Influencing factors of 90-day readmission of patients with heart failure based on the MIMIC-IV database
Highlight box
Key findings
• Charlson Comorbidity Index (CCI), red blood cell count (RBC), red cell distribution width (RDW), and age were the main factors influencing the 90-day readmission of patients with heart failure (HF). RBC and RDW were linearly correlated with the 90-day readmission of patients with HF, while CCI and age were nonlinearly correlated with the 90-day readmission of patients with HF.
What is known, and what is new?
• It is known that CCI, RBC, RDW, and age were the factors influencing the prognosis of patients with HF.
• This study revealed that RBC and RDW were linearly correlated with the 90-day readmission of patients with HF, while CCI and age were nonlinearly correlated with the 90-day readmission of patients with HF.
What is the implication, and what should change now?
• For patients with HF, clinicians should pay attention to patients’ CCI, RBC, RDW, and age.
Introduction
Cardiovascular diseases are a major public health problem that threatens the lives and health of the world’s residents. About 330 million people in China suffer from cardiovascular diseases, of which about 8.9 million suffer from heart failure (HF) (1). HF is a serious manifestation or advanced stage of various heart diseases (2). HF is a complex clinical syndrome characterized by dyspnea, fatigue, and fluid retention (pulmonary stasis, circulatory stasis, and peripheral edema) due to a variety of causes that result in abnormal changes in the structure and/or function of the heart, resulting in ventricular systolic and/or diastolic dysfunction (3). The prevalence of HF was approximately 2.4–3.0% in the American population, 2.3% in the French population, and 3–4% in the Canadian population; while the incidence rate of HF was approximately 2.2–3.2% in the American population, 6.5% in the Germany population, and 5.1–8.1% in the Canadian population (4).
Due to the severity of HF disease, HF patients have a poor prognosis. The hospitalization rate for the U.S. youth HF population was 4.32 per 10,000 people (5). The 30- and 60-day readmission rates of children with HF in the United States were 19.5% and 27.5% respectively (6). Soetjoadi et al. conducted a retrospective study of 208 patients with right HF and found that the overall hospital mortality rate for patients was 12.5% (7). Kito and other scholars investigated the elderly inpatients with acute decompensated HF and found that 20% of the elderly patients had decreased instrumental activities of daily living during hospitalization, 9.2% of the elderly patients died within one year, and 6.0% of the elderly patients died of cardiovascular diseases (8). In addition to this, HF is prone to complications, with 10% to 35% of patients with chronic HF having atrial fibrillation.
HF is characterized by high morbidity and high treatment costs. Prognostic analysis studies of HF patients are very important to reduce the healthcare costs of patients and improve clinical outcomes. At this stage, the prognostic analysis of HF patients mainly focused on death and 30-day readmission, and fewer studies focused on 90-day readmission; therefore, this study conducted a retrospective study based on a large sample of public databases to explore the risk factors for 90-day readmission in HF patients. We present this article in accordance with the TRIPOD reporting checklist (available at https://jtd.amegroups.com/article/view/10.21037/jtd-24-1738/rc).
Methods
Data source
Patient information collected for this study was obtained from the Medical Information Mart for Intensive Care (MIMIC)-IV database, which was established by the Laboratory of Computational Physiology at the Massachusetts Institute of Technology, the Beth Israel Deaconess Medical Center at Harvard Medical School, and Philips Healthcare to provide clinical researchers with a database of clinical data. The study was conducted in accordance with the Declaration of Helsinki (as revised in 2013).
Inclusion and exclusion criteria
Inclusion criteria: patients diagnosed with HF.
Exclusion criteria: patients with multiple records of HF, patients who died in hospital, patients who lacked readmission time, patients whose readmission time was less than 1 day, and patients whose hospitalization time was less than 2 days.
