This article has an erratum available at: http://dx.doi.org/10.21037/jtd-2023-20 the article has been update on 2023-12-20 at here.
Time-course transcriptome analysis of lungs from mice infected with inhaled aerosolized Stenotrophomonas maltophilia
Highlight box
Key findings
• This is the first study to investigate the pulmonary transcriptional response to Stenotrophomonas maltophilia (SMA) infection. The expression levels of Cxcl10, Cd14, Gbp5, Cxcr2, Tnip1, Zc3h12a, Egr1, Sell and Gbp2 were high in SMA pneumonia, and they may be important targets for future studies.
What is known and what is new?
• SMA has emerged as an important opportunistic pathogen capable of causing an invasive and fatal infection.
• RNA sequencing was used to create a transcriptional profile of the lungs of mice used for modelling over time. A gradual change in the lung’s transcriptional profile was observed, which was consistent with the expected disease progression.
What is the implication, and what should change now?
• The time-course transcriptome analysis shed light on the molecular mechanisms underlying the pathogenesis of SMA pneumonia, which may aid in the development of therapies to reduce the occurrence of SMA pulmonary infection.
Introduction
Stenotrophomonas maltophilia (SMA) is an important gram-negative multidrug-resistant bacterial pathogen that is widely distributed in the environment and has an effect on human health around the world (1). SMA is an opportunistic pathogen that causes a variety of serious infections in humans, including respiratory infections, bacteremia, endocarditis, gastrointestinal tract infections, liver infection, urinary tract infections, nervous system and spinal cord infections, eye infections, soft tissue and bone infections, and medical implant infections. Pneumonia and bacteremia are the most common clinical manifestations of this infection, with reported overall mortality rates ranging from 30% to 51% in patients with bacteremia (2), nearly 50% in patients with hospital-acquired pneumonia in intensive care units (3), and 100% in patients with hemorrhagic pneumonia (4). Despite the high mortality rate associated with SMA pneumonia, there is limited experience with the clinical management of pneumonia caused by this bacterium (5-7). In our previous research, outer membrane proteins (Omps) from SMA were isolated and potential vaccine candidates of Omps against SMA were identified (8). As a result, a better understanding of SMA pneumonia is needed.
The pathogenesis of SMA infection involves numerous virulence factors. Outer membrane vesicles (OMVs) secreted by SMA elicit early inflammatory responses in mouse lungs, including congestion and neutrophil infiltration, as well as elevated expression of proinflammatory cytokine and chemokine genes, and stimulate host defense responses (1,9). StmPr1, the major extracellular protease, degrades fibronectin, fibronectin receptor integrin a5b1, collagen, E-cadherin, tight junction proteins ZO-1, and occludin; disrupts the epithelial cell barrier; recruits neutrophils; inactivates host immune defense components and is a major player in lung cell injury (10). Flagella are directly involved in the adhesion to mouse tracheal mucus, and binding to mucus inhibits the movement of bacteria within the respiratory tract and protects them from the host’s immune response (11). Moreover, flagellin has been shown to increase the production of proinflammatory cytokines [interleukin (IL)-1β and tumor necrosis factor alpha (TNF-α)], increase the production of anti-inflammatory cytokines (IL-10), elevate the levels of myeloperoxidase and nitric oxide, significantly increase the engulfment activity of alveolar macrophages and the number of infiltrating leukocytes, and provide nonspecific protection against SMA challenge (12). Overall, these findings suggest that SMA infection may activate different gene expression patterns in various types of host cells, with varying effects on specific cell types. The pathogenesis of SMA infection and SMA-host interactions are complicated.
Several methods of inoculation have been reported for establishing SMA pneumonia models, including nasal inoculation, intratracheal instillation, and aerosol challenge in mice (13-15). In general, nasal inoculation may lead to unstable model establishment due to the inability to accurately control the dose of infection and the highly variable deposition of microorganisms in the lungs. Intratracheal instillation does not readily simulate naturally occurring aerosol-borne infections. Inhalational aerosol pneumonia models mostly involve the route of oral-nasal contact and systemic exposure, but accurate quantification of the administered dose may be limited. Unlike previous methods of inoculation, aerosol endotracheal inhalation uses a hand-held liquid aerosol pulmonary delivery device to directly place a known quantity of the organism into the lungs of mice (16). It is characterized by straightforward operation, high success rates, easy reproducibility and low animal mortality.
The lung is an important organ for SMA infection as well as a major immune organ in vertebrate animals. To investigate the mechanisms of SMA-host interactions, a detailed description of SMA pneumonia is needed. Due to the decreasing cost of next-generation sequencing (NGS), RNA sequencing (RNA-seq) has become a popular method for analyzing transcriptomes in recent years. Numerous studies have used RNA-seq to investigate host-pathogen interactions, host response mechanisms to genetic and infectious diseases (17). Similarly, we performed time-course RNA-seq of lung tissues from mice with SMA pulmonary infection via aerosolized intratracheal inhalation to investigate transcription profile changes in SMA-infected lungs and to identify genes or other valuable research targets. This research advances our understanding of the pathogenesis of SMA pneumonia. We present this article in accordance with the ARRIVE reporting checklist (available at https://jtd.amegroups.com/article/view/10.21037/jtd-23-1138/rc).
Methods
Bacterial strain and mice
The SMA strain K279a, which has been previously described (18), was stored in our lab. Bacteria were cultured overnight in lysogeny broth (LB) in a 37 ℃ shaker. On the second day, it was transferred to 50 mL LB medium at 1:100 for expansion to an optical density (OD) at 600 nm of 1.0 and centrifuged at 3,000 reactive centrifugal force (rcf) for 5 min. The bacterial pellets were washed twice with sterile phosphate-buffered saline (PBS) and adjusted to reach an OD at 600 nm of approximately 1.0 [approximately 5×108 colony forming units (CFU)/mL)]. The bacterial pellets obtained from 1.0 mL volumes of bacterial culture media were resuspended with 50 µL of sterile PBS, and were used to challenge the mice. Male C57BL/6J mice were purchased from SPF (Beijing, China) Biotechnology Co., Ltd. (Beijing, China) and maintained under specific pathogen-free conditions at the Academy of Military Medical Sciences (AMMS) animal facilities for 1 week before use. Six- to seven-week-old mice weighing 18–20 grams were used in the experiment. A protocol was prepared before the study without registration. Animal experiments were performed under a project license (No. IACUC-DWZX-2022-064) granted by Animal Care and Use Committee of the AMMS, in compliance with institutional guidelines for the care and use of animals.
