OSA group. Men accounted for 30.1% (n=1,098), whereas women accounted for 69.9% (n=2,552) because more women in the community were willing to participate. Participants in the OSA group were older and had a higher BMI, neck circumference, and waist circumference than those in the non-OSA group (P<0.01). There were no significant differences in marital status or education between the two groups. Among the survey population, 3,168 (86.8%) had never smoked or quit smoking and 482 (13.2%) were current smokers. The proportion of smokers in the OSA group was significantly higher than that in the non-OSA group (P<0.01). In the survey population, 3,114 participants (85.3%) never drank alcohol or abstained from alcohol, and 536 (14.7%) currently drank alcohol. The proportion of alcohol drinkers in the OSA group was significantly higher than that in the non-OSA group (P<0.01). In addition, 894 (24.5%) participants never drank tea, and 2,756 (75.5%) drank tea. The proportion of tea drinkers in the OSA group was significantly higher than that in the non-OSA group (P<0.01). Among the participants, 52.5% (n=1,917) exercised 5–7 days per week, 10.7% (n=391) exercised 3–4 days per week, 11.3% (n=413) exercised 1–2 days per week, and 9.0% (n=328) exercised no more than 3 days per month. Approximately 16.5% (n=601) had never exercised. Among the participants, 18.5% (n=675) had hypertension, and 81.5% (n=2,975) had no hypertension or were unclear. The proportion of patients with hypertension in the OSA group was significantly higher than that in the non-OSA group (P<0.01). In the community survey, 46.0% of participants (n=1,678) had dyslipidemia and 54.0% (n=1,972) did not. The proportion of patients with dyslipidemia in the OSA group was significantly higher than that in the non-OSA group (P<0.01). Biochemical examination showed that HDL-C levels in the OSA group were significantly lower than those in the non-OSA group (P<0.01), whereas triglyceride, creatinine, and uric acid levels in the OSA group were significantly higher than those in the non-OSA group (P<0.01).
Risk factor regression analysis shows that in the correction of the Logistic regression model, male sex, age, increasing BMI, neck circumference, waist circumference, smoking, drinking, drinking tea, exercising 5–7 days a week, hypertension, dyslipidemia, low HDL-C, and high triglycerides, creatinine, and uric acid levels, were independently associated with an increased risk of OSA (Table 5). After adjusting for confounding factors, male sex, increased age, increased BMI, increased neck circumference, smoking, and hypertension were significantly associated with the risk of OSA. The risk of OSA in women was 0.496 times that of men. For each 1-year increase in age, the risk of OSA increased 1.015 times. For every 1 unit increase in BMI, the risk of OSA increased 1.115 times. For every 1 cm increase in neck circumference, the risk of OSA increased 1.048 times. Smokers had a 0.642 times higher risk of OSA than non-smokers, and patients with hypertension had a 1.268 times higher risk of OSA.
Table 5
Risk factors | Unadjusted | Adjusted | |||
---|---|---|---|---|---|
OR (95% CI) | P value | OR (95% CI) | P value | ||
Gender | |||||
Male | |||||
Female | 0.390 (0.336–0.452) | <0.001 | 0.496 (0.380–0.646) | <0.001 | |
Age | 1.026 (1.020–1.032) | <0.001 | 1.015 (1.007–1.022) | <0.001 | |
BMI | 1.182 (1.155–1.209) | <0.001 | 1.115 (1.072–1.160) | <0.001 | |
Neck circumference | 1.198 (1.171–1.225) | <0.001 | 1.048 (1.016–1.081) | 0.003 | |
Waist circumference | 1.068 (1.059–1.076) | <0.001 | 1.009 (0.995–1.024) | 0.187 | |
Smoking status | |||||
Never-smokers/former smokers | |||||
Current smokers | 1.521 (1.247–1.855) | <0.001 | 0.642 (0.497–0.830) | 0.001 | |
Alcohol use | |||||
Never-drinker/former drinkers | |||||
Current drinkers | 1.780 (1.474–2.149) | <0.01 | 1.140 (0.907–1.433) | 0.262 | |
Drinking tea | |||||
Never-drinkers/former drinkers | |||||