Indicators
The main outcome of this study was 90-day readmission. In this study, the study population was divided into a control group and a 90-day readmission group based on whether the HF patients were readmitted within 90 days. The 90-day readmission group was readmitted within 90 days, whereas the control group was readmitted for more than 90 days.
Indicators included age, body mass index (BMI), glucose, Charlson Comorbidity Index (CCI), alanine aminotransferase (ALT), aspartate aminotransferase (AST), creatinine, potassium, sodium, calcium, estimated glomerular filtration rate (eGFR), blood urea nitrogen, platelets, red cell distribution width (RDW), white cell count (WBC), red blood cell count (RBC), hemoglobin, anion gap, bicarbonate, prothrombin time (PT), sex (male, female), race (white, other), marital status (single, married, divorce, widowed), echocardiography (yes, no), alcohol use (yes, no), tobacco use (yes, no), myocardial infarction (yes, no), diabetes (yes, no), stroke (yes, no), dyslipidemia (yes, no), hypertension (yes, no), atrial fibrillation (yes, no), renal disease (yes, no), peripheral vascular disease (yes, no), chronic pulmonary disease (yes, no), pneumonia (yes, no), asthma (yes, no), aspirin (yes, no), warfarin (yes, no), metoprolol (yes, no), atenolol (yes, no), furosemide (yes, no).
90-day readmission: the patient was readmitted to the hospital within 90 days after discharge due to illness and other reasons.
Statistical analysis
R language was used for data analysis. The quantitative data of non-normal distribution were described by median (P75–P25) and compared by the Mann-Whitney U test. Qualitative data were described by composition ratio, and compared between groups by Chi-squared test. Least absolute shrinkage and selection operator (LASSO) regression was used to perform variable screening. Multivariate logistic regression was used to analyze the influencing factors of 90-day readmission of patients with HF. Logistic classification, eXtreme gradient boosting (XGBoost) regression, and random forest were used to rank the influencing factors of 90-day readmission of patients with HF, and the Wayne diagram extracted the common factors of the top five influencing factors obtained by the three methods. Trend regression analysis and restricted cubic spline (RCS) analysis were used to analyze the correlation between these factors and the 90-day readmission of patients with HF. P<0.05 was considered to be statistically significant.
Results
Patient baseline information
A total of 8,300 patients with HF were enrolled in this study, of which 3,893 patients with HF were readmitted within 90 days. As shown in Table 1, there were differences between the two groups in age (P<0.001), BMI (P<0.001), CCI (P<0.001), potassium (P=0.03), calcium (P<0.001), eGFR (P<0.001), blood urea nitrogen (P<0.001), RDW (P<0.001), RBC (P<0.001), hemoglobin (P<0.001), bicarbonate (P=0.003), race (P<0.001), echocardiography (P<0.001), alcohol use (P<0.001), tobacco use (P<0.001), myocardial infarction (P=0.01), stroke (P<0.001), hypertension (P=0.006), atrial fibrillation (P=0.002), renal disease (P=0.01), peripheral vascular disease (P<0.001), chronic pulmonary disease (P=0.02), pneumonia (P=0.01), asthma (P<0.001), metoprolol (P=0.002), atenolol (P=0.047), furosemide (P<0.001).
