Development and validation of CT radiomics diagnostic models: differentiating benign from malignant pulmonary nodules and evaluating malignancy degree
Highlight box
Key findings
• By integrating clinical features and radiomics data, the established composite models show better performance in both differentiating the benign or malignant nature of pulmonary nodules (PNs) and predicting nodule malignancy compared to traditional clinical-radiological models.
What is known and what is new?
• Previously, traditional imaging-based diagnosis of PNs relied on features like size, shape, and density, which was subjective. Tumor marker detection also had limitations in terms of specificity. Existing radiomics studies mainly focused on extracting features from medical images but often faced challenges such as the instability of feature extraction and the lack of effective combination with clinical information.
• This manuscript presents a more comprehensive and accurate approach. It integrates diverse clinical features (e.g., patient age, gender, smoking history) with radiomics features. Through a clinically-relevant approach, it identifies key factors associated with nodule nature, crucial for guiding clinical decisions.
What is the implication, and what should change now?
• The implications are that these models can be applied in clinical practice to help doctors make more accurate treatment decisions. Clinicians should incorporate these models into their diagnostic processes. Future actions could include further validating these models in larger-scale, multi-center studies, and continuously optimizing the models by including more clinical factors and improving the radiomics feature extraction method.
Introduction
Lung cancer (LC) remains the most prevalent malignancy in China, according to the most recent data published by the National Cancer Society of China (1). In 2022, approximately 1.06 million new cases of LC were diagnosed in China, establishing it as the leading cause of cancer-related mortality among both males and females. The survival rates of LC vary by stage. According to the JCOG0201 study, the 5-year overall survival (OS) rate reached up to 90.4% for stage IA (sized <3 cm) LC but was typically ≤5% in patients diagnosed with stage IV LC. Therefore, early diagnosis of LC is critically important.
In recent years, the advent and wide application of medical imaging technologies [e.g., high-resolution computed tomography (CT)] have dramatically raised the detection rate of pulmonary nodules (PNs) (2,3), which has not only heightened public awareness of PNs but also presented significant challenges for clinical diagnosis and management. In the current clinical diagnosis of LC, non-invasive diagnostic methods play a crucial role. Imaging examinations are one of the commonly used means, and the judgment based on features such as nodule size, shape, and density is an important basis. Generally, the larger the diameter of the nodule, the more irregular its shape, and the higher its density, the greater the likelihood of malignancy. Henschke et al. (4) believed that ground-glass nodules (GGNs) were more likely to be malignant than solid nodules. Wang et al. (5) demonstrated that nodules characterized by a larger diameter, greater area, and increased diameter and area of solid components were more prone to being invasive adenocarcinoma (IA). Nevertheless, some imaging features are often subjective and may be inadequate for identifying the nature of a nodule. Hashimoto et al. (6) argued that nodules with a solid component that appeared to increase during follow-up, might nonetheless prove to be non-malignant tumors [e.g., solitary pulmonary capillary hemangioma (SPCH)]. At the same time, tumor marker testing is also a non-invasive diagnostic method. Common LC tumor markers include carcinoembryonic antigen (CEA), neuron-specific enolase (NSE), cytokeratin 19 fragment (CYFRA21-1), folate receptor-positive circulating tumor cells (FR+ CTCs), etc. (7). In patients with LC, the levels of these tumor markers may increase, so they can be used for auxiliary diagnosis.
However, these non-invasive diagnostic methods have certain limitations. The judgment of imaging features depends heavily on the experience and subjective judgment of doctors. Different doctors may have different interpretations of the size, shape, density, and other features of the same nodule, resulting in a lack of consistency and accuracy in diagnostic results. In addition, some benign lesions may also show imaging features similar to those of malignant tumors, which can easily lead to misdiagnosis. Although tumor marker testing is easy to operate, it has insufficient specificity. An increase in tumor marker levels does not necessarily mean that a patient has LC. Other benign diseases such as pulmonary inflammation and benign tumors can also cause an increase in tumor markers. Moreover, the tumor markers of some LC patients may not increase, leading to missed diagnoses. Therefore, it is crucial to find more accurate and reliable non-invasive diagnostic methods for the early diagnosis and treatment of LC.
Radiomics, as an innovative quantitative analytical approach, offers novel insights and methodologies for the diagnostic evaluation of PNs. The fundamental principle of radiomics involves utilizing computational algorithms to comprehensively mine and analyze radiological data obtained from modalities such as CT, magnetic resonance imaging (MRI), and positron emission tomography (PET)-CT. This process entails the extraction of high-throughput image features including both semantic and non-semantic attributes (8). Semantic features are defined as empirical characteristics proposed by radiologists for the qualitative description of lesions, including the size, shape, density, and edges of nodules. These features can be employed in radiological diagnosis and clinical applications; however, they lack valid mathematical expressions for their description. Non-semantic features are radiological attributes that can be quantitatively described through mathematical formulations, including the morphologic features (e.g., tumor volume, surface area, and compactness), the first-, second-, and higher-order texture features derived from the intensity distribution of each voxel within the region of interest (ROI), and the wavelet transform-based features. These features can reflect the characteristics of nodule tissues at the cellular level and thus predict a benign or malignant nodule more accurately. If the nodule is a tumor, radiomics can determine its aggressiveness through heterogeneity analysis (9). Furthermore, certain studies (10-12) have proposed that radiomics can be used to predict the presence (or not) of high-risk prognostic factors such as solid components, cribriform growth (13), micropapillary elements, spread through air spaces (STAS) (14), and visceral pleural invasion (VPI) (15). The presence of these high-risk factors can lead to a poorer prognosis, even in patients with early-stage disease (10). In this subset of patients, radical lobectomy has been associated with a significantly improved prognosis compared to sublobar resection. According to the JCOG0804 study, sublobar resection is a feasible and effective surgical approach for peripheral lung tumors measuring ≤2 cm that predominantly consist of ground-glass opacity [GGO; consolidation-to-tumor ratio (CTR) ≤0.25] and possess adequate surgical margins (≥5 mm) (16). This implies that radiomics can furnish surgeons with evidence to inform a more judicious selection of the resection extent when determining the surgical approach, by means of preoperative non-invasive pathological prediction, thereby optimizing patient outcomes.
Our present study was designed to investigate whether radiomics can serve as an accurate diagnostic tool for differentiating between benign PNs and primary pulmonary malignant tumor by developing a composite of one or more diagnostic models. For this purpose, patients with PNs who underwent surgical resection in the Department of Thoracic Surgery of the First Affiliated Hospital of Soochow University from September 2022 to September 2023 were retrospectively included. After screening according to strict inclusion and exclusion criteria, 202 eligible patients were selected and divided into a benign nodule group, a low-grade malignancy group, and a high-grade malignancy group based on postoperative pathological results. Secondly, the clinical and imaging data of the patients were collected, including baseline clinical information and imaging features of the nodules. Then, the ROI of PNs was delineated on thin-section CT images using specialized software, and a large number of radiomics features were extracted. These features were screened by the intraclass correlation coefficient (ICC) and the least absolute shrinkage and selection operator (LASSO) regression, and the radiomics score (Rad score) was calculated. Clinical-radiological models (CMs), radiomics models (RMs), and composite models (COMs) were constructed respectively. Finally, the performance of the constructed models was evaluated and compared using the receiver operating characteristic (ROC) curves, calibration curves, and decision curve analysis (DCA) to determine the optimal model. We present this article in accordance with the TRIPOD reporting checklist (available at https://jtd.amegroups.com/article/view/10.21037/jtd-2025-152/rc).