Current drinkers | 1.286 (1.086–1.521) | 0.003 | 0.912 (0.757–1.099) | 0.335 | |
Exercise | |||||
5–7 d/week | |||||
3–4 d/week | 0.761 (0.598–0.969) | 0.027 | 0.954 (0.736–1.237) | 0.722 | |
1–2 d/week | 0.770 (0.609–0.974) | 0.029 | 0.990 (0.765–1.281) | 0.941 | |
≤3 d/month | 0.633 (0.483–0.830) | 0.001 | 0.814 (0.607–1.092) | 0.170 | |
Never | 0.833 (0.682–1.017) | 0.072 | 1.038 (0.837–1.287) | 0.735 | |
Hypertension | |||||
Yes | 2.011 (1.693–2.388) | <0.001 | 1.268 (1.044–1.539) | 0.017 | |
No/unknown | |||||
Dyslipidemia | |||||
Yes | 1.383 (1.201–1.592) | <0.001 | 0.941 (0.795–1.114) | 0.482 | |
No | |||||
HDL-C | 0.440 (0.363–0.533) | <0.001 | 0.974 (0.760–1.248) | 0.833 | |
Triglycerides | 1.147 (1.083–1.216) | <0.001 | 1.009 (0.936–1.088) | 0814 | |
Creatinine | 1.019 (1.015–1.023) | <0.001 | 1.001 (0.997–1.006) | 0.6 | |
Uric acid | 1.004 (1.003–1.005) | <0.001 | 1.001 (1.000–1.002) | 0.082 |
OSA, obstructive sleep apnoea; OR, odds ratio; 95% CI, 95% confidence interval; BMI, body mass index; HDL-C, high-density lipoprotein cholesterol.
Characteristics of sleep
Table 6 shows the sleep characteristics of the population in different regions, and the OSA and non-OSA groups. Approximately 47.6% (1,736/3,650) of the community had good sleep quality and 52.4% (1,914/3,650) had poor sleep quality. Of the coastal community population, 50.4% (1,149/2,279) had good sleep quality and 49.6% (1,130/2,279) had poor sleep quality. Moreover, 42.8% (587/1,371) had good sleep quality in mountainous areas, and 57.2% (784/1,371) had poor sleep quality. The sleep quality in coastal areas was better than that in mountainous areas (P<0.01); 48.3% (541/1,119) of OSA patients had good sleep quality, 51.7% (578/1,119) had poor sleep quality, 47.2% (1,195/2,531) of non-OSA patients had good sleep quality, and 52.8% (1,336/2,531) had poor sleep quality. There was no difference in sleep quality between the two groups (P=0.53). Daytime sleepiness occurred in 6.6% (240/3,650) of the community population, 6.7% (152/2,279) of coastal communities, and 6.4% (88/1,371) of mountainous areas, with no difference in the proportion of daytime sleepiness between the two regions (P=0.767); daytime sleepiness was 7.2% (81/1,119) in patients with OSA and 6.3% (159/2,531) in patients without OSA. There was no difference in daytime sleepiness between the two groups (P=0.28). Approximately 36.0% (1,313/3,650) of the five community populations had insomnia, 31.2% (712/2,279) of the coastal community population, and 43.8% (601/1,371) of the mountainous community population had insomnia, which was significantly higher in the mountainous population than in the coastal population (P<0.01). There was no significant difference in population fatigue scores between the two communities (P=0.131).
Table 6
Characteristics | All subjects (N=3,650) | Region | Presence of OSA | |||||
---|---|---|---|---|---|---|---|---|
Coastal (N=2,279) | Mountain (N=1,371) | P value | OSA (N=1,119) | Non-OSA (N=2,531) | P value | |||
PSQI | <0.01 | 0.53 | ||||||
Good | 1,736 (47.6) | 1,149 (50.4) | 587 (42.8) | 541 (48.3) | 1,195 (47.2) | |||
Poor | 1,914 (52.4) | 1,130 (49.6) | 784 (57.2) | 578 (51.7) | 1,336 (52.8) | |||
ESS | 0.767 | 0.28 | ||||||
Daytime sleepiness | 240 (6.6) | 152 (6.7) | 88 (6.4) | 81 (7.2) | 159 (6.3) | |||
No daytime sleepiness | 3,410 (93.4) | 2,127 (93.3) | 1,283 (93.6) | 1,038 (92.8) | 2,372 (93.7) | |||
ISI | <0.01 | 0.02 | ||||||
Insomnia | 1,313 (36.0) | 712 (31.2) | 601 (43.8) | 371 (33.2) | 942 (37.2) | |||
No insomnia | 2,337 (64.0) | 1,567 (68.8) | 770 (56.2) | 748 (66.8) | 1,589 (62.8) | |||
FSS score | 27.48±13.70 | 27.76±13.52 | 27.03±14.00 | 0.131 | 27.32±13.69 | 27.52±13.57 | 0.7 |
Data are shown as n (%) or mean ± standard deviation. OSA, obstructive sleep apnoea; PSQI, Pittsburgh Sleep Quality Index; ESS, Epworth Sleepiness Scale; ISI, Insomnia Severity Index; FSS, Fatigue Severity Scale.
