Construction and analysis of the invasive prediction model for pulmonary nodules: based on clinical, CT image and DNA methylation characteristics
Highlight box
Key findings
• Construct and validate a statistical model that can accurately predict whether pulmonary nodules have entered the microinvasive or invasive carcinoma (MIC/IC) stage.
What is known and what is new?
• At present, there are many nodule prediction models, but there is no model specifically used to distinguish whether pulmonary nodules have entered the MIC/IC.
• This study has constructed and validated a model specifically for identifying whether pulmonary nodules have entered the phase MIC/IC, which has incorporated two computed tomography (CT) image features and four methylation site features (proportion of solid components, maximum CT value, SH3BP5_338_ CpG 4, PNPLA2_329_CpG 1, PNPLA2_329_CpG 4, and ARHGAP35 476_CpG_5). The area under the curve was 0.90, prediction accuracy was 82%, sensitivity was 82%, specificity was 82%.
What is the implication, and what should change now?
• The invasiveness predictive model for pulmonary nodules constructed by combining clinical, CT image, and methylation features in is worthy of further exploration and improvement. It is of great significance for the clinical formulation of reasonable treatment plans.
Introduction
Early diagnosis and early treatment are the keys to improving the therapeutic effect of lung cancer. Therefore, it is crucial to accurately distinguish the benign and malignant nature of pulmonary nodules detected by chest computed tomography (CT) and to handle malignant nodules as early as possible. Among them, atypical adenomatous hyperplasia (AAH), adenocarcinoma in situ (AIS), microinvasive adenocarcinoma (MIA), and invasive carcinoma (IC) are the four most common pathological types of surgically resected pulmonary nodules. AAH and AIS are precursor lesions, and their progression is relatively slow. Patients can choose the time for surgical treatment more calmly or undergo long-term follow-up observations to rule out non-neoplastic lesions. However, MIA and IC require prompt surgery or other treatments to prevent progression to advanced-stage lung cancer. Therefore, accurately differentiating whether pulmonary nodules have entered the invasive stage is of great clinical significance (1).
Nevertheless, it is difficult to accurately identify whether pulmonary nodules have entered the invasive stage based solely on CT images. The reported predictive models for pulmonary nodules based on clinical features and CT images all classify AIS and MIA together as malignant lung cancer for differentiation from benign nodules, and there is no model specifically for differentiating AIS and MIA (2-6). There is an urgent clinical need for a more accurate method to distinguish the nature of pulmonary nodules.
Deoxyribonucleic acid (DNA) methylation testing is one of the methods used to detect early lung cancer (7-9). Currently, some studies have combined the clinical features, CT image features, and DNA methylation test results of patients to construct statistical models to determine the nature of pulmonary nodules (10,11). Among them, the report by the He’s et al. (11) indicated that through the statistical model, 89% (105/118) of unnecessary surgeries and 73% (308/423) of delayed treatments could be reduced. However, this statistical model is complex, involving dozens of CT image features, and it still requires some time for clinical promotion and application. Moreover, most of the existing similar studies still analyze AIS together with MIC and IC as malignant tumors, and there is no model specifically for differentiating AIS from MICs (12,13).
We have been collecting the clinical features, CT image features, and DNA methylation test data of patients with pulmonary nodules undergoing surgical operations in our hospital since January 2022. Based on the premise of high accuracy, simplicity of use, and strong clinical applicability, we constructed a predictive model for the invasiveness of pulmonary nodules. Finally, relatively satisfactory results were obtained, and they are reported as follows. We present this article in accordance with the TRIPOD reporting checklist (available at https://jtd.amegroups.com/article/view/10.21037/jtd-24-1763/rc).
Methods
Patient screening and grouping
This study was registered at Chinese Clinical Trial Registry (ChiCTR) (identifier: ChiCTR2300067261). Patient data of those who underwent thoracoscopic pulmonary wedge resection or pulmonary segmentectomy due to pulmonary nodules at Xiamen Humanity Hospital of Fujian Medical University from January 2022 to December 2023 were collected. Inclusion criteria: (I) undergo surgery due to pulmonary nodules; (II) the maximum diameter of the pulmonary nodules was <2 cm; (III) only one pulmonary nodule was considered possibly malignant and was resected during this surgery; (IV) no biopsy was performed on the pulmonary nodules; (V) the postoperative pathology was AAH, AIS, MIA, IC or other benign lesions such as fibrous nodules. Exclusion criteria: (I) multiple pulmonary nodules, with some nodules of undetermined nature and not resected during this surgery; (II) staged surgeries for bilateral pulmonary nodules; (III) factors that might affect the results of DNA methylation testing: (i) having autoimmune diseases (such as systemic lupus erythematosus, rheumatoid arthritis, Hashimoto’s thyroiditis, scleroderma, polyarteritis nodosa, etc.); (ii) active pulmonary tuberculosis; (iii) females who were pregnant or within half a year after delivery; (iv) having taken leukocyte-increasing drugs (such as Leucogen, Batilol, and Vitamin B4, etc.) within the past 45 days, or having taken health products containing ingredients of leukocyte-increasing drugs; (v) having undergone any other surgeries within six months; (vi) having other uncured cancers or a history of other cancers within 3 years; (vii) having a history of major diseases such as cerebral infarction, cerebral hemorrhage, and myocardial infarction within 3 years; (viii) the results of blood routine tests performed simultaneously with the collection of blood samples for methylation testing exceeded the normal range [white blood cell count (3.5–9.5)×109/L, lymphocyte count (1.1–3.2)×109/L, neutrophil count (1.8–6.3)×109/L, basophil count (0.00–0.06)×109/L, eosinophil count (0.02–0.52)×109/L, and monocyte count (0.1–0.6)×109/L].
The eligible patients were divided into the invasive nodules group (IN group) and the non-invasive nodules group (non-IN group) according to the postoperative pathological results. The non-IN group included: AAH, AIS or other benign lesions such as fibrous nodules. The IN group included: MIA and IC.
A total of 294 patients were enrolled, including 183 in the IN group and 111 in the non-IN group. See Table 1. The study was conducted in accordance with the Declaration of Helsinki (as revised in 2013). This study was approved by the Medical Ethics Committee of Xiamen Humanity Hospital of Fujian Medical University (No. HAXM-MEC-20221201-035-01), and informed consent was taken from all individual participants or their legal guardians.