Methods
Patient data sources and grouping
Patients with PNs who underwent surgical resection in the Department of Thoracic Surgery at the First Affiliated Hospital of Soochow University between September 2022 and September 2023 and who fulfilled the inclusion criteria were retrospectively included. This study fully complied with the Declaration of Helsinki (2013 version) and was approved by the Ethics Committee of the First Affiliated Hospital of Soochow University (No. 2024668). As the analyzed data were anonymous and did not encroach on patient privacy, the need for obtaining signed informed consent was waived. The inclusion criteria were as follows: (I) had undergone high-resolution CT within 1 week prior to surgery; (II) the maximum diameter (including the greatest transverse diameter and the longest longitudinal axis) of the PNs, as measured on CT images, was <3 cm; (III) complete clinical baseline data were available; and (IV) complete postoperative pathological data. The exclusion criteria were as follows: (I) a prior history of malignancies (including primary pulmonary malignant tumor) or postoperative pathological findings indicative of metastatic cancer; (II) having undergone anti-inflammatory treatment within 1 month prior to surgery; (III) cases in which CT data were incomplete, of poor quality, or where the tumor was not readily distinguishable from surrounding tissues on CT imaging; or (IV) with incomplete or missing clinical data. Ultimately, a total of 202 patients with PNs were included in the analysis following the exclusion of 37 patients due to incomplete or substandard CT data.
The cases were divided into Groups A, B, and C based on their postoperative pathological findings. Patients in Group A (n=33) included those with chronic inflammation, benign tumors (e.g., leiomyomatoid hamartoma and sclerosing pneumocytoma), hyperplasia (e.g., alveolar epithelial hyperplasia and hypertrophic granulation tissue), bronchiolar adenoma, and other non-malignant lesions, for whom follow-up visits were arranged to determine the optimal timing for surgery. Group B (n=77) comprised patients with low-grade malignancies (e.g., adenocarcinoma in situ, minimally IA, and minimally IA in situ) who were candidates for early surgical intervention and could be treated with sublobar resection. Group C (n=92) included patients with IA (including lepidic, acinar, papillary, micropapillary, and solid patterns), poorly-differentiated carcinoma, mucinous adenocarcinoma, and other high-grade malignant lesions who required prompt surgical intervention and were likely to gain the most survival benefit from lobectomy.
For the purpose of establishing a model for the analysis of benign versus malignant nodules, Groups B and C were merged again to form Group D, which was then compared with Group A for analysis (hereinafter referred to as Study I). Due to the limited number of benign cases, stratified random sampling was utilized on the base of post-operative pathology to avoid selection bias and sampling bias. Groups A and D were randomly divided into the training group and the validation group at a ratio of 6:4 respectively.
When constructing a model for the analysis of malignancy, Groups B and C were incorporated into the study (hereinafter referred to as Study II). Groups B and C were allocated into a training set (n=101) and a validation set (n=68) in a ratio of 6:4.
Acquisition of CT data
CT imaging was performed using TOSHIBA (TOSHIBA, Tokyo, Japan), Philips 256 iCT (Philips Healthcare, Amsterdam, Netherlands), and SIEMENS Definition AS+128 (Siemens, Munich, Germany) CT scanners with the following settings: tube voltage, 120 kV; tube current (automatically adjusted), 110–240 mA; real-time dynamic exposure dose adjustment, enabled; collimation, 0.6 mm × 128 mm; rotation time, 0.5 s; pitch, 0.9; slice thickness, 5 mm; reconstructed slice thickness, 1 mm; and reorganization interval, 1 mm. Upon the scanning, all patients were instructed to raise their arms, enter the scanner head-first, and hold their breath following inhalation. The CT scan was conducted while the patient-maintained breath-hold. The scanning was performed from the level of the thoracic inlet to 5 cm below the costophrenic angle. DICOM format files for thin-slice CT scans were acquired for all patients.
Collection of clinicoradiological data
To demonstrate the advantages of the RM, we simultaneously collected the clinical and radiological semantic features of patients that are commonly used in clinical practice to construct clinical models (CMs). The baseline clinical data including gender, age, smoking history, and history of respiratory diseases [including chronic obstructive pulmonary disease (COPD)] were retrieved from the clinical database of the First Affiliated Hospital of Soochow University. The radiological features of nodules included size (maximum diameter of nodules on CT images), distribution in different lobes, anatomical location (peripheral or central) relative to the segmental bronchi, lobulation (the edge of the nodule resembles multiple arc-shaped protrusions, akin to the shape of a petal, with a relative depression formed between two adjacent arc-shaped protrusions), irregularity (the shape and boundary of the lesion deviate from the morphological norms of normal tissues and organs, and there is a lack of a typical geometric shape), spiculation (the boundary of the lesion exhibits a thin, short linear shadow extending into surrounding tissues, resembling sharp lines, with variable lengths and thicknesses distributed along the lesion’s edge, creating an uneven boundary), tumor-lung interface (there is a blurred boundary between the lesion and adjacent normal tissues, making it difficult to define the lesion’s precise extent), pleural indentation sign (one or more linear shadows connect the lesion to the pleura, causing the pleural image to distend in the direction of the lesion, presenting as triangular or flared depressions), vascular convergence sign (one or more blood vessels converge towards the lesion), vacuole sign (small, round, oval, or irregular low-density areas within the lesion, resembling vesicles), air bronchogram sign (air-containing bronchial shadows are visible within the nodule), and CTR (17). According to previous research, a CTR of ≥50% generally suggests a higher likelihood of malignancy. Consequently, we categorized nodules in our current study based on the CTR threshold value of ≥50%. All the radiological features were independently reviewed by two radiologists who had been interpreting images for a minimum of 3 years and were blinded to the patients’ final pathological results. In the event of discrepancies, a final evaluation was conducted by a radiologist with more than 10 years of experience.
CT image segmentation and radiomic feature extraction
The free and open-source software three-dimensional (3D) Slicer (version 5.6.2; https://download.slicer.org/) was employed to import the DICOM files of thin-slice CT scans for all patients in sequence. The ROI for the lung nodules was delineated, with uniform window width (WW) and window level (WL) applied prior to delineation. The delineation guidelines were as follows: (I) all the stretching lines involving the spicule sign and the pleural indentation sign of nodules were outlined; (II) the blood vessels clustered in the nodules were included in the delineation area, with attention given to avoiding bronchi and blood vessels unrelated to the nodules; (III) the CT images of each thin layer were delineated layer by layer, culminating in the generation of a 3D nodule model; (IV) the delineation was completed by the same thoracic surgeon to ensure the reproducibility of the data and calibrated by a single radiologist to ensure the accuracy of the delineation; and (V) 1 month after the completion of the delineation for all patients with nodules, the ROI was re-delineated in 30 randomly-selected patients from the entire cohort, with a radiologist invited to delineate simultaneously. The data from three delineations were evaluated using the ICC.
The extraction of radiomics features from the 3D nodule models was performed using the Python-based software package SlicerRadiomics, which is an extension for 3D Slicer. Prior to the feature extraction, the images were resampled to a uniform voxel size of 1 mm × 1 mm × 1 mm. Utilizing this software package, we extracted 851 radiomics features, including first-order statistics, shape, gray-level dependence matrix (GLDM), gray-level co-occurrence matrix (GLCM), gray-level run-length matrix (GLRLM), neighborhood gray-tone difference matrix (NGTDM), gray-level size-zone matrix (GLSZM), and wavelet transformed features.
Radiomics feature screening and establishment of RMs
For the 851 radiomics features extracted, the ICC was employed for preliminary feature screening. A total of 702 features were found to exhibit good stability, with ICC values exceeding 0.75. Subsequently, redundancy analysis was conducted to eliminate features with a correlation coefficient exceeding 0.9, which yielded 129 radiomics features. The correlation matrix for these features, derived from Pearson correlation filtering, is plotted in Figure 1.
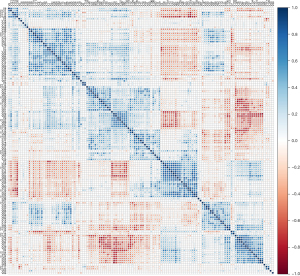
In both Study I and Study II, the LASSO regression was employed to further refine the radiomics features following the initial feature screening. To mitigate the risk of overfitting, a 10-fold cross-validation method was implemented to enhance the generalizability of the models. The Rad score, representing the combined effect of the final set of screened radiological features and their corresponding LASSO regression coefficients, was computed, and the results were used for constructing two RMs, I and II. The performance of these two models was assessed using the ROC curves.
Establishment and performance evaluation of CMs and COMs
In both Study I and Study II, univariate and multivariate logistic regression analyses were performed to identify independent predictors based on clinical and radiological features. Additionally, CMs were established and COMs were developed by integrating the RMs and the CMs. The performance of these three models was evaluated using DCA.