HSAT validation
Table 7 shows that among 305 community participants who underwent HSAT validation, 57.7% (176/305) were diagnosed with moderate-to-severe OSA using the type IV equipment ODI of ≥5. Based on the HSAT AHI of ≥5, 75.4% (230/305) of the participants were diagnosed with moderate-to-severe OSA.
Table 7
Group | ODI <5 | ODI ≥5 | Total |
---|---|---|---|
AHI <5 | 24 | 51 | 75 (24.6%) |
AHI ≥5 | 54 | 176 | 230 (75.4%) |
Total | 78 (25.6%) | 227 (74.4%) | 305 |
HSAT, home sleep apnea test; ODI, oxygen desaturation index; AHI, apnea-hypopnea index.
Among the 305 community participants who underwent HSAT validation, the ODI of type IV equipment was strongly correlated with the AHI of HSAT (R2=0.504, P<0.001) (Figure 2). The Bland-Altman curves of the ODI of type IV equipment and the AHI of HSAT are shown in Figure 3. Approximately 93% (284/305) of the scatterers were within 95% limits of agreement (LOA).
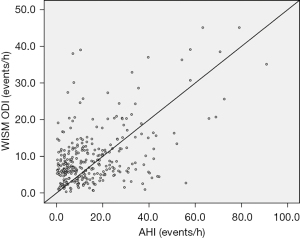
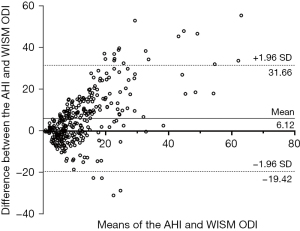
Discussion
Our study successfully applied a type IV WISM to screen OSA in a healthy community population and verified the feasibility and accuracy of this screening method in a large sample population. This OSA screening method has a high equipment recovery rate, a high screening success rate, and strong generalization in various communities. Our study found that in a large community population, the prevalence of OSA screened using type IV equipment was 30.7%, and the prevalence of high-risk OSA screened using the Stop-Bang questionnaire was 42.8% (1,563/3,650). It is necessary to screen for OSA as early as possible in large community populations.
The Wisconsin Sleep Cohort Study of 4,925 people between 30 and 60 years of age found that 93% of women and 82% of men with moderate-to-severe OSA were never clinically diagnosed (14). Similarly, a Sleep Heart Health study showed that up to 91.7% of patients with suspected OSA had frequent snoring and sleepiness that were not diagnosed (15). Awareness of OSA is low in the population, and most high-risk OSA patients have not been identified. When the disease complicates, the difficulty in its management significantly increases. A large clinical intervention trial showed that continuous positive pressure ventilation therapy did not prevent secondary cardiovascular events (16). Therefore, early screening is needed to prevent and control OSA. Our study aimed to carry out early OSA screening in healthy community dwellers and successfully verify its feasibility.
The primary diagnostic devices for OSA include PSG and type III HSAT. HSAT can be used to diagnose patients with moderate-to-severe OSA without comorbidities (17,18). These devices have high requirements for technicians and the screening environment. They are expensive, relatively inefficient, and not suitable for screening OSA in a large sample population. Screening for OSA in the population relies on various questionnaires (19), which are time-consuming and subject to the influence of participants and evaluators. In this study, a type IV WISM was used, and pulse oxygen saturation was the most commonly recorded signal (20). Some studies have shown that ODI monitored using type IV sleep monitoring equipment alone or in combination with the Stop-Bang questionnaire is significantly better than the Stop-Bang questionnaire alone in OSA screening, particularly for mild OSA (21). A review of previous studies by the United States Prevention Task Force found that type IV sleep monitoring devices are generally accurate in diagnosing OSA, although there is inconsistency in estimating the actual AHI (22). WISM is a compact device with no external wires and can be attached to the palm in one step. The low difficulty of application ensures the successful wearing, integrity, and effectiveness of data collection.