Table 1
Variables | Total (n=294) | Training set (n=221) | Validation set (n=73) | Statistic | P |
---|---|---|---|---|---|
Age (years) | 52.98±11.93 | 53.40±11.79 | 51.71±12.33 | t*=1.05 | 0.30 |
Gender | χ²**=0.04 | 0.84 | |||
Male | 138 (46.94) | 103 (46.61) | 35 (47.95) | ||
Female | 156 (53.06) | 118 (53.39) | 38 (52.05) | ||
Smoking history | χ²=0.17 | 0.68 | |||
0 (none) | 273 (92.86) | 206 (93.21) | 67 (91.78) | ||
1 (have) | 21 (7.14) | 15 (6.79) | 6 (8.22) | ||
Maximum diameter of pulmonary nodules (mm) |
11.45±4.96 | 11.71±4.79 | 10.64±5.39 | t=1.60 | 0.11 |
Consolidation tumor ratio (%) | 19.17±31.77 | 19.25±31.63 | 18.95±32.41 | t=0.07 | 0.94 |
Maximum CT value (Hu) | −144.03±292.77 | −142.29±286.11 | −149.27±314.09 | t=0.18 | 0.86 |
Benign features | χ²=0.60 | 0.44 | |||
0 (none) | 261 (88.78) | 198 (89.59) | 63 (86.30) | ||
1 (one or more) | 33 (11.22) | 23 (10.41) | 10 (13.70) | ||
Malignant features | χ²=0.41 | 0.52 | |||
0 (none) | 213 (72.45) | 158 (71.49) | 55 (75.34) | ||
1 (one or more) | 81 (27.55) | 63 (28.51) | 18 (24.66) | ||
Location of pulmonary nodules | χ²=2.27 | 0.69 | |||
1 (right upper lobe) | 81 (27.55) | 62 (28.05) | 19 (26.03) | ||
2 (right middle lobe) | 24 (8.16) | 19 (8.60) | 5 (6.85) | ||
3 (right lower lobe) | 48 (16.33) | 34 (15.38) | 14 (19.18) | ||
4 (left upper lobe) | 81 (27.55) | 64 (28.96) | 17 (23.29) | ||
5 (left lower lobe) | 60 (20.41) | 42 (19.00) | 18 (24.66) | ||
Shape of pulmonary nodules | χ²=1.24 | 0.74 | |||
1 (circular-like and well-defined border) | 165 (56.12) | 128 (57.92) | 37 (50.68) | ||
2 (irregular and well-defined border) | 48 (16.33) | 34 (15.38) | 14 (19.18) | ||
3 (circular-like and ill-defined border) | 51 (17.35) | 37 (16.74) | 14 (19.18) | ||
4 (irregular and ill-defined border) | 30 (10.20) | 22 (9.95) | 8 (10.96) | ||
Pathological diagnosis | – | – | |||
Other benign lesions | 12 | 8 | 4 | ||
AAH | 21 | 14 | 7 | ||
AIS | 78 | 54 | 24 | ||
MIA | 123 | 99 | 24 | ||
IC | 60 | 46 | 14 | ||
FYB CpG 2, 3 | 0.62±0.07 | 0.62±0.07 | 0.63±0.07 | t=−1.02 | 0.31 |
FYB CpG 4 | 0.68±0.11 | 0.68±0.11 | 0.69±0.10 | t=−0.39 | 0.70 |
FYB CpG 7 | 0.44±0.17 | 0.44±0.17 | 0.43±0.16 | t=0.20 | 0.84 |
FYB CpG 8 | 0.46±0.09 | 0.46±0.09 | 0.47±0.09 | t=−0.07 | 0.94 |
FYB CpG 9 | 0.27±0.09 | 0.27±0.09 | 0.27±0.09 | t=−0.09 | 0.93 |
FYB CpG 10, 11, 12 | 0.63±0.06 | 0.62±0.06 | 0.64±0.06 | t=−1.67 | 0.10 |
SH3BP5 338 CpG 1 | 0.31±0.10 | 0.31±0.10 | 0.32±0.09 | t=−1.09 | 0.28 |
SH3BP5 338 CpG 2 | 0.39±0.08 | 0.39±0.09 | 0.40±0.08 | t=−1.57 | 0.12 |
SH3BP5 338 CpG 4 | 0.62±0.21 | 0.62±0.21 | 0.59±0.21 | t=1.21 | 0.23 |
RAPSN 348 CpG 1 | 0.33±0.16 | 0.32±0.16 | 0.36±0.18 | t=−1.49 | 0.14 |
RAPSN 348 CpG 4 | 0.40±0.11 | 0.40±0.11 | 0.42±0.11 | t=−1.04 | 0.30 |
RAPSN 348 CpG 5 | 0.42±0.19 | 0.41±0.19 | 0.45±0.20 | t=−1.71 | 0.09 |
PNPLA2 329 CpG 1 | 0.80±0.08 | 0.80±0.08 | 0.81±0.08 | t=−1.38 | 0.17 |
PNPLA2 329 CpG 2 | 0.38±0.21 | 0.37±0.21 | 0.41±0.20 | t=−1.17 | 0.24 |
PNPLA2 329 CpG 3 | 0.33±0.15 | 0.33±0.16 | 0.33±0.14 | t=−0.22 | 0.83 |
PNPLA2 329 CpG 4 | 0.30±0.12 | 0.29±0.13 | 0.31±0.10 | t=−1.21 | 0.23 |
ARHGAP35 476 CpG 1 | 0.78±0.10 | 0.78±0.10 | 0.80±0.09 | t=−1.09 | 0.28 |
ARHGAP35 476 CpG 2 | 0.70±0.13 | 0.70±0.13 | 0.72±0.10 | t=−1.50 | 0.14 |
ARHGAP35 476 CpG 3 | 0.24±0.11 | 0.23±0.11 | 0.26±0.10 | t=−1.52 | 0.13 |
ARHGAP35 476 CpG 4 | 0.69±0.17 | 0.68±0.18 | 0.71±0.15 | t=−1.20 | 0.23 |
ARHGAP35 476 CpG 5 | 0.74±0.18 | 0.74±0.18 | 0.73±0.19 | t=0.41 | 0.68 |
ARHGAP35 476 CpG 7 | 0.77±0.10 | 0.77±0.10 | 0.79±0.09 | t=−1.21 | 0.23 |
ARHGAP35 476 CpG 8 | 0.77±0.14 | 0.77±0.14 | 0.77±0.15 | t=0.16 | 0.88 |
Data are presented as mean ± SD or n (%). *t, t-test; **χ², Chi-squared test. AAH, atypical adenomatous hyperplasia; AIS, adenocarcinoma in situ; CT, computed tomography; IC, invasive carcinoma; MIA, microinvasive adenocarcinoma; SD, standard deviation.
DNA methylation detection
When patients were admitted to the hospital for preoperative blood test and blood collection, 2 mL of peripheral blood was collected using ethylenediaminetetraacetic acid (EDTA) tubes. All blood samples were immediately stored in a −80 ℃ refrigerator after blood collection. DNA was extracted from the blood using a DNA extraction kit (TANTICA, Nanjing, China).
Bisulfite conversion: The DNA of each sample was subjected to bisulfite reaction conversion using the EZ-96 DNA Methylation Gold Kit (Zymo Research, Orange, USA) according to the manufacturer’s instructions. Bisulfite treatment can convert unmethylated cytosine (C) at CpG sites to uracil (U), while methylated cytosine remains unchanged.
