Quantitative CT evaluation of extrapulmonary lesions in chronic obstructive pulmonary disease: a narrative review
Introduction
Chronic obstructive pulmonary disease (COPD) is a complex and heterogeneous disease (1). Currently, the World Health Organization considers COPD as the third leading cause of death globally (2), predicting that over 2.9 million people die annually due to COPD and its complications (3). Characterized by limited airflow, COPD poses a significant challenge to global public health (4).
In recent years, advancements in medical imaging technology, particularly in the application of computed tomography (CT), have significantly enhanced the research and understanding of COPD (5). COPD often coexists with other diseases, some of which can influence the progression of COPD (1). CT not only provided detailed views of lung structures but also revealed extrapulmonary changes related to COPD, such as alterations in muscle tissue, fat distribution, bone density, and coronary artery changes, indicating the effects of extrapulmonary manifestations. These extrapulmonary presentations were crucial for understanding the comprehensive impact of COPD, improving patient management strategies, and optimizing treatment plans. A comprehensive literature review spanning the past 25 years was conducted using the PubMed and Web of Science databases. Following an extensive analysis and critical discussion of the retrieved studies, this review was subsequently completed.
In the field of COPD research, the diagnosis and exploration of extrapulmonary comorbidities not only focused on revealing the various disease characteristics and pathophysiological changes accompanying different individuals but also strived to correlate these characteristics with clinically significant outcomes. These include key indicators such as acute exacerbation of COPD, the ongoing deterioration of the disease, and mortality risk. Therefore, a significant number of articles in this field use prospective design methodologies to rigorously verify and quantify the analysis of every possible extrapulmonary manifestation and its relationship with COPD prognosis, aiming to provide more precise and comprehensive patient disease management strategies.
However, comprehensive analyses of extrapulmonary comorbidities of COPD using CT were relatively scarce in the literature. It was noted that most extrapulmonary manifestations shared common characteristics of chronic systemic inflammation, which all increase the incidence of COPD (6).
According to the content released by Global Initiative for Chronic Obstructive Lung Disease (GOLD)2024, CT can provide extensive information on extrapulmonary manifestations, including muscle mass, bone density, fat area, and coronary calcification (Table 1). We present this article in accordance with the Narrative Review reporting checklist (available at https://jtd.amegroups.com/article/view/10.21037/jtd-24-1074/rc).
Table 1
Quantitative metric | Definition | Comments |
---|---|---|
Midthigh muscle cross-sectional area | Obtained from CT scans of the thigh between the pubic symphysis and the femoral condyle | Related to predicting mortality |
Pectoralis muscle area | Area of the pectoral muscle measured in the first axial image immediately above the aortic arch | Positively correlated with lung function; predicts disease progression |
Erector spinae muscles | Measurement of the muscle area of erector spinae at the level of the 12th thoracic vertebra | Related to predicting mortality (7) |
Paoas muscles | Measured area of the left and right psoas muscles at the level of the third lumbar vertebra | Related to predicting mortality (8) |
Bone density (use mineral reference phantom) | Measured bone mineral density of three consecutive thoracic vertebrae from a section containing the caudal side of the left coronary artery | Low bone density was associated with fracture risk and mortality |
Bone density (no mineral reference phantom) | Average bone attenuation of thoracic vertebrae T4, T7, and T10 measured in HU | Low bone density was associated with fracture risk and mortality; negative correlation with the degree of emphysema |
Adipose tissue | Defined by measuring the area between the pectoralis major muscle and skin surface, identifying subcutaneous fat | Decrease in subcutaneous fat was related to increased mortality, while intramuscular fat has the opposite effect |
CT, computed tomography; HU, Hounsfield unit.
Methods
A comprehensive literature search was conducted to identify studies on extrapulmonary manifestations of COPD as observed through quantitative computed tomography (QCT). Utilizing PubMed and Web of Science databases, the search encompassed publications from January 1999 to May 2024. Keywords such as “COPD”, “chronic obstructive pulmonary disease”, “muscle”, “adipose tissue”, “coronary artery calcification”, “bone density”, “extrapulmonary manifestations”, and “Quantitative Computed Tomography” were employed. Inclusion criteria focused on studies involving COPD patients, using QCT to identify extrapulmonary manifestations, published in peer-reviewed journals, and available in English (Table 2, Table S1).