In this study, five communities were screened for OSA. Among 4,438 residents who completed registration and were successfully issued WISM, 120 (2.7%) opted out of the inspection on the spot. Most residents felt that it was inconvenient to return the device the next day, which may be a problem with portable home-monitoring devices. Of the 4,318 devices issued, 9 (0.21%) were lost during the study, and the rest were returned. The type IV device is taken home by the patient, which may pose a risk of theft or loss. We tried to minimize this risk as much as possible, proving that this method is also feasible when screening large populations. The recovery rate of valid data in the five communities was as high as 99.37%. Excluding the lack of valid data caused by the participants’ failure to follow the guidance of field researchers due to <7 h of wearing time, and the failure of data recovery due to the equipment, the overall screening success rate in the five communities was 89.63%, and the failure rate of screening was 10.37%. Our results are consistent with previous findings that between 5% and 20% of the data in portable studies were of poor quality (23).
Type III HSAT simplifies the monitoring channel, including only respiratory airflow, chest and abdominal movement, and blood oxygen signals, and can be used to diagnose patients with moderate-to-severe OSA without comorbidities (17). To further validate the screening efficacy of type IV WISM in a large community population, 305 participants were selected for an additional overnight HSAT comparison. Our study showed a strong correlation and consistency between ODI obtained by the type IV WISM and AHI obtained by HSAT, suggesting that the type IV WISM has a good diagnostic performance as a screening tool. This type IV equipment was also compared with PSG at the Sleep Center of Guangdong Provincial People’s Hospital, and it was found that the sensitivity and specificity of type IV equipment were higher (9). The type IV equipment is small, light, time-consuming, and can screen 200 people per day, but with great accuracy. It has no external lead, and the device can be operated in one step to increase OSA screening population participation, making it possible to quickly screen for OSA in communities or large sample populations.
In addition, our study recorded a 30.7% prevalence of OSA in a large community population using type IV equipment’s ODI of ≥7 times/h, which is consistent with a systematic review of a 9–38% prevalence of OSA in the general population when OSA was diagnosed with an AHI of ≥5 beats per hour (24). However, because awareness of OSA is low, the true prevalence of OSA in the population may be much higher (25). Our study, which was dominated by women, showed that the prevalence of OSA was higher in men than in women (24); therefore, our study may have underestimated the prevalence of OSA in healthy people. In this study, the prevalence of high-risk OSA screened using the Stop-Bang questionnaire was higher than that using WISM, which may be due to the low specificity of the Stop-Bang questionnaire alone, although it has high sensitivity, leading to a high prevalence of screening (26). In this study, there was no difference in sleep quality between patients with OSA and non-OSA patients in the community population, although some studies have shown that people at high risk of OSA are more likely to have poor sleep quality than those at low risk of OSA (27). Poor sleep quality can lead to excessive daytime sleepiness and fatigue (28); therefore, there was no difference in the proportion of daytime sleepiness between patients with OSA and non-OSA patients in our community population.
Among the sociodemographic factors, the risk of OSA increased with age, with a 1.015 times increase for each additional year, which is similar to other studies (29-33). It is well known that men are at high risk of OSA (30), and our study found that men have a higher risk of OSA than women, which is supported by other findings (34,35). Punjabi et al. reported that men are at an increased risk of OSA and that men and women experience different symptoms (36).
Obesity is an independent risk factor for OSA. Most early studies used a BMI >30 kg/m2 as a cutoff to assess the risk of OSA (30,34,37). Our study demonstrated that the risk of OSA increased as BMI increased. The Kuprio Sleep Apnea Group reports (37) that obesity and OSA are bidirectionally related, and that obesity increases the risk of upper airway collapse, leading to sleep fragmentation, which further increases appetite, leading to weight gain and obesity. Leptin suppresses hypothalamic appetite by counteracting the effects of the orexigenic hormones neuropeptide Y and ghrelin (38,39). Sleep fragmentation attenuates leptin signaling in the hypothalamus, leading to an increase in the ghrelin/leptin ratio (40), which leads to increased appetite and uncontrolled food intake, leading to weight gain and obesity (38,39). However, differences in regional fat distribution in obese individuals may be more relevant to OSA severity than general measures of obesity, such as BMI, and neck circumference is well correlated with obesity in adults (40). In adults, increased neck circumference is associated with both snoring and OSA (41,42), particularly after adjusting for height (43). Our study also found that an increased neck circumference was an independent risk factor for OSA, and the risk of OSA increased with an increase in neck circumference.
