Gene modules and genes associated with postoperative atrial fibrillation: weighted gene co-expression network analysis and circRNA-miRNA-mRNA regulatory network analysis
Highlight box
Key findings
• Weighted gene co-expression network analysis (WGCNA) identified 2 key gene modules and 44 key genes that were significantly related to postoperative atrial fibrillation (POAF). A circular RNA (circRNA)-microRNA (miRNA)-messenger RNA (mRNA) network associated with POAF was also obtained.
What is known and what is new?
• The pathogenesis of POAF is elusive, and relevant research has been sparse. KLF10 is a transcriptional factor and is crucial in cell proliferation, inflammation, and apoptosis. miR-19b and miR-30a are critical to the electrical and structural remodeling of AF.
• WGCNA analysis indicated that the key genes in POAF involving in endosomal transport, PKB signaling, and the transcription regulation biological processes. The circRNA-miRNA-mRNA network revealed that 2 novel circRNAs, hsa_circRNA_001654 and hsa_circRNA_005899, downregulated hsa-miR-19b-3p and hsa-miR-30a-5p, respectively, and finally targeted KLF10.
What is the implication, and what should change now?
• Our study provides novel insights into the underlying the pathogenesis of POAF. More relevant basic research is needed to further verify our findings.
Introduction
Atrial fibrillation (AF) is one of the most common complications in patients undergoing cardiac surgery (1). The incidence of postoperative AF (POAF) peaking within 5 days following surgery varies from approximately 20–50% depending on the procedure performed and baseline clinical conditions (2). Although POAF is mostly temporary, it is highly associated with high morbidity and mortality based on high risk of heart failure, ischemic stroke, and AF recurrence in long-term follow-up (3-6). Recently, increasing attention has been paid to the management and prognosis of patients with POAF (7,8); however, its pathogenesis has not been elucidated yet. The comprehensive exploration of the pathology and genetic etiology of POAF may provide candidate targets for understanding the underlying mechanism and subsequent treatment.
Weighted gene co-expression network analysis (WGCNA) is a novel, advanced bioinformatics methodology used for identifying critical genes and exploring molecular mechanisms. It can construct gene coexpression networks, calculate the adjacency of genes, screen out hub gene modules, and evaluate the associations with specific clinical features. WGCNA has been widely used in various diseases, such as in cancers (9,10), coronary artery disease, and heart failure (11,12). Currently, there is a paucity of data utilizing WGCNA to identify key modules or genes related to POAF.
Circular RNA (circRNA), a stable circular structure, has recently been shown to play a crucial role in cardiovascular diseases, particularly congenital heart disease, dilated cardiomyopathy, myocardial infarction, and heart failure (13-15). Unlike linear RNAs with 5'-end caps and 3'-end tails, circRNAs are characterized by covalently closed loop structures that are considered more stable and conserved. CircRNAs can act as microRNA (miRNA) sponges, competing for endogenous RNAs and participating in the process of transcription, translation, and even epigenetic regulation. Emerging data have supported the pathophysiological significance of circRNA and the circRNA-miRNA-messenger RNA (mRNA) regulatory network, basing on competitive endogenous RNA (ceRNA), in AF. One recent study proposed that circRNAs might have potential predictive values for POAF and extracted the circRNAs as the biomarkers for POAF through machine learning algorithms (16). However, few studies have explored the role of circRNA-miRNA-mRNA networks in POAF.
Therefore, the present study was conducted to identify key gene modules and genes and to conduct a circRNA-miRNA-mRNA regulatory network analysis of POAF on the basis of bioinformatic analysis. We present this article in accordance with the STREGA reporting checklist (available at https://jtd.amegroups.com/article/view/10.21037/jtd-23-1179/rc).