Experimental mouse model of acute lethal SMA pneumonia via aerosolized intratracheal inoculation
Mice were inoculated via the aerosolized intratracheal route with either PBS or SMA as described previously (16). In brief, isoflurane was administered through a small animal anesthesia machine (Yuyan Scientific Instrument Co., Ltd., Shanghai, China) to anesthetize the mice. Supine mice were secured after anesthesia. When the tracheal opening was fully exposed by a small animal laryngoscope (Huironghe Company, Beijing, China), 50 µL of the suspension was sprayed into the trachea (near the bifurcation of the trachea) by using an Intratracheal Aerosolizer Device (Huironghe Company, Beijing, China), followed by uniform dispersion of the solution throughout the lung. To establish an acute lethal pneumonia model, mice (n=10) were noninvasively intratracheally inoculated with 5×108 CFU/mL of SMA in 50 µL of PBS. Post-infection mouse survival rates were monitored for 14 days. For transcriptome analysis and histopathological analysis, mice were humanely sacrificed at 4, 12, 24, 48, and 72 hours post-infection (hpi). So, the mice were divided into 6 groups and 4 mice each group. The mouse lungs were removed and split into two pieces for total RNA extraction or histopathological analysis. An inoculation of 50 µL of PBS was used as a control at 0 hpi.
Histopathology analysis
Paraformaldehyde-fixed and paraffin-embedded lung sections were used. In accordance with standard protocols, 3–5 µm sections were cut and stained with hematoxylin & eosin (H&E), and then the sealing slides were observed by light microscopy (200×). By using H&E staining and a lung injury scoring system, the histology of the lungs was evaluated. Based on histological evaluations, injury scores were 0= none, 1= slight, 2= mild, 3= moderate, and 4= severe. The categorization of the lesions was related to the degree of pathological lesions as follows: inflammatory cell infiltration, interstitial inflammation, congestion, and edema. One-way analysis of variance (ANOVA) was used to analyze pathology scores.
RNA quantification, library construction and sequencing
The mouse lungs, each in 5 mL of TRIzol (Invitrogen, ThermoFisher Scientific Inc., Carlsbad, CA, USA), were stored at −80 ℃ prior to processing and shipped on dry ice. The quantity and integrity of total RNA was analyzed using a Bioanalyzer 2100 system (Agilent Technologies, CA, USA). mRNA was enriched from 3 µg of total RNA using poly-T oligo-attached magnetic beads and fragmented by using divalent cations under elevated temperature. Moloney murine leukemia virus (M-MuLV) reverse transcriptase, random hexamer primers, DNA polymerase I and deoxynucleotide triphosphate (dNTP) were used to create double-stranded complementary DNA (cDNA), which were then further purified with AMPure XP beads. The final cDNA libraries were created by further purifying and enriching the cDNA fragments using polymerase chain reaction (PCR) (15 cycles) and sequenced by using an Illumina NovaSeq 6000. Construction of the library and sequencing were carried out by Novogene Company (Beijing, China).
Analysis of RNA-seq data
The high-throughput sequencer’s measurements of image data were converted into sequence data (reads) by CASAVA (Illumina, San Diego, CA, USA) base recognition. By eliminating adapter and N-base-containing reads as well as low-quality reads from the raw data, clean data (clean reads) were obtained. The Q20, Q30 and GC contents of the clean data were calculated. Direct downloads of the reference genome and gene model annotation files from the genome (mm10) website were performed. Hisat2 (v2.0.5) was used to create an index of the reference genome and to match clean paired-end reads to the reference genome. Read counts that were mapped to each gene were counted using featureCounts (v1.5.0-p3, SourceForge, San Diego, CA, USA). The length of each gene and the number of reads mapped to it were used to compute the fragments per kilobase of transcript per million mapped reads (FPKM) of each gene. Principal component analysis (PCA) was used to analyze the distribution of samples to judge the quality of the data.
Identification of differentially expressed genes (DEGs) and functional classification of DEGs
The DESeq2 R package (1.20.0) (http://www.bioconductor.org/) was used to analyze differential expression between the two groups. P values were adjusted using Benjamini and Hochberg’s method to reduce the false discovery rate. Using an adjusted P value (Padj) £0.05 and |log2fold change| ³1 as cutoffs in this study, DEGs were identified and visualized using a volcano plot. DEGs were also functionally classified. Gene Ontology (GO) functional enrichment was conducted using the GO database (https://geneontology.org/), and Kyoto Encyclopedia of Genes and Genomes (KEGG) pathway enrichment was conducted using the KEGG database (http://www.genome.jp/kegg/).
Time courses analysis of gene expression
To cluster the genes identified in the time courses, we used the R package Mfuzz (http://www.bioconductor.org/) (19), which performs fuzzy c-means clustering. To remove the influence of expression magnitude and focus on the expression dynamics, DESeq2-normalized data were standardized in Mfuzz to have a mean value of zero and a standard deviation of one. A total of 9 clusters were used, and the fuzzifier coefficient, M, was set to 1.71.
Coexpression network establishment by weighted gene coexpression network analysis (WGCNA)
WGCNA is a computational technique for describing the mechanisms of gene relationships among various samples. Based on the coherence of gene sets and the association between gene sets and symptoms, it can be utilized to identify gene sets that are highly synergistically altered and suggest possible biomarkers or therapeutic targets (20). Using the WGCNA package in R, a weighted correlation network analysis was performed for the 22 lung samples to identify modules for all genes with various expression patterns. First, the data were filtered by calculating the coefficient of variation to remove genes with low coefficients of variation, which was 0.5 for this metric. The gene network was assumed to obey a scale-free network distribution, the gene coexpression correlation matrix was defined, and the coefficient of dissimilarity of different nodes was calculated. Using hierarchical clustering, genes with highly similar coexpression relationships were grouped according to their topological overlaps. Modules with the highest coefficients were selected as targets.
Immune cell infiltration score
The Immune Cell Abundance Identifier for mouse (ImmuCellAI-mouse, http://bioinfo.life.hust.edu.cn/ImmuCellAI-mouse/#!/) is a tool that estimates the relative proportions of 36 immune cell species based on mouse gene expression data (21). Using a hierarchical prediction strategy, ImmuCellAI-mouse was used to predict the abundance of immune cells and immune cell subtypes separately by simulating the flow cytometry analysis process. Layer 1 comprises seven different types of immune cells: T cells, B cells, natural killer (NK) cells, monocytes, granulocytes, macrophages, and dendritic cells (DCs). Layer 2 cells were subtypes of cells in the first layer, such as T-cell subtypes [CD4 T, NKT, CD8 T, and γδ T cells], B-cell subtypes (B1, memory B, marginal zone B, germinal center B, follicular B, and plasma B cells), subtypes of granulocytes (neutrophils, basophils, mast cells and eosinophils), subtypes of macrophages (M1 and M2 macrophages), and subtypes of DCs [monocyte-derived DCs (moDCs), plasmacytoid DCs (pDCs), type 1 conventional DCs (cDC1s) and type 2 conventional DCs (cDC2s)]. Finally, cells in layer 3 are CD4 T and CD8 T cell subtypes (including CD4 naïve, CD4 memory, T helper, regulatory T (Treg), CD8 naïve, CD8 effector memory, CD8 central memory, cytotoxic and exhausted cells). The prediction process used to analyze each sequential layer was performed separately.