Hypertension and metabolic abnormalities are the root causes of cardiovascular disease. There is a significant overlap between OSA and systemic blood pressure (44). The collapse of the upper respiratory tract in OSA leads to intermittent hypoxia, which leads to hyperactivation of the sympathetic output and endothelin system. As a result, a cascade of changes occurs that further leads to an increase in adrenergic tension, leading to hypertension (44). In our study, 26.3% of OSA-positive participants had hypertension, and 43.6% of patients with hypertension were estimated to have OSA. Further analysis showed that these patients had a 1.268 times greater risk of OSA than those with normotensive blood pressure, which is similar to the findings of other studies (31,34,36,45,46).
Our study concluded that the risk of OSA was not affected by tea consumption and that regular tea consumption did not increase the risk of OSA (P>0.05, 95% CI: 1.044–1.539). We did not find any studies that directly linked OSA with tea consumption. A meta-analysis reported that there are insufficient published data to assess whether OSA is associated with caffeine intake (47). This analysis also revealed that the association between OSA and tobacco use could not be confirmed based on the limited available evidence (47). Our study found that smoking was negatively associated with OSA after adjusting for confounding factors [UOR: 1.521 (1.247–1.855), AOR: 0.642 (0.497–0.830)]. Our findings are consistent with those of the Sleep Heart Health study, which also found an inverse association between smoking and OSA after adjusting for age and BMI (34). However, some studies have identified frequent chewing of tobacco or smoking as a potential risk factor for OSA (36,48). Smoking causes inflammation of the upper respiratory tract, leading to swelling of the uvula and narrowing and collapse of the airway (49). Further evidence is needed to clarify this issue, as there is insufficient evidence to confirm the association with tobacco (47).
In our study, ODI was used to diagnose OSA. ODI has been proved to be at least as useful a tool to screen, diagnose, and quantify OSA (50). However, the OSA phenotype may interfere with OSA diagnosis based on ODI alone. There is a study has shown that the OSA subtype is based on self-reported symptoms (51), and some OSA patients cannot rely on ODI alone for OSA diagnosis, and need to be combined with scale symptoms for subtype diagnosis.
The main advantage of this study is that it first used a type IV WISM to screen for OSA in a large sample of the community population. At the same time, the Stop-Bang questionnaire was also used to screen the prevalence and risk factors of OSA in a community healthy population. After screening, some individuals were selected to undergo HSAT and other confirmatory tests to prove the accuracy and feasibility of screening. Second, the adequate sample size, robust sampling methods, and use of standardized questionnaires increased the external validity of our study. Other potential risk factors, such as diabetes, asthma, chronic obstructive pulmonary disease, and other coexisting diseases, can be explored in future studies to overcome the limitations of the current study. In addition, tea intake, alcohol consumption, and smoking can be quantified to improve the reliability of the association between OSA and community risk factors.
Conclusions
In conclusion, our study confirmed the feasibility of using an objective type IV WISM to complete the screening of large community samples in a short period and validated the accuracy of this practical OSA screening method. The prevalence of high-risk OSA screened using the Stop-Bang questionnaire was higher than that screened using the objective screening device. The prevalence of OSA in the healthy community population was 30.7% using the objective screening device. This suggests the need for early screening of OSA in healthy community populations for definite diagnosis and treatment.
Acknowledgments
We thank the Sleep Center, Guangdong Provincial People’s Hospital (Guangdong Academy of Medical Sciences) for their assistance in this work.
Funding: This work was supported by
Footnote
Provenance and Peer Review: This article was a standard submission to the series “Sleep Section” published in the Journal of Thoracic Disease. The article has undergone external peer review.
