IRF8 and CD2 are potential targets of immunotherapy in non-small cell lung cancer
Highlight box
Key findings
• Discovering the new targets for combined immunotherapy (CD2 and IRF8).
What is known and what is new?
• IRF8 level of expression determines the development of classical dendritic cells (cDCs) into cDC1s.
• CD2 is involved in the formation of the corolla on the surface of CD8+ T cells to counteract the interaction between programmed death 1 (PD-1) and programmed death ligand 1 (PD-L1).
• The combination of CD2 and IRF8 can enhance the anti-tumor immune response.
What is the implication, and what should change now?
• IRF8 and CD2 may be new targets of combined immune checkpoint inhibitors (ICIs) for lung adenocarcinoma in non-small cell lung cancer (NSCLC).
Introduction
For patients with non-small cell lung cancer (NSCLC), the development of specific antibodies against the programmed death 1 (PD-1) receptor and programmed death ligand 1 (PD-L1) has led to unprecedented prolonged survival times in a subset of these patients (1,2). Although PD-1/PD-L1 blockade has shown significant clinical efficacy and prolonged patient survival, the response rate in nonselective NSCLC patients is approximately 20% (3). This good response tends to occur in patients with high PD-L1 expression (more than 50% of tumor cells) (4). Current researches have found multiple factors are involved in the tumor immune response, including tumor antigen exposure, antigen presentation, major histocompatibility complex (MHC) expression, and chemokine secretion, and endocytosis and degradation of immune checkpoint proteins (5-8). Although various oncology treatments aimed at these processes have been developed, their effectiveness remains limited (9). Therefore, it is important to identify the reasons for the low response rate to immunotherapy and to expand the pool of benefits of immunotherapy.
Recent studies have shown that the genomic and transcriptomic features of tumors can help predict the responses of tumor cells to immune checkpoint inhibitors (ICIs); for example, higher tumor mutational load, high PD-L1 expression, tumor antigen quality, and immune cell infiltration levels correlate with clinical benefit from immunotherapy (10-13). Some studies have found higher levels of immune cell infiltration around tumors, particularly infiltration by CD8+ T cells and natural killer (NK) cells, in patients who exhibit better prognosis and better response to treatment (14). Human type 1 classical dendritic cells (cDC1s) effectively induce cellular immunity against intracellular pathogens and tumors because of their efficient processing and cross-presentation of exogenous antigens on MHC class I molecules to activate CD8+ T cells, their recruitment of CD8+ T cells into the tumor microenvironment (15), their stimulation of CD8+ T cell proliferation and survival in the tumour microenvironment (TME) (16), and their ability to initiate type 1 T helper responses (17-22). It has been found that CD8+ T cells can form surface corollas and that the presence of corollas can largely counteract PD-1/PD-L1 interaction (23). Therefore, strategies designed to increase the abundance and enhance the function of cDC1 and CD8+ T cell infiltration of the TME are attractive new ways to enhance tumor immune responses and overcome resistance to immunotherapy.
In this study, the NSCLC patients with ICIs are the treatment cohort, and NSCLC patients who did not receive immunotherapy are the control cohort. We explored the changes in the tumor microenvironment of NSCLC before and after immunotherapy. At the same time, we screened and verified new prognostic indicators of NSCLC and new targets of combined immunotherapy. This may provide new insights into expanding the NSCLC immunotherapy population. We present this article in accordance with the TRIPOD reporting checklist (available at https://jtd.amegroups.com/article/view/10.21037/jtd-24-1589/rc).
Methods
Access to and processing of public data
This workflow is shown in Figure 1. The study was conducted in accordance with the Declaration of Helsinki (as revised in 2013). The Gene Expression Omnibus (GEO) database was searched for tumor transcriptome data from NSCLC patients who had been treated with ICIs, and a total of four datasets (GSE190265, GSE190266, GSE182328, and GSE135222) were obtained. GSE190265 and GSE190266 are PD-L1 blockade therapy, GSE182328 is PD-1 blockade therapy, and GSE135222 is PD-1 or PD-L1 therapy. Three tumor transcriptome datasets from NSCLC patients who did not receive ICI treatment (GSE181820, GSE81089, and GSE41271) were used as a control. After removing samples with incomplete information, 167 ICI treatment samples and 494 control samples were removed. All the above NSCLC cohort samples were fresh tumor tissue. The Cancer Genome Atlas (TCGA) database was searched to obtain transcriptomic data for 497 lung adenocarcinomas (LUADs) and 488 lung squamous carcinomas (LUSCs), as well as clinical and follow-up information on these samples. All transcriptome data were converted into transcripts per million reads (TPM) format, and log2(TPM +1) was transformed for subsequent data analysis.
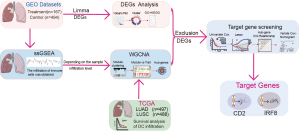
Identification and enrichment analysis of the differentially expressed genes (DEGs)
The limma package was used to conduct DEG analysis, and adjusted P values <0.05 and Log2 |fold change (FC)|>1 were used as thresholds to identify DEGs. The genes were mapped to the background gene set, and gene ontology (GO) annotation was performed on the genes. The minimum gene set was set as 5, the maximum gene set was 5,000, and 1,000 resamplings were performed.