Validation of RNA-seq data by quantitative real-time PCR (qRT-PCR)
To validate the RNA-seq data, 9 DEGs were randomly chosen for qRT-PCR validation of their expression patterns at five time points. Lung tissue from mice infected with SMA at 4, 12, 24, 48, and 72 hpi was dissected and snap-frozen in liquid nitrogen. RNA was extracted and measured using spectrophotometry. The TRIzol reagent was obtained from Sangon Biotech Co., Ltd. (Shanghai, China), as well as the AMV First Strand cDNA Synthesis Kit. PowerUpTM SYBRTM Green master mix (Thermo Scientific, USA) was used for qRT-PCR. The housekeeping reference gene Gapdh was used to compute relative quantification. Primers are listed in Table 1.
Table 1
Primer | Sequences (5' to 3') |
---|---|
Csf3-F | ATGGCTCAACTTTCTGCCCAG |
Csf3-R | CTGACAGTGACCAGGGGAAC |
Fos-F | CGGGTTTCAACGCCGACTA |
Fos-R | TTGGCACTAGAGACGGACAGA |
Fosl1-F | ATGTACCGAGACTACGGGGAA |
Fosl1-R | CTGCTGCTGTCGATGCTTG |
Ifng-F | ATGAACGCTACACACTGCATC |
Ifng-R | CCATCCTTTTGCCAGTTCCTC |
Mmp9-F | CTGGACAGCCAGACACTAAAG |
Mmp9-R | CTCGCGGCAAGTCTTCAGAG |
Tnfaip3-F | GAACAGCGATCAGGCCAGG |
Tnfaip3-R | GGACAGTTGGGTGTCTCACATT |
Myo1f-F | CTTTCACTGGCAGAGTCACAA |
Myo1f-R | ATGAAGCGTTTGCGGAGGTT |
Ptafr-F | TATACTGGGGGTGGTTGCCAA |
Ptafr-R | GCAGGTCAGCCATAGTGAGATTC |
Ncf1-F | ACACCTTCATTCGCCATATTGC |
Ncf1-R | TCGGTGAATTTTCTGTAGACCAC |
Gapdh-F | AGGTCGGTGTGAACGGATTTG |
Gapdh-R | TGTAGACCATGTAGTTGAGGTCA |
qRT-PCR, quantitative real-time polymerase chain reaction; F, forward primer; R, reverse primer.
Statistical analysis
Comparisons between two groups were carried out using two-tailed unpaired t-tests. Nonparametric analysis was performed on data that lacked a normal distribution using the Mann-Whitney U test. One-way ANOVA was used to analyze pathology scores. Using the Kaplan-Meier method and log rank test, survival experiments were analyzed. Results with P<0.05 were defined as statistically significant. Statistics were analyzed using SPSS 16 (IBM, Arnmonk, NY, USA) and GraphPad Prism 8.0 (GraphPad, La Jolla, CA, USA).
Results
An acute lethal pneumonia model and histopathological changes in the lungs via aerosolized intratracheal inoculation of SMA
Mice infected with SMA via aerosolized intratracheal inoculation were monitored daily for survival events for 14 days. A control inoculation of 50 µL of PBS was used. Two mice whose death occurred within the initial 3-hour period were deemed as unexpected deaths and hence eliminated from the research analysis. Very few animals (3 mice) died within the first 4 hpi, probably due to the combined effects of asphyxia and anesthesia. These animals were excluded from the experimental group. Most mice in the model group died within 4 days, and mortality in this group differed from that in the control group (P<0.01) (Figure 1A). As a result, we selected six time points for subsequent experiments and analysis: 0, 4, 12, 24, 48, and 72 hpi (Figure 1B). Histopathological changes assessed using H&E staining revealed neutrophil infiltration in lung tissue at 4 hpi, followed by a significant increase in the infiltration of neutrophils and monocytes at 12–72 hpi. At 72 hpi, there was massive destruction of alveolar structures, thickening of alveolar septa, and hemorrhage. The H&E staining results were similar at 48 hpi (not presented) and 72 hpi. Pathological staining of the lungs of mice that died from the infection showed severe pulmonary hemorrhage and a large level of neutrophil infiltration. This result is consistent with findings reported previously (22). The severity of the lung lesions increased over time, according to histological scoring (Figure 1C). During histopathological verification, mice infected with SMA were shown to exhibit excessive acute inflammation and severe lung damage. As a result, the mouse model of acute lethal SMA pneumonia was successfully established, and all subsequent experiments were conducted using this animal model.
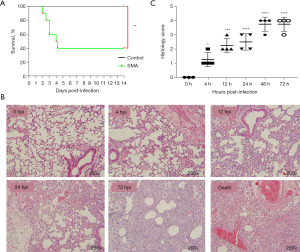
Overview of mRNA sequencing data and transcriptome analysis
To identify the expression levels of mRNAs in the lungs of mice infected by SMA at different times, 22 cDNA libraries were constructed and sequenced (Table 2). All 22 libraries were shown to be appropriate for future research. PCA is commonly used to assess between-group differences and sample replicates within groups. Our PCA of the gene expression value (FPKM) for all samples revealed that samples were dispersed across groups and clustered together within groups. Principal component 1 (PC1) was 37.79%, and principal component 2 (PC2) was 26.62% (Figure 2A). DEGs were identified across five time points after SMA infection using the control group (0 hpi) as a reference. A total of 3,165 upregulated DEGs and 2,231 downregulated DEGs were detected at 4 hpi, 2,798 upregulated DEGs and 2,774 downregulated DEGs were detected at 12 hpi, 2,423 upregulated DEGs and 2,398 downregulated DEGs were detected at 24 hpi, 2,601 upregulated DEGs and 2,877 downregulated DEGs were detected at 48 hpi, and 2,512 upregulated DEGs and 2,468 downregulated DEGs were detected at 72 hpi (Figure 2B,2C). A total of 1,078 common DEGs upregulated and 635 downregulated were identified for all time points (Figure 2D,2E). We focused on analyzing gene expression profiles in the lungs within the first 4 hours to increase understanding of the rapid and intense inflammatory response of the host. The top 20 genes with the most significant levels of increased expression at 4 hpi were selected. The Padj and log2fold change at each time point post-infection of these top 20 DEGs are shown in Table 3.