Statistical analysis
The statistical analysis was conducted using R Studio (an integrated development environment, version 4.2.1; https://cran.r-project.org/). Both continuous and categorical variables were collected for clinical and radiological features. The continuous variables are represented as the mean ± standard deviation (SD), whereas the categorical variables are expressed as number and rates. Differences among groups were assessed using the Mann-Whitney U test. Multivariate logistic regression was employed to identify independent predictors for the CMs, a P value less than 0.05 was considered statistically significant. The minimum Akaike’s information criterion (AIC) was utilized to select the optimal model parameters. The LASSO regression was utilized to establish the RMs. The Rad score, along with clinical-radiological features, was then employed to construct COMs, for which diagnostic performance was evaluated using the ROC curve. The areas under the ROC curve (AUCs) were compared among these models using the DeLong test. In addition, to compensate for the bias caused by the imbalanced data, we employed stratified random sampling during the grouping process. We stratified according to the postoperative pathology to ensure that the sample distributions in the training set and the validation set were consistent. In addition, the specificity, sensitivity and balanced accuracy were calculated in each group to further evaluate the performance of the model, sensitivity = true positives/(true positives + false negatives), and specificity = true negatives/(true negatives + false positives). Balanced accuracy = (sensitivity + specificity)/2. DCA was used to assess the predictive performance of the COMs.
All the codes employed in this study were written within the R Studio software environment. The packages used included: “ICC psych” for ICC calculation, “glmnet” for LASSO regression, and “glm stats” for multivariate logistic regression. All image plots were generated within R Studio. Calibration curves were generated using the val.prob function and the calibrate function from the “rms” package. DCA curves were created using the “rmda” package. The “ROCR” package was utilized for the comparison of ROC curves across multiple models and the “Dcurves” package for the comparison of DCA curves.
Results
A total of 202 patients entered the final analysis, comprising 67 males (33.17%) with an average age of 57 years and 135 females (66.83%) with an average age of 56 years. The lesions were identified as benign in 33 patients (i.e., Group A), low-grade malignant in 77 patients (i.e., Group B), and high-grade malignant in 92 patients (i.e., Group C). In Research I and Research II, all clinical data (including age, gender, smoking history, and underlying lung diseases) and radiological features (including maximal diameter of nodules, solid component proportion, lobes with nodules, peripheral or central type, lobulation sign, shape, spicule sign, blurred boundary, pleural indentation sign, vascular convergence sign, vacuolar sign, and air bronchogram sign) were examined. Univariate analysis and multivariate logistic regression analysis were employed in Study II to identify significant predictors.
Analysis of benign versus malignant lesions
Establishment and evaluation of the CM(I)
For the purpose of distinguishing benign and malignant nodules, the baseline clinical data and radiological features of Group A (n=20) and Group D (n=102) within the training group are presented in Table 1. Univariate logistic regression analysis of all variables revealed that only two variables had P values <0.05, which might be attributed to the relatively small number of benign cases. To maximize the capturing of potential variables, seven variables with P values <0.2 [including spicule sign (P=0.003), marginal blurring (P=0.14), vascular convergence sign (P=0.10), air bronchogram sign (P=0.12), gender (P=0.10), CTR (P=0.20), and right lower lung (P=0.03)] were selected for multivariate logistic regression analysis. The minimum AIC was utilized to select the optimal model parameters, and the results revealed that female gender [odds ratio (OR): 4.967; 95% confidence interval (CI): 1.423–19.50], positive air bronchogram sign (OR: 4.545; 95% CI: 0.842–37.98), positive spicule sign (OR: 3.643; 95% CI: 0.963–13.73), and positive vascular convergence sign (OR: 2.509; 95% CI: 0.723–9.144) were associated with an increased likelihood of malignancy. Nodules with a CTR ≥50% (OR: 0.528; 95% CI: 0.196–1.386) are more likely to be benign nodules. The univariate and multivariate logistic regression coefficients of each variable are shown in Table 2, and the nomogram is presented in Figure 2. CM(I) was established as follows:
Table 1
Factors | All (n=122) | Group A (n=20) | Group D (n=102) | P value |
---|---|---|---|---|
Gender | 0.16 | |||
Male | 36 [30] | 9 [45] | 27 [26] | |
Female | 86 [70] | 11 [55] | 75 [74] | |
Age (years) | 56 [45, 66.75] | 56.5 [49.75, 62] | 56 [44.25, 67] | 0.86 |
Smoking | 0.64 | |||
No | 113 [93] | 18 [90] | 95 [93] | |
Yes | 9 [7] | 2 [10] | 7 [7] | |
Pulmonary disease | >0.99 | |||
No | 119 [98] | 20 [100] | 99 [97] | |
Yes | 3 [2] | 0 [0] | 3 [3] | |
CTR | 0.29 | |||
<50% | 71 [58] | 9 [45] | 62 [61] | |
≥50% | 51 [42] | 11 [55] | 40 [39] | |
Diameter (mm) | 10 [8, 15] | 9.5 [7, 14.25] | 11 [8, 16] | 0.38 |
Lobe | 0.03 | |||
RUL | 33 [27] | 4 [20] | 29 [28] | |
RML | 8 [7] | 2 [10] | 6 [6] | |
RLL | 17 [14] | 7 [35] | 10 [10] | |
LUL | 47 [39] | 4 [20] | 43 [42] | |
LLL | 17 [14] | 3 [15] | 14 [14] | |
Location† | >0.99 | |||
Central | 4 [3] | 0 [0] | 4 [4] | |
Peripheral | 118 [97] | 20 [100] | 98 [96] | |
Lobulation | 0.94 | |||
No | 54 [44] | 9 [45] | 45 [44] | |
Yes | 68 [56] | 11 [55] | 57 [56] | |
Irregularity | 0.95 | |||
No | 51 [42] | 9 [45] | 42 [41] | |
Yes | 71 [58] | 11 [55] | 60 [59] | |
Spicule sign | 0.005 | |||
No | 16 [13] | 7 [35] | 9 [9] | |
Yes | 106 [87] | 13 [65] | 93 [91] | |
Marginal burring | 0.22 | |||
No | 49 [40] | 11 [55] | 38 [37] | |
Yes | 73 [60] | 9 [45] | 64 [63] | |
Pleural indentation sign | 0.40 | |||
No | 72 [59] | 14 [70] | 58 [57] | |
Yes | 50 [41] | 6 [30] | 44 [43] | |
Vascular convergence sign | 0.15 | |||
No | 41 [34] | 10 [50] | 31 [30] | |
Yes | 81 [66] | 10 [50] | 71 [70] | |
Vacuole sign | >0.99 | |||
No | 59 [48] | 10 [50] | 49 [48] | |
Yes | 63 [52] | 10 [50] | 53 [52] | |
Air bronchogram sign | 0.15 | |||
No | 92 [75] | 18 [90] | 74 [73] | |
Yes | 30 [25] | 2 [10] | 28 [27] |
Data are presented as the median [Q1, Q3] or n [%]. Group A: benign group. Group D: Groups B and C were merged. Group B: low-grade malignant group. Group C: high-grade malignant group. †, location: taking the segmental bronchi as the boundary, the proximal part is classified as the central type, and the distal part is classified as the peripheral type. CTR, consolidation-to-tumor ratio; LLL, left lower lung; LUL, left upper lung; Q1, the first quartile; Q3, the third quartile; RLL, right lower lung; RML, right middle lung; RUL, right upper lung.