Table 1
Variable | Control | 90-day readmission | P |
---|---|---|---|
Age (years) | 73 [63–82] | 75 [64–84] | <0.001 |
BMI (kg/m2) | 29.6 [25.2–35.1] | 28.4 [24.4–33.6] | <0.001 |
Glucose (mg/dL) | 119 [98–155] | 119 [97–157] | 0.54 |
CCI (scores) | 6 [5–8] | 7 [5–8] | <0.001 |
ALT (IU/L) | 22 [14–39] | 22 [14–41] | 0.40 |
AST (IU/L) | 28 [19–44] | 28 [20–47] | 0.11 |
Creatinine (mg/dL) | 1.2 [0.9–1.8] | 1.2 [0.9–1.8] | 0.23 |
Potassium (mEq/L) | 4.2 [3.8–4.6] | 4.20 [3.8–4.6] | 0.03 |
Sodium (mEq/L) | 139 [137–141] | 139 [136–141] | 0.07 |
Calcium (mg/dL) | 8.8 [8.4–9.2] | 8.8 [8.3–9.1] | <0.001 |
eGFR (mL/min/1.73 m2) | 0.993 [0.792–1.036] | 0.992 [0.788–1.030] | <0.001 |
Blood urea nitrogen (mg/dL) | 24 [17–38] | 26 [18–40] | <0.001 |
Platelets (k/µL) | 211 [163–267] | 210 [158–278] | 0.71 |
RDW (%) | 14.7 [13.80–16.0] | 15.0 [13.9–16.5] | <0.001 |
WBC (k/µL) | 8.1 [6.3–10.9] | 8.4 [6.3–11.3] | 0.06 |
RBC (m/µL) | 3.78 [3.29–4.26] | 3.66 [3.18–4.16] | <0.001 |
Hemoglobin (g/dL) | 11.1 [9.6–12.6] | 10.8 [9.3–12.3] | <0.001 |
Anion gap (mEq/L) | 15 [13–17] | 15 [13–17] | 0.94 |
Bicarbonate (mEq/L) | 26 [23–28] | 25 [23–28] | 0.003 |
PT (s) | 13.9 [12.3–18.9] | 14.0 [12.4–18.2] | 0.34 |
Sex | 0.40 | ||
Male | 2,326 (52.780) | 2,019 (51.862) | |
Female | 2,081 (47.220) | 1,874 (48.138) | |
Race | <0.001 | ||
White | 3,076 (72.788) | 2,938 (77.255) | |
Other | 1,150 (27.212) | 865 (22.745) | |
Marital status | 0.17 | ||
Single | 1,038 (24.011) | 906 (23.594) | |
Married | 2,009 (46.472) | 1,743 (45.391) | |
Divorce | 338 (7.819) | 280 (7.292) | |
Widowed | 938 (21.698) | 911 (23.724) | |
Echocardiography | <0.001 | ||
Yes | 1,595 (36.192) | 1,989 (51.092) | |
No | 2,812 (63.808) | 1,904 (48.908) | |
Alcohol use | <0.001 | ||
Yes | 3,915 (88.836) | 3,551 (91.215) | |
No | 492 (11.164) | 342 (8.785) | |
Tobacco use | <0.001 | ||
Yes | 2,588 (58.725) | 2,492 (64.012) | |
No | 1,819 (41.275) | 1,401 (35.988) | |
Myocardial infarction | 0.01 | ||
Yes | 3,553 (80.622) | 3,051 (78.371) | |
No | 854 (19.378) | 842 (21.629) | |
Diabetes | 0.27 | ||
Yes | 2,405 (54.572) | 2,172 (55.792) | |
No | 2,002 (45.428) | 1,721 (44.208) | |
Stroke | <0.001 | ||
Yes | 4,143 (94.010) | 3,585 (92.088) | |
No | 264 (5.990) | 308 (7.912) | |
Dyslipidemia | 0.67 | ||
Yes | 3 (0.519) | 2 (0.355) | |
No | 575 (99.481) | 562 (99.645) | |
Hypertension | 0.006 | ||
Yes | 2,726 (61.856) | 2,522 (64.783) | |
No | 1,681 (38.144) | 1,371 (35.217) | |
Atrial fibrillation | 0.002 | ||
Yes | 2,514 (57.046) | 2,091 (53.712) | |
No | 1,893 (42.954) | 1,802 (46.288) | |
Renal disease | 0.01 | ||
Yes | 2,695 (61.153) | 2,277 (58.490) | |
No | 1,712 (38.847) | 1,616 (41.510) | |
Peripheral vascular disease | <0.001 | ||
Yes | 3,814 (86.544) | 3,240 (83.226) | |
No | 593 (13.456) | 653 (16.774) | |
Chronic pulmonary disease | 0.02 | ||
Yes | 2,748 (62.355) | 2,526 (64.886) | |