TME immune score and single-sample gene set enrichment analysis (ssGSEA) immune cell annotation
The Estimate package was used to score the level of immune cell infiltration of the TME. ssGSEA was used to quantify the level of infiltration of the TME of each sample by 28 types of immune cells, with reference to the immune cell tagging gene set proposed by Charoentong et al. (14). The relative level of infiltration by each type of immune cell was expressed as a concentration fraction in the ssGSEA and normalizes to a uniform distribution from 0 to 1.
Screening for dendritic cell (DC) infiltration-related immune signature genes
Weighted gene co-expression network analysis (WGCNA) was used to identify highly correlated gene modules, and correlate modules with clinical traits in external samples using the signature gene network approach (24). The study incorporated the entire gene expression matrix of 661 samples from the GEO database for analysis. The 661 samples from the GEO database were divided into 28 immune cell subtype “high infiltration” and “low infiltration” groups based on the 28 immune cell subtype scores in ssGSEA, which were used as external clinical traits of the samples in WGCNA. The WGCNA package was used to construct the module in relation to clinical traits. The criteria for screening immune signature genes were set as |gene significance (GS)| >0.2 and |module member (MM)| >0.8 (25).
Protein-protein interaction (PPI) network analysis
STRING is an online database that retrieves interactions between sets of proteins and is used in PPI network analysis. The nodes in the PPI network diagram represent genes, and the connecting lines in the diagram represent the links between genes (26). PPI networks were set to a minimum interaction requirement score >0.4, and Cytoscape software was used to visualize the PPI networks (27).
Univariate Cox regression analysis and least absolute shrinkage and selection operator (LASSO) regression analysis of immune signature genes
In this study, gene expression data from 497 columns of LUAD patients in the TCGA database were included in the LASSO regression analysis. The LASSO regression was used for correlation analysis and comparison to explore the influencing factors of overall survival (OS). In addition, we set up a 10-fold cross-validation to obtain the optimal model. We set the value of λ to 0.02. The maxstat R package was used to calculate the optimal cut-off value for the RiskScore, setting the minimum grouping sample size greater than 25% and the maximum sample size grouping less than 75% and further using the survival package to analyze the difference in prognosis between the two groups.
Gene set variation analysis (GSVA) and differential expression analysis
To estimate gene set enrichment scores, we applied GSVA R package (version 1.32.0) to fulfill GSVA (28). Kruskal-Wallis test was performed on the enrichment scores between the two groups. T cells and DCs immune genetic feature set from the GSEA web site (http://www.gsea-msigdb.org/gsea/msigdb/human/genese). In addition, we also collected gene sets for Antigen presentation (29), Macrophage and DC traffic (30), and T cell cytotoxicity (31). The limma R package (version 3.42.2) was used to calculate the differential activities of gene sets between groups. A Benjamini-Hochberg-corrected P value of ≤0.05 was used to identify significantly altered gene sets.
Construction of predictive models
A nomogram can be used to calculate the predictive value of an outcome event for an individual by constructing a multivariate Cox regression model, assigning a score to each value level of each influencing factor according to the degree of contribution of each influencing factor to the outcome variable in the model and then summing the individual scores to obtain the total score (32). Using the gene expression data, clinical information and follow-up information on 497 LUAD patients in the TCGA database as the basis for analysis, a nomogram was created to assess the prognostic significance of the target genes in the 497 samples using the R package rms.
Statistical analysis
The statistical analysis in this study was performed with R 4.2.0. For quantitative data, t-tests were estimated for outcomes that were statistically significant for normally distributed variables, and Wilcoxon rank sum tests were estimated for nonnormally distributed variables. When more than two groups were analyzed, the Kruskal-Wallis test was applied to nonparametric tests, and analysis of variance (ANOVA) was applied to parametric tests (33). Event rates were calculated using Fisher’s exact test. Prognostic differences between the two groups were analyzed using the survival package, and the log-rank test method was used to assess the significance of the prognostic differences between the different groups in the sample. Receiver operating characteristic (ROC) curves were plotted using the pROC package, and the area under the curve (AUC) and confidence intervals (CIs) were calculated. Correlations between genes were analyzed by Pearson correlation analysis. All comparisons were two-sided, and P<0.05 was considered statistically significant. The Benjamini-Hochberg method was used to perform multiple hypothesis testing of the false discovery rate (FDR) (34).
Results
There was heterogeneity between the immunotherapy cohort and the non-immunotherapy cohort
A total of 167 samples from four NSCLC datasets of patients who were treated with ICIs (GSE190266, GSE190265, GSE182328, and GSE135222) were obtained from the GEO database, and 494 samples from three NSCLC datasets from patients who were not treated with ICIs (GSE41271, GSE181820, and GSE81089) were used as controls. A total of 413 DEGs that are differentially expressed in the treatment and control groups are identified by differential expression analysis. CT47A1, CDY2A, CT47A11 and other genes (153 genes in total) are upregulated, and KIR3DL1, ARHGDIG, CCDC73, and other genes (260 genes in total) were downregulated (Figure 2A and Table S1). Cluster analysis of the top 100 DEGs showed good discrimination (Figure 2B). Further clustering analysis by treatment showed that DEGs were clearly differentiated between the two groups (Figure S1).