Table 2
Groups | Samples | Raw reads | Raw bases (Gb) | Clean reads | Clean bases (Gb) | Error rate | Q20 | Q30 | GC_pct |
---|---|---|---|---|---|---|---|---|---|
PBS_0hpi | PBS_0hpi_1 | 42,228,594 | 6.33 | 41,759,534 | 6.26 | 0.03 | 97.14 | 92.31 | 50.33 |
PBS_0hpi_2 | 43,839,812 | 6.58 | 43,216,564 | 6.48 | 0.03 | 97.28 | 92.65 | 50.42 | |
PBS_0hpi_3 | 43,244,250 | 6.49 | 42,623,410 | 6.39 | 0.03 | 97.24 | 92.57 | 50.70 | |
SMA_4hpi | SMA_4hpi_1 | 42,063,630 | 6.31 | 41,313,924 | 6.20 | 0.03 | 97.28 | 92.70 | 50.25 |
SMA_4hpi_2 | 41,305,014 | 6.20 | 40,731,388 | 6.11 | 0.03 | 96.99 | 91.93 | 49.97 | |
SMA_4hpi_3 | 42,436,230 | 6.37 | 42,044,286 | 6.31 | 0.03 | 97.15 | 92.29 | 50.16 | |
SMA_4hpi_4 | 44,325,732 | 6.65 | 43,860,822 | 6.58 | 0.03 | 97.15 | 92.34 | 49.96 | |
SMA_12hpi | SMA_12hpi_1 | 42,304,498 | 6.35 | 41,936,772 | 6.29 | 0.03 | 97.18 | 92.38 | 50.79 |
SMA_12hpi_2 | 40,587,140 | 6.09 | 40,089,834 | 6.01 | 0.03 | 97.09 | 92.22 | 50.62 | |
SMA_12hpi_3 | 42,085,216 | 6.31 | 41,490,048 | 6.22 | 0.03 | 97.13 | 92.29 | 50.70 | |
SMA_12hpi_4 | 40,875,702 | 6.13 | 40,252,592 | 6.04 | 0.03 | 97.44 | 93.01 | 50.59 | |
SMA_24hpi | SMA_24hpi_1 | 44,136,712 | 6.62 | 43,427,232 | 6.51 | 0.03 | 96.95 | 91.95 | 50.82 |
SMA_24hpi_2 | 39,886,074 | 5.98 | 39,395,930 | 5.91 | 0.03 | 97.29 | 92.66 | 51.00 | |
SMA_24hpi_3 | 42,948,088 | 6.44 | 42,331,144 | 6.35 | 0.03 | 97.09 | 92.18 | 50.98 | |
SMA_24hpi_4 | 43,979,296 | 6.60 | 43,343,624 | 6.50 | 0.03 | 97.13 | 92.34 | 51.03 | |
SMA_48hpi | SMA_48hpi_1 | 39,302,900 | 5.90 | 38,824,468 | 5.82 | 0.03 | 97.01 | 92.05 | 51.22 |
SMA_48hpi_2 | 43,664,558 | 6.55 | 43,074,616 | 6.46 | 0.03 | 97.30 | 92.71 | 50.83 | |
SMA_48hpi_3 | 40,546,928 | 6.08 | 40,176,842 | 6.03 | 0.03 | 97.20 | 92.45 | 50.94 | |
SMA_48hpi_4 | 42,796,292 | 6.42 | 41,952,940 | 6.29 | 0.03 | 97.32 | 92.67 | 50.59 | |
SMA_72hpi | SMA_72hpi_1 | 41,582,504 | 6.24 | 41,139,320 | 6.17 | 0.03 | 96.76 | 91.43 | 51.16 |
SMA_72hpi_2 | 42,573,664 | 6.39 | 41,991,574 | 6.30 | 0.03 | 97.25 | 92.63 | 50.99 | |
SMA_72hpi_3 | 41,089,246 | 6.16 | 40,405,246 | 6.06 | 0.03 | 97.33 | 92.74 | 51.21 |
Gb, giga base pairs; GC_pct, percentage of guanine and cytosine of the four bases in clean reads; PBS, phosphate-buffered saline; hpi, hours post infection; SMA, Stenotrophomonas maltophilia.
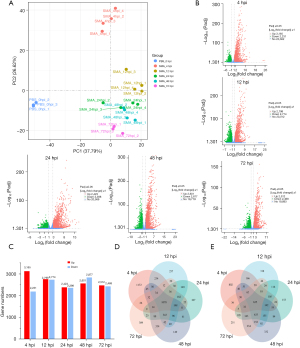
Table 3
Gene names | 4 hpi | 12 hpi | 24 hpi | 48 hpi | 72 hpi | |||||||||
---|---|---|---|---|---|---|---|---|---|---|---|---|---|---|
Padj | Log2FC | Padj | Log2FC | Padj | Log2FC | Padj | Log2FC | Padj | Log2FC | |||||
Cxcl10 | 0.00E+00 | 10.82 | 1.71E−139 | 7.89 | 4.97E−159 | 6.21 | 0.00E+00 | 8.34 | 1.05E−43 | 5.64 | ||||
Cd14 | 5.95E−287 | 7.01 | 4.00E−253 | 5.79 | 5.09E−70 | 5.27 | 3.62E−245 | 5.09 | 7.02E−94 | 4.03 | ||||
Gbp5 | 2.01E−282 | 5.77 | 9.55E−61 | 4.66 | 3.55E−43 | 3.32 | 1.02E−198 | 5.40 | 2.02E−12 | 3.54 | ||||
Marcksl1 | 1.65E−263 | 6.20 | 7.05E−150 | 5.84 | 5.34E−62 | 4.32 | 4.95E−187 | 5.02 | 1.38E−81 | 3.86 | ||||
Cxcr2 | 9.69E−250 | 6.81 | 5.40E−98 | 6.31 | 5.67E−84 | 6.24 | 2.10E−162 | 5.00 | 9.51E−74 | 4.99 | ||||
Pim1 | 2.34E−247 | 5.02 | 8.59E−114 | 4.36 | 1.02E−53 | 3.71 | 8.06E−109 | 3.76 | 1.76E−16 | 2.29 | ||||
Csrnp1 | 1.38E−239 | 5.58 | 8.13E−71 | 4.03 | 1.98E−46 | 3.34 | 3.81E−71 | 2.98 | 1.90E−11 | 1.62 | ||||
Zfp36 | 4.80E−238 | 4.84 | 1.90E−64 | 3.36 | 2.60E−15 | 2.30 | 3.17E−36 | 2.08 | 2.80E−09 | −1.45 | ||||
Ifi205 | 2.19E−218 | 6.19 | 2.40E−195 | 5.43 | 1.71E−86 | 4.34 | 6.64E−138 | 5.25 | 6.13E−43 | 3.47 | ||||
Junb | 6.61E−217 | 5.01 | 1.24E−113 | 4.45 | 4.32E−51 | 3.73 | 5.99E−111 | 3.42 | 1.96E−32 | 2.42 | ||||
Socs3 | 2.39E−216 | 5.84 | 4.09E−133 | 5.40 | 7.31E−66 | 4.68 | 2.01E−129 | 4.38 | 7.12E−43 | 3.14 | ||||
Tnip1 | 4.58E−216 | 3.78 | 5.73E−97 | 4.30 | 1.70E−70 | 3.75 | 4.45E−82 | 3.54 | 8.98E−49 | 2.94 | ||||
Zc3h12a | 4.02E−214 | 5.67 | 1.60E−180 | 5.54 | 2.08E−90 | 5.27 | 7.86E−121 | 4.31 | 2.70E−42 | 3.30 | ||||
Ifit1 | 1.06E−204 | 5.79 | 7.13E−79 | 3.55 | 1.10E−59 | 3.01 | 1.06E−163 | 3.52 | 7.49E−13 | 1.82 | ||||
Tnfaip3 | 7.19E−203 | 6.51 | 4.56E−200 | 6.29 | 1.17E−90 | 5.34 | 3.87E−199 | 4.95 | 6.84E−55 | 3.47 | ||||
Egr1 | 4.28E−202 | 5.48 | 1.63E−46 | 3.48 | 3.38E−25 | 3.14 | 1.06E−34 | 2.55 | 1.12E−07 | 1.54 | ||||
Sell | 3.11E−198 | 4.94 | 2.93E−97 | 3.46 | 6.50E−37 | 3.97 | 1.16E−178 | 4.43 | 4.16E−87 | 3.85 | ||||
Gbp2 | 2.37E−195 | 4.85 | 1.80E−62 | 4.02 | 5.56E−49 | 2.89 | 1.10E−251 | 4.98 | 1.81E−18 | 3.83 | ||||
Plek | 6.77E−194 | 4.97 | 3.19E−117 | 3.92 | 1.49E−35 | 3.41 | 3.11E−124 | 3.44 | 3.43E−35 | 2.53 | ||||
Mmp8 | 9.47E−194 | 7.21 | 3.82E−126 | 4.75 | 5.86E−30 | 5.03 | 1.29E−224 | 5.24 | 5.66E−93 | 5.29 |
FC, fold change; DEGs, differentially expressed genes; hpi, hours post infection; Padj, adjusted P value.