Table 2
Items | Specification |
---|---|
Date of search | June 10, 2024 |
Databases and other sources searched | PubMed and Web of Science databases |
Search terms used | COPD-related terms: “COPD” (MeSH), “chronic obstructive pulmonary disease” (free text) |
Extrapulmonary Manifestations Terms: “muscle” (MeSH), “muscle” (free text), “adipose tissue” (MeSH), “adipose tissue” (free text), “coronary artery calcification” (MeSH), “coronary artery calcification” (free text), “bone density” (MeSH), “bone density” (free text), “extrapulmonary manifestations” (free text) | |
CT-related terms: “Quantitative Computed Tomography” (MeSH), “Computed Tomography” (free text), “CT” (free text) | |
Timeframe | January 1, 1999 to May 31, 2024 |
Inclusion and exclusion criteria | Inclusion criteria: research involving patients with chronic obstructive pulmonary disease; using nuclear medicine related equipment (such as X-rays, CT) to identify extrapulmonary manifestations; published in peer-reviewed journals; there was an English version available |
Exclusion criteria: the study does not focus on the extrapulmonary manifestations of chronic obstructive pulmonary disease; research does not use nuclear medicine related equipment (such as X-rays, CT) as diagnostic tools; review articles, case reports, conference abstracts, or editorials; there was no English version available for the research | |
Selection process | The selection process was independently conducted by two respiratory doctors, C.M. and F.W. Any differences were resolved through discussion or negotiation with a third, more experienced respiratory specialist, R.C., to reach a consensus |
COPD, chronic obstructive pulmonary disease; CT, computed tomography; MeSH, medical subject headings.
Literature search and screening results
Using databases such as PubMed and Web of Science, a total of 221 articles were initially identified for further screening. Among these, 81 duplicate studies were excluded. Each article was evaluated based on its title and abstract, leading to the exclusion of studies with inconsistent populations and case reports. Ultimately, 41 studies pertinent to the research topic were identified (Figure S1).
CT measurement of muscle mass
The term “muscle mass” referred to a concept rather than a specific muscle. The relevance of muscle to COPD can be traced back to the correlation between the cross-sectional area of leg muscles and the survival rate of COPD patients. It was believed that the cross-sectional area of leg muscles has a closer relationship with patient survival compared to body weight (9). The pectoralis muscle, often studied, was measured by CT in terms of its cross-sectional area, which was thought to predict disease progression (10-14) and was related to systemic inflammation (Figure 1) (15). Some researchers have measured the erector spinae muscles (7,12,16) and the bilateral psoas muscles (8), all of which were associated with the mortality rate of COPD patients. Compared to the cross-sectional area of the pectoralis muscles, the erector spinae muscles were believed to have a more significant relationship with the mortality rate in COPD patients (12).
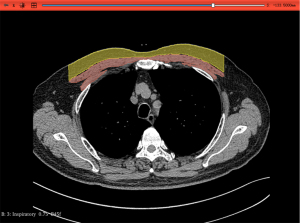
Interestingly, pectoral muscle area (PMA), as a non-respiratory muscle, participated in assisting respiration and was considered related to lung function (11,12,14,17). This may be due to a decline in lung function in COPD patients, leading to increased muscle catabolism, muscle atrophy, and overall fatigue (17), although a study does not associate lung function decline with COPD (18). This could be due to differences in inclusion criteria, with some teams mostly including patients in the early to middle stages of COPD, where muscle loss was less (17); or due to variations in patient positioning, affecting the results of the analysis; additionally, different CT measurement locations can also lead to varying results. With age, muscle area and density decrease, which also impacts our measurements, and this decline was faster in COPD patients (19). Excessive chemotaxis of neutrophils and heightened extracellular protease activity may reflect the significant effects of lung inflammation on systemic circulation, skeletal muscle, and bone marrow, potentially leading to the induction or exacerbation of comorbidities such as sarcopenia associated with COPD (20).
