A new prognostic model of esophageal squamous cell carcinoma based on Cloud-least squares support vector machine
Highlight box
Key findings
• The Cloud-least squares support vector machine (LSSVM) model provides a clear prognostic superiority over the random forest, nomogram, and other models. This model is more advantageous in dealing with uncertain problems.
What is known and what is new?
• We know that entropy can be a measure of both the randomness of qualitative concepts as well as the value range of Cloud droplets allowed by qualitative concepts in the domain space of the Cloud model.
• The new model used the powerful learning and non-linear mapping abilities of the LSSVM. The penalty parameter C (Ex) and kernel function parameter (Entropy) were obtained by inverse normalization calculation of the Cloud parameters.
What is the implication, and what should change now?
• The popularization of the new model can improve the accuracy of esophageal squamous cell carcinoma prognosis, which is conducive to the choice of treatment for clinicians.
Introduction
Esophageal cancer is the eighth most common cancer in the world and ranks seventh in terms of mortality, and its histological types are mainly divided into esophageal squamous cell carcinoma (ESCC) and esophageal adenocarcinoma (EAC) (1-3). More than 80% of the world’s new cases and deaths occur in less developed regions. Histologically, approximately 90% of ESCC cases occur in high-incidence areas, with 60% of cases occurring in China. ESCC is characterized by high aggressiveness and poor prognosis. Despite treatment with a combination of surgery, radiotherapy, and chemotherapy, the 5-year survival rate is still less than 22% (4,5). The significant geographical variation in the incidence of esophageal cancer suggests that environmental and genetic factors may play an important role in the occurrence and development of esophageal cancer (6). The known risk factors for esophageal cancer include smoking and alcohol consumption, whereas fruit and vegetable intake has a high likelihood of preventing esophageal cancer (7-9). Currently, the tumor-node-metastasis (TNM) staging system is used to predict the prognosis of ESCC patients, but its clinical value is limited. Given that the clinical course of ESCC patients with the same clinical stage often varies greatly, a new ESCC prognosis system is needed for more accurate prognostic prediction, so as to achieve more targeted treatment and improve the prognosis of the disease (10,11).
The random forest algorithm and nomogram have not only been frequently used to predict the survival of patients with all types of cancer but also to successfully quantify risk prediction by illustrating important factors for tumor prognosis (12-14). Machine learning is widely used in cancer research, mainly in cancer diagnosis, image recognition, prognosis prediction and so on. While the results are encouraging, machine learning has its limitations, such as being less sensitive to missing data and simpler algorithms, which are usually used for text classification (15). The amount of data that neural networks need to process is too large to handle multi-dimensional data. Although the nomogram is simple and easy to use, it has defects in processing continuous variables (16-18). They cannot deal with uncertainty, and their accuracy needs to be improved. The characteristics of LSSVM in solving nonlinear and high-dimensional pattern recognition make us choose this method
Therefore, this study used the SEER and HCDC databases as the basis to explore the risk factors for patient prognosis and further establish and verify the Cloud-LSSVM prognostic model to assist clinical practice. We present this article in accordance with the TRIPOD reporting checklist (available at https://jtd.amegroups.com/article/view/10.21037/jtd-23-1058/rc).
Methods
Patient population
We extracted the ESCC cases from the SEER database (available at https://seer.cancer.gov/), and had obtained permission to obtain a research data file in the SEER database from the National Cancer Institute (reference number: 14574-Nov2019) (19,20). We used SEER*Stat Version 8.3.6 (Information Management Services, Inc., Calverton, MD, USA) to download all primary ESCC patient data from the SEER Database from 1973 to 2016 (21,22). The inclusion and exclusion criteria were as follows: (I) patients’ basic personal information is missing, such as age at diagnosis, Sex, race, etc.; (II) TNM stage, tumor size, and number of lymph nodes are unknown; and (III) pathological type was not adenocarcinoma or squamous cell carcinoma. Variables such as race, age, sex, city, tumor location, degree of differentiation, TNM, differentiation grade (well differentiated as differentiation grade I, moderately differentiated as differentiation grade II, poorly differentiated as differentiation grade III, and undifferentiated anaplastic as differentiation grade IV), histological type, tumor size, number of lymph nodes, and survival status and time were extracted and analyzed.