Time courses gene clustering
To investigate the dynamics of gene expression, we examined time-associated gene clusters of all genes from different time points using Mfuzz 2.58.0 (Figure 3A). In each cluster, the GO enrichment analysis is shown (Figure 3B). We found that the identified processes corresponded to the molecular pathophysiology of disease progression.
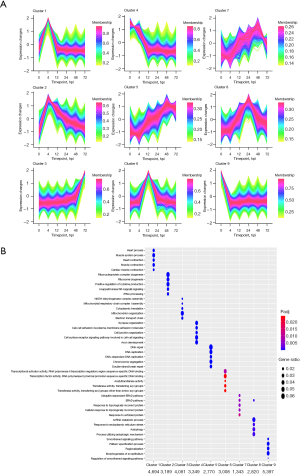
Gene expression in Cluster 1 and Cluster 2 rapidly peaked at 4 hpi and then declined. GO analysis showed that 4,694 genes in Cluster 1 were enriched for biological processes related to circulatory and muscular functions, including heart process, muscle system process, heart contraction, muscle contraction and cardiac muscle contraction. A total of 3,189 genes in Cluster 2 were enriched for biological processes related to the inflammatory signaling pathway and cytokine production, such as positive regulation of cytokine production, I-κB kinase (IKK)/NF-κB signaling, regulation of innate immune response and cytokine-mediated signaling pathway.
Gene expression levels in Clusters 5 and 7 began to rise immediately after infection. According to GO analysis, the 2,770 and 1,343 genes in Clusters 5 and 7, respectively, were primarily enriched for biological processes related to DNA replication and repair and protein repair, such as DNA repair, DNA replication, DNA-dependent DNA replication, chromosome segregation, double-strand break repair, ubiquitin-dependent endoplasmic reticulum-associated protein degradation (ERAD) pathway, ERAD pathway, response to topologically incorrect protein, cellular response to topologically incorrect protein, and response to unfolded protein.
After infection, the gene expression levels in Clusters 4 and 9 decreased. The 3,349 and 5,397 genes in Clusters 4 and 9, respectively, were mainly enriched for biological processes related to cell adhesion, cell junction, and smooth muscle cell contraction, such as cell-cell adhesion via plasma-membrane adhesion molecules, cell junction organization, smoothened signaling pathway, and smoothened signaling pathway regulation.
Cluster 6 gene expression peaked at 12 hours post-incubation. These 3,008 genes were enriched for biological processes related to transcriptional activity, such as transcriptional activator activity, RNA polymerase II transcription regulatory region sequence-specific DNA binding, transcription factor activity, and RNA polymerase II proximal promoter sequence-specific DNA binding, according to GO analysis.
Cluster 8 gene expression levels peaked at 24 hpi. GO analysis revealed that these 2,820 genes were enriched in biological processes related to noncoding RNA metabolic processes, response to endoplasmic reticulum stress (ERS), autophagy, processes utilizing autophagic mechanisms and the ERAD pathway.
WGCNA and enrichment analysis for WGCNA module genes
WGCNA hierarchical clustering was used to create dendrograms of 20 distinct modules of highly coexpressed genes; each module is colored differently, and genes with no cluster were found in the gray module (Figure 4A). The correlation between the infection process (hpi) and the module genes was then calculated (Figure 4B). Enrichment analysis was performed on modules with correlation coefficients greater than 0.9 to determine their importance in our study.
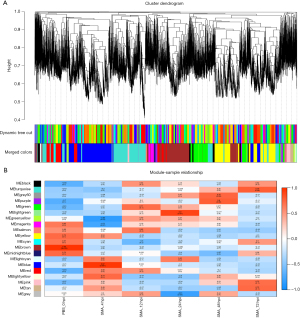
According to our module-trait association analysis, the blue module had a positive relationship with 4 hpi. According to GO analysis, this module was primarily enriched in muscle system processes, such as striated muscle contraction, myofibril assembly, muscle contraction, and cardiac muscle contraction. The main enriched pathways, according to KEGG analysis, were related to the IL-17 signaling pathway, the citrate cycle (TCA cycle), cardiac muscle contraction, tight junction, complement, and coagulation cascades, and the TNF signaling pathway (Figure 5A).

At 24 hpi, the light green module was critical. GO analysis revealed that this module was primarily enriched in ERS, including ERS response, endoplasmic reticulum unfolded protein response, cellular response to topologically incorrect protein, and response to unfolded protein. The main enriched pathways, according to KEGG analysis, were related to folate biosynthesis, amino sugar and nucleotide sugar metabolism, cell adhesion molecules, and endocytosis (Figure 5B).
Analysis of immune cell abundance
Using transcriptomic data, ImmuCellAI-mouse was used to assess the abundance of 36 immune cell types in lung tissue at different infection stages. ImmuCellAI-mouse was used to perform a hierarchical strategy to divide immune cell types into three layers. B cell, NK cell, and T cell are three of the lymphoid lineage cells in layer 1, and macrophage, DC, monocyte, and granulocyte are four of the myeloid lineage cells. Cells in layer 3 are subtypes of CD4 T and CD8 T cells, while cells in layer 2 are subtypes of cells in layer 1 (23). Figure 6A depicts abundant immune cell populations of various types in each sample. As shown in Figure 6B, regarding the levels of seven major immune cell types in layer 1, granulocytes, B cells, NK cells, and DCs showed an overall decreasing trend with time, while the levels of macrophages and monocytes showed an overall increasing trend. In layer 2, the abundance of 10 immune cell subtypes was examined further, and a significant increase in the levels of innate immune cells, including neutrophils, monocytes and macrophages, was found (Figure 6C). This suggests that innate immunity takes precedence after infection.