Methods
Microarray data
The gene expression profiling data sets GSE143924 and GSE97455 were obtained from the Gene Expression Omnibus (GEO; https://www.ncbi.nlm.nih.gov/geo/). GSE143924 was based on the GPL25483 (platform of Affymetrix Human Gene 2.0 ST Array) and comprised of 30 epicardial adipose tissue samples including 15 POAF patients and 15 non-POAF patients. GSE97455 was established on GPL21825 (074301 Arraystar Human CircRNA microarray V2), which was generated from 15 POAF plasma samples and 15 non-POAF plasma samples. The raw data in CEL files were subjected to background correction, log2 transformation, and normalization with the “limma” package in R software (version 3.6.3; The R Foundation for Statistical Computing). The study was conducted in accordance with the Declaration of Helsinki (as revised in 2013).
WGCNA analysis and key gene module identification
After preprocessing and normalizing were completed, a set of 8,596 genes from the GSE143924 data set was evaluated for the WGCNA. The “WGCNA” package in R software was applied to construct the gene co-expression network to identify key gene modules and genes related to POAF. To obtain a scale-free topology of the adjacency matrix network, the soft-thresholding power ranging from 1 to 20 was tested, and an appropriate power (β) of 3 was chosen, as it met the scale independence of 0.85 with the minimum power value. The adjacency matrix was transformed into a topological overlap matrix (TOM) to measure gene connectivity in the network. Gene modules were calculated via hierarchical clustering analysis according to the TOM-based dissimilarity measure with the setting cutoff as 0.25, and the minimum module size of 100 genes (17). Module eigengenes (MEs) were considered as the first principal component of each module. Gene significance (GS) was defined as the mediated P value of each gene in the linear regression between gene expression and the clinical traits. Module significance (MS) was computed with the average absolute GS of all the genes involved in the module. After division into different gene modules was completed, ME, GS, and MS were calculated, using the dynamic tree cut algorithm. The module-trait relationship was determined as the correlation between MEs and clinical traits and displayed via a heatmap. Key modules were selected according to the MS score and the correlation between MEs and clinical traits. Candidate genes in the selected modules were then filtered by the weight score and exported into Cytoscape software (3.9.1). The key genes were further assessed and visualized with the Cytoscape plugin, MCODE (18). Utilizing the MCODE plugin in Cytoscape for clustering, we opted for the cluster with the highest score to compare its genes with the module candidate genes and to identify the overlapping genes as key genes for POAF.
Identification of differentially expressed circRNAs (DECs) and target prediction
The DECs in the GSE97455 data set were screened between POAF patients and non-POAF patients in the preprocessed data. The genes with |log2 fold change (FC)| >1 and adjusted P value <0.05 were considered to be differentially expressed. A volcano plot of the DECs was generated using the “pheatmap” package in R software.
The target miRNAs based on the DECs were predicted with both circBank (http://www.circbank.cn/) and miRanda. Additionally, the AF-related miRNAs were searched in the Human microRNA Disease Database (HMDD; http://www.cuilab.cn/hmdd), a comprehensive database of miRNA-disease associations. A total of 74 AF-related miRNAs were extracted and are listed in Table S1. The overlapping miRNAs between the DECs target miRNAs and the AF-related miRNAs were visualized with Venn diagram analysis. Three different databases were used for further miRNA target prediction, including miRTarBase (https://mirtarbase.cuhk.edu.cn/~miRTarBase/miRTarBase_2022/php/index.php), TargetScan (https://www.targetscan.org/vert_80/), and miRDB (https://mirdb.org/), to which the above-mentioned overlapping miRNAs were uploaded. Only the mRNAs verified with all 3 databases were selected for further analysis.
Functional enrichment analysis
Gene Ontology (GO) and Kyoto Encyclopedia of Genes and Genomes (KEGG) pathway analyses were conducted to investigate the functions of the screened genes using Database for Annotation, Visualization, and Integrated Discovery (DAVID; 2021; https://david.ncifcrf.gov/). Molecular function (MF), cellular composition (CC), and biological process (BP) were all carried out in GO enrichment analysis.
Construction of the circRNA-miRNA-mRNA network
The circRNA-miRNA-mRNA regulatory network was constructed with Cytoscape software. The circRNAs and miRNAs in the network were screened as described above. The threshold of differentially expressed genes (DEGs) in GSE143924 was set as |log2FC| >0.5 and P value <0.05. The candidate mRNAs for network construction were selected from the overlap between the above-mentioned DEGs and the predicted miRNA target genes. The network was then visualized in Cytoscape.