No | 1,659 (37.645) | 1,367 (35.114) | |
Pneumonia | 0.01 | ||
Yes | 3,809 (86.431) | 3,287 (84.434) | |
No | 598 (13.569) | 606 (15.566) | |
Asthma | <0.001 | ||
Yes | 3,902 (88.541) | 3,550 (91.189) | |
No | 505 (11.459) | 343 (8.811) | |
Aspirin | 0.20 | ||
Yes | 1,371 (31.110) | 1,160 (29.797) | |
No | 3,036 (68.890) | 2,733 (70.203) | |
Warfarin | 0.63 | ||
Yes | 3,010 (68.300) | 2,678 (68.790) | |
No | 1,397 (31.700) | 1,215 (31.210) | |
Metoprolol | 0.002 | ||
Yes | 1,572 (35.671) | 1,262 (32.417) | |
No | 2,835 (64.329) | 2,631 (67.583) | |
Atenolol | 0.047 | ||
Yes | 4,136 (93.851) | 3,693 (94.863) | |
No | 271 (6.149) | 200 (5.137) | |
Furosemide | <0.001 | ||
Yes | 1,109 (25.165) | 853 (21.911) | |
No | 3,298 (74.835) | 3,040 (78.089) |
Continuous data are expressed as median and quartile. The categorical data are expressed as n (%). There were 271 data missing from race, 137 data missing from marital status and 7,158 data missing from dyslipidemia. ALT, aminotransferase; AST, aspartate aminotransferase; BMI, body mass index; CCI, Charlson Comorbidity Index; eGFR, estimated glomerular filtration rate; PT, prothrombin time; RBC, red blood cell count; RDW, red cell distribution width; WBC, white cell count.
Influencing factors of 90-day readmission of patients with HF
Indicators with differences in baseline information were included in the LASSO regression analysis to screen variables. When the standard error coefficient of minimum distance λ=0.012, the optimal LASSO model was established which included 12 influence factors: age, CCI, blood urea nitrogen, RDW, RBC, bicarbonate, race, echocardiography, stroke, chronic pulmonary disease, asthma, furosemide (Figure 1). We further carried out multivariate logistic regression on 12 influencing factors. The results of multivariate logistic regression (Table 2) suggested that age, CCI, blood urea nitrogen, RDW, RBC, other race, echocardiography, stroke, chronic pulmonary disease, and furosemide were independently related to 90-day readmission of patients with HF.
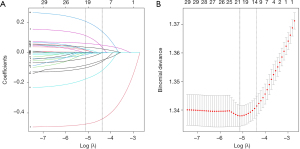
Table 2
Predictor | OR (95% CI) | P |
---|---|---|
Intercept | 0.360 (0.199–0.649) | 0.001 |
Age (years) | 1.005 (1.001–1.009) | 0.02 |
CCI (scores) | 1.052 (1.028–1.077) | <0.001 |
Blood urea nitrogen (mg/dL) | 0.998 (0.995–1.000) | 0.04 |
RDW (%) | 1.074 (1.050–1.098) | <0.001 |
RBC (m/µL) | 0.898 (0.840–0.960) | 0.001 |
Bicarbonate (mEq/L) | 0.996 (0.985–1.006) | 0.41 |
Other race | 0.885 (0.794–0.986) | 0.03 |
Echocardiography | 0.541 (0.494–0.593) | <0.001 |
Stroke | 1.325 (1.102–1.594) | 0.003 |
Chronic pulmonary disease | 0.864 (0.775–0.962) | 0.008 |
Asthma | 0.899 (0.759–1.064) | 0.21 |
Furosemide | 1.231 (1.106–1.371) | <0.001 |
CCI, Charlson Comorbidity Index; CI, confidence interval; HF, heart failure; OR, odds ratio; RBC, red blood cell count; RDW, red cell distribution width.