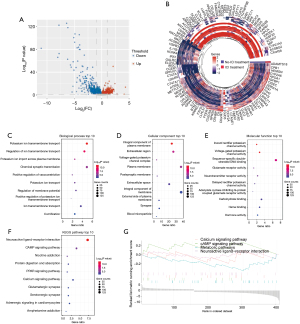
The observed DEGs may be unrelated to the classical immune response
GO and Kyoto Encyclopedia of Genes and Genomes (KEGG) enrichment showed that the DEGs were mainly associated with (I) biological processes such as transmembrane transport of potassium ions, regulation of ion transport across membranes, and input of potassium ions across the plasma membrane; (II) cellular components, including integral components of the plasma membrane, extracellular region, and voltage-gated potassium channel complex; (III) molecular functions such as inward rectifier potassium channel activity, voltage-gated potassium channel activity, and sequence-specific double-stranded DNA binding (Figure 2C-2F). GSEA enriched analysis showed that the DEGs were mainly associated with the Calcium signaling pathway, neuroactive ligand-receptor interaction, and cyclic adenosine monophosphate (cAMP) signaling pathway (Figure 2G). In summary, the identified DEGs were not enriched in processes related to the classical immune response.
The tumor immune response is not well activated in ICI-treated NSCLC patients
The estimate immune infiltration analysis showed no significant difference in immune infiltration scores between the two groups (Figure S2). The ssGSEA was then used to score 28 types of immune cell infiltrates in the two groups (Figure 3A,3B). Only memory B cell, neutrophil, and type 17 T helper cell infiltrates were increased in the ICI-treated cohort. The infiltrate level for most types of immune cells, including activated CD8+ T cells, activated CD4+ T cells, and NK T cells, all of which play crucial cellular roles in the antitumor process, did not differ significantly between the two groups. In contrast, activated DCs, immature DCs, plasmacytoid DCs, central memory CD4+ T cells, central memory CD8+ T cells, and type 1 T helper cells showed less infiltration in the treatment group than in the control group (Figure 3B).
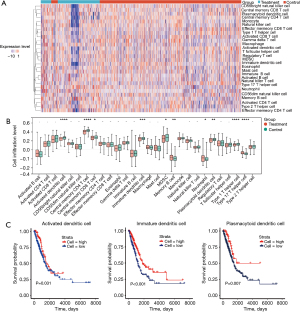
Transcriptomic datasets and clinical follow-up information were obtained from the TCGA database for 985 NSCLC patients, including 497 patients with LUAD and 488 patients with LUSC. The ssGSEA algorithm was used to score these samples for 28 types of immune cell infiltration. The patients were divided into a high immune cell infiltration group and a low immune cell infiltration group according to their scores; their survival was then analyzed, and Kaplan-Meier (KM) curves were plotted (Figure S3A,S3B). For the patients in the LUAD group, there is a strong correlation between the level of infiltration by most types of immune cells and survival. Patients with higher levels of infiltrating immune cells in the TME had better prognosis and survival, except a few types of immune cells that are not statistically significant (Figure S3A). The level of immune cell infiltration of the TME had a smaller effect on survival among the patients in the LUSC group than among those in the LUAD group (Figure S3B).
Immune signature genes are involved in the immune response
ssGSEA reveals that infiltration of the TME by three types of DCs (activated DCs, immature DCs, and plasmacytoid DCs) were reduced in patients treated with ICIs compared to the control group (Figure 3B). The results also confirmed that patients in the TCGA cohort with high DC infiltration had better prognosis (Figure 3C). Research has shown that DCs are central to the initiation of antigen-specific immunity and immune tolerance and that they are also essential for modulating tumor immune responses (35,36). Therefore, DCs represent the key to censoring immune signature genes.
The full gene expression matrix of 661 samples from the GEO database was included in the WGCNA for analysis. The value of Signed R2 was used as the basis for selecting the soft threshold, as shown in Figure 4A. When R2 is greater than 0.8, the soft threshold is 5, and the degree of association is the greatest at this time; based on this, we set 5 as the soft threshold for subsequent analysis. Figure 4B shows R2 =0.86 and slope =−2, consistent with a scale-free network distribution. A total of 11 modules were identified, as shown in Figure 4C. A heatmap of the correlations between the modules and infiltration by 28 types of immune cells is shown in Figure 4D. Three types of DCs (activated DCs, immature DCs, and plasmacytoid DCs) were clearly found to be positively correlated with the blue module and negatively correlated with the yellow module. The blue module and the yellow module were used to construct scatter plots showing activated DCs, mature DCs and plasmacytoid DCs. The correlations between the blue module and activated DCs, mature DCs, and plasmacytoid DCs were 0.73, 0.55, and 0.77, respectively (P<0.001) (Figure 4E-4G). The correlations between the yellow module and activated DCs, mature DCs, and plasmacytoid DCs were 0.31, 0.63, and 0.52, respectively (P<0.001) (Figure 4H-4J). The immune signature genes were screened using the criteria |GS|>0.2 and |MM|>0.8. Using these criteria, a total of 23 immune signature genes in the blue module, including APBB1IP, ARHGAP15, CD2, IRF8, GIMAP5, and other genes are identified, and 18 immune signature genes in the yellow module, including FOXM1, DLGAP5, MCM10, ASPM, AURKB, and other genes were obtained (Tables S2,S3). Protein interaction network analysis shows that the immune signature genes in the blue and yellow modules were highly correlated (Figure 4K). GO enrichment analyses of the immune signature genes in the blue and yellow modules reveal significant enrichment in cell division, spindle organisation, antigen contact activation of T cells via T-cell receptor binding to MHC molecules on antigen presenting cells, DNA replication initiation, and T-cell activation (Figure 5A-5C). KEGG pathway analysis showed significant enrichment in NK cell-mediated cytotoxicity, cell adhesion molecules, phagosomes, tuberculosis and viral myocarditis (Figure 5D).