Results
Weighted coexpression network construction
After data preprocessing, the expression profile of 8,596 genes was acquired in the GSE143924 data set including 15 POAF samples and 15 non-POAF samples. All genes were subjected to coexpression network analysis. A sample dendrogram is presented in Figure 1A with 2 different clinical statuses, POAF or non-POAF. To ensure a scale-free network, the soft-thresholding power was picked as 3 (R2=0.9) after both the scale-independence and mean connectivity were balanced (Figure 1B). The adjacency matrix and TOM were each calculated. As shown in Table S2 and Figure 2A, 13 modules were obtained on the basis of the dissimilarity measure (1−TOM). Among them, the brown module (r=−0.38; P=0.04) and the magenta module (r=−0.39; P=0.04) were significantly related with POAF (Figure 2B). Consequently, these 2 modules were regarded as candidate modules for further analysis.
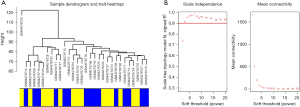
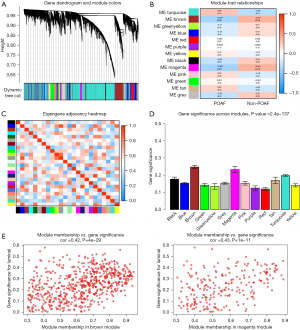
Key gene modules and gene identification
An obvious independence among the 13 gene modules was estimated with an eigengene adjacency heatmap (Figure 2C), and the MS scores were also compared (Figure 2D). In addition to the higher correlation with POAF, the MS score in the brown and magenta modules was also higher than that in the other 11 modules. Therefore, these 2 modules, the brown and magenta modules, were delineated as the key gene modules for POAF. A scatter plot of the module membership (MM) versus GS is presented in Figure 2E. A total of 109 hub genes in the brown module and 21 hub genes in the magenta module with |MM| >0.8 and |GS| >0.2 were selected. Genes in the 2 modules were explored using the MCODE algorithm. Two clusters were identified in the brown module, and the one with the highest score was chosen; meanwhile, only one significant cluster was detected in the magenta module. As shown in Figure 3A,3B, 38 nodes and 630 edges were selected in the brown module while 14 nodes and 79 edges were selected in the magenta module. The nodes were extracted as candidate genes and were further compared with the hub genes to determine the overlapping genes that were the key genes of POAF. As shown in Figure 3C,3D, 34 key genes were found in the brown module and 10 in the magenta module.
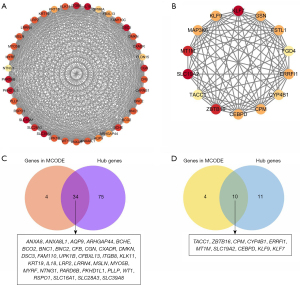
GO and KEGG analyses were performed on the key genes in the 2 key gene modules, as presented in Figure 4. Unfortunately, there was no relevant KEGG pathway concerning the key genes. Key genes in the brown module were found to be related to endosomal transport (GO:0016197), regulation of phospholipase A2 activity (GO:1900138), and nucleobase transport (GO:0015855, GO:1904823) in BP; cell junction (GO:0030054), plasma membrane (GO:0016323, GO:0005886), and extracellular exosome (GO:0070062) in cell component (CC); and purine nucleobase transmembrane transporter activity (GO:0005345), carboxylic acid transmembrane transporter activity (GO:0046943), ribosomal DNA binding (GO:0000182), transcription regulatory region sequence-specific DNA binding (GO:0000976), and calcium-dependent phospholipid binding (GO:0005544) in MF. For the magenta module, the regulation of transcription from RNA polymerase II promoter (GO:0006357) was chiefly involved in BP, nucleus (GO:0005634) in CC, and polymerase II core promoter proximal region sequence-specific DNA binding (GO:0000978) in MF.