CT measurement of bone density
At the initial stage of using CT to assess bone mineral density (BMD), people will use a mineral reference (bone) phantom to scan CT with patients to ensure that the measured Hounsfield unit (HU) value can be converted into reference values such as BMD (21-23). However, more and more studies have shown that HU value was closely related to BMD, and BMD can be determined only by HU value (24,25). Some studies may choose the left main coronary artery level (LML) as the entry point to measure the HU value of the region of interest (21,22,25,26) (Figure 2), while others may choose to measure the HU value of the region of interest of thoracic (T) 4, T7, T10, and first lumbar vertebra (24,27,28). Both of these measurement methods were widely accepted.
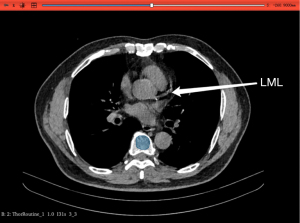
In the 1970s, the Hounsfield-developed clinical CT provided precise information on tissue density from X-ray attenuation, making it akin to a scanner. Nowadays, most studies measuring bone density using CT utilize bone attenuation values, which, in comparison to dual-energy X-ray absorptiometry (DXA), made CT more practical (29), and even better at identifying low bone density than DXA (30). Bone density was considered closely related to low bone density and fracture risk in most studies (10,23), and low bone density was associated with mortality (25,31). Interestingly, a study has found no relationship between bone density and all-cause mortality (24). The conclusions of these studies may vary due to differences in sample characteristics and size, with patient groups differing in age, sex, disease severity, and other health conditions.
COPD patients generally experienced significant oxidative stress, with excessive free radical production and accumulated cellular damage, not only harming lung tissue but also potentially disrupting the balance of bone cells, leading to a decline in bone density (32). In an earlier study using CT to measure bone density, it was believed that bone density had a negative correlation with emphysema indicators (28). In 2012, the team discovered that subjects with a history of acute exacerbations exhibited a greater decline in thoracic vertebral BMD compared to those without such a history (33). Additionally, Graumam found a correlation between bone density and a decline in forced expiratory volume in 1 second (FEV1) (34). However, Kenichi Goto’s 2018 publication argued that bone density was not related to FEV1 (35), possibly due to differences in testing methods: Graumam used DXA technology to measure lumbar spine, femoral neck, and total femur density (34), while Goto used CT to measure the attenuation value of all thoracic vertebrae (35).
Their inclusion criteria and statistical methods also differed, leading to different measurement results. Overall, CT’s application in assessing bone density in COPD patients has shown unique advantages. It not only reflects pathological changes in the skeletal system of COPD patients but also aids in predicting the prognosis and risk of complications. Although in some cases, the method of assessing bone density with thoracic vertebral CT remains limited in its accuracy and inability to cover all osteoporosis-related factors comprehensively, its convenience in clinical practice and the potential for concurrent assessment with lung disease make it a promising evaluation tool.
Measurement of adipose tissue
In some studies, the measurement of fat was conducted simultaneously with the measurement of the pectoral muscles, as the chosen cross-sections were the same, most commonly at the level of the sternal notch, which was easily accessible (Figure 1) (15,36). A reduction in subcutaneous fat has been associated with an increased mortality rate, whereas the role of intermuscular fat appears to be the opposite (37). In fat-related research, gender factors were considered, revealing a significant association between the area of subcutaneous fat and the concentration of C-reactive protein and fibrinogen in the blood (r=0.51) (15). This could be due to the dysfunction of adipose tissue, such as a state of chronic low-grade inflammation, which might affect the immune response in the lungs and promote airway hyperreactivity (38).
Measurement of coronary artery calcification (CAC)
Cardiovascular diseases accounted for the majority of deaths in mild to moderate COPD, with most patients being asymptomatic (39). Long-standing hypoxemia and limited respiratory function in COPD patients further increase the risk of cardiovascular disease (40). In the intrinsic connection between COPD and coronary artery disease (CAD), both shared several pathophysiological mechanisms, such as endothelial dysfunction and increased arterial stiffness (6). Over-inflation of the lungs, leading to decreased efficiency of the respiratory muscles and respiratory distress, together with systemic inflammation and oxidative stress, promotes the development and progression of arteriosclerosis (41). Moreover, the acute exacerbation of COPD was related to the increase of cardiovascular disease (42).