Matrix-assisted laser desorption ionization time-of-flight (MALDI-TOF) mass spectrometry: polymerase chain reaction (PCR) was used to amplify the amplicons of 23 methylation sites in 5 genes (see Table 2 for details, hereinafter referred to as 23 sites). The PCR products were incubated with shrimp alkaline phosphatase (SAP), processed by T-cut assay (Agena Bioscience, San Diego, California, USA), and further purified by resin. The final product was transferred to SpectroCHIP G384 and detected by MALDI-TOF mass spectrometry (MassARRAY® Nucleic Acid Mass Spectrometry System, Agena Bioscience, San Diego, California, USA). The quantitative methylation level of each CpG site was collected by SpectroACQUIRE v3.3.1.3 software and visualized by EpiTyper v1.3 software.
Table 2
Genes | Methylation sites |
---|---|
FYB | FYB_CpG_2.3, FYB_CpG_4, FYB_CpG_7, FYB_CpG_8, FYB_CpG_9, FYB_CpG_10.11.12 |
SH3BP5_338 | SH3BP5_338_CpG_1, SH3BP5_338_CpG_2, SH3BP5_338_CpG_4 |
RAPSN_348 | RAPSN_348_CpG_1, RAPSN_348_CpG_4, RAPSN_348_CpG_5 |
PNPLA2_329 | PNPLA2_329_CpG_1, PNPLA2_329_CpG_2, PNPLA2_329_CpG_3, PNPLA2_329_CpG_4 |
ARHGAP35_476 | ARHGAP35_476_CpG_1, ARHGAP35_476_CpG_2, ARHGAP35_476_CpG_3, ARHGAP35_476_CpG_4, ARHGAP35_476_CpG_5, ARHGAP35_476_CpG_7, ARHGAP35_476_CpG_8 |
The DNA methylation detection in this study was completed by Nanjing Tengchen Biotechnology Co., Ltd. See Table 2.
Observation indicator
(I) Clinical characteristics of patients: age, gender, smoking history, surgical procedure, and postoperative pathology. (II) Image features of pulmonary nodules: location of pulmonary nodules, maximum diameter of pulmonary nodule, consolidation tumor ratio (CTR), maximum CT value, shape of pulmonary nodules, benign features, and malignant features. (III) Detection results of 23 methylation sites of 5 genes.
Some definitions of the observation indicators are as follows: (I) CTR: the ratio of the maximum diameter of the solid component of the pulmonary nodule to the maximum diameter of the pulmonary nodule on thin-layer CT scans (14). (II) Maximum CT value: the maximum CT value of the entire pulmonary nodule, but the measurement point should not include the blood vessels, bronchi running within the nodule, and the pleura at the edge of the nodule. (III) Shape of pulmonary nodules (15): including four types: round-like with clear boundaries, irregular with clear boundaries, round-like with unclear boundaries, and irregular with unclear boundaries. (IV) Benign features (15,16): whether there are benign features on the CT images of pulmonary nodules. Benign features include: calcification foci and cord-like shadows. (V) Malignant features (15,16): whether there are malignant features on the CT images of pulmonary nodules. Malignant features include: lobulation sign, vascular convergence sign, short spicule sign, pleural traction sign, halo sign, vacuole sign, and bronchial truncation sign.
Statistical analysis
Statistical analysis was performed using R language (version 4.2.3). Measurement data of normal distribution were expressed as mean ± standard deviation (), measurement data with non-normal distribution were expressed as the median and interquartile range (IQR), and categorical data were expressed as counts (composition ratios). The t-test was used for continuous variables, and the Chi-squared test or Fisher’s exact probability method was used for categorical variables. The test level was α=0.05.
Pmsampsize R package (15,16) was used to estimate the sample size required for constructing the model, for binary outcomes (logistic prediction models), with parameters r-squared (17,18) =0.74, parameters =33, and prevalence =0.378. It is estimated that the required sample size is greater than 159 cases.
Using the random sampling operation of R language, 1/4 of the total dataset was randomly sampled as the validation set, and the remaining 3/4 was the training set. The equilibrium test was conducted on the data of the two sets. In the training set, firstly, t-tests or Chi-squared tests were conducted on the patients’ clinical characteristics, image features of pulmonary nodules, and the detection results of 23 methylation sites to screen out the observation indicators with statistical differences. Secondly, the screened indicators were divided into three groups: clinical and image features, methylation features, and comprehensive features combining the two, and univariate logistic regression was performed. Thirdly, the variables with significance in the univariate analysis (P<0.05) were included in the multivariate Logistic regression analysis to further screen out the observation indicators with statistical differences. The test level was set as α=0.05. The indicators screened by the multivariate logistic regression of the three groups in the training set were respectively used to construct the prediction model of pulmonary nodule invasion and verified in the validation set. Finally, the model effect was evaluated using indicators such as receiver operating characteristic (ROC) curve, area under the curve (AUC), sensitivity, specificity, Hosmer-Lemeshow test, and calibration curve.
Results
Basic information of patients
A total of 221 patients were included in the training set, among which 145 cases were in the IN group and 76 cases were in the non-IN group. There were 73 cases in the validation set, including 38 cases in the IN group and 35 cases in the non-IN group. The two data sets were balanced. See Table 1.
Variable screening of the training set
The t-test or Chi-squared test was conducted to analyze the clinical features, image features of pulmonary nodules, and the test results of 23 methylation sites in patients of the IN group and the non-IN group in the training set. The results showed that there were 13 observation indicators with P<0.05: age (P=0.004), maximum diameter of pulmonary nodules (P=0.005), CTR (P<0.001), maximum CT value (P<0.001), shape of pulmonary nodules (P=0.04), malignant features (P=0.04), FYB_CpG_4 (P=0.02), FYB_CpG_7 (P=0.01), SH3BP5_338_CpG_4 (P=0.01), RAPSN_348_CpG_5 (P=0.03), PNPLA2_329_CpG_1 (P=0.02), PNPLA2_329_CpG_4 (P=0.002), ARHGAP35_476_CpG_5 (P<0.001). See Table 3.