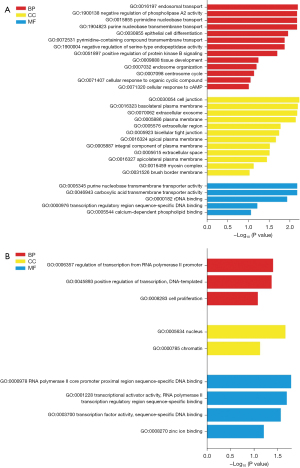
DECs and target miRNA identification
A total of 55 circRNAs, including 34 upregulated and 21 downregulated, were identified as DECs in the comparative analysis of the GSE97455 data set. The volcano plot and heatmap plot of DECs between POAF and non-POAF patients are presented in Figure 5. The potential miRNA targets of DECs were first predicted by both circBank and miRanda and then validated by using the AF-related miRNAs from HMDD (Table S1). Ultimately, 11 overlapping target miRNAs were identified (Table 1, Figure S1).
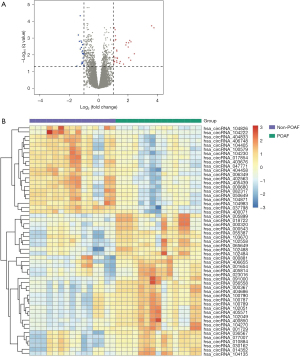
Table 1
miRNAs | circRNAs† |
---|---|
hsa-miR-19b-3p | hsa_circRNA_001654 |
hsa-miR-183-5p | hsa_circRNA_010884 |
hsa-miR-199a-3p | hsa_circRNA_100790 |
hsa-miR-199a-5p | hsa_circRNA_104135 |
hsa-miR-199b-3p | hsa_circRNA_100790 |
hsa-miR-19a-3p | hsa_circRNA_001654, hsa_circRNA_056558 |
hsa-miR-30a-5p | hsa_circRNA_005899 |
hsa-miR-3149 | hsa_circRNA_000543 |
hsa-miR-3171 | hsa_circRNA_104405 |
hsa-miR-377-5p | hsa_circRNA_001654 |
hsa-miR-466 | hsa_circRNA_001654 |
†, the circRNAs were acquired from the differential expression analysis of GSE97455 dataset. miRNA, microRNA; circRNA, circular RNA.
To predict the mRNAs, the aforementioned 11 miRNAs were searched for in the miRTarBase, TargetScan, and miRDB databases, with 452 mRNAs were recognized by all 3 databases. The biological function of the 452 predicted mRNAs was subsequently scrutinized, and the top 10 results of GO and KEGG pathways analyses are displayed in Figure S2. Transcription regulation from RNA polymerase II promoter (GO:0045944) and protein phosphorylation (GO:0006468) were significantly enriched in BP terms; cytosol (GO:0005829), nucleoplasm (GO:0005654), and cytoplasm (GO:0005737) were significantly enriched in CC; and protein binding (GO:0005515) and DNA binding (GO:0003700) were significantly enriched in MF. Additionally, the most significantly involved KEGG pathways were cellular senescence (has04218) and FoxO signaling pathway (hsa04068).
The circular RNA-miRNA-mRNA regulatory network construction
The target mRNAs were further ascertained via the overlap between the 452 predicted mRNAs and the differentially expressed mRNAs in the GSE143924 data set (Figure 6A). Overall, 3 mRNAs, ZFAND5, SOX6, and KLF10, were identified. Similar patterns of expression between the DECs and DEGs were selected as the miRNA-sponge effect of circRNA. Only KLF10 and its interaction circRNA-miRNAs were further selected for circRNA-miRNA-mRNA network construction, as illustrated in Figure 6B.