Correlation between influencing factors and 90-day readmission of patients with HF
Three different machine learning methods (logistic classification, XGBoost regression, and random forest) were used to rank the influencing factors (Table 3). The Wayne diagram (data not shown) intersected the top five influencing factors among the three machine learning methods and found that there were four common influencing factors—CCI, RBC, RDW, and age. As can be seen from Table 4 of the trend regression analysis, with the increase of CCI, the risk of 90-day readmission patients with HF increased, and the same results were observed in terms of RDW and age (CCI: P for trend <0.001, RDW: P for trend <0.001, age: P for trend =0.001). Especially, when CCI ≥8 (P=0.003), RDW ≥14.9% (P=0.04), and age ≥84 years (P=0.004), the risk of 90-day readmission was significantly increased. At the same time, the RBC value was negatively associated with the risk of 90-day readmission (P for trend <0.001), and RBC ≥3.73 m/µL can significantly decrease the risk of 90-day readmission (P=0.003).
Table 3
Variable | Weight importance | ||
---|---|---|---|
Logistic classification | XGBoost regression | Random forest | |
Echocardiography | 0.352 | 63 | 0.027 |
RDW (%) | 0.051 | 776 | 0.2 |
CCI (scores) | 0.029 | 352 | 0.103 |
RBC (m/µL) | 0.017 | 930 | 0.226 |
Age (years) | 0.007 | 705 | 0.171 |
Blood urea nitrogen (mg/dL) | 0 | 749 | 0.193 |
Race | 0 | 85 | 0.02 |
Stroke | 0 | 25 | 0.012 |
Chronic pulmonary disease | 0 | 88 | 0.026 |
Furosemide | 0 | 81 | 0.02 |
CCI, Charlson Comorbidity Index; RBC, red blood cell count; RDW, red cell distribution width.
Table 4
Variable | OR (95% CI) | P |
---|---|---|
CCI (scores) | ||
1–5 | Reference | |
6–7 | 1.123 (0.993–1.269) | 0.07 |
8 | 1.272 (1.086–1.491) | 0.003 |
9–18 | 1.411 (1.219–1.635) | <0.001 |
P for trend | 1.123 (1.071–1.176) | <0.001 |
RBC (m/µL) | ||
1.40–3.23 | Reference | |
3.24–3.72 | 0.922 (0.811–1.048) | 0.21 |
3.73–4.22 | 0.820 (0.719–0.935) | 0.003 |
4.23–6.85 | 0.786 (0.688–0.899) | <0.001 |
P for trend | 0.920 (0.881–0.960) | <0.001 |
RDW (%) | ||
11.5–13.9 | Reference | |
14.0–14.8 | 1.008 (0.886–1.145) | 0.91 |
14.9–16.3 | 1.143 (1.009–1.296) | 0.04 |
16.4–29.5 | 1.387 (1.219–1.578) | <0.001 |
P for trend | 1.114 (1.069–1.161) | <0.001 |
Age (years) | ||
18–63 | Reference | |
64–74 | 0.906 (0.795–1.033) | 0.14 |
75–83 | 0.995 (0.866–1.143) | 0.94 |
84–91 | 1.238 (1.071–1.430) | 0.004 |
P for trend | 1.081 (1.032–1.131) | 0.001 |
In this analysis, significant influencing factors other than the variables studied were adjusted. CCI, Charlson Comorbidity Index; CI, confidence interval; OR, odds ratio; RBC, red blood cell count; RDW, red cell distribution width.
The RCS analysis (Figure 2) showed that after adjusting for confounding factors, CCI, RBC, RDW, and age were all correlated with the 90-day readmission of patients with HF, among which RBC and RDW were linearly correlated with the 90-day readmission of patients with HF, while CCI and age were nonlinearly correlated with 90-day readmission of patients with HF. In addition, RCS analysis also indicated 4 key turning points that affected the association between the 4 factors and readmission risk, finding that the turning points were similar to that of trend regression analysis. These results highlighted the stability of our findings.