Reporting Checklist: The authors have completed the STROBE reporting checklist. Available at https://jtd.amegroups.com/article/view/10.21037/jtd-22-1538/rc
Data Sharing Statement: Available at https://jtd.amegroups.com/article/view/10.21037/jtd-22-1538/dss
Peer Review File: Available at https://jtd.amegroups.com/article/view/10.21037/jtd-22-1538/prf
Conflicts of Interest: All authors have completed the ICMJE uniform disclosure form (available at https://jtd.amegroups.com/article/view/10.21037/jtd-22-1538/coif). The series “Sleep Section” was commissioned by the editorial office without any funding or sponsorship. The authors have no other conflicts of interest to declare.
Ethical Statement: The authors are accountable for all aspects of the work in ensuring that questions related to the accuracy or integrity of any part of the work are appropriately investigated and resolved. The study was conducted in accordance with the Declaration of Helsinki (as revised in 2013). This study was approved by the Ethics Committee of Guangdong Provincial People’s Hospital [approval No. GDREC2020221H (R1)]. All the participants provided written informed consent.
Open Access Statement: This is an Open Access article distributed in accordance with the Creative Commons Attribution-NonCommercial-NoDerivs 4.0 International License (CC BY-NC-ND 4.0), which permits the non-commercial replication and distribution of the article with the strict proviso that no changes or edits are made and the original work is properly cited (including links to both the formal publication through the relevant DOI and the license). See: https://creativecommons.org/licenses/by-nc-nd/4.0/.
References
- Malhotra A, White DP. Obstructive sleep apnoea. Lancet 2002;360:237-45. [Crossref] [PubMed]
- Peppard PE, Young T, Barnet JH, et al. Increased prevalence of sleep-disordered breathing in adults. Am J Epidemiol 2013;177:1006-14. [Crossref] [PubMed]
- Benjafield AV, Ayas NT, Eastwood PR, et al. Estimation of the global prevalence and burden of obstructive sleep apnoea: a literature-based analysis. Lancet Respir Med 2019;7:687-98. [Crossref] [PubMed]
- Jaiswal SJ, Owens RL, Malhotra A. Raising awareness about sleep disorders. Lung India 2017;34:262-8. [Crossref] [PubMed]
- Watson NF. Health Care Savings: The Economic Value of Diagnostic and Therapeutic Care for Obstructive Sleep Apnea. J Clin Sleep Med 2016;12:1075-7. [Crossref] [PubMed]
- Young T, Palta M, Dempsey J, et al. The occurrence of sleep-disordered breathing among middle-aged adults. N Engl J Med 1993;328:1230-5. [Crossref] [PubMed]
- Thornton JD, Chandriani K, Thornton JG, et al. Assessing the prioritization of primary care referrals for polysomnograms. Sleep 2010;33:1255-60. [Crossref] [PubMed]
- Ulasli SS, Gunay E, Koyuncu T, et al. Predictive value of Berlin Questionnaire and Epworth Sleepiness Scale for obstructive sleep apnea in a sleep clinic population. Clin Respir J 2014;8:292-6. [Crossref] [PubMed]
- Xu Y, Ou Q, et al. Comparative study of a wearable intelligent sleep monitor and polysomnography monitor for the diagnosis of obstructive sleep apnea. Sleep Breath 2023;27:205-12. [Crossref] [PubMed]
- He H, Pan L, Hu Y, et al. The diverse life-course cohort (DLCC): protocol of a large-scale prospective study in China. Eur J Epidemiol 2022;37:871-80. [Crossref] [PubMed]
- He H, Pan L, Pa L, et al. Data Resource Profile: The China National Health Survey (CNHS). Int J Epidemiol 2018;47:1734-1735f. [Crossref] [PubMed]
- Tsutsui JM, Xie F, Cloutier D, et al. Real-time dobutamine stress myocardial perfusion echocardiography predicts outcome in the elderly. Eur Heart J 2008;29:377-85. [Crossref] [PubMed]