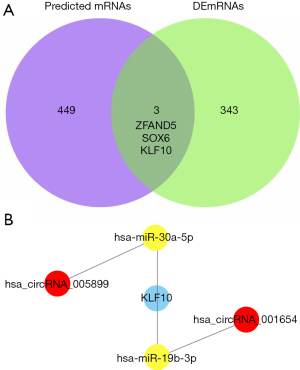
Discussion
The pathogenesis of POAF is complex and poorly understood. Similar to nonsurgical AF, POAF is likely caused by vulnerable triggers and existing substrate (1). The transient triggers mainly include postoperative inflammation, autonomic dysfunction, and oxidative stress (4,19). Both white blood cells and inflammatory markers, for example interleukin 1 (IL-1) and IL-6, are elevated in patients with POAF (1). Epicardial adipose tissue-mediated IL-1β levels have also been reported to be associated with the occurrence of POAF, which may be mediated through the activation of NLRP3 inflammasome (20). It has been found that atrial cardiomyocyte NLRP3 inflammasome activation could be an underlying mechanism in POAF (21). In addition, multiple genetic and epigenetic processes involving transcription factors, noncoding RNAs, and DNA methylation have been acknowledged to contribute to AF (22). The key transcription factors in AF include GATA4, GATA6, NKX2-5, and TBX5 (23). Moreover, miR-1 and miR-133A have been confirmed to regulate cardiomyocyte apoptosis in POAF (24). Furthermore, a recent study demonstrated that DNA methylation may be highly correlated with the incidence of POAF (25).
However, the underlying mechanism of POAF remains elusive, and research to this end has thus far been insufficient. Because of the limited data released in GEO database, we used 2 available data sets, GSE143924 and GSE97455, for further exploring POAF by means of bioinformatic analysis.
In our study, WGCNA was applied to identify the gene modules and genes. After constructing the gene co-expression network, we found 2 key gene modules that were significantly related with POAF. A total of 44 key genes that overlapped between the hub genes and genes in the significant cluster of the key gene module were selected. Functional enrichment analysis of those key genes pointed to the following BPs: endosomal transport, protein kinase B (PKB/AKT) signaling and transcription regulation. It is widely acknowledged that channel trafficking via endosomal transport is an important cause of arrythmias (26). Additionally, the PKB/AKT signaling is closely crosstalked with nuclear factor κB (NF-κB) which can be stimulated by AngII and is independently associated with POAF (27). Higher NF-κB protein levels trigger the activation of NLRP3 inflammasome (28). Also, phospholipase A2 activity has been reported positively associated with AF (29). The negative regulation of phospholipase A2 activity shown in our functional enrichment analysis (Figure 4A) seems to be contradictory to this. However, our findings may be due to the limited number of key genes found in our analysis.
The circRNA-miRNA-mRNA regulatory network constructed in this study suggested that KLF10 may figure prominently in POAF. KLF10, also known as TGF-beta inducible early gene 1 (TIEG1), is a transcriptional factor and plays a critical role in TGFβ-mediated cell proliferation, inflammation, and apoptosis (30). Prior research with KLF10 demonstrated that TIEG1−/− knockout mice developed features of cardiac hypertrophy (31). In addition, Li et al. (32) demonstrated that KLF10 can suppress Ang II-induced hypertrophic cardiomyocytes through the GATA4 signal pathway. Additionally, KLF10 was reported to induce apoptosis and inhibit proliferation through PTEN/AKT signaling in cardiac myocytes (33). Therefore, KLF10 may be a novel target for further investigation into understanding the underlying mechanism of POAF.
Noncoding RNAs, especially miRNAs, have been verified to participate in the electrical and structural remodeling of AF. Yamac et al. (34) discovered that the decreased expression level of miR-199a with subsequent activation of the SIRT1 protein could induce oxidative stress, thus contributing to POAF. Genome-wide association study (GWAS) has identified the high susceptibility of Paired-like homeodomain transcription factor 2 (PITX2) to AF (35). A study by Wang et al. (36) confirmed that Pitx2 could positively regulate miRNAs, including miR-19b which inhibits the predisposition to AF. In our results, hsa_circRNA_001654 was screened as the DECs (Figure 5B) which was significantly upregulated in the condition of POAF. KLF10 was also significantly upregulated in the condition of POAF as shown in Figure 6A. In Figure 6B, hsa_circRNA_001654-miR-19b-KLF10 was predicted to participate in the circRNA-miRNA-mRNA network. Therefore, those findings may hint that the downregulation of miR-19b could promote the occurrence of POAF, which was in line with the results by Wang. Furthermore, as depicted in Figure 6B, miR30a was found to be associated with POAF. Besides, miR-30a was also reported to induce myocardial fibrosis and promote AF via targeting the Snail 1 protein (37). In addition, T-box transcription factor 5 (TBX5) is an important transcription factor in AF. Wang et al. (38) showed miR-30a could downregulate TBX5 expression. Their zebrafish model assays demonstrated the interaction of miR-30a and TBX5 3'-UTR, which also support the role of miR-30a in AF.