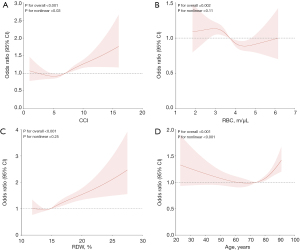
Discussion
HF has become the fastest-growing cardiovascular disease in the world, characterized by high mortality and hospitalization rates due to global population growth and aging. Predicting the risk of hospitalization within 90 days in patients with HF can help to manage the disease, prolong the survival time, and improve the quality of life of patients. In this study, we identified CCI, RBC, RDW, and age as the main factors influencing the 90-day readmission of patients with HF by screening predictors with various machine learning models. RBC and RDW were linearly correlated with the 90-day readmission of patients with HF, while CCI and age were nonlinearly correlated with the 90-day readmission of patients with HF.
In our study, the 90-day readmission rate in HF patients was found to be 46.90%. A study in China found readmission rates of 16.79% at 90 days and 40.12% at 180 days after discharge in patients with chronic HF (9). The 90-day readmission rate of HF patients in Korea was 25.8% (10). The 90-day readmission rate of patients with HF in the United States was 31.2% (11). The 90-day readmission rate of HF varied greatly in different countries, which may be related to the survey population and medical level. In our study, we found that age was nonlinearly correlated with the 90-day readmission of patients with HF. The inflection points in this study mainly appeared in the age groups of 60–70 and 70–80 years. After the second inflection point, the risk of the 90-day readmission of patients with HF was positively correlated with age. With the increase of age, patients’ physical function declines, and the rate of heart function deterioration may accelerate, which will lead to an increase in the risk of readmission. This study suggested that more attention should be paid to the prognosis of elderly HF patients over 80 years old.
CCI is an indicator that assesses the severity of the disease. We found a nonlinear relationship between CCI and 90-day readmission of patients with HF. Some scholars have found similar results. A study of 2008 HF patients by Chinese scholars found a curvilinear relationship between CCI and short-term readmissions in HF patients, with a significant increase in short-term readmissions in HF patients with a high CCI (CCI >2.97) (12). Another large retrospective study in China also found a nonlinear relationship between CCI and readmission of patients with HF within 6 months, and the inflection point of CCI was 1 (13). CCI is widely used to predict patient prognosis. CCI predicted in-hospital death in patients with acute respiratory failure (14). High CCI indicated that the overall survival time of esophageal cancer patients was short (15).
RBC refers to the number of red blood cells contained in a unit volume of blood. Few studies have found a correlation between RBC and HF. It was found that RBC was a risk factor for the 1- and 3-year comprehensive results of patients who received primary prevention cardiac resynchronization therapy-defibrillator for HF (16). RDW is an index to measure the size variability of red blood cells in peripheral blood. In our study, we found that RDW is a risk factor for the 90-day readmission of patients with HF. It was found that RDW was related to various prognoses of HF. It is found that RDW is an independent predictor of cardiovascular events in 90 days of acute HF (17). A study based on patients with HF in China found that RDW was a risk factor for readmission within 90 days, and the combination of N-terminal pro-B-type natriuretic peptide and CCI had good predictive performance (18). A study based on the MIMIC-III database found that RDW had a nonlinear relationship with 30-day, 90-day, 1-year, and 4-year all-cause mortality in patients with congestive HF (19). Non-invasive and repeatable indexes such as RBC and RDW for disease assessment and prognosis prediction have become hot spots in the field of clinical marker research and are widely used. In future research, more attention should be paid to the potential significance of the change of each parameter level for the diagnosis and treatment of diseases.