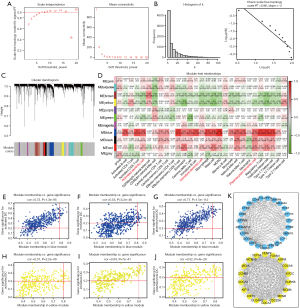
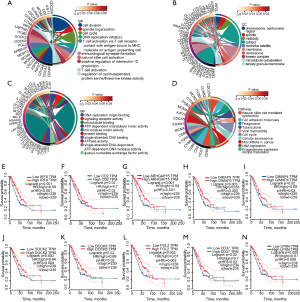
The immune signature genes in the blue and yellow modules were intersected with DEGs. The results show that no intersections were found and the immune signature genes were not differentially expressed (Figure S4). This shows that immune-related signature genes are not well activated or suppressed in the TME of NSCLC patients treated with ICIs; it also suggests that activating or suppressing these immune signature genes may be helpful for immunotherapy.
Immune signature genes associated with survival in LUAD patients
GEPIA was used to perform univariate Cox analysis of the immune signature genes in the blue module, and KM curves were plotted. The results show that a total of 10 genes in the blue module (ARHGAP15, CD2, CD37, DOCK2, DOCK8, FGL2, GIMAP4, GIMAP5, IRF8, and LPXN) are strongly associated with OS in LUAD patients (Figure 5E-5N). Patients with LUAD who exhibit high expression of these genes have a higher 5-year survival rate than patients who exhibit low expression of these genes, indicating that high expression of these genes is a protective factor for LUAD patients. Expression of these genes did not affect the 5-year survival rate of LUSC patients; for those patients, no significant difference in survival is found. The same method was used to perform univariate Cox analysis of the genes in the yellow module and to plot KM curves. The results show that all 18 genes in the yellow module are associated with OS in LUAD patients. However, the results obtained for these genes contrast with those obtained for the genes in the blue module, for which high expression is a risk factor for LUAD patients (Figure S5). Like the genes in the blue module, the immune signature genes in the yellow module did not affect the 5-year survival rate of LUSC patients. This result confirms that high expression of genes that are positively associated with immune cell infiltration in WGCNA is associated with better prognosis in LUAD patients, while high expression of genes negatively associated with immune cell infiltration is associated with worse prognosis in LUAD patients. The results of univariate Cox regression analysis suggested that immune signature genes are not significantly associated with survival outcome or OS in patients with LUSC.
Six hub genes retained in LASSO regression
Twenty-eight candidate immune signature genes (10 genes in the blue module and 18 genes in the yellow module) associated with prognosis in LUAD patients were identified through univariate Cox regression analysis. The expression data for 28 survival-related immune signature genes in 497 LUAD patients in the TCGA database and clinical follow-up information were used in LASSO regression analysis. Six hub genes (RHGAP15, CD2, GIMAP5, IRF8, DLGAP5, and FOXM1) were finally obtained through this analysis (Figure 6A). Further analysis using the survival R package showed a significant difference in prognosis between the high-risk score and low-risk score groups [log-rank test, P<0.001, hazard ratio (HR) =2.45, 95% confidence interval (CI): 1.69–3.55], with the high-risk score group having a worse prognosis, as shown in Figure 6B. The relationships between risk score and patient follow-up time, events and changes in the expression of the six hub genes were then analyzed, and a heatmap of the associated survival was produced (Figure 6C). From the graph, it can be observed that the survival rate of patients decreased significantly as the risk score increased. As expected, ARHGAP15, CD2, GIMAP5, and IRF8 were protective factors and showed a trend of downregulation in expression with increasing risk score, while DLGAP5 and FOXM1 genes were risk factors and showed an upregulation trend in expression with increasing risk score. The ROC curves showed that the AUCs and confidence intervals for the 365-, 1,095- and 1,825-day time points were 0.73 (0.66–0.80), 0.77 (0.70–0.83) and 0.78 (0.70–0.86), respectively (Figure 6D).