A study indicated that CT imaging of CAC provided a more effective risk assessment than scoring systems used in the general population (43). Williams found that the coronary artery calcification score (CACS) was not related to emphysema and lung function, but an increase in CACS was associated with an increased mortality rate in COPD patients (44). In the article published in 2011, it was mentioned that electrocardiogram (ECG)-gated and non-gated CT were almost equally accurate in measuring thoracic aortic calcification (45). Research teams, through baseline CT scans, assessed Agatston and Weston scores, finding an inverse relationship between FEV1 and Agatston score for coronary calcification, with visual scoring of CAC related to airflow obstruction and cardiovascular events. The team also believed that visual scoring was more applicable in clinical practice (39).
However, coronary angiography remains the gold standard for the assessment of CAD. But CT examination plays a significant role in early screening and risk prediction of coronary arteriosclerosis in patients, and can reduce the cost of examination.
Future research directions
CT was becoming increasingly important in the diagnosis and treatment of COPD, particularly regarding its extrapulmonary manifestations (46). Recent advancements have highlighted CT’s role in assessing disease progression and treatment response (47). While routine clinical examinations may overlook extrapulmonary signs, CT images provide crucial insights, especially in predicting patient mortality and detecting latent issues early (48) (Table 3).
Table 3
Study focus | Study design | Sample sizes | Key findings related to COPD |
---|---|---|---|
Cross-sectional area of leg muscles (9) | Prospective cohort | 142 COPD patients | Significant predictor of mortality rate |
Pectoralis muscle | Prospective cohort (14) | 1,352 subjects | Positive correlation with lung function |
Cross-sectional observational study (10) | Two queues totaling 1,309 subjects | Significant correlation with lung function, dyspnea, and 6-minute walking distance | |
Spinae muscles (49) | Prospective cohort | 150 subjects | Significantly correlated with previously reported prognostic factors |
Psoas muscles (8) | Prospective cohort | 220 COPD patients | Variables independently correlated with all-cause mortality rate |
T4, T7, T10, and L1 bone density (28) | Cross-sectional observational study | 65 male COPD patients | Correlated with the extent of pulmonary emphysema significantly |
Bone density at the left main coronary artery (25) | Prospective cohort | 322 subjects | Significant correlation between patient mortality and disease severity |
Adipose tissue (37) | Prospective cohort | 2,994 subjects | Negative correlation with mortality rate |
Coronary artery calcification (44) | Prospective cohort | 942 subjects | Associated with increased dyspnoea, reduced exercise capacity and increased mortality |
COPD, chronic obstructive pulmonary disease.
However, there were significant challenges in current practices, including the variability in interpreting CT findings and the potential for missed diagnoses of extrapulmonary complications (49). These limitations underscore the need for improved diagnostic tools.
In this context, the integration of artificial intelligence (AI) techniques presents a promising solution. AI can enhance the analysis of CT images, improving the accuracy of extrapulmonary manifestation detection and enabling better risk stratification for patients (48). By automating and standardizing image analysis, AI can help overcome the subjective nature of traditional assessments.
Currently, a significant breakthrough was reported in a 2023 academic paper by the research team led by Professor Daniel Genkin, who developed an innovative process for the fully automatic extraction of PMA from chest CT scans (50). This advanced automated processing pipeline successfully achieves precise identification of CT slices at the level of the aortic arch and nearly perfectly simulates the manual segmentation technique of the pectoralis major muscle, with results nearly indistinguishable from manual operations. This key technological breakthrough significantly advances the automation process of muscle quantification, making it more convenient to assess muscle mass in the future.
The future research trend and technological development in this field were characterized by multi-dimensionality, precision, and intelligence. Scientists were dedicated to developing and perfecting advanced CT analysis methods aimed at providing one-stop solutions for simultaneous assessments of multiple systems such as pulmonary, cardiovascular, skeletal, and muscular. Systemic indicators like CAC, thoracic aorta calcification (51,52), bone density, and muscle mass were expected to be more comprehensively analyzed in a single CT scan, deeply revealing the overall health status of COPD patients.
The development of intelligent automation technology has become a key driving force in this area, especially through the creation of fully automated or semi-automated analysis platforms integrating AI and machine learning algorithms (53). Such platforms can accurately extract and quantify various extrapulmonary disease indicators from chest CT images, such as pectoralis major area, subcutaneous fat, visceral fat, and bone density, thereby significantly reducing human error and enhancing diagnostic accuracy and efficiency.