Table 2
Variables | Univariable logistic regression | Multivariable logistic regression | |||||
---|---|---|---|---|---|---|---|
β | OR (95% CI) | P value | β | OR (95% CI) | P value | ||
Gender | 0.821 | 2.273 (0.833–6.102) | 0.10 | 1.603 | 4.967 (1.423–19.50) | 0.02 | |
Air bronchogram sign | 1.225 | 3.405 (0.902–22.29) | 0.12 | 1.514 | 4.545 (0.842–37.98) | 0.11 | |
Right lower lung | −1.624 | 0.197 (0.043–0.789) | 0.02 | ||||
Vascular convergence sign | 0.829 | 2.29 (0.858–6.136) | 0.10 | 0.92 | 2.509 (0.723–9.144) | 0.15 | |
Marginal blurring | 0.722 | 2.058 (0.783–5.547) | 0.14 | ||||
Spicule sign | 1.716 | 5.564 (1.734–17.66) | 0.003 | 1.293 | 3.643 (0.963–13.73) | 0.053 | |
CTR ≥50% | −0.639 | 0.528 (0.196–1.386) | 0.20 | −1.422 | 0.241 (0.066–0.782) | 0.02 |
β value (regression coefficient) represents the magnitude and direction of the influence of the independent variable on the dependent variable. CI, confidence interval; CTR, consolidation-to-tumor ratio; OR, odds ratio.
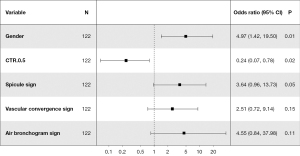
The AUC was 0.763 (95% CI: 0.623–0.902) for the training group and 0.823 (95% CI: 0.700–0.945) for the validation group. The specificity was 0.60 for the training group and 0.69 for the validation group. The sensitivity was 0.90 for the training group and 0.85 for the validation group. The balanced accuracy was 0.75 for the training group and 0.77 for the validation group. The calibration chart demonstrated good consistency between the training set and the validation set. The DCA curve indicated that the clinical-radiological curve was reliable for the discrimination of benign and malignant nodules (Figure 3).
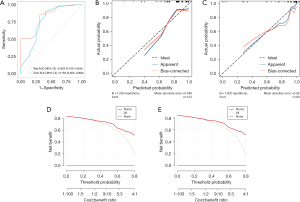
Establishment and evaluation of the RM(I)
In the analysis of benign versus malignant nodules, the LASSO algorithm was employed to select eight optimal radiomics features from the 129 features that were initially screened using ICC. These eight features included “SurfaceVolumeRatio”, “X10Percentile”, “ClusterProminence.1”, “Imc1.1”, “Correlation.2”, “SizeZoneNonUniformityNormalized.4”, “Contrast.11”, and “ClusterProminence.6”. Figure 4 illustrates the LASSO 10-fold cross-validation chart and the Rad score for both the training group and the validation group. The corresponding impact coefficients for each radiomics feature are shown in Figure 5. The formula for calculating the RM(I) (Rad score) is as follows:
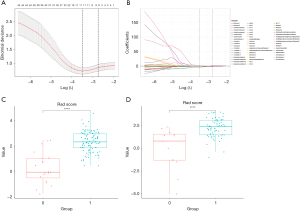
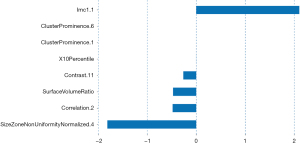
The waterfall plot depicted that the RM(I) had a high accuracy (Figure 6), with an AUC of 0.895 (95% CI: 0.814–0.975) in the training group and 0.808 (95% CI: 0.673–0.944) in the validation group. The specificity was 0.65 for the training group and 0.54 for the validation group. The sensitivity was 0.98 for the training group and 0.91 for the validation group. The balanced accuracy was 0.82 for the training group and 0.73 for the validation group. The DCA and calibration curves also indicated satisfactory performance (Figure 7).

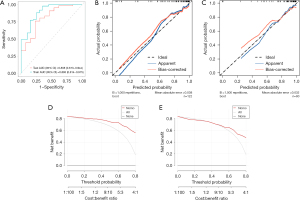
Establishment of COM(I) and multi-model evaluation
The Rad score was integrated with gender (0/1), spicule sign (0/1), vascular convergence sign (0/1), air bronchogram sign (0/1), and CTR (0/1) to establish a COM(I), with the formula for calculating the score of the COM(I) (Comscore) as follows:
A nomogram depicting each feature in the COM(I) is presented in Figure 8. The AUC of the COM was 0.927 (95% CI: 0.854–1.000) in the training group and 0.854 (95% CI: 0.723–0.985) in the validation group. The specificity was 0.80 for the training group and 0.54 for the validation group. The sensitivity was 0.97 for the training group and 0.94 for the validation group. The balanced accuracy was 0.88 for the training group and 0.74 for the validation group. The DCA and calibration curves indicated high model performance (Figure 9).
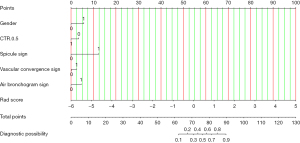
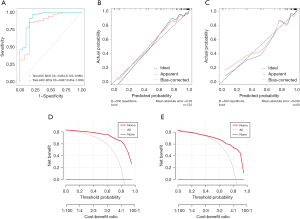
Finally, the AUCs and DCA scores of the COM(I), the CM(I), and the RM(I) were compared in Study I for analysis of benign versus malignant nodules (Figure 10). The results revealed that the COM(I) outperformed the CM(I) in the training group (P=0.01), whereas its performance was not statistically different from that of the RM(I) (P=0.10). There was no statistical difference in the prediction performance among these three models in the validation set, indicating that both the COM(I) and the RM(I) had robust performance.
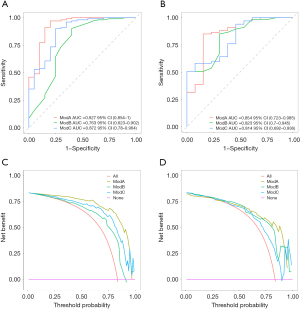
Analysis of the degree of malignancy
Establishment and evaluation of the CM(II)
In the analysis of malignancy degree, the baseline clinical data and radiological features of Group B (n=45) and Group C (n=56) in the training group are shown in Table 3. Univariate logistic regression analysis showed that air bronchogram sign (P<0.001), vacuole sign (P<0.001), marginal blurring (P<0.001), irregularity (P<0.001), lobulation sign (P<0.001), diameter (P<0.001), age (P<0.001), vascular convergence sign (P=0.001), CTR ≥50% (P=0.001), pleural indentation sign (P=0.006), spicule sign (P=0.04), and gender (P=0.04) were statistically significant and were included in multivariate logistic regression analysis (Table 4). Again, AIC was used for selecting the optimal model parameters, which showed that older age (OR: 1.046; 95% CI: 0.999–1.099), longer maximal diameter (OR: 1.449; 95% CI: 1.225–1.795), irregularity (OR: 2.589; 95% CI: 0.770–8.805), and CTR ≥50% (OR: 3.700; 95% CI: 1.109–13.52) were associated with a higher likeliness of malignant nodules. The univariate and multivariate logistic regression coefficients of each variable are shown in Table 4, and the CM(II) was established as follows:
Table 3
Factors | All (n=101) | Group B (n=45) | Group C (n=56) | P value |
---|---|---|---|---|
Gender | 0.15 | |||
Male | 31 [31] | 10 [22] | 21 [38] | |
Female | 70 [69] | 35 [78] | 35 [62] | |
Age (years) | 57 [45, 67] | 48 [39, 59] | 63 [55, 68] | <0.001 |
Smoking | 0.22 | |||
No | 95 [94] | 44 [98] | 51 [91] | |
Yes | 6 [6] | 1 [2] | 5 [9] | |
Pulmonary function | 0.13 | |||
No | 97 [96] | 45 [100] | 52 [93] | |
Yes | 4 [4] | 0 [0] | 4 [7] | |
CTR | <0.001 | |||
<50% | 54 [53] | 33 [73] | 21 [38] | |
≥50% | 47 [47] | 12 [27] | 35 [63] | |
Diameter (mm) | 11 [8, 16.25] | 8 [7, 10] | 16 [12.5, 19] | <0.001 |
Lobe | 0.34 | |||
RUL | 30 [30] | 14 [31] | 16 [29] | |
RML | 10 [10] | 4 [9] | 6 [11] | |
RLL | 9 [9] | 4 [9] | 5 [9] | |
LUL | 39 [39] | 14 [31] | 25 [45] | |
LLL | 13 [13] | 9 [20] | 4 [7] | |
Location | >0.99 | |||
Central | 4 [4] | 2 [4] | 2 [4] | |
Peripheral | 97 [96] | 43 [96] | 54 [96] | |
Lobulation | <0.001 | |||
No | 40 [40] | 33 [73] | 7 [13] | |
Yes | 61 [60] | 12 [27] | 49 [88] | |
Irregularity | <0.001 | |||
No | 42 [42] | 33 [73] | 9 [16] | |
Yes | 59 [58] | 12 [27] | 47 [84] | |
Spicule sign | 0.043 | |||
No | 7 [7] | 6 [13] | 1 [2] | |
Yes | 94 [93] | 39 [87] | 55 [98] | |
Marginal burring | 0.006 | |||
No | 40 [40] | 25 [56] | 15 [27] | |
Yes | 61 [60] | 20 [44] | 41 [73] | |
Pleural indentation sign | <0.001 | |||
No | 53 [52] | 34 [76] | 19 [34] | |
Yes | 48 [48] | 11 [24] | 37 [66] | |
Vascular convergence sign | <0.001 | |||
No | 29 [29] | 22 [49] | 7 [13] | |
Yes | 72 [71] | 23 [51] | 49 [88] | |
Vacuole sign | <0.001 | |||
No | 44 [44] | 33 [73] | 11 [20] | |
Yes | 57 [56] | 12 [27] | 45 [80] | |
Air bronchogram sign | <0.001 | |||
No | 68 [67] | 39 [87] | 29 [52] | |
Yes | 33 [33] | 6 [13] | 27 [48] |
Data are presented as the median [Q1, Q3] or n [%]. Group B: low-grade malignant group. Group C: high-grade malignant group. Location: taking the segmental bronchi as the boundary, the proximal part is classified as the central type, and the distal part is classified as the peripheral type. CTR, consolidation-to-tumor ratio; LLL, left lower lung; LUL, left upper lung; Q1, the first quartile; Q3, the third quartile; RLL, right lower lung; RML, right middle lung; RUL, right upper lung.