Table 3
Variables | Total (n=221) | Non-IN group (n=76) | IN group (n=145) | Statistic | P |
---|---|---|---|---|---|
Age (years) | 53.40±11.79 | 50.28±10.78 | 55.03±12.00 | t*=−2.90 | 0.004 |
Gender | χ²**=0.94 | 0.33 | |||
Male | 103 (46.61) | 32 (42.11) | 71 (48.97) | ||
Female | 118 (53.39) | 44 (57.89) | 74 (51.03) | ||
Smoking history | χ²=2.56 | 0.11 | |||
0 (none) | 206 (93.21) | 68 (89.47) | 138 (95.17) | ||
1 (have) | 15 (6.79) | 8 (10.53) | 7 (4.83) | ||
Maximum diameter of pulmonary nodules (mm) | 11.71±4.79 | 10.46±5.08 | 12.37±4.51 | t=−2.86 | 0.005 |
Consolidation tumor ratio (%) | 19.25±31.63 | 5.53±22.47 | 26.44±33.38 | t=−5.53 | <0.001 |
Maximum CT value (Hu) | −142.29±286.11 | −353.34±203.53 | −31.68±260.11 | t=−10.11 | <0.001 |
Benign features | χ²=3.60 | 0.06 | |||
0 (none) | 198 (89.59) | 64 (84.21) | 134 (92.41) | ||
1 (one or more) | 23 (10.41) | 12 (15.79) | 11 (7.59) | ||
Malignant features | χ²=4.37 | 0.04 | |||
0 (none) | 158 (71.49) | 61 (80.26) | 97 (66.90) | ||
1 (one or more) | 63 (28.51) | 15 (19.74) | 48 (33.10) | ||
Location of pulmonary nodules | χ²=2.53 | 0.64 | |||
1 (right upper lobe) | 62 (28.05) | 21 (27.63) | 41 (28.28) | ||
2 (right middle lobe) | 19 (8.60) | 4 (5.26) | 15 (10.34) | ||
3 (right lower lobe) | 34 (15.38) | 14 (18.42) | 20 (13.79) | ||
4 (left upper lobe) | 64 (28.96) | 21 (27.63) | 43 (29.66) | ||
5 (left lower lobe) | 42 (19.00) | 16 (21.05) | 26 (17.93) | ||
Shape of pulmonary nodules | χ²=8.13 | 0.04 | |||
1 (circular-like and well-defined border) | 128 (57.92) | 52 (68.42) | 76 (52.41) | ||
2 (irregular and well-defined border) | 34 (15.38) | 12 (15.79) | 22 (15.17) | ||
3 (circular-like and ill-defined border) | 37 (16.74) | 6 (7.89) | 31 (21.38) | ||
4 (irregular and ill-defined border) | 22 (9.95) | 6 (7.89) | 16 (11.03) | ||
FYB CpG 2, 3 | 0.62±0.07 | 0.62±0.06 | 0.62±0.07 | t=0.09 | 0.93 |
FYB CpG 4 | 0.68±0.11 | 0.66±0.11 | 0.69±0.10 | t=−2.46 | 0.02 |
FYB CpG 7 | 0.44±0.17 | 0.40±0.14 | 0.46±0.18 | t=−2.47 | 0.01 |
FYB CpG 8 | 0.46±0.09 | 0.46±0.09 | 0.47±0.10 | t=−1.10 | 0.27 |
FYB CpG 9 | 0.27±0.09 | 0.26±0.08 | 0.28±0.09 | t=−1.09 | 0.28 |
FYB CpG 10, 11, 12 | 0.62±0.06 | 0.62±0.06 | 0.62±0.07 | t=0.29 | 0.77 |
SH3BP5 338 CpG 1 | 0.31±0.10 | 0.32±0.08 | 0.30±0.10 | t=1.00 | 0.32 |
SH3BP5 338 CpG 2 | 0.39±0.09 | 0.39±0.07 | 0.38±0.09 | t=0.83 | 0.41 |
SH3BP5 338 CpG 4 | 0.62±0.21 | 0.57±0.21 | 0.65±0.21 | t=−2.58 | 0.01 |
RAPSN 348 CpG 1 | 0.32±0.16 | 0.34±0.15 | 0.32±0.16 | t=0.97 | 0.33 |
RAPSN 348 CpG 4 | 0.40±0.11 | 0.40±0.10 | 0.40±0.11 | t=−0.17 | 0.87 |
RAPSN 348 CpG 5 | 0.41±0.19 | 0.45±0.17 | 0.39±0.20 | t=2.14 | 0.03 |
PNPLA2 329 CpG 1 | 0.80±0.08 | 0.81±0.08 | 0.79±0.07 | t=2.36 | 0.02 |
PNPLA2 329 CpG 2 | 0.37±0.21 | 0.41±0.21 | 0.36±0.21 | t=1.90 | 0.06 |
PNPLA2 329 CpG 3 | 0.33±0.16 | 0.34±0.13 | 0.32±0.17 | t=0.99 | 0.33 |
PNPLA2 329 CpG 4 | 0.29±0.13 | 0.32±0.09 | 0.27±0.14 | t=3.14 | 0.002 |
ARHGAP35 476 CpG 1 | 0.78±0.10 | 0.77±0.09 | 0.78±0.11 | t=−0.70 | 0.48 |
ARHGAP35 476 CpG 2 | 0.70±0.13 | 0.70±0.09 | 0.70±0.15 | t=−0.27 | 0.79 |
ARHGAP35 476 CpG 3 | 0.23±0.11 | 0.23±0.11 | 0.23±0.12 | t=0.04 | 0.96 |
ARHGAP35 476 CpG 4 | 0.68±0.18 | 0.67±0.16 | 0.68±0.18 | t=−0.54 | 0.59 |
ARHGAP35 476 CpG 5 | 0.74±0.18 | 0.68±0.19 | 0.77±0.17 | t=−3.87 | <0.001 |
ARHGAP35 476 CpG 7 | 0.77±0.10 | 0.78±0.08 | 0.76±0.10 | t=1.37 | 0.17 |
ARHGAP35 476 CpG 8 | 0.77±0.14 | 0.75±0.14 | 0.78±0.14 | t=−1.72 | 0.09 |
Data are presented as mean ± SD or n (%). *t, t-test; **χ², Chi-square test. CT, computed tomography; IN, invasive nodules; SD, standard deviation.
Logistic regression analysis and model construction
Clinical and image features model
Univariate logistic regression was performed on the above screened clinical and image features. The results showed that there were six observation indicators with P<0.05: age (P=0.005), maximum diameter of pulmonary nodules (P=0.006), CTR (P<0.001), maximum CT value (P<0.001), shape of pulmonary nodules (P=0.009), and malignant features (P=0.04). Further multivariate logistic regression analysis was conducted, and the results showed that only the two observation indicators with P<0.05: CTR (P=0.02) and maximum CT value (P<0.001). Binary logistic regression analysis was performed on these two indicators, and an invasive prediction model for pulmonary nodules was constructed. Malignant probability = ex/(1 + ex); x = 2.79 − (0.02 × CTR) + (0.01 × maximum CT value), where e is the natural logarithm. The effect of this model was evaluated. The AUC of the training set and the validation set were 0.83 and 0.85, respectively. With 0.594 as the cut-off value, the prediction accuracies were 78% and 79%, the sensitivities were 71% and 74%, and the specificities were 81% and 84%, respectively. See Tables 4,5 and Figures 1,2.