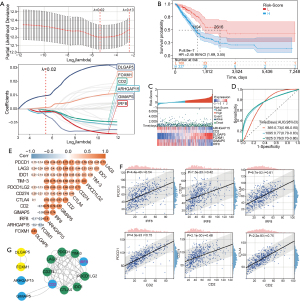
IRF8 and CD2 have a stronger correlation than other genes with ICGs
The expression data for six hub genes (RHGAP15, CD2, GIMAP5, IRF8, DLGAP5, and FOXM1) and immune checkpoint genes (IDO1, CD274, TIM-3, PDCD1, CTLA-4, LAG3 and PDCD1LG2) in 497 LUAD patients in the TCGA database were used in correlation analysis. The results showed that CD2 was strongly correlated with PDCD1 and CTLA-4 (r>0.7) and moderately correlated with other ICGs and that IRF8 was strongly correlated with TIM-3 and moderately correlated with other ICGs (Figure 6E). And scatter plots were plotted for the correlation of the 6 hub-genes with PDCD1, CTLA-4 and CD274. The correlations of IRF8 with PDCD1, CD274 and CTLA-4 were 0.54, 0.42 and 0.61, respectively. The correlations of CD2 with PDCD1, CD274 and CTLA-4 were 0.75, 0.48 and 0.75, respectively (Figure 6F). ARHGAP15 and GIMAP5 were moderately correlated with ICGs, and DLGAP5 and FOXM1 were weakly correlated with ICGs (r<0.3) (Figure S6). Protein interaction network analysis of ARHGAP15, CD2, GIMAP5, IRF8, IDO1, CD274, TIM-3, PDCD1, CTLA-4, LAG3, and PDCD1LG also showed that CD2 and IRF8 are more closely related to ICGs than were the other genes, as shown in Figure 6G.
High expression of IRF8 and CD2 effectively enhance the anti-tumor response
Using the median, we divided the LUAD samples in TCGA into both IRF8 and CD2 high expression groups and both low expression groups. ssGSEA was used to evaluate the infiltration level of immune cells (activated CD8 T cell, activated CD8 T cell, effector memory CD8 T cell, activated DC, and plasmacytoid DC) in the TME. Kruskal-Wallis test was used to compare the differences between the two groups. The results showed that the levels of infiltration of these cells in IRF8 and CD2 high expression groups were significantly higher than those in IRF8 and CD2 low expression groups (P<0.001) (Figure 7A,7B). We next applied GSVA to analyze the enrichment scores of Cytotoxicity, Antigen presentation, and Macrophage and DC traffic between the two groups. The results showed that the levels of Cytotoxicity, Antigen presentation, and Macrophage and DC traffic in the IRF8 and CD2 high expression groups were significantly higher than those in the IRF8 and CD2 low expression groups (Kruskal-Wallis test, P<0.001) (Figure 7A,7C). Thus, the ability of DCs to migrate to the tumor microenvironment and to display their antigen presentation ability were significantly increased in IRF8 and CD2 high expression groups (30). The results also showed that the cytotoxicity of CD8+ T cells against tumor cells was significantly increased. Finally, we applied GSVA to analyze the T cell and DC related immune functions. The results showed that the tumor immune response, cytokine production, cytotoxicity, and proliferation ability of T cells in the high expression group of IRF8 and CD2 were significantly increased (Kruskal-Wallis test, P<0.001), as shown in Figure 7A,7D. In addition, the ability of DC activation, cytokine production, and antigen processing, and presenting were significantly increased (Kruskal-Wallis test, P<0.001), as shown in Figure 7A,7E. To summarize, the high expression of IRF8 and CD2 increased the infiltration level of CD8+ T cells and DC in the tumor microenvironment, and effectively enhanced the anti-tumor immune response of patients.
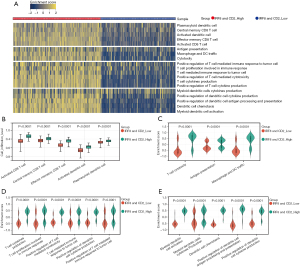
Discussion
ICIs has achieved significant clinical efficacy, but the response rate in nonselective NSCLC patients is only approximately with 20% (3). There is an urgent need to expand the benefits of PD-1/PD-L1 immunotherapy. Promisingly, in addition to the PD-1/PD-L1 axis, immune coinhibitory/costimulatory molecules and other components of the TME such as cytokines and levels of immune cell infiltration are thought to be important factors that contribute to regulation of the antitumor immune response (37-40). Therefore, we creatively integrate the entire transcriptomes of NSCLC tumors from patients who had been treated with ICIs from the GEO database, and try to find the reasons for the low response rate of NSCLC immunotherapy and to search for new targets of combined immunotherapy.
The role of immunophenotype in determining the prognosis of various types of cancer is increasingly recognised (41). Recent research also highlights the fact that baseline levels of tumor-infiltrating lymphocytes are significantly associated with the likelihood of immune response and with survival outcome (42). In our study, DEGs from NSCLC immunotherapy cohorts and non-immunotherapy cohorts were not enriched in the classical immune response as shown by functional enrichment analysis. Using ssGSEA, tumor immune cell infiltration in the TME was at a low level in the immunotherapy cohort. Survival analysis also showed that higher levels of DC infiltration resulted in better survival prognosis for patients. As mediators of adaptive immune responses, DCs are important targets for cancer immunotherapy (36). Recent studies have highlighted the specific role of DCs subpopulations in antitumor immunity; this role is of therapeutic importance, as DCs can effectively process and deliver tumor antigens to activate T cells, thereby enhancing the tumor immune response (43,44). In summary, low response rate of ICI-treated NSCLC patients may be closely related to the low levels antitumor immune cell infiltration and unactivated classical antitumor immune response in the TME. They also suggest that the activation of genes associated with DC infiltration may be crucial in enhancing tumor immunity.