Interdisciplinary research was deepening, exploring the intrinsic connections between COPD and other chronic diseases such as cardiovascular disease, osteoporosis, and muscle atrophy, and using CT imaging to find new early warning biomarkers and prognostic indicators to provide a scientific basis for developing personalized treatment strategies (54).
Moreover, by delving deeper into CT data, researchers will further reveal the close relationship between extrapulmonary manifestations and the prognosis of COPD patients, including core elements such as survival rate, incidence rate, and quality of life. Based on this, treatment plans and prevention strategies can be optimized, pushing disease management towards greater precision.
Based on the systemic disease information obtained from CT assessments, researchers can finely stratify risks for COPD patients, designing targeted individualized treatment plans for different types of patients (55). For example, specific interventions can be implemented for COPD patients with concurrent osteoporosis, and appropriate nutritional supplements and exercise therapies can be recommended for patients with decreased muscle mass.
Technological advancements will also promote the development of real-time monitoring and long-term tracking techniques, using advanced dynamic CT imaging technology and rigorous follow-up protocols to continuously and real-time monitor changes in the body composition of COPD patients, enabling timely adjustment of treatment plans and preventing complications.
To address the challenges in assessing extrapulmonary manifestations in COPD, it was essential to establish a standardized framework for CT analysis. Currently, inconsistencies in methodologies can lead to variability in results, undermining the reliability and comparability of findings across studies. Thus, standardization and normalization of CT analysis processes were critical. By implementing unified standards and guidelines, we can enhance the consistency of CT assessments globally, making them widely recognized and adopted.
Furthermore, another significant issue was the lack of standardization for normal and reference values related to muscle assessments in CT. This gap highlights the necessity for a comprehensive review that explores reference values and their implications in clinical practice. Addressing these standardization issues not only clarifies existing problems but also paves the way for more accurate and universally applicable assessments in COPD research.
Conclusions
In summary, CT was becoming increasingly important in the diagnosis and treatment of COPD, and its importance in extrapulmonary manifestations was equally significant. In recent years, CT has been widely used to assess the progression of COPD and its response to treatment. Through this comprehensive analysis, we recognize that even though extrapulmonary signs in COPD patients may not be easily detected in routine clinical examinations, they were clearly visible in CT images, especially in predicting patient mortality risk and early detection of latent issues. Further exploration and analysis of extrapulmonary manifestations, based on a detailed assessment of pulmonary changes, will aid in devising more accurate and effective treatment plans for patients. Given the importance of CT in diagnosing extrapulmonary manifestations of COPD, more scientific research is urgently needed to determine the most suitable measurement tools and methods and define critical thresholds that reflect treatment efficacy.
Acknowledgments
None.
Footnote
Reporting Checklist: The authors have completed the Narrative Review reporting checklist. Available at https://jtd.amegroups.com/article/view/10.21037/jtd-24-1074/rc
Peer Review File: Available at https://jtd.amegroups.com/article/view/10.21037/jtd-24-1074/prf
Funding: This study was funded by
Conflicts of Interest: All authors have completed the ICMJE uniform disclosure form (available at https://jtd.amegroups.com/article/view/10.21037/jtd-24-1074/coif). R.C. serves as an unpaid associate Editor-in-Chief of Journal of Thoracic Disease. The other authors have no conflicts of interest to declare.
Ethical Statement: The authors are accountable for all aspects of the work in ensuring that questions related to the accuracy or integrity of any part of the work are appropriately investigated and resolved.
Open Access Statement: This is an Open Access article distributed in accordance with the Creative Commons Attribution-NonCommercial-NoDerivs 4.0 International License (CC BY-NC-ND 4.0), which permits the non-commercial replication and distribution of the article with the strict proviso that no changes or edits are made and the original work is properly cited (including links to both the formal publication through the relevant DOI and the license). See: https://creativecommons.org/licenses/by-nc-nd/4.0/.