- Sui X, Hooker SP, Lee IM, et al. A prospective study of cardiorespiratory fitness and risk of type 2 diabetes in women. Diabetes Care 2008;31:550-5. [Crossref] [PubMed]
- Young T, Evans L, Finn L, et al. Estimation of the clinically diagnosed proportion of sleep apnea syndrome in middle-aged men and women. Sleep 1997;20:705-6. [Crossref] [PubMed]
- Kapur V, Strohl KP, Redline S, et al. Underdiagnosis of sleep apnea syndrome in U.S. communities. Sleep Breath 2002;6:49-54. [Crossref] [PubMed]
- McEvoy RD, Antic NA, Heeley E, et al. CPAP for Prevention of Cardiovascular Events in Obstructive Sleep Apnea. N Engl J Med 2016;375:919-31. [Crossref] [PubMed]
- Kapur VK, Auckley DH, Chowdhuri S, et al. Clinical Practice Guideline for Diagnostic Testing for Adult Obstructive Sleep Apnea: An American Academy of Sleep Medicine Clinical Practice Guideline. J Clin Sleep Med 2017;13:479-504. [Crossref] [PubMed]
- Behar JA, Palmius N, Zacharie S, et al. Single-channel oximetry monitor versus in-lab polysomnography oximetry analysis: does it make a difference? Physiol Meas 2020;41:044007. [Crossref] [PubMed]
- Chiu HY, Chen PY, Chuang LP, et al. Diagnostic accuracy of the Berlin questionnaire, STOP-BANG, STOP, and Epworth sleepiness scale in detecting obstructive sleep apnea: A bivariate meta-analysis. Sleep Med Rev 2017;36:57-70. [Crossref] [PubMed]
- Practice parameters for the use of portable recording in the assessment of obstructive sleep apnea. Standards of Practice Committee of the American Sleep Disorders Association. Sleep 1994;17:372-7. [PubMed]
- Mashaqi S, Staebler D, Mehra R. Combined nocturnal pulse oximetry and questionnaire-based obstructive sleep apnea screening - A cohort study. Sleep Med 2020;72:157-63. [Crossref] [PubMed]
- US Preventive Services Task Force. Screening for Obstructive Sleep Apnea in Adults: US Preventive Services Task Force Recommendation Statement. JAMA 2017;317:407-14. [Crossref] [PubMed]
- Bruyneel M, Ninane V. Unattended home-based polysomnography for sleep disordered breathing: current concepts and perspectives. Sleep Med Rev 2014;18:341-7. [Crossref] [PubMed]
- Senaratna CV, Perret JL, Lodge CJ, et al. Prevalence of obstructive sleep apnea in the general population: A systematic review. Sleep Med Rev 2017;34:70-81. [Crossref] [PubMed]
- Chen X, Wang R, Zee P, et al. Racial/Ethnic Differences in Sleep Disturbances: The Multi-Ethnic Study of Atherosclerosis (MESA). Sleep 2015;38:877-88. [Crossref] [PubMed]
- Chung F, Abdullah HR, Liao P. STOP-Bang Questionnaire: A Practical Approach to Screen for Obstructive Sleep Apnea. Chest 2016;149:631-8. [Crossref] [PubMed]
- Aggarwal K, Akhtar N, Mallick H. Sleep quality mediates the relationship between risk of obstructive sleep apnea and acute stress in young adults. J Physiol Pharmacol 2021;72: [Crossref] [PubMed]
- Sleep-related breathing disorders in adults: recommendations for syndrome definition and measurement techniques in clinical research. The Report of an American Academy of Sleep Medicine Task Force. Sleep 1999;22:667-89. [Crossref] [PubMed]
- Qaseem A, Dallas P, Owens DK, et al. Diagnosis of obstructive sleep apnea in adults: a clinical practice guideline from theAmerican College of Physicians. Ann Intern Med 2014;161:210-20. [Crossref] [PubMed]
- Al-Jewair TS, Nazir MA, Al-Masoud NN, et al. Prevalence and risks of habitual snoring and obstructive sleep apnea symptoms in adult dental patients. Saudi Med J 2016;37:183-90. [Crossref] [PubMed]
- Desalu OO, Onyedum CC, Adeoti AO, et al. Identifying patients at high risk for obstructive sleep apnoea syndrome in Nigeria: A multicentre observational study. Malawi Med J 2017;29:183-8. [Crossref] [PubMed]
- Cadelis G, Fayad Y, Monteagudo OE. Prevalence of symptoms and risk of obstructive sleep apnea syndrome assessed by the Berlin Questionnaire among professionals of a health facility. Rev Epidemiol Sante Publique 2016;64:405-14. [Crossref] [PubMed]