Table 4
Variables | Univariable logistic regression | Multivariable logistic regression | |||||
---|---|---|---|---|---|---|---|
β | OR (95% CI) | P value | β | OR (95% CI) | P value | ||
Gender | −0.93 | 0.395 (0.153–0.954) | 0.04 | ||||
Air bronchogram sign | 2.232 | 9.317 (3.231–34.05) | <0.001 | ||||
Vacuole sign | 1.792 | 6 (2.608–14.55) | <0.001 | ||||
Vascular convergence sign | 1.646 | 5.185 (2.021–14.68) | 0.001 | ||||
Marginal blurring | 1.482 | 4.404 (1.946–10.38) | <0.001 | ||||
Spicule sign | 1.712 | 5.538 (1.302–38.05) | 0.04 | ||||
CTR ≥50% | 1.401 | 4.058 (1.763–9.87) | 0.001 | 1.308 | 3.700 (1.109–13.52) | 0.04 | |
Pleural indentation sign | 1.145 | 3.143 (1.406–7.286) | 0.006 | ||||
Irregularity | 1.961 | 7.104 (3.029–17.68) | <0.001 | 0.951 | 2.589 (0.770–8.805) | 0.12 | |
Lobulation sign | 2.485 | 12 (4.909–31.77) | <0.001 | ||||
Diameter (mm) | 0.48 | 1.617 (1.368–2.007) | <0.001 | 0.371 | 1.449 (1.225–1.795) | <0.001 | |
Age (years) | 0.083 | 1.086 (1.049–1.131) | <0.001 | 0.045 | 1.046 (0.999–1.099) | 0.06 |
β value (regression coefficient) represents the magnitude and direction of the influence of the independent variable on the dependent variable. CI, confidence interval; CTR, consolidation-to-tumor ratio; OR, odds ratio.
The nomogram of each coefficient is shown in Figure 11. The AUC was 0.924 (95% CI: 0.873–0.975) for the training group and 0.950 (95% CI: 0.901–0.999) for the validation group. The specificity was 0.94 for the training group and 0.93 for the validation group. The sensitivity was 0.82 for the training group and 0.89 for the validation group. The balanced accuracy was 0.87 for the training group and 0.91 for the validation group. Both the DCA and the calibration curves showed that the model had potential clinical utility (Figure 12).
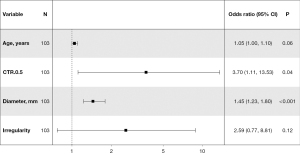
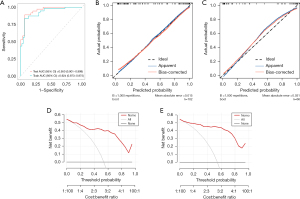
Establishment and evaluation of the RM(II)
In the analysis of malignancy degree, both ICC and the LASSO algorithm were employed to select eight optimal radiomics features including “Sphericity”, “MaximumProbability.1”, “Coarseness.1”, “Complexity.1”, “MaximumProbability.2”, “Imc1.3”, “MaximumProbability.6”, and “SmallDependenceLowGrayLevelEmphasis.6”. Figure 13 illustrates the LASSO 10-fold cross-validation chart and the Rad score. The feature coefficients are shown in Figure 14, and Figure 15 is the waterfall plot. The formula for calculating the RM(II) is as follows:
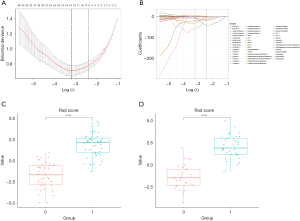
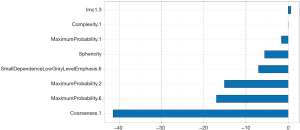
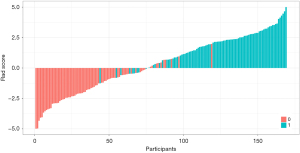
The AUC of the RM(II) was 0.966 (95% CI: 0.938–0.994) in the training group and 0.959 (95% CI: 0.916–1.000) in the validation group. The specificity was 1.00 for the training group and 0.90 for the validation group. The sensitivity was 0.82 for the training group and 0.88 for the validation group. The balanced accuracy was 0.91 for the training group and 0.89 for the validation group. Evaluation with calibration curves and DCA revealed that the RM(II) performed better than the CM(II) (Figure 16).
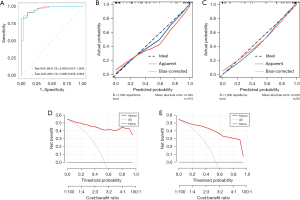
Establishment and evaluation of the COM(II)
The Rad score II was integrated with age, diameter, irregularity (0/1), and CTR (0 if <50%/1 if ≥50%) to establish COM(II). The nomogram of each feature is shown in Figure 17, and the formula for COM(II) is as follows:
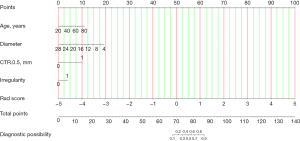
The AUC of the COM(II) was 0.972 (95% CI: 0.948–0.996) in the training group and 0.967 (95% CI: 0.928–1.000) in the validation group. The specificity was 1.00 for the training group and 0.90 for the validation group. The sensitivity was 0.86 for the training group and 0.91 for the validation group. The balanced accuracy was 0.93 for the training group and 0.91 for the validation group. The DCA and calibration curves indicate high model performance (Figure 18).
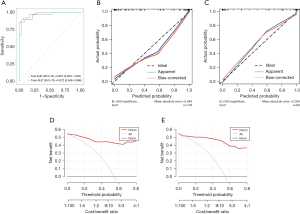
Finally, the AUCs and DCA curves of the COM(II), the CM(II), and the RM(II) were compared in Study II for analysis of malignancy degrees (Figure 19). The results revealed that the COM(II) outperformed the CM(II) in the training group (P=0.02), whereas its performance was not statistically different from that of the RM(II) (P=0.49). There was no statistical difference in the prediction performance among these three models in the validation set, indicating that both the COM(II) and the RM(II) had robust performance.