IRF8 and CD2, which are hub genes for antitumor immunity, were resolved by univariate Cox and LASSO regression and correlation analysis. At the same time, the nomogram showed a significant improvement in survival for patients with high IRF8 and CD2 expression. IRF8, like other members of the interferon regulatory factor family of proteins, contains a DNA-binding domain, a linker domain and an IRF-associated structural domain (45). Human classical DCs (cDCs) can be divided into two distinct subpopulations, cDC1 and cDC2 (17). The transcription factor IRF8 plays an important role in the development of cDCs, and its level of expression determines the development of cDCs into cDC1s (46). Human cDC1s excel in antitumor cellular immunity. cDC1s initiate CD8+ T cells upon migration to tumor-draining lymph nodes, recruit CD8+ T cells, secrete cytokines and present tumor antigens in the TME, thereby enhancing local T-cell cytotoxicity (43). In our study, we found that patients with high expression of both IRF8 and CD2 had significantly increased levels of activated DCs and CD8+ T cell infiltration in the tumor microenvironment. moreover, the antigen presentation ability, chemotactic function and cytokine secretion of DCs were also significantly enhanced.
CD8+ T cells can form surface corollas on their surfaces. The presence of a surface corolla largely counteracts the PD-1-PD-L1 interaction, and corolla formation is largely dependent on the level of expression of CD2 molecules (23). CD2 expression levels in colorectal cancer patients treated with PD-1 monoclonal antibodies have a significant impact on treatment outcome, as reduced CD2 expression may counteract the benefits of PD-1-targeted therapy. In the MCA-38 mouse colon adenocarcinoma model, CD2 expression was found to be reduced, and tumor-infiltrating lymphocytes showed defective cytotoxicity (47). CD2 molecules were also found to be expressed at lower levels in CD8+ T cells in patients with various types of cancer (48). Finally, a weak but very significant negative correlation between the depletion gene signature and CD2 expression was found in publicly available single-cell RNA sequencing data (23). Our results showed that patients with high expression of both IRF8 and CD2 had significantly higher scores of T cell cytotoxicity and immune response to tumors than those with low expression. Therefore, targeted activation of IRF8 and CD2 brings hope to improve anti-tumor response.
There are some limitations in this study. This study did not validate the results using our RNA sequencing results from NSCLC samples and clinical information. There is also no information on the treatment response of NSCLC patients after immunotherapy in the TCGA database and the GEO database, and this may lead to the development of predictive models that do not fully explain the effect of target gene combination immunotherapy.
Conclusions
The findings of this study reveal that the low response rate to immunotherapy in NSCLC may be closely related to the inactivation of classical immune responses and antitumor immune cell infiltration in the TME. IRF8 and CD2 are promising targets for combined immunotherapy and are potential indicators of prognosis for LUAD in NSCLC.
Acknowledgments
We would like to express our gratitude to Dr. Feng-ying Du (Shandong Provincial Hospital Affiliated to Shandong First Medical University, Shandong First Medical University, Jinan, China) for method guidance and professional statistical advice.
Footnote
Reporting Checklist: The authors have completed the TRIPOD reporting checklist. Available at https://jtd.amegroups.com/article/view/10.21037/jtd-24-1589/rc
Peer Review File: Available at https://jtd.amegroups.com/article/view/10.21037/jtd-24-1589/prf
Funding: This study was supported by
Conflicts of Interest: All authors have completed the ICMJE uniform disclosure form (available at https://jtd.amegroups.com/article/view/10.21037/jtd-24-1589/coif). The authors have no conflicts of interest to declare.
Ethical Statement: The authors are accountable for all aspects of the work in ensuring that questions related to the accuracy or integrity of any part of the work are appropriately investigated and resolved. The study was conducted in accordance with the Declaration of Helsinki (as revised in 2013).
Open Access Statement: This is an Open Access article distributed in accordance with the Creative Commons Attribution-NonCommercial-NoDerivs 4.0 International License (CC BY-NC-ND 4.0), which permits the non-commercial replication and distribution of the article with the strict proviso that no changes or edits are made and the original work is properly cited (including links to both the formal publication through the relevant DOI and the license). See: https://creativecommons.org/licenses/by-nc-nd/4.0/.