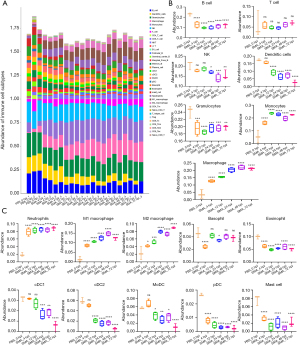
Validation of the transcriptome data
To validate the RNA-seq data, we randomly selected 9 DEGs, namely, Csf3, Fos, Fosl1, Ifng, Mmp9, Myo1f, Ncf1, Ptafr, and Tnfaip3, for qRT-PCR analysis at five different time points. The Gapdh gene was used as an internal standard. According to the qRT-PCR results, the 9 DEGs showed upregulated or downregulated expression patterns that were similar to the RNA-seq results (Figure 7). RNA-seq and qRT-PCR detected similar variations in the levels of DEGs.
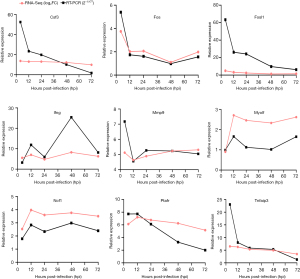
Discussion
In this study, C57BL/6 mice were challenged with 5×108 CFU/mL of SMA by aerosolized intratracheal inhalation, lung sections from challenged mice were subjected to H&E staining, and lung time-course transcriptome expression was analyzed by RNA-seq. A mouse model of acute lethal SMA pneumonia was successfully validated by aerosolized intratracheal inoculation. Using this model, we investigated, for the first time, changes in the time-course transcriptome profile of infected mouse lungs at different time points to better understand SMA lung infection and to identify genes or targets with research potential.
To avoid the drawback of using a single dataset per infection time, we used the temporal clustering of samples to group infection times that shared similar expression patterns. Multiple bioinformatics analysis methods, including Mfuzz time clustering, WGCNA, and ImmuCellAI-mouse, were used in this study to gain insight into the gene expression pattern during SMA pulmonary infection.
Transcriptome analysis revealed a rapid increase in the expression of genes encoding proinflammatory mediators (chemokines, cytokines, and transcription factors) early in the infection (4 hpi), indicating the initiation of an acute inflammatory response. In the Mfuzz analysis, gene expression levels for Cluster 2 peaked at 4 hpi, and GO analysis revealed increased gene enrichment for biological processes related to inflammatory signaling pathways, regulation of innate immune response, and cytokine production. The blue module, which was found to be strongly associated with the 4 hpi timepoint in WGCNA, was linked to inflammatory signaling pathways, such as the IL-17 signaling pathway, complement and coagulation cascades, and the TNF signaling pathway. Similarly, the abundance of innate immune cells determined during ImmuCellAI-mouse analysis increased at 4 hpi. IKK is primarily involved in inflammatory and innate immune responses, mediating the rapid response of recruited immune cells to inflammation and injury via NF-κB signaling (24). The NF-κB signaling pathways play a crucial role in inflammatory cytokine production as part of the innate immune system (25). GO analysis revealed that the blue module strongly associated with the 4 hpi timepoint was primarily enriched in muscle system processes; genes in Clusters 1 and 3 were mainly enriched in biological processes related to circulation and muscle function, as well as mitochondrial respiratory chain function. Energy is needed to fight infection and for both anti-inflammatory and proinflammatory immune responses (26). For energy to be supplied to the body, cardiac metabolism is essential (27). Energy is produced primarily through the mitochondrial respiratory chain. In the Mfuzz analysis, GO analysis of the expression levels of genes that peaked at 24 hpi revealed that these genes were enriched in biological processes associated with processes that respond to ERS, autophagy and the use of autophagic mechanisms. ERS and autophagy played an important role in infectious pneumonia. GO analysis linked the light green module, which was found to be strongly associated with 24 hpi in WGCNA, to ERS. Infections that cause inflammation are closely linked to ERS (28). In infected cells, autophagy occurs downstream of ERS signals (29). GO analysis showed that genes for biological processes enriched in Clusters 4 and 9, in which gene expression levels were reduced, were associated with cell adhesion, cell junctions and smooth muscle cell contraction. These findings suggest that intercellular adhesion may be compromised, which may be related to increased permeability of the alveolar-capillary barrier and the development of pulmonary edema.
In the immune cell infiltration analysis, the levels of neutrophils, monocytes, and macrophages were consistently increased after SMA infection. In response to bacterial infection, innate immune cells, such as neutrophils, monocytes, and macrophages become activated during sepsis, resulting in an inflammatory response and recruitment of immune cells into tissues (30). When neutrophils are overrecruited and activated following severe infection, the exaggerated inflammatory response can lead to severe tissue damage and result in organ failure (31). This was reflected in our pathological findings, which showed that after infection, damage to lung tissue structures occurred after lung immune cells were significantly activated and recruited. However, the number of NK cells decreased from 24 hpi. In contrast to the response to SMA infection, the levels of NK cells remain unchanged or increase during the majority of bacterial lung infections (32-35). When pathogens infiltrate the lungs, NK cells play a critical role in the immune response, and they are closely associated with fighting bacterial infections. The response to the host may be bidirectional (36,37). As a result, we hypothesize that SMA lung infection reduces the levels of NK cells, resulting in reduced inflammatory cell infiltration. The role of NK cells in lethal SMA pneumonia, which could lead to new therapeutic targets, warrants further investigation and clarification.
Several of the top 20 genes upregulated during infection are linked to gram-negative bacteria-induced inflammatory responses, while some are linked to innate immune responses. Cxcl10 encodes interferon gamma (IFN-γ) inducible protein 10 (IP-10), a neutrophil chemotactic factor (38). CD14 binds to monomeric lipopolysaccharides and transports them to the LY96/TLR4 complex, where they are processed and used to mediate the innate immune response to bacterial lipopolysaccharides (39). Gbp5, an activator of Nlrp3 inflammasome assembly, is involved in innate immunity and inflammation (40). CXCR2 binds to IL-8, CXCL3, growth regulated protein (GRO)/melanoma growth stimulatory activity (MGSA) and neutrophil-activating peptide 2 (NAP-2) with high affinity and plays an important role in neutrophil recruitment (41). EGR1, a nucleic zinc finger transcription factor, regulates inflammatory and immune responses (42). In a model of skin infection, phenol-soluble modulins (PSMs), produced and secreted by Staphylococcus aureus, were found to directly activate Egr1 and rapidly recruit leukocytes to the site of infection (43). The Sell gene encodes l-selectin, which is responsible for recruiting circulating leukocytes to endothelial inflammation sites (44). By hydrolyzing guanosine triphosphate (GTP) to guanosine monophosphate (GMP), Gbp2 increases oxidative killing and delivers antimicrobial peptides to autophagolysosomes (45). Given the continuing elevated expression levels and multiple functions of these genes in SMA-infected lungs, as well as the fact that they have not previously been reported in SMA pneumonia, they are likely to be suitable therapeutic targets for intervention in SMA-induced lung injury and warrant further investigation.