Table 4
Logistic regression analysis | Variables | β | S.E | Z | P | OR (95% CI) |
---|---|---|---|---|---|---|
Univariate | Age | 0.03 | 0.01 | 2.81 | 0.005 | 1.04 (1.01–1.06) |
Maximum diameter of pulmonary nodules | 0.11 | 0.04 | 2.72 | 0.006 | 1.11 (1.03–1.20) | |
Consolidation tumor ratio | 0.04 | 0.01 | 3.98 | <0.001 | 1.04 (1.02–1.05) | |
Maximum CT value | 0.01 | 0.00 | 6.40 | <0.001 | 1.01 (1.01–1.01) | |
Malignant features | ||||||
• 0 (none) | 1.00 (reference) | |||||
• 1 (one or more) | 0.70 | 0.34 | 2.07 | 0.04 | 2.01 (1.04–3.90) | |
Shape of pulmonary nodules | ||||||
• 1 (circular-like and well-defined border) | 1.00 (reference) | |||||
• 2 (irregular and well-defined border) | 0.23 | 0.40 | 0.56 | 0.57 | 1.25 (0.57–2.76) | |
• 3 (circular-like and ill-defined border) | 1.26 | 0.48 | 2.63 | 0.009 | 3.54 (1.38–9.07) | |
• 4 (irregular and ill-defined border) | 0.60 | 0.51 | 1.18 | 0.24 | 1.82 (0.67–4.97) | |
Multivariate (bidirectional stepwise regression) | Intercept | 2.79 | 0.50 | 5.61 | <0.001 | 16.24 (6.13–43.05) |
Consolidation tumor ratio | −0.02 | 0.01 | −2.38 | 0.02 | 0.98 (0.96–0.99) | |
Maximum CT value | 0.01 | 0.00 | 5.72 | <0.001 | 1.01 (1.01–1.01) |
CT, computed tomography; CI, confidence interval; OR, odds ratio; S.E, standard error.
Table 5
Model | AUC (95% CI) | Accuracy (95% CI) | Sensitivity (95% CI) | Specificity (95% CI) | PPV (95% CI) | NPV (95% CI) | Cut off |
---|---|---|---|---|---|---|---|
Clinical and imaging feature model | |||||||
Training set | 0.83 (0.78–0.89) | 0.78 (0.72–0.83) | 0.71 (0.61–0.81) | 0.81 (0.75–0.88) | 0.67 (0.56–0.77) | 0.84 (0.78–0.90) | 0.594 |
Validation set | 0.85 (0.76–0.94) | 0.79 (0.68–0.88) | 0.74 (0.60–0.89) | 0.84 (0.73–0.96) | 0.81 (0.68–0.95) | 0.78 (0.65–0.91) | 0.594 |
Methylation feature model | |||||||
Training set | 0.78 (0.71–0.84) | 0.76 (0.70–0.81) | 0.72 (0.62–0.82) | 0.78 (0.71–0.85) | 0.63 (0.53–0.73) | 0.84 (0.78–0.90) | 0.596 |
Validation set | 0.74 (0.63–0.86) | 0.71 (0.59–0.81) | 0.77 (0.63–0.91) | 0.66 (0.51–0.81) | 0.68 (0.53–0.82) | 0.76 (0.61–0.90) | 0.596 |
Comprehensive feature model | |||||||
Training set | 0.90 (0.85–0.94) | 0.82 (0.76–0.87) | 0.82 (0.73–0.90) | 0.82 (0.76–0.88) | 0.70 (0.61–0.80) | 0.89 (0.84–0.95) | 0.619 |
Validation set | 0.87 (0.78–0.95) | 0.82 (0.71–0.90) | 0.80 (0.67–0.93) | 0.84 (0.73–0.96) | 0.82 (0.70–0.95) | 0.82 (0.70–0.94) | 0.619 |
AUC, area under the curve; CI, confidence interval; PPV, positive predictive value; NPV, negative predictive value.
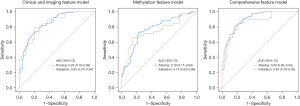
Methylation features model
Univariate logistic regression was performed on the methylation features screened above (variable screening of the training set). The results showed that there were seven observation indicators with P<0.05: FYB_CpG_4 (P=0.02), FYB_CpG_7 (P=0.02), SH3BP5_338_CpG_4 (P=0.01), RAPSN_348_CpG_5 (P=0.04), PNPLA2_329_CpG_1 (P=0.02), PNPLA2_329_CpG_4 (P=0.008), and ARHGAP35_476_CpG_5 (P<0.001). Further multivariate logistic regression was conducted, and the results showed that the six observation indicators with P<0.05: FYB_CpG_7 (P=0.002), SH3BP5_338_CpG_4 (P=0.01), RAPSN_348_CpG_5 (P=0.09), PNPLA2_329_CpG_1 (P=0.004), PNPLA2_329_CpG_4 (P<0.001), and ARHGAP35_476_CpG_5 (P<0.001). Binary logistic regression analysis was performed on these six indicators, and an invasive prediction model for pulmonary nodules was constructed. Malignant probability = ex/(1 + ex); x = 3.88 + 3.60 × (FYB_CpG_7) + 1.25 × (SH3BP5_338_CpG_4) − 1.54 × (RAPSN_348_CpG_5) − 7.17 × (PNPLA2_329_CpG_1) − 4.58 × (PNPLA2_329_CpG_4) + 3.06 × (ARHGAP35_476_CpG_5), where e is the natural logarithm. The effect of this model was evaluated. The AUC of the training set and the validation set were 0.78 and 0.74, respectively. With 0.596 as the cut-off value, the prediction accuracies were 76% and 71%, the sensitivities were 72% and 77%, and the specificities were 78% and 66%, respectively. See Tables 5,6 and Figures 2,3.
Table 6
Logistic regression analysis | Variables | β | S.E | Z | P | OR (95% CI) |
---|---|---|---|---|---|---|
Univariate | FYB CpG 4 | 3.33 | 1.42 | 2.35 | 0.02 | 27.97 (1.74–450.76) |
FYB CpG 7 | 2.17 | 0.90 | 2.41 | 0.02 | 8.74 (1.50–50.91) | |
SH3BP5 338 CpG 4 | 1.71 | 0.68 | 2.52 | 0.01 | 5.54 (1.47–20.90) | |
RAPSN 348 CpG 5 | −1.59 | 0.75 | −2.10 | 0.04 | 0.20 (0.05–0.90) | |
PNPLA2 329 CpG 1 | −4.86 | 2.11 | −2.30 | 0.02 | 0.01 (0.00–0.49) | |
PNPLA2 329 CpG 4 | −3.09 | 1.17 | −2.64 | 0.008 | 0.05 (0.00–0.45) | |
ARHGAP35 476 CpG 5 | 2.94 | 0.81 | 3.62 | <0.001 | 19.01 (3.85–93.78) | |
Multivariate (bidirectional stepwise regression) | Intercept | 3.88 | 2.27 | 1.71 | 0.09 | 48.47 (0.57–4,115.74) |
FYB CpG 7 | 3.60 | 1.14 | 3.16 | 0.002 | 36.46 (3.92–338.94) | |
SH3BP5 338 CpG 4 | 1.25 | 0.77 | 1.61 | 0.01 | 3.47 (0.76–15.87) | |
RAPSN 348 CpG 5 | −1.54 | 0.91 | −1.69 | 0.09 | 0.21 (0.04–1.28) | |
PNPLA2 329 CpG 1 | −7.17 | 2.48 | −2.89 | 0.004 | 0.00 (0.00–0.10) | |
PNPLA2 329 CpG 4 | −4.58 | 1.33 | −3.44 | <0.001 | 0.01 (0.00–0.14) | |
ARHGAP35 476 CpG 5 | 3.06 | 0.92 | 3.33 | <0.001 | 21.41 (3.52–130.20) |
CI, confidence interval; OR, odds ratio; S.E, standard error.