References
- Venkatesan P. GOLD COPD report: 2023 update. Lancet Respir Med 2023;11:18. [Crossref] [PubMed]
- Liang Z, Long F, Wang F, et al. Identification of clinically relevant subgroups of COPD based on airway and circulating autoantibody profiles. Mol Med Rep 2019;20:2882-92. [Crossref] [PubMed]
- López-Campos JL, Tan W, Soriano JB. Global burden of COPD. Respirology 2016;21:14-23. [Crossref] [PubMed]
- Mannino DM, Buist AS. Global burden of COPD: risk factors, prevalence, and future trends. Lancet 2007;370:765-73. [Crossref] [PubMed]
- McDonough JE, Yuan R, Suzuki M, et al. Small-airway obstruction and emphysema in chronic obstructive pulmonary disease. N Engl J Med 2011;365:1567-75. [Crossref] [PubMed]
- Cavaillès A, Brinchault-Rabin G, Dixmier A, et al. Comorbidities of COPD. Eur Respir Rev 2013;22:454-75. [Crossref] [PubMed]
- Tanimura K, Sato S, Fuseya Y, et al. Quantitative Assessment of Erector Spinae Muscles in Patients with Chronic Obstructive Pulmonary Disease. Novel Chest Computed Tomography-derived Index for Prognosis. Ann Am Thorac Soc 2016;13:334-41. [Crossref] [PubMed]
- Ezponda A, Casanova C, Cabrera C, et al. Psoas Muscle Density Evaluated by Chest CT and Long-Term Mortality in COPD Patients. Arch Bronconeumol 2021;57:533-9. [PubMed]
- Marquis K, Debigaré R, Lacasse Y, et al. Midthigh muscle cross-sectional area is a better predictor of mortality than body mass index in patients with chronic obstructive pulmonary disease. Am J Respir Crit Care Med 2002;166:809-13. [Crossref] [PubMed]
- McDonald ML, Diaz AA, Ross JC, et al. Quantitative computed tomography measures of pectoralis muscle area and disease severity in chronic obstructive pulmonary disease. A cross-sectional study. Ann Am Thorac Soc 2014;11:326-34. [Crossref] [PubMed]
- Bak SH, Kwon SO, Han SS, et al. Computed tomography-derived area and density of pectoralis muscle associated disease severity and longitudinal changes in chronic obstructive pulmonary disease: a case control study. Respir Res 2019;20:226. [Crossref] [PubMed]
- Attaway AH, Welch N, Yadav R, et al. Quantitative Computed Tomography Assessment of Pectoralis and Erector Spinae Muscle Area and Disease Severity in Chronic Obstructive Pulmonary Disease Referred for Lung Volume Reduction. COPD 2021;18:191-200. [Crossref] [PubMed]
- Wilson AC, Bon JM, Mason S, et al. Increased chest CT derived bone and muscle measures capture markers of improved morbidity and mortality in COPD. Respir Res 2022;23:311. [Crossref] [PubMed]
- Zhou K, Wu F, Zhao N, et al. Association of pectoralis muscle area on computed tomography with airflow limitation severity and respiratory outcomes in COPD: A population-based prospective cohort study. Pulmonology 2025;31:2416782. [Crossref] [PubMed]
- Diaz AA, Zhou L, Young TP, et al. Chest CT measures of muscle and adipose tissue in COPD: gender-based differences in content and in relationships with blood biomarkers. Acad Radiol 2014;21:1255-61. [Crossref] [PubMed]
- Shirahata T, Sato H, Yogi S, et al. The product of trunk muscle area and density on the CT image is a good indicator of energy expenditure in patients with or at risk for COPD. Respir Res 2021;22:18. [Crossref] [PubMed]
- Qiao X, Hou G, Kang J, et al. CT Attenuation and Cross-Sectional Area of the Pectoralis Are Associated With Clinical Characteristics in Chronic Obstructive Pulmonary Disease Patients. Front Physiol 2022;13:833796. [Crossref] [PubMed]
- O'Brien ME, Zou RH, Hyre N, et al. CT pectoralis muscle area is associated with DXA lean mass and correlates with emphysema progression in a tobacco-exposed cohort. Thorax 2023;78:394-401. [PubMed]