The overall survival estimate registered in the SEER database is the “cause-specific classification of death”, and stratified “dead (attributable to this cancer dx)” or “alive or dead of other cause”. Survival time was calculated from the diagnosis date to the date of death or last contact. The last contact, or the cut-off date of the study, was December 31, 2019, which was the last date of update on the follow-up time. The T, N, and M of all patients were staged according to the eighth edition of The American Joint Committee on Cancer (AJCC) esophageal cancer staging protocol. The patient exclusion and inclusion criteria are shown in Figure 1. The validation data were ESCC patients from 2003 to 2016 in China, Henan Provincial Center for Disease Control and Prevention, and the exclusion and inclusion criteria were the same as those applied for the SEER data. The study was conducted in accordance with the Declaration of Helsinki (as revised in 2013).
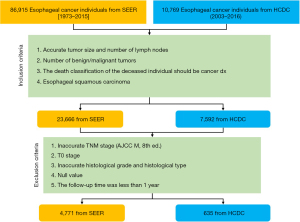
Statistical analysis
Firstly, we calculated the correlations and P values of each clinical characteristic. Next, the risk factors were analyzed by the log-rank method, and survival curves were drawn using the Kaplan-Meier method (23). Then, the significant risk factors in the univariate Cox analysis were introduced into the Cox proportional risk model for multivariate analysis, and the independent prognostic factors of esophageal cancer were obtained (21,24). The test standard was defined as P<0.01, which was considered statistically significant. Finally, the independent prognostic factors were used to construct the nomogram, random forest, and Cloud-LSSVM prognostic model of esophageal cancer (25-27). The C-index between the predicted probability and the actual outcome was calculated to judge the prognostic accuracy of the model. In this paper, C-index less than 0.65 was considered as low accuracy, and C-index more than 0.65 was considered as high accuracy. The Hmisc, Survival, RMS, Complex Heatmap, and other software packages of RStudio Version 1.1.463 (RStudio, Inc., Boston, MA, USA) were mainly utilized for the above calculation (28,29). The Cloud-LSSVM model employed libsvm and other software packages of MATLAB R2016a Version 9.2.341360 (MathWorks, Inc., Natick, MA, USA).
Establishment of the Cloud-LSSVM prognosis model
The factors affecting prognosis are generally uncertain, and the probability theory and fuzzy mathematics are insufficient to deal with the this problem. We hypothesized that the Cloud theory could solve this issue. Cloud is the model of the uncertain transition between the qualitative and quantitative concept that is described by language; it completely collects fuzziness and randomness together. The Cloud model can be reflected by its three characteristics: Expected value (Ex), Entropy (En), and Hyper-Entropy (He) (30). Two parameters, namely kernel function parameter σ and penalty parameter C, are required to determine the radial basis kernel function LSSVM that is commonly used in prediction. These two parameters have a considerable influence on LSSVM’s learning and generalization ability. The traditional parameter optimization method is not good at dealing with the uncertainty problem, but the optimization of the Cloud model least squares support vector machine (Cloud-LSSVM) solves this problem.
In this study, data distribution curves and the normal Cloud were obtained by Cloud transformation for factors affecting prognosis. Then, according to the reverse Cloud generator algorithm, the digital features of the Cloud (Ex, En and He) were obtained. In the Cloud model combined with LSSVM, the penalty parameter C is replaced by the Cloud model Ex, and the kernel parameter σ is replaced by entropy En. In this way, the new model can realize the unity of the randomness and fuzziness of the Cloud model and make use of the powerful learning and non-linear mapping abilities of LSSVM.
According to the Cloud transformation calculation method, the diagnostic age data was taken as an example. First, the data were normalized to [0,1] to obtain the data distribution curve of the diagnostic age, and then the maximum method Cloud transformation was carried out for the diagnostic age to obtain a normal Cloud, as shown in Figure 2A,2B. At the same time, according to the Cloud transformation calculation method, the Sex data showed two peaks in the distribution curve, so it had two Clouds (Figure 2C,2D).