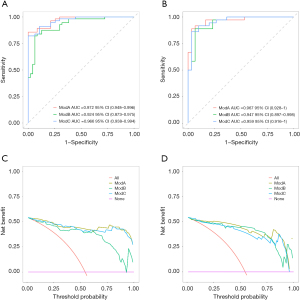
Discussion
Although state-of-the-art imaging technologies can now reveal the heterogeneity within nodules at the tissue and cell levels, the human eye and brain lack the capability to fully interpret the extensive data in these images. Consequently, radiomics has emerged as a field that, leveraging computer algorithms and software, facilitates the extraction and processing of feature information from tissue models within the imaged region. Ultimately, it culminates in the construction of predictive models that can estimate tumor stage, extent of invasion, and genetic mutations [e.g., epidermal growth factor receptor (EGFR) mutations] (18,19).
In the present study, we employed radiomics to develop two sets of models for determining and measuring the malignancy of <3 cm PNs observed on thin-section CT images. The COMs in both sets showed strong performance and significant potential for clinical use in early nodule nature assessment. In this study, radiomics played a significant role in enhancing the performance of the models. In Study I, which focused on differentiating between benign and malignant nodules, the CM(I) had an AUC of 0.763, a specificity of 0.60, a sensitivity of 0.90, and a balanced accuracy of 0.75 in the training group, and an AUC of 0.823, a specificity of 0.69, a sensitivity of 0.85, and a balanced accuracy of 0.77 in the validation group. The RM(I) achieved an AUC of 0.895, a specificity of 0.65, a sensitivity of 0.98, and a balanced accuracy of 0.82 in the training group, and an AUC of 0.808, a specificity of 0.54, a sensitivity of 0.91, and a balanced accuracy of 0.73 in the validation group. In Study II, which analyzed the degree of malignancy, the CM(II) had an AUC of 0.924, a specificity of 0.94, a sensitivity of 0.82, and a balanced accuracy of 0.87 in the training group, and an AUC of 0.950, a specificity of 0.93, a sensitivity of 0.89, and a balanced accuracy of 0.91 in the validation group. The RM(II) had an AUC of 0.966, a specificity of 1.00, a sensitivity of 0.82, and a balanced accuracy of 0.91 in the training group, and an AUC of 0.959, a specificity of 0.90, a sensitivity of 0.88, and a balanced accuracy of 0.89 in the validation group. The improvements in AUC, specificity, sensitivity, and balanced accuracy of the RMs indicate that radiomics can extract in-depth features from images, providing more abundant information for the models. This significantly enhances the models’ ability to distinguish between benign and malignant PNs and evaluate the degree of malignancy, thus improving the prediction performance.
In fact, a more conservative approach can be taken for nodules suspected of being benign; for nodules suspected of low-grade malignancy, early treatment may be advised, considering the patient’s overall health; in contrast, for nodules suspected of high-grade malignancy, an aggressive treatment strategy is warranted, with preoperative planning for wider surgical resection margins to optimize survival benefits.
In this study, the unique contribution of clinical features lies in providing crucial information for judging the nature of PNs. Multivariate regression analysis in our current study revealed that the likelihood of nodule malignancy was increased in the presence of female gender, positive air bronchogram sign, positive spicule sign, positive vascular convergence sign, and a CTR of <50%. Among patients with a predicted malignancy, the risk of high-grade malignancy was higher in the presence of the following factors: older age (with a ROC cut-off point of 56.5 years), longer maximal diameter of the lesion (ROC cut-point value =11.5 mm), irregular shape, and a CTR of ≥50%. Considering clinical features can improve the accuracy of radiomics-based models for three reasons. First, clinical features can supplement information not covered by radiomics features, such as patients’ basic information and medical history, which are closely related to tumor development. Second, it helps to exclude interfering factors when judging imaging features, and combining with medical history can more accurately assess the nature of nodules. Third, the combination of the two can construct a more comprehensive and interpretable model, facilitating clinicians’ understanding and rational application in practice (20).
Tobacco consumption has been broadly acknowledged as a predominant risk factor for LC (21). In our current study, however, smoking history was not found to be a significant contributor to the determination of whether a PN is malignant or exhibits a higher degree of malignancy. Two reasons might explain this phenomenon: first, the study population comprised a preponderance of women (n=135), constituting approximately 66.8% of the total sample, among whom only one individual reported a history of smoking. This finding was associated with the demographic reality that the majority of smokers in China are male. In our current study, approximately 18% of the male participants had a history of smoking. Second, smoking is commonly believed to be closely associated with the incidence of lung squamous cell carcinoma (LSCC). In the present study, there were only nine cases of central nodules, which were located above the bronchial segments of the lungs, and all of these cases were diagnosed as adenocarcinomas.
In Study I, the RM(I) incorporated eight features, which were derived from a synthesis of diverse preprocessing techniques [e.g., intensity normalization (22) and filtering] and sophisticated mathematical procedures (e.g., matrix transformation and polynomial computation) applied to radiological data. Three of these features are more intuitive to grasp: (I) “SurfaceVolumeRatio” is used to measure the relationship between the surface area and volume of the ROI. Normally, an object with many indentations, protrusions, or lobes gets a relatively high surface-to-volume ratio. In the RM(I), this feature acted as a protective factor, which might be attributed to the fact that the majority of PNs in this study were subsolid and predominantly exhibited regular morphological traits, indicative of a potentially higher likelihood of high-grade malignancy. (II) “ClusterProminence” is used to measure the prominence of a certain cluster in the overall distribution. It can help to identify which cluster is more prominent in texture among these areas, thus providing clues for the analysis of tumor internal heterogeneity. (III) “SizeZoneNonUniformityNormalized” is used to evaluate the non-uniformity of the size of the regions within the ROI and has been normalized. It can help to identify those lesions with more complex internal structures and larger differences in region size.
In Study II, the RM(II) also incorporated eight features, among which three were more comprehensible: (I) “Sphericity” is used to describe the degree of similarity between the ROI and the shape of a sphere. A higher sphericity value, indicative of a more perfect spherical morphology, was posited by the model as a protective factor. Malignant nodules typically exhibit greater irregularity, which correlates with a higher likelihood of malignancy—a notion that aligned with our CMs. (II) “MaximumProbability” denotes the likelihood of occurrence of a specific gray level within the ROI. It was found to be a protective factor in all the three groups in our models. (III) “Coarseness” pertains to the variation in gray values within the local area of an image. A significant variation in grayscale values across a small area leads to elevated coarseness values.
In our present study, we investigated the association between the nature of PNs and their radiological features, aiming to utilize such a correlation for informing treatment strategies. It did not conflict with findings from previous research. It was found that non-solid nodules in female patients were more prone to being LC. Similarly, many studies have demonstrated gender disparities in the incidence of non-small cell LC (NSCLC) (23,24). A study conducted across 13 countries revealed a rising incidence of LC among women and a declining trend among men (25). Furthermore, women exhibited a higher mortality rate from LC compared to men (25), possibly due to higher exposure of women to cooking fumes within the household; in addition, unmitigated second-hand tobacco smoke exposure and hormonal fluctuations (estrogen levels) also contribute to an increased risk of LC in women (23). Our present study indicated that the lobulation sign, pleural indentation sign, and vacuole sign were not risk factors for malignant nodules. However, a CTR of <50% was found to be a risk factor for malignancy (OR: 4.419; 95% CI: 1.282–15.152), which may be associated with the early growth patterns and cellular characteristics of the tumors: cancer cells grow adherently along the alveolar septum, leading to the thickening of alveolar walls; however, the alveolar cavity is not completely occluded. Accordingly, GGNs appeared on CT (26). As the tumor cells continuously proliferate and differentiate and as they infiltrate surrounding tissues, the alveolar spaces progressively collapse and become occluded, leading to an increase in density, which ultimately manifests as solid nodules. This also accounts for our finding that solid nodules with a CTR of ≥50% tended to be associated with a higher degree of malignancy. This distinctive growth pattern is crucial for evaluating PNs. Wu et al. (27) and Takashima et al. (28) demonstrated that the presence of vascular convergence sign may suggest an elevated potential for malignancy, which could be explained by the faster metabolic rate of tumors because heightened metabolism over an extended period may lead to the dilation of peripheral blood vessels and the convergent alterations in the tumor microenvironment. Tu et al. (29) also found that tumor size, vascular convergence, and lobulation were all indicative of a higher probability of malignancy. The maximum diameter of tumors has emerged as a crucial feature for distinguishing between preinvasive lesions and invasive lesions, although the optimal cut-off value for the differentiation varies across studies (30-32). Chang et al. (33) identified that the optimal cut-off value for differentiating atypical adenomatous hyperplasia, adenocarcinoma in situ, and microinvasive adenocarcinoma from IA was 10.5 mm, which was essentially in alignment with the cut-off value of 11.5 mm in our current study. Consistently, Kou et al. (34) discovered that surgical patients with non-invasive lesions were of a younger age compared to those in the IA group. The transition to an infiltrating component in GGN typically took a period of approximately three and a half years, with a reported doubling time that extended up to 9 years (35).