Conclusions
Our work is the first study to investigate the pulmonary transcriptional response to SMA infection. The findings suggest that SMA causes acute lethal inflammation in the lung that worsens over time, causing lung structure damage. NK cells are expected to be a new therapeutic target for SMA pneumonia after attention is drawn to their possible role in lethal SMA pneumonia. Cxcl10, Cd14, Gbp5, Cxcr2, Tnip1, Zc3h12a, Egr1, Sell, and Gbp2, which have consistently high or unreported levels of expression in SMA pneumonia, may be important targets for future research. And, the roles of infiltrated NK cells and mechanism of recruitment of NK cells in protecting host is worth of further study. Finally, our findings validate the findings of previous studies on SMA pneumonia while also providing new insights into SMA pneumonia that hold promise for the development of therapeutic approaches to reduce SMA lung inflammation.
Acknowledgments
Funding: None.
Footnote
Reporting Checklist: The authors have completed the ARRIVE reporting checklist. Available at https://jtd.amegroups.com/article/view/10.21037/jtd-23-1138/rc
Data Sharing Statement: Available at https://jtd.amegroups.com/article/view/10.21037/jtd-23-1138/dss
Peer Review File: Available at https://jtd.amegroups.com/article/view/10.21037/jtd-23-1138/prf
Conflicts of Interest: All authors have completed the ICMJE uniform disclosure form (available at https://jtd.amegroups.com/article/view/10.21037/jtd-23-1138/coif). The authors have no conflicts of interest to declare.
Ethical Statement: The authors are accountable for all aspects of the work in ensuring that questions related to the accuracy or integrity of any part of the work are appropriately investigated and resolved. Animal experiments were performed under a project license (No. IACUC-DWZX-2022-064) granted by Animal Care and Use Committee of the Academy of Military Medical Sciences, in compliance with institutional guidelines for the care and use of animals.
Open Access Statement: This is an Open Access article distributed in accordance with the Creative Commons Attribution-NonCommercial-NoDerivs 4.0 International License (CC BY-NC-ND 4.0), which permits the non-commercial replication and distribution of the article with the strict proviso that no changes or edits are made and the original work is properly cited (including links to both the formal publication through the relevant DOI and the license). See: https://creativecommons.org/licenses/by-nc-nd/4.0/.
References
- Brooke JS. Advances in the Microbiology of Stenotrophomonas maltophilia. Clin Microbiol Rev 2021;34:e0003019. [Crossref] [PubMed]
- Jian J, Xie Z, Chen L. Risk Factors for Mortality in Hospitalized Patients with Stenotrophomonas maltophilia Bacteremia. Infect Drug Resist 2022;15:3881-6. [Crossref] [PubMed]
- Wang N, Tang C, Wang L. Risk Factors for Acquired Stenotrophomonas maltophilia Pneumonia in Intensive Care Unit: A Systematic Review and Meta-Analysis. Front Med (Lausanne) 2021;8:808391. [Crossref] [PubMed]
- Imoto W, Yamada K, Yamairi K, et al. Clinical Characteristics of Rapidly Progressive Fatal Hemorrhagic Pneumonia Caused by Stenotrophomonas maltophilia. Intern Med 2020;59:193-8. [Crossref] [PubMed]
- Pek Z, Cabanilla MG, Ahmed S. Treatment refractory Stenotrophomonas maltophilia bacteraemia and pneumonia in a COVID-19-positive patient. BMJ Case Rep 2021;14:e242670. [Crossref] [PubMed]
- Imoto W, Yamada K, Kuwabara G, et al. In which cases of pneumonia should we consider treatments for Stenotrophomonas maltophilia? J Hosp Infect 2021;111:169-75. [Crossref] [PubMed]
- Puech B, Canivet C, Teysseyre L, et al. Effect of antibiotic therapy on the prognosis of ventilator-associated pneumonia caused by Stenotrophomonas maltophilia. Ann Intensive Care 2021;11:160. [Crossref] [PubMed]
- Xu G, Tang X, Shang X, et al. Identification of immunogenic outer membrane proteins and evaluation of their protective efficacy against Stenotrophomonas maltophilia. BMC Infect Dis 2018;18:347. [Crossref] [PubMed]
- Menetrey Q, Sorlin P, Jumas-Bilak E, et al. Achromobacter xylosoxidans and Stenotrophomonas maltophilia: Emerging Pathogens Well-Armed for Life in the Cystic Fibrosis Patients' Lung. Genes (Basel) 2021;12:610. [Crossref] [PubMed]
- Molloy K, Cagney G, Dillon ET, et al. Impaired Airway Epithelial Barrier Integrity in Response to Stenotrophomonas maltophilia Proteases, Novel Insights Using Cystic Fibrosis Bronchial Epithelial Cell Secretomics. Front Immunol 2020;11:198. [Crossref] [PubMed]
- Wu CM, Huang HH, Li LH, et al. Molecular Characterization of Three Tandemly Located Flagellin Genes of Stenotrophomonas maltophilia. Int J Mol Sci 2022;23:3863. [Crossref] [PubMed]
- Zgair AK, Chhibber S. Stenotrophomonas maltophilia flagellin restricts bacterial colonization in BALB/c mouse lung in vivo. FEMS Immunol Med Microbiol 2012;66:191-200. [Crossref] [PubMed]
- Fratoni AJ, Nicolau DP, Kuti JL. Minocycline pharmacodynamics against Stenotrophomonas maltophilia in the neutropenic murine infection model: implications for susceptibility breakpoints. J Antimicrob Chemother 2022;77:1052-60. [Crossref] [PubMed]
- Imoto W, Kaneko Y, Yamada K, et al. A mouse model of rapidly progressive fatal haemorrhagic pneumonia caused by Stenotrophomonas maltophilia. J Glob Antimicrob Resist 2020;23:450-5. [Crossref] [PubMed]
- Han P, Zhang W, Pu M, et al. Characterization of the Bacteriophage BUCT603 and Therapeutic Potential Evaluation Against Drug-Resistant Stenotrophomonas maltophilia in a Mouse Model. Front Microbiol 2022;13:906961. [Crossref] [PubMed]