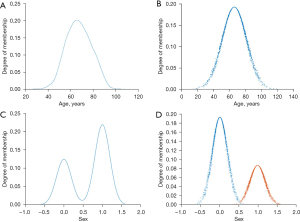
The penalty parameter C (Ex) and kernel function parameter (Entropy) were obtained by inverse normalization calculation of the Cloud parameters. Entropy can be a measure of both the randomness of qualitative concepts as well as the value range of Cloud droplets allowed by qualitative concepts in the domain space of the Cloud model. In this way, the new model can realize the unity of the randomness and fuzziness of the Cloud model. The new model used the powerful learning and non-linear mapping abilities of the LSSVM. In general, the higher the entropy, fuzziness, and randomness, the harder to quantify determinism.
Results
Univariate Cox analysis
According to the SEER database, which included 86,915 patients with esophageal cancer from 1973 to 2015, 14,708 patients with esophageal cancer were selected in strict accordance with the inclusion criteria. There were 9,550 cases of adenocarcinoma and 4,771 cases of squamous cell carcinoma. The overall median survival time was 14 months, the mean survival time was 26.5 months, and the 3-year survival rate was 65.8%. Univariate Cox analysis of the clinical factors showed that age at diagnosis (P<0.001), sex (P<0.001), race (P=0.001), differentiation grade (P<0.001), pathologic T category (P<0.001), regional lymph node (P<0.001), and pathologic M category (P<0.001) were associated with patient survival. The numbers of malignant tumors (P=0.078) and benign tumors (P=0.459) were not correlated with prognosis.
Meanwhile, according to the HCDC database, which included 10,769 patients with ESCC from 2003 to 2016, 635 patients were selected in strict accordance with the inclusion criteria. Univariate Cox analysis of clinical factors showed that age at diagnosis (P<0.001), differentiation grade (P<0.001), pathologic T category (P<0.001), regional lymph node (P<0.001), and sex (P=0.049) were associated with patient survival. The pathologic M category (P=0.093) and race were not correlated with prognosis (Table 1).
Table 1
Characteristics | SEER database | HCDC database | |||||||||||
---|---|---|---|---|---|---|---|---|---|---|---|---|---|
N | Univariate Cox analysis | Multivariate Cox analysis | N | Univariate Cox analysis | Multivariate Cox analysis | ||||||||
χ2 | P value | χ2 | P value | χ2 | P value | χ2 | P value | ||||||
Age at diagnosis (years) | 40.575 | <0.001 | 90.219 | <0.001 | 21.21 | <0.001 | 14.136 | 0.03 | |||||
<60 | 1,215 | 238 | |||||||||||
60–70 | 1,556 | 317 | |||||||||||
71–80 | 1,261 | 77 | |||||||||||
81–85 | 422 | 3 | |||||||||||
>85 | 317 | 0 | |||||||||||
Differentiation grade | 30.776 | <0.001 | 15.121 | <0.001 | 38.79 | <0.001 | 33.211 | <0.001 | |||||
I | 256 | 109 | |||||||||||
II | 1,968 | 308 | |||||||||||
III | 1,598 | 78 | |||||||||||
IV | 26 | 3 | |||||||||||
Other | 923 | 65 | |||||||||||
Pathologic T category | 42.5 | <0.001 | 30.489 | <0.001 | 49.44 | <0.001 | 21.126 | <0.001 | |||||
T1 | 1,503 | 125 | |||||||||||
T2 | 569 | 143 | |||||||||||
T3 | 1,809 | 328 | |||||||||||
T4 | 890 | 39 | |||||||||||
Pathologic N category | 27.798 | <0.001 | 0.37 | 0.543 | 37.62 | <0.001 | 31.824 | <0.001 | |||||
N0 | 2,154 | 384 | |||||||||||
N1 | 2,043 | 222 | |||||||||||
N2 | 457 | 24 | |||||||||||
N3 | 117 | 5 | |||||||||||
Pathologic M category | 444.688 | <0.001 | 388.414 | <0.001 | 2.81 | 0.093 | – | – | |||||
M0 | 3,831 | 633 | |||||||||||
M1 | 940 | 2 | |||||||||||
Race | 11.176 | 0.001 | 9.727 | 0.002 | – | – | – | – | |||||
Black | 1,181 | 0 | |||||||||||
White | 3,084 | 0 | |||||||||||
Other | 506 | 635 | |||||||||||
Sex | 53.451 | <0.001 | 36.647 | 0.001 | 3.861 | 0.049 | 3.662 | 0.056 | |||||
Female | 1,729 | 352 | |||||||||||
Male | 3,042 | 283 | |||||||||||
Malignant tumors | – | 3.1 | 0.078 | – | – | – | – | – | – | – | |||
Benign tumors | – | 0.548 | 0.459 | – | – | – | – | – | – | – |
ESCC, esophageal squamous cell carcinoma; SEER, Surveillance, Epidemiology, and End Results; HCDC Henan Provincial Center for Disease Control and Prevention.