Comprehensive features model
Based on the above (variable screening of the training set) screened clinical features, CT image features and methylation features, univariate logistic regression was conducted. The results showed that there were 13 observation indicators with P<0.05: age (P=0.005), maximum diameter of pulmonary nodules (P=0.006), CTR (P<0.001), maximum CT value (P≤0.001), shape of pulmonary nodules (P=0.009), malignant features (P=0.04), FYB_CpG_4 (P=0.02), FYB_CpG_7 (P=0.02), SH3BP5_338_CpG_4 (P=0.01), RAPSN_348_CpG_5 (P=0.04), PNPLA2_329_CpG_1 (P=0.02), PNPLA2_329_CpG_4 (P=0.008), and ARHGAP35_476_CpG_5 (P<0.001). Further multivariate logistic regression analysis was performed, and the results showed that there were six observation indicators with P<0.05: CTR (P=0.002), maximum CT value (P<0.001), SH3BP5_338_CpG_4 (P=0.01), PNPLA2_329_CpG_1 (P=0.01), PNPLA2_329_CpG_4 (P=0.01), and ARHGAP35_476_CpG_5 (P<0.001). Binary Logistic regression analysis was performed on these six indicators, and a invasive prediction model for pulmonary nodules was constructed. Malignant probability = ex/(1 + ex); x = 2.32 − (0.03 × CTR) + (0.01 × maximum CT value) + 2.46 × (SH3BP5_338_CpG_4) − 6.83 × (PNPLA2_329_CpG_1) − 4.42 × (PNPLA2_329_CpG_4) + 4.17 × (ARHGAP35_476_CpG_5), where e is the natural logarithm. The effect of this model was evaluated. The AUC of the training set and the validation set were 0.90 and 0.87, respectively. With 0.619 as the cut-off value, the prediction accuracies were both 82%, the sensitivities were 82% and 80%, the specificities were 82% and 84%, the positive predictive values (PPVs) were 70% and 82%, and the negative predictive values (NPVs) were 89% and 82%, respectively. See Tables 5,7 and Figure 4.
Table 7
Logistic regression analysis | Variables | β | S.E | Z | P | OR (95% CI) |
---|---|---|---|---|---|---|
Univariate | Age | 0.03 | 0.01 | 2.81 | 0.005 | 1.04 (1.01–1.06) |
Maximum diameter of pulmonary nodules | 0.11 | 0.04 | 2.72 | 0.006 | 1.11 (1.03–1.20) | |
Consolidation tumor ratio | 0.04 | 0.01 | 3.98 | <0.001 | 1.04 (1.02–1.05) | |
Maximum CT value | 0.01 | 0.00 | 6.40 | <0.001 | 1.01 (1.01–1.01) | |
Malignant features | ||||||
• 0 (none) | 1.00 (reference) | |||||
• 1 (one or more) | 0.70 | 0.34 | 2.07 | 0.04 | 2.01 (1.04–3.90) | |
Shape of pulmonary nodules | ||||||
• 1 (circular-like and well-defined border) | 1.00 (reference) | |||||
• 2 (irregular and well-defined border) | 0.23 | 0.40 | 0.56 | 0.57 | 1.25 (0.57–2.76) | |
• 3 (circular-like and ill-defined border) | 1.26 | 0.48 | 2.63 | 0.009 | 3.54 (1.38–9.07) | |
• 4 (irregular and ill-defined border) | 0.60 | 0.51 | 1.18 | 0.24 | 1.82 (0.67–4.97) | |
FYB CpG 4 | 3.33 | 1.42 | 2.35 | 0.02 | 27.97 (1.74–450.76) | |
FYB CpG 7 | 2.17 | 0.90 | 2.41 | 0.02 | 8.74 (1.50–50.91) | |
SH3BP5 338 CpG 4 | 1.71 | 0.68 | 2.52 | 0.01 | 5.54 (1.47–20.90) | |
RAPSN 348 CpG 5 | −1.59 | 0.75 | −2.10 | 0.04 | 0.20 (0.05–0.90) | |
PNPLA2 329 CpG 1 | −4.86 | 2.11 | −2.30 | 0.02 | 0.01 (0.00–0.49) | |
PNPLA2 329 CpG 4 | −3.09 | 1.17 | −2.64 | 0.008 | 0.05 (0.00–0.45) | |
ARHGAP35 476 CpG 5 | 2.94 | 0.81 | 3.62 | <0.001 | 19.01 (3.85–93.78) | |
Multivariate (bidirectional stepwise regression) | Intercept | 2.32 | 2.75 | 0.85 | 0.40 | 10.22 (0.05–2,221.75) |
Consolidation tumor ratio | −0.03 | 0.01 | −3.06 | 0.002 | 0.97 (0.94–0.99) | |
Maximum CT value | 0.01 | 0.00 | 5.46 | <0.001 | 1.01 (1.01–1.01) | |
FYB CpG 4 | 4.58 | 2.47 | 1.86 | 0.06 | 97.45 (0.77–12,276.08) | |
SH3BP5 338 CpG 4 | 2.46 | 0.99 | 2.49 | 0.01 | 11.76 (1.68–82.13) | |
PNPLA2 329 CpG 1 | −6.83 | 2.66 | −2.56 | 0.01 | 0.00 (0.00–0.20) | |
PNPLA2 329 CpG 4 | −4.42 | 1.74 | −2.54 | 0.01 | 0.01 (0.00–0.36) | |
ARHGAP35 476 CpG 5 | 4.17 | 1.21 | 3.44 | <0.001 | 64.85 (6.01–700.12) |
CI, confidence interval; CT, computed tomography; OR, odds ratio; S.E, standard error.
The predictive effect of the comprehensive features model was better than that of the clinical and image features model and the methylation features model. The Hosmer-Lemeshow test and calibration curve showed that the goodness of fit of the model was relatively high. See Figure 2.
Discussion
The common pathological types of pulmonary nodules after surgical resection include AAH, AIS, MIA, IC, etc. Accurately determining the nature of pulmonary nodules before surgery enables clinicians to better formulate treatment plans. However, it is extremely difficult to precisely determine the specific pathological type. Currently, apart from pathological biopsy, there is no non-invasive method to identify the specific pathological type of pulmonary nodules. In fact, only identifying whether pulmonary nodules have entered the MIA or IC stage can meet the needs of actual clinical tasks. AIS can be observed continuously or undergo surgery at an elective time, while MIA and IC require prompt treatment such as surgery. AAH is usually a smaller pure ground-glass nodule, which is easily distinguishable on image. The main difficulty lies in the similarity of CT image features between AIS and MIA, making them difficult to distinguish. The purpose of this study is to explore methods for identifying whether pulmonary nodules have entered the MIC or IC stage.