References
- Howlader N, Forjaz G, Mooradian MJ, et al. The Effect of Advances in Lung-Cancer Treatment on Population Mortality. N Engl J Med 2020;383:640-9. [Crossref] [PubMed]
- Reck M, Remon J, Hellmann MD. First-Line Immunotherapy for Non-Small-Cell Lung Cancer. J Clin Oncol 2022;40:586-97. Erratum in: J Clin Oncol 2022;40:1265. [Crossref] [PubMed]
- Shukuya T, Carbone DP. Predictive Markers for the Efficacy of Anti-PD-1/PD-L1 Antibodies in Lung Cancer. J Thorac Oncol 2016;11:976-88. [Crossref] [PubMed]
- Limagne E, Nuttin L, Thibaudin M, et al. MEK inhibition overcomes chemoimmunotherapy resistance by inducing CXCL10 in cancer cells. Cancer Cell 2022;40:136-152.e12. [Crossref] [PubMed]
- Wu J, Li L, Wu S, Xu B. CMTM family proteins 1-8: roles in cancer biological processes and potential clinical value. Cancer Biol Med 2020;17:528-42. [Crossref] [PubMed]
- Chen DS, Mellman I. Oncology meets immunology: the cancer-immunity cycle. Immunity 2013;39:1-10. [Crossref] [PubMed]
- Chen DS, Mellman I. Elements of cancer immunity and the cancer-immune set point. Nature 2017;541:321-30. [Crossref] [PubMed]
- Boussiotis VA. Molecular and Biochemical Aspects of the PD-1 Checkpoint Pathway. N Engl J Med 2016;375:1767-78. [Crossref] [PubMed]
- Zhu L, Ye D, Lei T, et al. Cancer mutation profiles predict ICIs efficacy in patients with non-small cell lung cancer. Expert Rev Mol Med 2022;24:e16. [Crossref] [PubMed]
- Rizvi H, Sanchez-Vega F, La K, et al. Molecular Determinants of Response to Anti-Programmed Cell Death (PD)-1 and Anti-Programmed Death-Ligand 1 (PD-L1) Blockade in Patients With Non-Small-Cell Lung Cancer Profiled With Targeted Next-Generation Sequencing. J Clin Oncol 2018;36:633-641. Erratum in: J Clin Oncol 2018;36:1645. [Crossref] [PubMed]
- Hellmann MD, Nathanson T, Rizvi H, et al. Genomic Features of Response to Combination Immunotherapy in Patients with Advanced Non-Small-Cell Lung Cancer. Cancer Cell 2018;33:843-852.e4. [Crossref] [PubMed]
- Chen H, Yang M, Wang Q, et al. The new identified biomarkers determine sensitivity to immune check-point blockade therapies in melanoma. Oncoimmunology 2019;8:1608132. [Crossref] [PubMed]
- Cristescu R, Mogg R, Ayers M, et al. Pan-tumor genomic biomarkers for PD-1 checkpoint blockade-based immunotherapy. Science 2018;362:eaar3593. [Crossref] [PubMed]
- Charoentong P, Finotello F, Angelova M, et al. Pan-cancer Immunogenomic Analyses Reveal Genotype-Immunophenotype Relationships and Predictors of Response to Checkpoint Blockade. Cell Rep 2017;18:248-62. [Crossref] [PubMed]
- Spranger S, Dai D, Horton B, et al. Tumor-Residing Batf3 Dendritic Cells Are Required for Effector T Cell Trafficking and Adoptive T Cell Therapy. Cancer Cell 2017;31:711-723.e4. [Crossref] [PubMed]
- Dannull J, Nair S, Su Z, et al. Enhancing the immunostimulatory function of dendritic cells by transfection with mRNA encoding OX40 ligand. Blood 2005;105:3206-13. [Crossref] [PubMed]
- Merad M, Sathe P, Helft J, et al. The dendritic cell lineage: ontogeny and function of dendritic cells and their subsets in the steady state and the inflamed setting. Annu Rev Immunol 2013;31:563-604. [Crossref] [PubMed]
- Schlitzer A, McGovern N, Ginhoux F. Dendritic cells and monocyte-derived cells: Two complementary and integrated functional systems. Semin Cell Dev Biol 2015;41:9-22. [Crossref] [PubMed]
- Mildner A, Jung S. Development and function of dendritic cell subsets. Immunity 2014;40:642-56. [Crossref] [PubMed]
- Chiang MC, Tullett KM, Lee YS, et al. Differential uptake and cross-presentation of soluble and necrotic cell antigen by human DC subsets. Eur J Immunol 2016;46:329-39. [Crossref] [PubMed]
- Sittig SP, Bakdash G, Weiden J, et al. A Comparative Study of the T Cell Stimulatory and Polarizing Capacity of Human Primary Blood Dendritic Cell Subsets. Mediators Inflamm 2016;2016:3605643. [Crossref] [PubMed]
- Segura E, Durand M, Amigorena S. Similar antigen cross-presentation capacity and phagocytic functions in all freshly isolated human lymphoid organ-resident dendritic cells. J Exp Med 2013;210:1035-47. [Crossref] [PubMed]
- Demetriou P, Abu-Shah E, Valvo S, et al. A dynamic CD2-rich compartment at the outer edge of the immunological synapse boosts and integrates signals. Nat Immunol 2020;21:1232-43. [Crossref] [PubMed]