Our study has several limitations worth discussing. First, more clinical variables could be added to the WGCNA, as there was limited clinical information provided by the data sets. Second, only 1 circRNA dataset was available for POAF in GEO up to now that we utilized for differential expression analysis. Third, different sample types from different data sets might have biased our results, and clinical samples are needed for verification. Fourth, a larger sample size would have been preferable and probably more informative. Importantly, our findings should be validated with future in vivo and ex vivo experiments.
Conclusions
This study was the first to apply WGCNA and investigate the circRNA-miRNA-mRNA regulatory networks in POAF. Ultimately, 2 key gene modules and 44 key genes were discovered that were mainly involved in endosomal transport, PKB/AKT signaling, and transcription regulation during functional enrichment analysis. In addition, the circRNA-miRNA-mRNA regulatory network, including 2 circRNAs, 2 miRNAs, and 1 mRNA, provided novel insights into the underlying pathogenesis of AF. However, further research is needed for a more comprehensive exploration.
Acknowledgments
Funding: This study was supported by
Footnote
Reporting Checklist: The authors have completed the STREGA reporting checklist. Available at https://jtd.amegroups.com/article/view/10.21037/jtd-23-1179/rc
Peer Review File: Available at https://jtd.amegroups.com/article/view/10.21037/jtd-23-1179/prf
Conflicts of Interest: All authors have completed the ICMJE uniform disclosure form (available at https://jtd.amegroups.com/article/view/10.21037/jtd-23-1179/coif). JDP receives research funding via a grant from KSQ Therapeutics, Inc. and is on the Board of Directors for the non-profit Thoracic Surgery Outcomes Research Network, Inc. The other authors have no conflicts of interest to declare.
Ethical Statement: The authors are accountable for all aspects of the work in ensuring that questions related to the accuracy or integrity of any part of the work are appropriately investigated and resolved. The study was conducted in accordance with the Declaration of Helsinki (as revised in 2013).
Open Access Statement: This is an Open Access article distributed in accordance with the Creative Commons Attribution-NonCommercial-NoDerivs 4.0 International License (CC BY-NC-ND 4.0), which permits the non-commercial replication and distribution of the article with the strict proviso that no changes or edits are made and the original work is properly cited (including links to both the formal publication through the relevant DOI and the license). See: https://creativecommons.org/licenses/by-nc-nd/4.0/.