Multivariate Cox analysis
Multivariate Cox analysis was performed for factors with statistically significant values according to the SEER database. The results showed that age at diagnosis (P<0.001), sex (P=0.001), race (P=0.002), differentiation grade (P<0.001), pathologic T category (P<0.001), and pathologic M category (P<0.001) were the factors affecting the prognosis of patients with ESCC. Pathologic N category (P=0.543) were not associated with prognosis. Meanwhile, multivariate Cox analysis was performed for factors with P<0.05 according to the HCDC database. The results showed that age at diagnosis (P=0.03), differentiation grade (P<0.001), and pathologic N category (P<0.001) were the factors affecting the prognosis of patients with ESCC. Sex (P=0.056) was not associated with prognosis (Table 1).
Further analysis was performed on the six significant factors obtained from the multivariate Cox analysis of the SEER database, and the survival curve and correlation coefficient graph were drawn, as shown in Figure 3. The results showed that the prognosis of the low-diagnostic age group was significantly better than that of the high-diagnostic age group (Figure 3A); the prognosis of differentiation grade I was better than that of grades II, III and IV, and the prognosis of grades II was better than that of grade III and IV (Figure 3B); and the prognosis of the pathologic T category was that T2 was better than T3, T3 was better than T1, and T1 was better than T4 (Figure 3C); the prognosis of females was better than that of males (Figure 3D). The prognosis of White people was better than that of Black people (Figure 3E); the prognosis of ESCC patients without the pathologic M category was better than that of ESCC patients with the pathologic M category (Figure 3F). Correlation analysis showed that age at diagnosis was associated with the pathologic T category, race was associated with the pathologic T category and pathologic M category, differentiation grade was not associated with the pathologic M category and sex, the other features were related (Figure 3G).
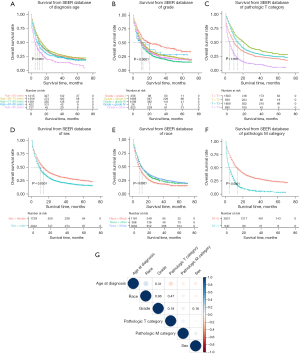
Uncertainty analysis
The multivariate Cox analysis showed that age at diagnosis, sex, race, differentiation grade, pathologic T category, and pathologic M category were the factors affecting the prognosis of patients with ESCC. Uncertainty analysis was performed for factors with multivariate Cox analysis results with P<0.01 according to the SEER database. The parameters after Cloud transformation are shown in Table 2, illustrating that there were two Clouds for race (Entropy =0.766), which also indicates that the fuzziness and randomness of the race data itself were the largest. Sex (Entropy =0.245) and age at diagnosis (Entropy =0.246) had the lowest entropy. Pathologic T category (Entropy =0.699) also showed great fuzziness and randomness. The parameters shows in Table 2 were substituted into the Cloud-LSSVM model, and the final prognosis result was obtained by weighting calculation.