In comparison with prior studies, the novel contributions of our current study are as follows: (I) all the nodules <3 cm on CT, regardless of whether they were pathologically malignant, were included for analysis, which aligned more closely with clinical practice. Although lobectomy has traditionally been the standard surgical procedure for resectable NSCLC, recent research has indicated that segmentectomy should be considered a standard component of treatment for ground-glass-dominant LC with a tumor diameter of ≤3 cm including GGO (36). Jiang et al. (37) also found that the sublobar resection group had lower incidence of “chest tightness”, “breath shortness”, “breathlessness”, “cough”, and “expectoration” than the lobectomy group. In our current study, the models developed through the integration of radiomics features with clinical-radiological features showed robust performance as they helped avoid unnecessary surgical intervention for certain benign lesions and could predict the degree of malignancy of nodules prior to surgical intervention. Segmentectomy can be considered for patients with low preoperatively-predicted malignancy to reduce the increase in surgical risk due to lobectomy and the decline in postoperative quality of life. (II) Prior retrospective studies typically involved the selection of a specific pathological type (e.g., adenocarcinoma, squamous cell carcinoma, or small cell carcinoma) based on postoperative pathological findings (36,38,39). This approach often introduced a significant selection bias. Although pathological classification can be obtained through preoperative procedures such as needle biopsy, bronchoscopy, or liquid biopsy (40), these methods also carry the risk of implantation metastasis (41) and may result in increased economic burden for patients. Wang et al. (41) also indicated that patients with early-stage, operable peripheral LC may benefit from forgoing a lung biopsy. Therefore, our current study was more aligned with the logic of clinical decision-making by encompassing a diverse array of benign and malignant pathological outcomes, thereby facilitating more precise clinical judgment prior to invasive examinations, minimizing patient discomfort, and enhancing economic efficiency.
However, our present also had several limitations: (I) selection bias could not be avoided due to its retrospective design. (II) It was carried out in a single center with a relatively small sample size. Furthermore, as surgical criteria may vary across different centers, external validation through multi-center and large-scale studies are warranted to further assess the reliability of the models. (III) Although potential confounding biases were mitigated by standardizing the collection parameters and employing the ICC method, biases still existed due to the absence of standardization in ROI delineation (as ROIs were manually delineated by physicians). In the future, artificial intelligence (AI) (42) may enhance the robustness and standardization of this process as it can automate ROI delineation or directly mine comprehensive features from the entire lungs (43). Admittedly, the current number of cases remains insufficient for AI. We intend to incorporate imaging and clinical data from multiple centers in the future for the purpose of conducting external validation. (IV) No cases of LSCC were enrolled in this analysis. On the one hand, the incidence of lung adenocarcinoma is significantly higher than that of LSCC, and the number of LSCC cases is small. Our future studies may include more patients for model optimization. On the other hand, LSCC is predominantly central in location and can be difficult to differentiate from the hilar structures on plain chest CT. Therefore, LSCC cases might have been removed due to poor image quality to avoid discrepancies.
Conclusions
In our present study, the RMs, which were based on the principles of radiomics, exhibited promising diagnostic performance in distinguishing benign and malignant lung nodules sized less than 3 cm in diameter and in determining their malignancy levels. The COMs, which were constructed upon a combination of independent predictors and RMs, outperformed CMs in diagnostic performance, showing potential for clinical application. With the use of these diagnostic models, nodules that are predicted to be benign can be managed with more conservative treatment strategies such as watch-and-wait. For low-grade malignant LC, procedures such as sublobectomy, including lung wedge resection and segmentectomy, can be employed as they can preserve lung function while ensuring the complete removal of the tumor. Furthermore, lymph node sampling can be employed to accurately stage the cancer and mitigate the risk of complications (e.g., lymphatic fistula) that may arise following lymph node dissection. Given the potential discrepancies between rapid intraoperative frozen pathology and conventional postoperative pathology, it is advisable to opt for radical lobectomy for LC cases with a high degree of malignancy to ensure a more thorough removal of tumor tissue. Additionally, systematic lymph node dissection can be performed to minimize the risk of residual cancer tissue recurrence within the lymphatic system and mitigate the pain associated with subsequent surgery due to the potential escalation of pathological grades.
Acknowledgments
None.
Footnote
Reporting Checklist: The authors have completed the TRIPOD reporting checklist. Available at https://jtd.amegroups.com/article/view/10.21037/jtd-2025-152/rc
Data Sharing Statement: Available at https://jtd.amegroups.com/article/view/10.21037/jtd-2025-152/dss
Peer Review File: Available at https://jtd.amegroups.com/article/view/10.21037/jtd-2025-152/prf
Funding: None.
Conflicts of Interest: All authors have completed the ICMJE uniform disclosure form (available at https://jtd.amegroups.com/article/view/10.21037/jtd-2025-152/coif). The authors have no conflicts of interest to declare.
Ethical Statement: The authors are accountable for all aspects of the work in ensuring that questions related to the accuracy or integrity of any part of the work are appropriately investigated and resolved. This study fully complied with the Declaration of Helsinki (2013 version) and was approved by the Ethics Committee of the First Affiliated Hospital of Soochow University (No. 2024668). As the analyzed data were anonymous and did not encroach on patient privacy, the need for obtaining signed informed consent was waived.
Open Access Statement: This is an Open Access article distributed in accordance with the Creative Commons Attribution-NonCommercial-NoDerivs 4.0 International License (CC BY-NC-ND 4.0), which permits the non-commercial replication and distribution of the article with the strict proviso that no changes or edits are made and the original work is properly cited (including links to both the formal publication through the relevant DOI and the license). See: https://creativecommons.org/licenses/by-nc-nd/4.0/.