References
- Dobrev D, Aguilar M, Heijman J, et al. Postoperative atrial fibrillation: mechanisms, manifestations and management. Nat Rev Cardiol 2019;16:417-36. [Crossref] [PubMed]
- Taha A, Nielsen SJ, Franzén S, et al. Stroke Risk Stratification in Patients With Postoperative Atrial Fibrillation After Coronary Artery Bypass Grafting. J Am Heart Assoc 2022;11:e024703. [Crossref] [PubMed]
- Hyun J, Cho MS, Nam GB, et al. Natural Course of New-Onset Postoperative Atrial Fibrillation after Noncardiac Surgery. J Am Heart Assoc 2021;10:e018548. [Crossref] [PubMed]
- Maesen B, Nijs J, Maessen J, et al. Post-operative atrial fibrillation: a maze of mechanisms. Europace 2012;14:159-74. [Crossref] [PubMed]
- Bianco V, Kilic A, Yousef S, et al. The long-term impact of postoperative atrial fibrillation after cardiac surgery. J Thorac Cardiovasc Surg 2023;166:1073-83.e10. [Crossref] [PubMed]
- Wang H, Wang Z, Zhou M, et al. Postoperative atrial fibrillation in pneumonectomy for primary lung cancer. J Thorac Dis 2021;13:789-802. [Crossref] [PubMed]
- Gaudino M, Di Franco A, Rong LQ, et al. Postoperative atrial fibrillation: from mechanisms to treatment. Eur Heart J 2023;44:1020-39. [Crossref] [PubMed]
- Zhang H, Qiao H, Yang B, et al. Development and validation of a diagnostic model based on left atrial diameter to predict postoperative atrial fibrillation after off-pump coronary artery bypass grafting. J Thorac Dis 2023;15:3708-25. [Crossref] [PubMed]
- Yu W, Liu F, Lei Q, et al. Identification of Key Pathways and Genes Related to Immunotherapy Resistance of LUAD Based on WGCNA Analysis. Front Oncol 2021;11:814014. [Crossref] [PubMed]
- Zhao C, Xiong K, Zhao F, et al. Glycosylation-Related Genes Predict the Prognosis and Immune Fraction of Ovarian Cancer Patients Based on Weighted Gene Coexpression Network Analysis (WGCNA) and Machine Learning. Oxid Med Cell Longev 2022;2022:3665617. [Crossref] [PubMed]
- Yang Y, Xu X. Identification of key genes in coronary artery disease: an integrative approach based on weighted gene co-expression network analysis and their correlation with immune infiltration. Aging (Albany NY) 2021;13:8306-19. [Crossref] [PubMed]
- Zhu Y, Yang X, Zu Y. Integrated analysis of WGCNA and machine learning identified diagnostic biomarkers in dilated cardiomyopathy with heart failure. Front Cell Dev Biol 2022;10:1089915. [Crossref] [PubMed]
- Zhang Y, Huang G, Yuan Z, et al. Circular RNA Expression for Dilated Cardiomyopathy in Hearts and Pluripotent Stem Cell-Derived Cardiomyocytes. Front Cell Dev Biol 2021;9:760515. [Crossref] [PubMed]
- Zhou J, He S, Wang B, et al. Construction and Bioinformatics Analysis of circRNA-miRNA-mRNA Network in Acute Myocardial Infarction. Front Genet 2022;13:854993. [Crossref] [PubMed]
- Zhou Y, Wu Q, Ni G, et al. Immune-associated pivotal biomarkers identification and competing endogenous RNA network construction in post-operative atrial fibrillation by comprehensive bioinformatics and machine learning strategies. Front Immunol 2022;13:974935. [Crossref] [PubMed]
- Chen Y, Ouyang T, Yin Y, et al. Analysis of infiltrated immune cells in left atriums from patients with atrial fibrillation and identification of circRNA biomarkers for postoperative atrial fibrillation. Front Genet 2022;13:1003366. [Crossref] [PubMed]
- Xu M, Zhou H, Hu P, et al. Identification and validation of immune and oxidative stress-related diagnostic markers for diabetic nephropathy by WGCNA and machine learning. Front Immunol 2023;14:1084531. [Crossref] [PubMed]
- Li Y, Wang B, Sun W, et al. Screening the immune-related circRNAs and genes in mice of spinal cord injury by RNA sequencing. Front Immunol 2022;13:1060290. [Crossref] [PubMed]
- Karamnov S, Muehlschlegel JD. Inflammatory Responses to Surgery and Postoperative Atrial Fibrillation. Anesthesiology 2022;136:877-9. [Crossref] [PubMed]