- Jia Q, Wu W, Wang Y, et al. Local mutational diversity drives intratumoral immune heterogeneity in non-small cell lung cancer. Nat Commun 2018;9:5361. [Crossref] [PubMed]
- Langfelder P, Horvath S. WGCNA: an R package for weighted correlation network analysis. BMC Bioinformatics 2008;9:559. [Crossref] [PubMed]
- Tang J, Kong D, Cui Q, et al. Prognostic Genes of Breast Cancer Identified by Gene Co-expression Network Analysis. Front Oncol 2018;8:374. [Crossref] [PubMed]
- Szklarczyk D, Gable AL, Nastou KC, et al. The STRING database in 2021: customizable protein-protein networks, and functional characterization of user-uploaded gene/measurement sets. Nucleic Acids Res 2021;49:D605-12. [Crossref] [PubMed]
- Shannon P, Markiel A, Ozier O, et al. Cytoscape: a software environment for integrated models of biomolecular interaction networks. Genome Res 2003;13:2498-504. [Crossref] [PubMed]
- Hänzelmann S, Castelo R, Guinney J. GSVA: gene set variation analysis for microarray and RNA-seq data. BMC Bioinformatics 2013;14:7. [Crossref] [PubMed]
- Liu Y, He S, Wang XL, et al. Tumour heterogeneity and intercellular networks of nasopharyngeal carcinoma at single cell resolution. Nat Commun 2021;12:741. [Crossref] [PubMed]
- Bagaev A, Kotlov N, Nomie K, et al. Conserved pan-cancer microenvironment subtypes predict response to immunotherapy. Cancer Cell 2021;39:845-865.e7. [Crossref] [PubMed]
- Li D, Yu H, Hu J, et al. Comparative profiling of single-cell transcriptome reveals heterogeneity of tumor microenvironment between solid and acinar lung adenocarcinoma. J Transl Med 2022;20:423. [Crossref] [PubMed]
- Balachandran VP, Gonen M, Smith JJ, et al. Nomograms in oncology: more than meets the eye. Lancet Oncol 2015;16:e173-80. [Crossref] [PubMed]
- Hazra A, Gogtay N. Biostatistics Series Module 3: Comparing Groups: Numerical Variables. Indian J Dermatol 2016;61:251-60. [Crossref] [PubMed]
- Love MI, Huber W, Anders S. Moderated estimation of fold change and dispersion for RNA-seq data with DESeq2. Genome Biol 2014;15:550. [Crossref] [PubMed]
- Steinman RM. Decisions about dendritic cells: past, present, and future. Annu Rev Immunol 2012;30:1-22. [Crossref] [PubMed]
- Wculek SK, Cueto FJ, Mujal AM, et al. Dendritic cells in cancer immunology and immunotherapy. Nat Rev Immunol 2020;20:7-24. [Crossref] [PubMed]
- Anderson AC, Joller N, Kuchroo VK. Lag-3, Tim-3, and TIGIT: Co-inhibitory Receptors with Specialized Functions in Immune Regulation. Immunity 2016;44:989-1004. [Crossref] [PubMed]
- Berraondo P, Sanmamed MF, Ochoa MC, et al. Cytokines in clinical cancer immunotherapy. Br J Cancer 2019;120:6-15. [Crossref] [PubMed]
- Jeong S, Park SH. Co-Stimulatory Receptors in Cancers and Their Implications for Cancer Immunotherapy. Immune Netw 2020;20:e3. [Crossref] [PubMed]
- Li X, Wenes M, Romero P, et al. Navigating metabolic pathways to enhance antitumour immunity and immunotherapy. Nat Rev Clin Oncol 2019;16:425-41. [Crossref] [PubMed]
- Mahajan UM, Langhoff E, Goni E, et al. Immune Cell and Stromal Signature Associated With Progression-Free Survival of Patients With Resected Pancreatic Ductal Adenocarcinoma. Gastroenterology 2018;155:1625-1639.e2. [Crossref] [PubMed]
- Galon J, Bruni D. Approaches to treat immune hot, altered and cold tumours with combination immunotherapies. Nat Rev Drug Discov 2019;18:197-218. [Crossref] [PubMed]
- Böttcher JP, Reis e Sousa C. The Role of Type 1 Conventional Dendritic Cells in Cancer Immunity. Trends Cancer 2018;4:784-92. [Crossref] [PubMed]
- Demoulin S, Herfs M, Delvenne P, et al. Tumor microenvironment converts plasmacytoid dendritic cells into immunosuppressive/tolerogenic cells: insight into the molecular mechanisms. J Leukoc Biol 2013;93:343-52. [Crossref] [PubMed]
- Taniguchi T, Ogasawara K, Takaoka A, et al. IRF family of transcription factors as regulators of host defense. Annu Rev Immunol 2001;19:623-55. [Crossref] [PubMed]
- Kim S, Bagadia P, Anderson DA 3rd, et al. High Amount of Transcription Factor IRF8 Engages AP1-IRF Composite Elements in Enhancers to Direct Type 1 Conventional Dendritic Cell Identity. Immunity 2020;53:759-774.e9. [Crossref] [PubMed]
- Koneru M, Monu N, Schaer D, et al. Defective adhesion in tumor infiltrating CD8+ T cells. J Immunol 2006;176:6103-11. [Crossref] [PubMed]