Table 2
Factors | Cloud | Entropy | The penalty parameter C |
---|---|---|---|
Age at diagnosis | 1 | 0.246 | 1.971 |
Sex | 2 | 0.245 | 0.993 |
Race | 2 | 0.766 | 3.978 |
Differentiation grade | 4 | 0.295 | 1.97 |
Pathologic T category | 4 | 0.699 | 3.013 |
Pathologic M category | 2 | 0.257 | 0.993 |
Comparison of the discriminative ability of the prognostic prediction models containing different artificial intelligence algorithms
To further evaluate the predictive capacity and accuracy of Cloud-LSSVM models, likelihood ratio χ2, and Akaike information criterion (AIC) values were then calculated (Table 3). Higher C-index and likelihood ratio χ2 scores denoted better prognostic performance of the system; meanwhile, the lower the AIC value, the better the system (31,32). We also found that the likelihood liner trend χ2 values of the Cloud-LSSVM (857.43) were higher compared with those of the TNM stage, differentiation grade, random forest, and nomogram (265.8, 52.52, 37.75, and 665.45, respectively) in both cohorts. The AIC values were 52,520.26, 52,733.55, 17,705.76, 25,893, and 12,952.97 for the TNM stage, differentiation grade, random forest, nomogram, and Cloud-LSSVM in the SEER database, respectively. Regarding the validation cohort, the AIC values were 3,530.48, 3,591.95, 3,593.88, 3,119.95, and 2,981.26 for differentiation grade, random forest, nomogram, and Cloud-LSSVM in the HCDC database, respectively.
Table 3
Model | SEER database | HCDC database | |||||
---|---|---|---|---|---|---|---|
Likelihood ratio χ2 | AIC | C-index | Likelihood ratio χ2 | AIC | C-index | ||
TNM stage | 265.8 | 52,520.26 | 0.611 | 40.47 | 3,530.48 | 0.522 | |
Differentiation grade | 52.52 | 52,733.55 | 0.548 | 2.3 | 3,591.95 | 0.506 | |
Random forest | 37.75 | 17,705.76 | 0.649 | 0.37 | 3,593.88 | 0.498 | |
Nomogram | 665.45 | 25,893 | 0.659 | 68.92 | 3,119.95 | 0.563 | |
Cloud-LSSVM | 857.43 | 12,952.97 | 0.71 | 755.2 | 2,981.26 | 0.689 |
ESCC, esophageal squamous cell carcinoma; AIC, Akaike information criterion; C-index, Concordance index; SEER, Surveillance, Epidemiology, and End Results; HCDC, Henan Provincial Center for Disease Control and Prevention; TNM, tumor-node-metastasis; LSSVM, least squares support vector machine.
Furthermore, the C-index between the predicted probability and actual outcome was calculated. The C-index for predicting overall survival (OS) among the TNM stage, differentiation grade, random forest, nomogram, and Cloud-LSSVM were 0.611, 0.548, 0.649, 0.659, and 0.71 in the SEER database, respectively. As for the validation cohort, the C-index was 0.522 (TNM stage), 0.506 (differentiation grade), 0.498 (random forest), 0.563 (nomogram), and 0.689 (Cloud-LSSVM).
Discussion
Traditionally, clinical cancer prognosis study employed univariate and multivariate Cox analyses or nomograms to predict the survival status of patients. With the rise of artificial intelligence algorithms, random forest, neural networks, and other artificial intelligence algorithms have been introduced into cancer prognostic investigations; however, these algorithms often have limitations and cannot deal with uncertain factors (33). In our previous study, most of the screening of prognostic factors utilized univariate and multivariate Cox analyses. These analyses only study the effect of variables on survival but ignored the effect of the variables themselves, such as uncertainty and randomness (34).
In previous study, it was difficult for researchers to directly explore the effect between the independent and dependent variables due to the existence of many other variables that confound the relationship between them, and selective errors can be controlled and eliminated by propensity score matching (PSM) when dealing with the effects between variables (35). In this study, we found that pathologic T category and race have large fuzziness and randomness. The PSM algorithm cannot solve the errors caused by fuzziness and randomness, while the Cloud-LSSVM model in this study solved this problem.