- Young T, Skatrud J, Peppard PE. Risk factors for obstructive sleep apnea in adults. JAMA 2004;291:2013-6. [Crossref] [PubMed]
- Singh A, Prasad R, Garg R, et al. A study to estimate prevalence and risk factors of Obstructive Sleep Apnoea Syndrome in a semi-urban Indian population. Monaldi Arch Chest Dis 2017;87:773. [Crossref] [PubMed]
- Modena DAO, Cazzo E, Cândido EC, et al. Obstructive sleep apnea syndrome among obese individuals: A cross-sectional study. Rev Assoc Med Bras (1992) 2017;63:862-8. [Crossref] [PubMed]
- Punjabi NM. The epidemiology of adult obstructive sleep apnea. Proc Am Thorac Soc 2008;5:136-43. [Crossref] [PubMed]
- Tuomilehto H, Gylling H, Peltonen M, et al. Sustained improvement in mild obstructive sleep apnea after a diet- and physical activity-based lifestyle intervention: postinterventional follow-up. Am J Clin Nutr 2010;92:688-96. [Crossref] [PubMed]
- Hakim F, Wang Y, Carreras A, et al. Chronic sleep fragmentation during the sleep period induces hypothalamic endoplasmic reticulum stress and PTP1b-mediated leptin resistance in male mice. Sleep 2015;38:31-40. [Crossref] [PubMed]
- Spiegel K, Tasali E, Penev P, et al. Brief communication: Sleep curtailment in healthy young men is associated with decreased leptin levels, elevated ghrelin levels, and increased hunger and appetite. Ann Intern Med 2004;141:846-50. [Crossref] [PubMed]
- Ben-Noun L, Sohar E, Laor A. Neck circumference as a simple screening measure for identifying overweight and obese patients. Obes Res 2001;9:470-7. [Crossref] [PubMed]
- Stradling JR, Crosby JH. Predictors and prevalence of obstructive sleep apnoea and snoring in 1001 middle aged men. Thorax 1991;46:85-90. [Crossref] [PubMed]
- Caffo B, Diener-West M, Punjabi NM, et al. A novel approach to prediction of mild obstructive sleep disordered breathing in a population-based sample: the Sleep Heart Health Study. Sleep 2010;33:1641-8. [Crossref] [PubMed]
- Davies RJ, Ali NJ, Stradling JR. Neck circumference and other clinical features in the diagnosis of the obstructive sleep apnoea syndrome. Thorax 1992;47:101-5. [Crossref] [PubMed]
- Hla KM, Young TB, Bidwell T, et al. Sleep apnea and hypertension. A population-based study. Ann Intern Med 1994;120:382-8. [Crossref] [PubMed]
- Sahlin C, Franklin KA, Stenlund H, et al. Sleep in women: Normal values for sleep stages and position and the effect of age, obesity, sleep apnea, smoking, alcohol and hypertension. Sleep Med 2009;10:1025-30. [Crossref] [PubMed]
- Nieto FJ, Young TB, Lind BK, et al. Association of sleep-disordered breathing, sleep apnea, and hypertension in a large community-based study. Sleep Heart Health Study. JAMA 2000;283:1829-36. [Crossref] [PubMed]
- Taveira KVM, Kuntze MM, Berretta F, et al. Association between obstructive sleep apnea and alcohol, caffeine and tobacco: A meta-analysis. J Oral Rehabil 2018;45:890-902. [Crossref] [PubMed]
- Krishnan V, Dixon-Williams S, Thornton JD. Where there is smoke…there is sleep apnea: exploring the relationship between smoking and sleep apnea. Chest 2014;146:1673-80. [Crossref] [PubMed]
- Kim KS, Kim JH, Park SY, et al. Smoking induces oropharyngeal narrowing and increases the severity of obstructive sleep apnea syndrome. J Clin Sleep Med 2012;8:367-74. [Crossref] [PubMed]
- Rashid NH, Zaghi S, Scapuccin M, et al. The Value of Oxygen Desaturation Index for Diagnosing Obstructive Sleep Apnea: A Systematic Review. Laryngoscope 2021;131:440-7. [Crossref] [PubMed]
- Ye L, Pien GW, Ratcliffe SJ, et al. The different clinical faces of obstructive sleep apnoea: a cluster analysis. Eur Respir J 2014;44:1600-7. [Crossref] [PubMed]