- Wang Y, Li S, Zhang Z, et al. Accelerated loss of trunk muscle density and size at L1 vertebral level in male patients with COPD. Front Endocrinol (Lausanne) 2022;13:1087110. [PubMed]
- Gea J, Pascual S, Casadevall C, et al. Muscle dysfunction in chronic obstructive pulmonary disease: update on causes and biological findings. J Thorac Dis 2015;7:E418-38. [PubMed]
- Budoff MJ, Hamirani YS, Gao YL, et al. Measurement of thoracic bone mineral density with quantitative CT. Radiology 2010;257:434-40. [PubMed]
- Budoff MJ, Khairallah W, Li D, et al. Trabecular bone mineral density measurement using thoracic and lumbar quantitative computed tomography. Acad Radiol 2012;19:179-83. [PubMed]
- Jaramillo JD, Wilson C, Stinson DS, et al. Reduced Bone Density and Vertebral Fractures in Smokers. Men and COPD Patients at Increased Risk. Ann Am Thorac Soc 2015;12:648-56. [PubMed]
- Romme EA, Murchison JT, Edwards LD, et al. CT-measured bone attenuation in patients with chronic obstructive pulmonary disease: relation to clinical features and outcomes. J Bone Miner Res 2013;28:1369-77. [Crossref] [PubMed]
- Hwang HJ, Lee SM, Seo JB, et al. Quantitative Vertebral Bone Density Seen on Chest CT in Chronic Obstructive Pulmonary Disease Patients: Association with Mortality in the Korean Obstructive Lung Disease Cohort. Korean J Radiol 2020;21:880-90. [Crossref] [PubMed]
- Mao SS, Li D, Syed YS, et al. Thoracic Quantitative Computed Tomography (QCT) Can Sensitively Monitor Bone Mineral Metabolism: Comparison of Thoracic QCT vs Lumbar QCT and Dual-energy X-ray Absorptiometry in Detection of Age-relative Change in Bone Mineral Density. Acad Radiol 2017;24:1582-7. [Crossref] [PubMed]
- Kim YW, Kim JH, Yoon SH, et al. Vertebral bone attenuation on low-dose chest CT: quantitative volumetric analysis for bone fragility assessment. Osteoporos Int 2017;28:329-38. [Crossref] [PubMed]
- Ohara T, Hirai T, Muro S, et al. Relationship between pulmonary emphysema and osteoporosis assessed by CT in patients with COPD. Chest 2008;134:1244-9. [Crossref] [PubMed]
- Romme EA, Murchison JT, Phang KF, et al. Bone attenuation on routine chest CT correlates with bone mineral density on DXA in patients with COPD. J Bone Miner Res 2012;27:2338-43. [Crossref] [PubMed]
- Fountoulis G, Kerenidi T, Kokkinis C, et al. Assessment of Bone Mineral Density in Male Patients with Chronic Obstructive Pulmonary Disease by DXA and Quantitative Computed Tomography. Int J Endocrinol 2016;2016:6169721. [Crossref] [PubMed]
- Katsura H, Kida K. A comparison of bone mineral density in elderly female patients with COPD and bronchial asthma. Chest 2002;122:1949-55. [Crossref] [PubMed]
- Stanojkovic I, Kotur-Stevuljevic J, Spasic S, et al. Relationship between bone resorption, oxidative stress and inflammation in severe COPD exacerbation. Clin Biochem 2013;46:1678-82. [Crossref] [PubMed]
- Kiyokawa H, Muro S, Oguma T, et al. Impact of COPD exacerbations on osteoporosis assessed by chest CT scan. COPD 2012;9:235-42. [Crossref] [PubMed]
- Graumam RQ, Pinheiro MM, Nery LE, et al. Increased rate of osteoporosis, low lean mass, and fragility fractures in COPD patients: association with disease severity. Osteoporos Int 2018;29:1457-68. [Crossref] [PubMed]
- Goto K, Ogawa E, Shimizu K, et al. Relationship of annual change in bone mineral density with extent of emphysematous lesions and pulmonary function in patients with COPD. Int J Chron Obstruct Pulmon Dis 2018;13:639-44. [Crossref] [PubMed]
- McDonald MN, Diaz AA, Rutten E, et al. Chest computed tomography-derived low fat-free mass index and mortality in COPD. Eur Respir J 2017;50:1701134. [Crossref] [PubMed]