According to the analysis of the SEER database in Table 1, race and pathologic M category were found to be important factors affecting prognosis. However, patients in the validation group from the HCDC data were all Asian people from China, which seriously interfered with the data. In addition, all the data were early-stage cases of ESCC, and there were only two patients with M1 and 653 patients with M0, so these two important factors could not be predicted by conventional methods. If prognostic algorithms such as nomograms and random forest are used, race and pathologic M category would be missing, thereby making the prediction results extremely inaccurate. There is a great deal of fuzziness and randomness in the race and pathologic T category (Table 2). The Cloud-LSSVM algorithm has a considerable advantage in solving these kinds of issues, and this result was also confirmed in the verification using the SEER and HCDC databases (Table 3). However, this model is limited by the limitations of the LSSVM algorithm itself and is not good at dealing with large samples.
In this study, the SEER data were divided into two parts, and 3,000 cases were randomly selected as the training set and 1,771 cases as the test set. The test results in the SEER database showed that the prognostic ability of Cloud-LSSVM was higher than that of other models. However, in the uncertain HCDC database test, the prognostic ability of Cloud-LSSVM was markedly higher than those of other models. Thus, it is proven that the Cloud-LSSVM model not only has higher prognostic ability than other machine learning models but also has more advantages in dealing with uncertain problems. In previous study, Cloud models have been applied to power system load forecasting and temperature prediction. This is the first time that Cloud models have been applied in the medical field (36). The results of this study indicate that this algorithm has broad development prospects for the prognostic evaluation of patients with ESCC.
Conclusions
In conclusion, we developed and validated a novel Cloud-LSSM model for predicting survival in ESCC patients. With the advantages of dealing with uncertain problems and ease of use, this Cloud-LSSM model offers clear prognostic superiority over the random forest, nomogram, and other models.
Acknowledgments
We would like to sincerely thank all of the participants of this research for their valuable time. We also thank Dr. Noriyuki Hirahara (Shimane University Faculty of Medicine, Shimane, Japan), Dr. Toshiro Iizuka (Tokyo Metropolitan Cancer and Infectious Disease Center, Komagome Hospital, Tokyo, Japan) for the critical comments and valuable advice on this study.
Funding: This work was supported by
Footnote
Reporting Checklist: The authors have completed the TRIPOD reporting checklist. Available at https://jtd.amegroups.com/article/view/10.21037/jtd-23-1058/rc
Peer Review File: Available at https://jtd.amegroups.com/article/view/10.21037/jtd-23-1058/prf
Conflicts of Interest: All authors have completed the ICMJE uniform disclosure form (available at https://jtd.amegroups.com/article/view/10.21037/jtd-23-1058/coif). The authors have no conflicts of interest to declare.
Ethical Statement: The authors are accountable for all aspects of the work in ensuring that questions related to the accuracy or integrity of any part of the work are appropriately investigated and resolved. The study was conducted in accordance with the Declaration of Helsinki (as revised in 2013).
Open Access Statement: This is an Open Access article distributed in accordance with the Creative Commons Attribution-NonCommercial-NoDerivs 4.0 International License (CC BY-NC-ND 4.0), which permits the non-commercial replication and distribution of the article with the strict proviso that no changes or edits are made and the original work is properly cited (including links to both the formal publication through the relevant DOI and the license). See: https://creativecommons.org/licenses/by-nc-nd/4.0/.