Clinically, the nature of pulmonary nodules is mainly identified through CT image features, followed by positron emission tomography (PET)-CT, percutaneous lung biopsy, navigational bronchoscopy biopsy, liquid biopsy, etc. The metabolism of ground-glass nodules is not high, and PET-CT is not very helpful in improving the accuracy of differentiating AIS and MIA (19-21). Percutaneous lung biopsy and navigational bronchoscopy biopsy are invasive examinations and are not suitable for widespread implementation among patients with pulmonary nodules. Currently, there is no satisfactory statistical model that can distinguish AIS from MIA only through CT image features. In this study, 10 observation indicators that are relatively easy to obtain in clinical practice were selected from the clinical and image characteristics of patients with pulmonary nodules. Two factors (CTR and maximum CT value) were extracted through logistic regression analysis to construct a statistical model for differentiating the invasiveness of pulmonary nodules. The result showed an AUC of 0.80, a prediction accuracy of 78%, a sensitivity of 71%, and a specificity of 81%, with a mediocre effect. In this model, the CTR was negatively correlated with the malignant probability. It is considered that this was caused by some completely solid benign fibrous nodules in the included cases. If the number of cases is increased and the double truncation method (10) is used, the prediction accuracy may be further improved.
Liquid biopsy is one of the important methods for identifying malignant tumors, mainly including the detection of: circulating tumor cells (CTCs), circulating tumor DNA (ctDNA), Exosomes, circulating RNA, and peripheral blood DNA methylation. Previous studies have found that in the early stage of malignant tumor occurrence, there can be abnormal DNA methylation in the peripheral blood, such as hypermethylation of tumor suppressor genes or hypomethylation of oncogenes (22-25). Therefore, DNA methylation has received significant attention as a minimally invasive method for detecting early-stage cancers (26,27).
Qiao et al. (28-31) found that 23 methylation sites of 5 genes, namely FYB, PNPLA2_329, SH3BP5_338, RAPSN_348, and ARHGAP35_476 in the peripheral blood (that is, the 23 methylation sites detected in this study) were associated with early-stage lung cancer. However, the diagnostic ability of methylation sites of a single gene is limited. Some scholars have improved the efficacy of identifying early-stage lung cancer by jointly detecting methylation sites of several different genes (32-34). In this study, logistic regression analysis was conducted on the detection results of 23 methylation sites of 5 genes in the peripheral blood of 221 patients with pulmonary nodules in the training set. Six significant methylation sites were extracted to construct a statistical model for differentiating the invasiveness of pulmonary nodules. The result showed an AUC of 0.78, an accuracy of 76%, a sensitivity of 72%, and a specificity of 78%. It can be seen that the combination of methylation sites of multiple genes cannot satisfactorily distinguish whether pulmonary nodules are ICs.
Finally, we combined the clinical features, image features, and multiple gene methylation site features of patients with pulmonary nodules to construct a statistical model for identifying the invasiveness of pulmonary nodules. Eventually, a model with an AUC of 90%, an accuracy of 82%, a sensitivity of 82%, and a specificity of 82% was mentioned. The model was verified in the validation set, and the results of each evaluation index were similar to those of the training set. The predictive effect of this model is comparable to that of He’s model (11), but it incorporates fewer factors and is more suitable for rapid popularization in clinical practice.
Of course, there are some limitations in this study. The ideal model is to accurately predict the specific pathological types of nodules, such as benign fibrous nodules, AAH, AIS, MIA, IC, etc. However, the number of cases included in this study is small, and the collected cases of benign fibrous nodules and AAH are even fewer, which is insufficient to construct a precise pathological type prediction model. Therefore, we had to make do with the second-best option and construct an invasiveness prediction model to predict whether pulmonary nodules have entered the MIA or IC stage, but this has preliminarily met clinical needs. Additionally, the model constructed in this study is only the result of a single-center, small-sample, retrospective, and exploratory analysis. There may be biases in case selection and other aspects, and the model cannot be directly used in clinical work. Larger sample sizes and prospective studies are needed to verify and improve the model and enhance the prediction accuracy. Finally, the methylation sites detected in this study were selected based on some previous research results. More methylation sites and the models constructed by their combinations may have different prediction effects, which requires further exploration.
Conclusions
The invasive prediction model for pulmonary nodules constructed in this study by combining clinical, image, and methylation features has a relatively satisfactory effect and is worthy of further exploration and improvement.
Acknowledgments
The authors would like to express gratitude to Dr. Zhinuan Hong (Department of Thoracic Surgery, Fujian Medical University Union Hospital) for his valuable insights and contributions to the writing of this article.
Footnote
Reporting Checklist: The authors have completed the TRIPOD reporting checklist. Available at https://jtd.amegroups.com/article/view/10.21037/jtd-24-1763/rc
Data Sharing Statement: Available at https://jtd.amegroups.com/article/view/10.21037/jtd-24-1763/dss
Peer Review File: Available at https://jtd.amegroups.com/article/view/10.21037/jtd-24-1763/prf
Funding: This study was supported by
Conflicts of Interest: All authors have completed the ICMJE uniform disclosure form (available at https://jtd.amegroups.com/article/view/10.21037/jtd-24-1763/coif). The authors have no conflicts of interest to declare.
Ethical Statement: The authors are accountable for all aspects of the work in ensuring that questions related to the accuracy or integrity of any part of the work are appropriately investigated and resolved. The study was conducted in accordance with the Declaration of Helsinki (as revised in 2013). This study was approved by the Medical Ethics Committee of Xiamen Humanity Hospital of Fujian Medical University (No. HAXM-MEC-20221201-035-01), and informed consent was taken from all individual participants or their legal guardians.
Open Access Statement: This is an Open Access article distributed in accordance with the Creative Commons Attribution-NonCommercial-NoDerivs 4.0 International License (CC BY-NC-ND 4.0), which permits the non-commercial replication and distribution of the article with the strict proviso that no changes or edits are made and the original work is properly cited (including links to both the formal publication through the relevant DOI and the license). See: https://creativecommons.org/licenses/by-nc-nd/4.0/.
References
- Yotsukura M, Asamura H, Motoi N, et al. Long-Term Prognosis of Patients With Resected Adenocarcinoma In Situ and Minimally Invasive Adenocarcinoma of the Lung. J Thorac Oncol 2021;16:1312-20. [Crossref] [PubMed]
- Lin J, Yu Y, Zhang X, et al. Classification of Histological Types and Stages in Non-small Cell Lung Cancer Using Radiomic Features Based on CT Images. J Digit Imaging 2023;36:1029-37. [Crossref] [PubMed]
- González Maldonado S, Delorme S, Hüsing A, et al. Evaluation of prediction models for identifying malignancy in pulmonary nodules detected via low-dose computed tomography. JAMA Netw Open 2020;3:e1921221. [Crossref] [PubMed]
- Winter A, Aberle DR, Hsu W. External validation and recalibration of the Brock model to predict probability of cancer in pulmonary nodules using NLST data. Thorax 2019;74:551-63. [Crossref] [PubMed]
- Swensen SJ, Silverstein MD, Ilstrup DM, et al. The probability of malignancy in solitary pulmonary nodules. Application to small radiologically indeterminate nodules. Arch Intern Med 1997;157:849-55.