- Pishgar F, Shabani M, Quinaglia A C, Silva T, et al. Quantitative Analysis of Adipose Depots by Using Chest CT and Associations with All-Cause Mortality in Chronic Obstructive Pulmonary Disease: Longitudinal Analysis from MESArthritis Ancillary Study. Radiology 2021;299:703-11. [Crossref] [PubMed]
- Palma G, Sorice GP, Genchi VA, et al. Adipose Tissue Inflammation and Pulmonary Dysfunction in Obesity. Int J Mol Sci 2022;23:7349. [Crossref] [PubMed]
- Bhatt SP, Kazerooni EA, Newell JD Jr, et al. Visual Estimate of Coronary Artery Calcium Predicts Cardiovascular Disease in COPD. Chest 2018;154:579-87. [Crossref] [PubMed]
- Paganelli F, Gaudry M, Ruf J, et al. Recent advances in the role of the adenosinergic system in coronary artery disease. Cardiovasc Res 2021;117:1284-94. [Crossref] [PubMed]
- Roversi S, Roversi P, Spadafora G, et al. Coronary artery disease concomitant with chronic obstructive pulmonary disease. Eur J Clin Invest 2014;44:93-102. [Crossref] [PubMed]
- Donaldson GC, Hurst JR, Smith CJ, et al. Increased risk of myocardial infarction and stroke following exacerbation of COPD. Chest 2010;137:1091-7. [PubMed]
- Yeboah J, McClelland RL, Polonsky TS, et al. Comparison of novel risk markers for improvement in cardiovascular risk assessment in intermediate-risk individuals. JAMA 2012;308:788-95. [PubMed]
- Williams MC, Murchison JT, Edwards LD, et al. Coronary artery calcification is increased in patients with COPD and associated with increased morbidity and mortality. Thorax 2014;69:718-23. [PubMed]
- Budoff MJ, Nasir K, Kinney GL, et al. Coronary artery and thoracic calcium on noncontrast thoracic CT scans: comparison of ungated and gated examinations in patients from the COPD Gene cohort. J Cardiovasc Comput Tomogr 2011;5:113-8. [PubMed]
- Lin A, Kolossváry M, Motwani M, et al. Artificial intelligence in cardiovascular CT: Current status and future implications. J Cardiovasc Comput Tomogr 2021;15:462-9. [PubMed]
- Park C, Lee BC, Jeong WG, et al. Coronary Artery Calcification on Low-Dose Lung Cancer Screening CT in South Korea: Visual and Artificial Intelligence-Based Assessment and Association With Cardiovascular Events. AJR Am J Roentgenol 2024;222:e2430852. [Crossref] [PubMed]
- Wu Y, Xia S, Liang Z, et al. Artificial intelligence in COPD CT images: identification, staging, and quantitation. Respir Res 2024;25:319. [Crossref] [PubMed]
- Tanimura K, Sato S, Fuseya Y, et al. Quantitative Assessment of Erector Spinae Muscles in Patients with Chronic Obstructive Pulmonary Disease. Novel Chest Computed Tomography-derived Index for Prognosis. Ann Am Thorac Soc 2016;13:334-41. [Crossref] [PubMed]
- Genkin D, Jenkins AR, van Noord N, et al. A fully automated pipeline for the extraction of pectoralis muscle area from chest computed tomography scans. ERJ Open Res 2024;10:00485-2023. [Crossref] [PubMed]
- Jobst BJ, Owsijewitsch M, Kauczor HU, et al. GOLD stage predicts thoracic aortic calcifications in patients with COPD. Exp Ther Med 2019;17:967-73. [PubMed]
- Dransfield MT, Huang F, Nath H, et al. CT emphysema predicts thoracic aortic calcification in smokers with and without COPD. COPD 2010;7:404-10. [Crossref] [PubMed]
- Makimoto K, Hogg JC, Bourbeau J, et al. CT Imaging With Machine Learning for Predicting Progression to COPD in Individuals at Risk. Chest 2023;164:1139-49. [Crossref] [PubMed]
- Pasteur-Rousseau A, Paul JF. Artificial Intelligence and teleradiology in cardiovascular imaging by CT-Scan and MRI. Ann Cardiol Angeiol (Paris) 2021;70:339-47. [Crossref] [PubMed]
- Zou D, Zhu X. Association of CT phenotype with pulmonary function in patients with chronic obstructive pulmonary disease and influencing factors of prognosis. Am J Transl Res 2023;15:2164-74. [PubMed]