References
- Liang H, Fan JH, Qiao YL. Epidemiology, etiology, and prevention of esophageal squamous cell carcinoma in China. Cancer Biol Med 2017;14:33-41. [Crossref] [PubMed]
- Chen B, Liang S, Guo H, et al. OPN Promotes Cell Proliferation and Invasion through NF-κB in Human Esophageal Squamous Cell Carcinoma. Genet Res (Camb) 2022;2022:3154827. [Crossref] [PubMed]
- Katada C, Yokoyama T, Hirasawa D, et al. Curative Management After Endoscopic Resection for Esophageal Squamous Cell Carcinoma Invading Muscularis Mucosa or Shallow Submucosal Layer-Multicenter Real-World Survey in Japan. Am J Gastroenterol 2023;118:1175-83. [Crossref] [PubMed]
- Bray F, Ferlay J, Soerjomataram I, et al. Global cancer statistics 2018: GLOBOCAN estimates of incidence and mortality worldwide for 36 cancers in 185 countries. CA Cancer J Clin 2018;68:394-424. [Crossref] [PubMed]
- Gao SG, Yang JQ, Ma ZK, et al. Preoperative serum immunoglobulin G and A antibodies to Porphyromonas gingivalis are potential serum biomarkers for the diagnosis and prognosis of esophageal squamous cell carcinoma. BMC Cancer 2018;18:17. [Crossref] [PubMed]
- Cui MY, Yi X, Zhu DX, et al. Identification of Differentially Expressed Genes Related to the Lipid Metabolism of Esophageal Squamous Cell Carcinoma by Integrated Bioinformatics Analysis. Curr Oncol 2022;30:1-18. [Crossref] [PubMed]
- Lin W, Huang Y, Zhu L, et al. Pembrolizumab combined with paclitaxel and platinum as induction therapy for locally advanced esophageal squamous cell carcinoma: a retrospective, single-center, three-arm study. J Gastrointest Oncol 2022;13:2758-68. [Crossref] [PubMed]
- Sugimura K, Miyata H, Shinno N, et al. Prognostic Factors for Esophageal Squamous Cell Carcinoma Treated with Neoadjuvant Docetaxel/Cisplatin/5-Fluorouracil Followed by Surgery. Oncology 2019;97:348-55. [Crossref] [PubMed]
- Wu H, Yu J, Li Y, et al. Single-cell RNA sequencing reveals diverse intratumoral heterogeneities and gene signatures of two types of esophageal cancers. Cancer Lett 2018;438:133-43. [Crossref] [PubMed]
- Cao J, Yuan P, Wang L, et al. Clinical Nomogram for Predicting Survival of Esophageal Cancer Patients after Esophagectomy. Sci Rep 2016;6:26684. [Crossref] [PubMed]
- Higuchi T, Shoji Y, Koyanagi K, et al. Multimodal Treatment Strategies to Improve the Prognosis of Locally Advanced Thoracic Esophageal Squamous Cell Carcinoma: A Narrative Review. Cancers (Basel) 2022;15:10. [Crossref] [PubMed]
- Liu J, Ma X, Cao L, et al. Computational Drug Repurposing Approach to Identify Novel Inhibitors of ILK Protein for Treatment of Esophageal Squamous Cell Carcinoma. J Oncol 2022;2022:3658334. [Crossref] [PubMed]
- Sun Y, Wang J, Li Y, et al. Nomograms to predict survival rates for esophageal cancer patients with malignant behaviors based on ICD-0-3. Future Oncol 2019;15:121-32. [Crossref] [PubMed]
- Zhang WY, Chen XX, Chen WH, et al. Nomograms for predicting risk of locoregional recurrence and distant metastases for esophageal cancer patients after radical esophagectomy. BMC Cancer 2018;18:879. [Crossref] [PubMed]
- Lin Y, Tang M, Liu Y, et al. A narrative review on machine learning in diagnosis and prognosis prediction for tongue squamous cell carcinoma. Transl Cancer Res 2022;11:4409-15. [Crossref] [PubMed]
- Deng J, Weng X, Chen W, et al. A nomogram and risk classification model predicts prognosis in Chinese esophageal squamous cell carcinoma patients. Transl Cancer Res 2022;11:3128-40. [Crossref] [PubMed]
- Lian L, Teng SB, Xia YY, et al. Development and verification of a hypoxia- and immune-associated prognosis signature for esophageal squamous cell carcinoma. J Gastrointest Oncol 2022;13:462-77. [Crossref] [PubMed]