- Li Y, Chen K, Sui X, et al. Establishment of a mathematical prediction model for judging the benign and malignant nature of solitary pulmonary nodules. Journal of Peking University: Health Sciences 2011;43:5.
- Zang R, Wang X, Jin R, et al. Translational value of IDH1 and DNA methylation biomarkers in diagnosing lung cancers: a novel diagnostic panel of stage and histology-specificity. J Transl Med 2019;17:430. [Crossref] [PubMed]
- Batochir C, Kim IA, Jo EJ, et al. Discrimination of Lung Cancer and Benign Lung Diseases Using BALF Exosome DNA Methylation Profile. Cancers (Basel) 2024;16:2765. [Crossref] [PubMed]
- Jin Y, Mu W, Shi Y, et al. Development and validation of an integrated system for lung cancer screening and post-screening pulmonary nodules management: a proof-of-concept study (ASCEND-LUNG). EClinicalMedicine 2024;75:102769. [Crossref] [PubMed]
- Liang W, Tao J, Cheng C, et al. A clinically effective model based on cell-free DNA methylation and low-dose CT for risk stratification of pulmonary nodules. Cell Rep Med 2024;5:101750. [Crossref] [PubMed]
- He J, Wang B, Tao J, et al. Accurate classification of pulmonary nodules by a combined model of clinical, imaging, and cell-free DNA methylation biomarkers: a model development and external validation study. Lancet Digit Health 2023;5:e647-e656. [Crossref] [PubMed]
- Zhang J, Yao H, Lai C, et al. A novel multimodal prediction model based on DNA methylation biomarkers and low-dose computed tomography images for identifying early-stage lung cancer. Chin J Cancer Res 2023;35:511-25. [Crossref] [PubMed]
- Xing W, Sun H, Yan C, et al. A prediction model based on DNA methylation biomarkers and radiological characteristics for identifying malignant from benign pulmonary nodules. BMC Cancer 2021;21:263. [Crossref] [PubMed]
- Suzuki K, Koike T, Asakawa T, et al. A prospective radiological study of thin-section computed tomography to predict pathological noninvasiveness in peripheral clinical IA lung cancer (Japan Clinical Oncology Group 0201). J Thorac Oncol 2011;6:751-6. [Crossref] [PubMed]
- Wang M, Wei Y, Zhu M, et al. The Value of Topological Radiomics Analysis in Predicting Malignant Risk of Pulmonary Ground-Glass Nodules: A Multi-Center Study. Technol Cancer Res Treat 2024;23:15330338241287089. [Crossref] [PubMed]
- Xie J, He Y, Che S, et al. Differential diagnosis of benign and lung adenocarcinoma presenting as larger solid nodules and masses based on multiscale CT radiomics. PLoS One 2024;19:e0309033. [Crossref] [PubMed]
- Riley RD, Snell KIE, Ensor J, et al. Minimum sample size for developing a multivariable prediction model: Part I - Continuous outcomes. Stat Med 2019;38:1262-75. [Crossref] [PubMed]
- Liao J, Tao L, Xu L, et al. Sample size calculation for the establishment of prediction models for binary or survival outcomes. Chinese Journal of Pediatrics 2023;61:804.
- Chun EJ, Lee HJ, Kang WJ, et al. Differentiation between malignancy and inflammation in pulmonary ground-glass nodules: The feasibility of integrated (18)F-FDG PET/CT. Lung Cancer 2009;65:180-6. [Crossref] [PubMed]
- Tsushima Y, Tateishi U, Uno H, et al. Diagnostic performance of PET/CT in differentiation of malignant and benign non-solid solitary pulmonary nodules. Ann Nucl Med 2008;22:571-7. [Crossref] [PubMed]
- Chiu CF, Lin YY, Hsu WH, et al. Shorter-time dual-phase FDG PET/CT in characterizing solid or ground-glass nodules based on surgical results. Clin Imaging 2012;36:509-14. [Crossref] [PubMed]
- Feng LY, Chen CX, Li L. Hypermethylation of tumor suppressor genes is a risk factor for poor prognosis in ovarian cancer: A meta-analysis. Medicine (Baltimore) 2019;98:e14588. [Crossref] [PubMed]
- Debernardi C, Libera L, Berrino E, et al. Evaluation of global and intragenic hypomethylation in colorectal adenomas improves patient stratification and colorectal cancer risk prediction. Clin Epigenetics 2021;13:154. [Crossref] [PubMed]
- Liang R, Li X, Li W, et al. DNA methylation in lung cancer patients: Opening a “window of life” under precision medicine. Biomed Pharmacother 2021;144:112202. [Crossref] [PubMed]
- Pan Y, Liu G, Zhou F, et al. DNA methylation profiles in cancer diagnosis and therapeutics. Clin Exp Med 2018;18:1-14. [Crossref] [PubMed]
- Nonaka T, Wong DTW. Liquid Biopsy in Head and Neck Cancer: Promises and Challenges. J Dent Res 2018;97:701-8. [Crossref] [PubMed]
- Li P, Liu S, Du L, et al. Liquid biopsies based on DNA methylation as biomarkers for the detection and prognosis of lung cancer. Clin Epigenetics 2022;14:118. [Crossref] [PubMed]
- Li M, Qiao R, Zhong R, et al. FYB methylation in peripheral blood as a potential marker for the early-stage lung cancer: a case-control study in Chinese population. Biomarkers 2022;27:79-85. [Crossref] [PubMed]
- Qiao R, Li M, Zhong R, et al. The Association Between PNPLA2 Methylation in Peripheral Blood and Early-Stage Lung Cancer in a Case-Control Study. Cancer Manag Res 2021;13:7919-27. [Crossref] [PubMed]
- Qiao R, Zhong R, Liu C, et al. Novel blood-based hypomethylation of SH3BP5 is associated with very early-stage lung adenocarcinoma. Genes Genomics 2022;44:445-53. [Crossref] [PubMed]
- Qiao R, Di F, Wang J, et al. The Association Between RAPSN Methylation in Peripheral Blood and Early Stage Lung Cancer Detected in Case-Control Cohort. Cancer Manag Res 2020;12:11063-75. [Crossref] [PubMed]
- Ooki A, Maleki Z, Tsay JJ, et al. A Panel of Novel Detection and Prognostic Methylated DNA Markers in Primary Non-Small Cell Lung Cancer and Serum DNA. Clin Cancer Res 2017;23:7141-52. [Crossref] [PubMed]
- Powrózek T, Krawczyk P, Kuźnar-Kamińska B, et al. Analysis of RTEL1 and PCDHGB6 promoter methylation in circulating-free DNA of lung cancer patients using liquid biopsy: A pilot study. Exp Lung Res 2016;42:307-13. [Crossref] [PubMed]
- Vrba L, Oshiro MM, Kim SS, et al. DNA methylation biomarkers discovered in silico detect cancer in liquid biopsies from non-small cell lung cancer patients. Epigenetics 2020;15:419-30. [Crossref] [PubMed]