- Hu X, Yu Y, Feng J, et al. Pathologic changes and immune responses against Coxiella burnetii in mice following infection via non-invasive intratracheal inoculation. PLoS One 2019;14:e0225671. [Crossref] [PubMed]
- Price A, Caciula A, Guo C, et al. DEvis: an R package for aggregation and visualization of differential expression data. BMC Bioinformatics 2019;20:110. [Crossref] [PubMed]
- Hisatomi A, Shiwa Y, Fujita N, et al. Identification and structural characterisation of a catecholate-type siderophore produced by Stenotrophomonas maltophilia K279a. Microbiology (Reading) 2021; Erratum in: Microbiology (Reading) 2021 doi: 10.1099/mic.0.001081. [Crossref]
- Li Q, Chen JX, Wu Y, et al. The mechanism of FZXJJZ decoction suppresses colorectal liver metastasis via the VDR/TGF-β/Snail1 signaling pathways based on network pharmacology-TCGA data-transcriptomics analysis. J Ethnopharmacol 2022;287:114904. [Crossref] [PubMed]
- Liu K, Chen S, Lu R. Identification of important genes related to ferroptosis and hypoxia in acute myocardial infarction based on WGCNA. Bioengineered 2021;12:7950-63. [Crossref] [PubMed]
- Miao YR, Zhang Q, Lei Q, et al. ImmuCellAI: A Unique Method for Comprehensive T-Cell Subsets Abundance Prediction and its Application in Cancer Immunotherapy. Adv Sci (Weinh) 2020;7:1902880. [Crossref] [PubMed]
- Di Bonaventura G, Pompilio A, Zappacosta R, et al. Role of excessive inflammatory response to Stenotrophomonas maltophilia lung infection in DBA/2 mice and implications for cystic fibrosis. Infect Immun 2010;78:2466-76. [Crossref] [PubMed]
- Miao YR, Xia M, Luo M, et al. ImmuCellAI-mouse: a tool for comprehensive prediction of mouse immune cell abundance and immune microenvironment depiction. Bioinformatics 2022;38:785-91. [Crossref] [PubMed]
- Barnabei L, Laplantine E, Mbongo W, et al. NF-κB: At the Borders of Autoimmunity and Inflammation. Front Immunol 2021;12:716469. [Crossref] [PubMed]
- Caetano-Silva ME, Rund LA, Vailati-Riboni M, et al. Copper-Binding Peptides Attenuate Microglia Inflammation through Suppression of NF-kB Pathway. Mol Nutr Food Res 2021;65:e2100153. [Crossref] [PubMed]
- Yuan Q, Zeng ZL, Yang S, et al. Mitochondrial Stress in Metabolic Inflammation: Modest Benefits and Full Losses. Oxid Med Cell Longev 2022;2022:8803404. [Crossref] [PubMed]
- Hu X, Ou-Yang Q, Wang L, et al. AdipoRon prevents l-thyroxine or isoproterenol-induced cardiac hypertrophy through regulating the AMPK-related pathway. Acta Biochim Biophys Sin (Shanghai) 2019;51:20-30. [Crossref] [PubMed]
- Sweet LA, Kuss-Duerkop SK, Keestra-Gounder AM. IRE1α-Driven Inflammation Promotes Clearance of Citrobacter rodentium Infection. Infect Immun 2022;90:e0048121. [Crossref] [PubMed]
- Cao X, Wan H, Wan H. Urolithin A induces protective autophagy to alleviate inflammation, oxidative stress, and endoplasmic reticulum stress in pediatric pneumonia. Allergol Immunopathol (Madr) 2022;50:147-53. [Crossref] [PubMed]
- Elemam NM, Ramakrishnan RK, Hundt JE, et al. Innate Lymphoid Cells and Natural Killer Cells in Bacterial Infections: Function, Dysregulation, and Therapeutic Targets. Front Cell Infect Microbiol 2021;11:733564. [Crossref] [PubMed]
- Burn GL, Foti A, Marsman G, et al. The Neutrophil. Immunity 2021;54:1377-91. [Crossref] [PubMed]
- Schuijs MJ, Png S, Richard AC, et al. ILC2-driven innate immune checkpoint mechanism antagonizes NK cell antimetastatic function in the lung. Nat Immunol 2020;21:998-1009. [Crossref] [PubMed]
- Pan Z, Xu ML. T-cell and NK-cell lymphomas in the lung. Semin Diagn Pathol 2020;37:273-82. [Crossref] [PubMed]
- Esaulova E, Das S, Singh DK, et al. The immune landscape in tuberculosis reveals populations linked to disease and latency. Cell Host Microbe 2021;29:165-178.e8. [Crossref] [PubMed]
- Witkowski M, Tizian C, Ferreira-Gomes M, et al. Untimely TGFβ responses in COVID-19 limit antiviral functions of NK cells. Nature 2021;600:295-301. [Crossref] [PubMed]
- Frank K, Paust S. Dynamic Natural Killer Cell and T Cell Responses to Influenza Infection. Front Cell Infect Microbiol 2020;10:425. [Crossref] [PubMed]
- Bergantini L, d'Alessandro M, Cameli P, et al. NK and T Cell Immunological Signatures in Hospitalized Patients with COVID-19. Cells 2021;10:3182. [Crossref] [PubMed]
- Li M, Chen Y, Li H, et al. Serum CXCL10/IP-10 may be a potential biomarker for severe Mycoplasma pneumoniae pneumonia in children. BMC Infect Dis 2021;21:909. [Crossref] [PubMed]
- Ciesielska A, Krawczyk M, Sas-Nowosielska H, et al. CD14 recycling modulates LPS-induced inflammatory responses of murine macrophages. Traffic 2022;23:310-30. [Crossref] [PubMed]
- Ding K, Li X, Ren X, et al. GBP5 promotes liver injury and inflammation by inducing hepatocyte apoptosis. FASEB J 2022;36:e22119. [Crossref] [PubMed]
- Pavlidis P, Tsakmaki A, Pantazi E, et al. Interleukin-22 regulates neutrophil recruitment in ulcerative colitis and is associated with resistance to ustekinumab therapy. Nat Commun 2022;13:5820. [Crossref] [PubMed]
- Banerji R, Saroj SD. Early growth response 1 (EGR1) activation in initial stages of host-pathogen interactions. Mol Biol Rep 2021;48:2935-43. [Crossref] [PubMed]
- Nguyen TH, Cheung GYC, Rigby KM, et al. Rapid pathogen-specific recruitment of immune effector cells in the skin by secreted toxins. Nat Microbiol 2022;7:62-72. [Crossref] [PubMed]
- Mocanu CA, Fuior EV, Voicu G, et al. P-selectin targeted RAGE-shRNA lipoplexes alleviate atherosclerosis-associated inflammation. J Control Release 2021;338:754-72. [Crossref] [PubMed]
- Zhang Y, Liao Y, Hang Q, et al. GBP2 acts as a member of the interferon signalling pathway in lupus nephritis. BMC Immunol 2022;23:44. [Crossref] [PubMed]