- Liu C, Han J, Han D, et al. A new risk score model based on lactate dehydrogenase for predicting prognosis in esophageal squamous cell carcinoma treated with chemoradiotherapy. J Thorac Dis 2023;15:2116-28. [Crossref] [PubMed]
- Danese M, Gricar J, Abraham P. Real-world outcomes with second-line therapy in advanced esophageal squamous cell carcinoma using SEER-Medicare data. Future Oncol 2022;18:927-36. [Crossref] [PubMed]
- He H, Chen N, Hou Y, et al. Trends in the incidence and survival of patients with esophageal cancer: A SEER database analysis. Thorac Cancer 2020;11:1121-8. [Crossref] [PubMed]
- Tian D, Li HX, Yang YS, et al. The minimum number of examined lymph nodes for accurate nodal staging and optimal survival of stage T1-2 esophageal squamous cell carcinoma: A retrospective multicenter cohort with SEER database validation. Int J Surg 2022;104:106764. [Crossref] [PubMed]
- Tang X, Zhou X, Li Y, et al. A Novel Nomogram and Risk Classification System Predicting the Cancer-Specific Survival of Patients with Initially Diagnosed Metastatic Esophageal Cancer: A SEER-Based Study. Ann Surg Oncol 2019;26:321-8. [Crossref] [PubMed]
- Wang S, Guan X, Ma M, et al. Reconsidering the prognostic significance of tumour deposit count in the TNM staging system for colorectal cancer. Sci Rep 2020;10:89. [Crossref] [PubMed]
- Dai D, Shi R, Wang Z, et al. Competing Risk Analyses of Medullary Carcinoma of Breast in Comparison to Infiltrating Ductal Carcinoma. Sci Rep 2020;10:560. [Crossref] [PubMed]
- Zang Z, Liu Y, Wang J, et al. Dietary patterns and severity of symptom with the risk of esophageal squamous cell carcinoma and its histological precursor lesions in China: a multicenter cross-sectional latent class analysis. BMC Cancer 2022;22:95. [Crossref] [PubMed]
- Kitasaki N, Hamai Y, Emi M, et al. Prognostic Factors for Patients With Esophageal Squamous Cell Carcinoma After Neoadjuvant Chemotherapy Followed by Surgery. In Vivo 2022;36:2852-60. [Crossref] [PubMed]
- Yu Y, Sun C, Zhang B, et al. Temperature prediction based on cloud model RBF neural network data center. Journal of Shenyang Ligong University 2013;32:9-14.
- Yang X, Zhu H, Qin Q, et al. Genetic variants and risk of esophageal squamous cell carcinoma: a GWAS-based pathway analysis. Gene 2015;556:149-52. [Crossref] [PubMed]
- Balachandran VP, Gonen M, Smith JJ, et al. Nomograms in oncology: more than meets the eye. Lancet Oncol 2015;16:e173-80. [Crossref] [PubMed]
- Liu F. Interactive Music Learning Model Based on RBF Algorithm. Comput Intell Neurosci 2022;2022:5759986. [Crossref] [PubMed]
- Shiozaki H, Slack RS, Chen HC, et al. Metastatic Gastroesophageal Adenocarcinoma Patients Treated with Systemic Therapy Followed by Consolidative Local Therapy: A Nomogram Associated with Long-Term Survivors. Oncology 2016;91:55-60. [Crossref] [PubMed]
- Liu K, Jiao YL, Shen LQ, et al. A Prognostic Model Based on mRNA Expression Analysis of Esophageal Squamous Cell Carcinoma. Front Bioeng Biotechnol 2022;10:823619. [Crossref] [PubMed]
- Qiu J, Peng B, Tang Y, et al. CpG Methylation Signature Predicts Recurrence in Early-Stage Hepatocellular Carcinoma: Results From a Multicenter Study. J Clin Oncol 2017;35:734-42. [Crossref] [PubMed]
- Gao S, Liu Y, Duan X, et al. Porphyromonas gingivalis infection exacerbates oesophageal cancer and promotes resistance to neoadjuvant chemotherapy. Br J Cancer 2021;125:433-44. [Crossref] [PubMed]
- Gao S, Liu K, Jiao Y, et al. Selective activation of TGFβ signaling by P. gingivalis-mediated upregulation of GARP aggravates esophageal squamous cell carcinoma. Am J Cancer Res 2023;13:2013-29.
- Jiang B, Liu D, Karimi HR, et al. RBF Neural Network Sliding Mode Control for Passification of Nonlinear Time-Varying Delay Systems with Application to Offshore Cranes. Sensors (Basel) 2022;22:5253. [Crossref] [PubMed]
(English Language Editor: A. Kassem)