- Cabaro S, Conte M, Moschetta D, et al. Epicardial Adipose Tissue-Derived IL-1β Triggers Postoperative Atrial Fibrillation. Front Cell Dev Biol 2022;10:893729. [Crossref] [PubMed]
- Heijman J, Muna AP, Veleva T, et al. Atrial Myocyte NLRP3/CaMKII Nexus Forms a Substrate for Postoperative Atrial Fibrillation. Circ Res 2020;127:1036-55. [Crossref] [PubMed]
- Lozano-Velasco E, Franco D, Aranega A, et al. Genetics and Epigenetics of Atrial Fibrillation. Int J Mol Sci 2020;21:5717. [Crossref] [PubMed]
- Fatkin D, Santiago CF, Huttner IG, et al. Genetics of Atrial Fibrillation: State of the Art in 2017. Heart Lung Circ 2017;26:894-901. [Crossref] [PubMed]
- Tsoporis JN, Fazio A, Rizos IK, et al. Increased right atrial appendage apoptosis is associated with differential regulation of candidate MicroRNAs 1 and 133A in patients who developed atrial fibrillation after cardiac surgery. J Mol Cell Cardiol 2018;121:25-32. [Crossref] [PubMed]
- Fischer MA, Mahajan A, Cabaj M, et al. DNA Methylation-Based Prediction of Post-operative Atrial Fibrillation. Front Cardiovasc Med 2022;9:837725. [Crossref] [PubMed]
- Schumacher SM, Martens JR. Ion channel trafficking: a new therapeutic horizon for atrial fibrillation. Heart Rhythm 2010;7:1309-15. [Crossref] [PubMed]
- Zhao QD, Viswanadhapalli S, Williams P, et al. NADPH oxidase 4 induces cardiac fibrosis and hypertrophy through activating Akt/mTOR and NFκB signaling pathways. Circulation 2015;131:643-55. [Crossref] [PubMed]
- Olsen MB, Gregersen I, Sandanger Ø, et al. Targeting the Inflammasome in Cardiovascular Disease. JACC Basic Transl Sci 2022;7:84-98. [Crossref] [PubMed]
- Garg PK, Bartz TM, Norby FL, et al. Association of lipoprotein-associated phospholipase A(2) and risk of incident atrial fibrillation: Findings from 3 co-horts. Am Heart J 2018;197:62-69. [Crossref] [PubMed]
- Hefferan TE, Subramaniam M, Khosla S, et al. Cytokine-specific induction of the TGF-beta inducible early gene (TIEG): regulation by specific members of the TGF-beta family. J Cell Biochem 2000;78:380-90. [Crossref] [PubMed]
- Bos JM, Subramaniam M, Hawse JR, et al. TGFβ-inducible early gene-1 (TIEG1) mutations in hypertrophic cardiomyopathy. J Cell Biochem 2012;113:1896-903. [Crossref] [PubMed]
- Li Q, Shen P, Zeng S, et al. TIEG1 Inhibits Angiotensin II-induced Cardiomyocyte Hypertrophy by Inhibiting Transcription Factor GATA4. J Cardiovasc Pharmacol 2015;66:196-203. [Crossref] [PubMed]
- Cen M, Hu P, Cai Z, et al. TIEG1 deficiency confers enhanced myocardial protection in the infarcted heart by mediating the Pten/Akt signalling pathway. Int J Mol Med 2017;39:569-78. [Crossref] [PubMed]
- Yamac AH, Kucukbuzcu S, Ozansoy M, et al. Altered expression of micro-RNA 199a and increased levels of cardiac SIRT1 protein are associated with the occurrence of atrial fibrillation after coronary artery bypass graft surgery. Cardiovasc Pathol 2016;25:232-6. [Crossref] [PubMed]
- Nielsen JB, Thorolfsdottir RB, Fritsche LG, et al. Biobank-driven genomic discovery yields new insight into atrial fibrillation biology. Nat Genet 2018;50:1234-9. [Crossref] [PubMed]
- Wang J, Bai Y, Li N, et al. Pitx2-microRNA pathway that delimits sinoatrial node development and inhibits predisposition to atrial fibrillation. Proc Natl Acad Sci U S A 2014;111:9181-6. [Crossref] [PubMed]
- Yuan CT, Li XX, Cheng QJ, Wang YH, Wang JH, Liu CL. MiR-30a regulates the atrial fibrillation-induced myocardial fibrosis by targeting snail 1. Int J Clin Exp Pathol 2015;8:15527-36.
- Wang F, Liu D, Zhang RR, et al. A TBX5 3'UTR variant increases the risk of congenital heart disease in the Han Chinese population. Cell Discov 2017;3:17026. [Crossref] [PubMed]
(English Language Editor: J. Gray)