References
- Siegel RL, Miller KD, Fuchs HE, et al. Cancer statistics, 2022. CA Cancer J Clin 2022;72:7-33. [PubMed]
- Sun Y, Ge X, Niu R, et al. PET/CT radiomics and deep learning in the diagnosis of benign and malignant pulmonary nodules: progress and challenges. Front Oncol 2024;14:1491762. [PubMed]
- Liang W, Tao J, Cheng C, et al. A clinically effective model based on cell-free DNA methylation and low-dose CT for risk stratification of pulmonary nodules. Cell Rep Med 2024;5:101750. [PubMed]
- Henschke CI, Yankelevitz DF, Mirtcheva R, et al. CT screening for lung cancer: frequency and significance of part-solid and nonsolid nodules. AJR Am J Roentgenol 2002;178:1053-7. [PubMed]
- Wang H, Weng Q, Hui J, et al. Value of TSCT Features for Differentiating Preinvasive and Minimally Invasive Adenocarcinoma From Invasive Adenocarcinoma Presenting as Subsolid Nodules Smaller Than 3 cm. Acad Radiol 2020;27:395-403. [PubMed]
- Hashimoto H, Matsumoto J, Murakami M, et al. Progressively increasing density of the solid center of a ground-glass nodule in a solitary pulmonary capillary hemangioma: A case report. Pathol Int 2020;70:568-73. [Crossref] [PubMed]
- Wu GF, Chen RC, Luo J, et al. Diagnostic accuracy of folate receptor-positive circulating tumor cells in differentiating between benign and malignant pulmonary nodules. Transl Cancer Res 2024;13:6982-94. [Crossref] [PubMed]
- Lambin P, Leijenaar RTH, Deist TM, et al. Radiomics: the bridge between medical imaging and personalized medicine. Nat Rev Clin Oncol 2017;14:749-62. [Crossref] [PubMed]
- Choi ER, Lee HY, Jeong JY, et al. Quantitative image variables reflect the intratumoral pathologic heterogeneity of lung adenocarcinoma. Oncotarget 2016;7:67302-13. [Crossref] [PubMed]
- Ye G, Wu G, Li K, et al. Development and Validation of a Deep Learning Radiomics Model to Predict High-Risk Pathologic Pulmonary Nodules Using Preoperative Computed Tomography. Acad Radiol 2024;31:1686-97. [Crossref] [PubMed]
- Onozato Y, Nakajima T, Yokota H, et al. Radiomics is feasible for prediction of spread through air spaces in patients with nonsmall cell lung cancer. Sci Rep 2021;11:13526. [Crossref] [PubMed]
- Hou X, Wu M, Chen J, et al. Establishment and verification of a prediction model based on clinical characteristics and computed tomography radiomics parameters for distinguishing benign and malignant pulmonary nodules. J Thorac Dis 2024;16:1984-95. [Crossref] [PubMed]
- Yang F, Dong Z, Shen Y, et al. Cribriform growth pattern in lung adenocarcinoma: More aggressive and poorer prognosis than acinar growth pattern. Lung Cancer 2020;147:187-92. [Crossref] [PubMed]
- Willner J, Narula N, Moreira AL. Updates on lung adenocarcinoma: invasive size, grading and STAS. Histopathology 2024;84:6-17. [Crossref] [PubMed]
- Zhang Y, Deng C, Zheng Q, et al. Selective Mediastinal Lymph Node Dissection Strategy for Clinical T1N0 Invasive Lung Cancer: A Prospective, Multicenter, Clinical Trial. J Thorac Oncol 2023;18:931-9. [Crossref] [PubMed]
- Nakagawa K, Yotsukura M, Mimae TThe Lung Cancer Surgical Study Group of the Japan Clinical Oncology Group, et al. outstanding contribution and entering a new phase. Jpn J Clin Oncol 2024;54:1237-43. [Crossref] [PubMed]
- Tsuchida H, Tanahashi M, Suzuki E, et al. Pathologically noninvasive cancer predictors and surgical procedure for peripheral lung cancer. Thorac Cancer 2023;14:289-97. [Crossref] [PubMed]
- Rios Velazquez E, Parmar C, Liu Y, et al. Somatic Mutations Drive Distinct Imaging Phenotypes in Lung Cancer. Cancer Res 2017;77:3922-30. [Crossref] [PubMed]
- Gong J, Liu J, Li H, et al. Deep Learning-Based Stage-Wise Risk Stratification for Early Lung Adenocarcinoma in CT Images: A Multi-Center Study. Cancers (Basel) 2021;13:3300. [Crossref] [PubMed]
- Xu S, Ge J, Liu X, et al. The predictive value of chest computed tomography images, tumor markers, and metabolomics in the identification of benign and malignant pulmonary nodules. J Thorac Dis 2023;15:2668-79. [Crossref] [PubMed]
- Li N, Tan F, Chen W, et al. One-off low-dose CT for lung cancer screening in China: a multicentre, population-based, prospective cohort study. Lancet Respir Med 2022;10:378-91. [Crossref] [PubMed]
- Salome P, Sforazzini F, Grugnara G, et al. MR Intensity Normalization Methods Impact Sequence Specific Radiomics Prognostic Model Performance in Primary and Recurrent High-Grade Glioma. Cancers (Basel) 2023;15:965. [Crossref] [PubMed]
- Clément-Duchêne C, Vignaud JM, Stoufflet A, et al. Characteristics of never smoker lung cancer including environmental and occupational risk factors. Lung Cancer 2010;67:144-50. [Crossref] [PubMed]
- Siegfried JM. Sex and Gender Differences in Lung Cancer and Chronic Obstructive Lung Disease. Endocrinology 2022;163:bqab254. [Crossref] [PubMed]
- Florez N, Kiel L, Riano I, et al. Lung Cancer in Women: The Past, Present, and Future. Clin Lung Cancer 2024;25:1-8. [Crossref] [PubMed]
- Zhang Y, Fu F, Chen H. Management of Ground-Glass Opacities in the Lung Cancer Spectrum. Ann Thorac Surg 2020;110:1796-804. [Crossref] [PubMed]
- Wu F, Tian SP, Jin X, et al. CT and histopathologic characteristics of lung adenocarcinoma with pure ground-glass nodules 10 mm or less in diameter. Eur Radiol 2017;27:4037-43. [Crossref] [PubMed]
- Takashima S, Maruyama Y, Hasegawa M, et al. CT findings and progression of small peripheral lung neoplasms having a replacement growth pattern. AJR Am J Roentgenol 2003;180:817-26. [Crossref] [PubMed]
- Tu Y, Wu Y, Lu Y, et al. Development of risk prediction models for lung cancer based on tumor markers and radiological signs. J Clin Lab Anal 2021;35:e23682. [Crossref] [PubMed]
- Lee SM, Park CM, Goo JM, et al. Invasive pulmonary adenocarcinomas versus preinvasive lesions appearing as ground-glass nodules: differentiation by using CT features. Radiology 2013;268:265-73. [Crossref] [PubMed]
- Li H, Luo Q, Zheng Y, et al. A nomogram for predicting invasiveness of lung adenocarcinoma manifesting as ground-glass nodules based on follow-up CT imaging. Transl Lung Cancer Res 2024;13:2617-35. [PubMed]
- He XQ, Huang XT, Luo TY, et al. The differential computed tomography features between small benign and malignant solid solitary pulmonary nodules with different sizes. Quant Imaging Med Surg 2024;14:1348-58. [PubMed]
- Chang B, Hwang JH, Choi YH, et al. Natural history of pure ground-glass opacity lung nodules detected by low-dose CT scan. Chest 2013;143:172-8. [PubMed]
- Kou J, Gu X, Kang L. Correlation Analysis of Computed Tomography Features and Pathological Types of Multifocal Ground-Glass Nodular Lung Adenocarcinoma. Comput Math Methods Med 2022;2022:7267036. [Crossref] [PubMed]
- Song YS, Park CM, Park SJ, et al. Volume and mass doubling times of persistent pulmonary subsolid nodules detected in patients without known malignancy. Radiology 2014;273:276-84. [Crossref] [PubMed]
- Aokage K, Suzuki K, Saji H, et al. Segmentectomy for ground-glass-dominant lung cancer with a tumour diameter of 3 cm or less including ground-glass opacity (JCOG1211): a multicentre, single-arm, confirmatory, phase 3 trial. Lancet Respir Med 2023;11:540-9. [Crossref] [PubMed]
- Jiang S, Wang B, Zhang M, et al. Quality of life after lung cancer surgery: sublobar resection versus lobectomy. BMC Surg 2023;23:353. [Crossref] [PubMed]
- Liu C, Zhao W, Xie J, et al. Development and validation of a radiomics-based nomogram for predicting a major pathological response to neoadjuvant immunochemotherapy for patients with potentially resectable non-small cell lung cancer. Front Immunol 2023;14:1115291. [PubMed]
- Perez-Johnston R, Araujo-Filho JA, Connolly JG, et al. CT-based Radiogenomic Analysis of Clinical Stage I Lung Adenocarcinoma with Histopathologic Features and Oncologic Outcomes. Radiology 2022;303:664-72. [PubMed]
- Malapelle U, Pisapia P, Pepe F, et al. The evolving role of liquid biopsy in lung cancer. Lung Cancer 2022;172:53-64. [PubMed]
- Wang Z, Chen C, Fang L, et al. The probability of implantation metastasis after peripheral lung cancer biopsy. Transl Cancer Res 2024;13:4654-8. [Crossref] [PubMed]
- Binczyk F, Prazuch W, Bozek P, et al. Radiomics and artificial intelligence in lung cancer screening. Transl Lung Cancer Res 2021;10:1186-99. [Crossref] [PubMed]
- Liu J, Qi L, Wang Y, et al. Diagnostic performance of a deep learning-based method in differentiating malignant from benign subcentimeter (≤10 mm) solid pulmonary nodules. J Thorac Dis 2023;15:5475-84. [Crossref] [PubMed]