There are some limitations in this study. First, although the study was conducted based on a large sample of data bureaus and included a large number of patients, it was a single-center retrospective study, which inevitably suffers from bias. Second, this study lacked some data on biomarkers of HF, such as C-reactive protein.
Conclusions
CCI, RBC, RDW, and age were the main factors influencing the 90-day readmission of patients with HF. RBC and RDW were linearly correlated with the 90-day readmission of patients with HF, while CCI and age were nonlinearly correlated with the 90-day readmission of patients with HF.
Acknowledgments
None.
Footnote
Reporting Checklist: The authors have completed the TRIPOD reporting checklist. Available at https://jtd.amegroups.com/article/view/10.21037/jtd-24-1738/rc
Peer Review File: Available at https://jtd.amegroups.com/article/view/10.21037/jtd-24-1738/prf
Funding: None.
Conflicts of Interest: All authors have completed the ICMJE uniform disclosure form (available at https://jtd.amegroups.com/article/view/10.21037/jtd-24-1738/coif). The authors have no conflicts of interest to declare.
Ethical Statement: The authors are accountable for all aspects of the work in ensuring that questions related to the accuracy or integrity of any part of the work are appropriately investigated and resolved. The study was conducted in accordance with the Declaration of Helsinki (as revised in 2013).
Open Access Statement: This is an Open Access article distributed in accordance with the Creative Commons Attribution-NonCommercial-NoDerivs 4.0 International License (CC BY-NC-ND 4.0), which permits the non-commercial replication and distribution of the article with the strict proviso that no changes or edits are made and the original work is properly cited (including links to both the formal publication through the relevant DOI and the license). See: https://creativecommons.org/licenses/by-nc-nd/4.0/.
References
- National Centre for Cardiovascular Disease CCHaDRWG. Summary of China Cardiovascular Health and Disease Report 2023. China Circulation Magazine 2024;39:625-60.
- Li M, Gao X, Wang H, et al. Phosphoglycerate mutase 2 is elevated in serum of patients with heart failure and correlates with the disease severity and patient's prognosis. Open Med (Wars) 2021;16:1134-42. [Crossref] [PubMed]
- Cardiovascular Disease Branch of Chinese Medical Association CPBoCPA, Heart Failure Committee of Chinese Physicians Association, et al. Chinese Guidelines for the Diagnosis and Treatment of Heart Failure 2024. Chinese Journal of Cardiovascular Disease 2024;52:235-75.
- Savarese G, Becher PM, Lund LH, et al. Global burden of heart failure: a comprehensive and updated review of epidemiology. Cardiovasc Res 2023;118:3272-87. [Crossref] [PubMed]
- Jain V, Minhas AMK, Khan SU, et al. Trends in HF Hospitalizations Among Young Adults in the United States From 2004 to 2018. JACC Heart Fail 2022;10:350-62. [Crossref] [PubMed]
- Amdani S, Lopez R, Schold JD, et al. 30- and 60-Day Readmission Rates for Children With Heart Failure in the United States. JACC Heart Fail 2024;12:83-96. [Crossref] [PubMed]
- Soetjoadi H, Friska D, Siswanto BB, et al. Impact of Estimated Plasma Volume Status on Mortality in Right Heart Failure Patients: A Retrospective Cohort Study in Indonesia. Glob Heart 2022;17:60. [Crossref] [PubMed]
- Kito K, Mori Y, Watanabe D, et al. Relationship between instrumental activities of daily living decline during hospitalization and one-year mortality in elderly patients with heart failure: A multi-center prospective cohort study. Arch Gerontol Geriatr 2023;110:104985. [Crossref] [PubMed]
- Jiahuan Li LW. Jing Qu Survey on readmission of patients with chronic heart failure and analysis of factors influencing it. South China Preventive Medicine 2024;50:323-8.
- Son YJ, Shim DK, Seo EK, et al. Gender differences in the impact of frailty on 90-day hospital readmission in heart failure patients: a retrospective cohort study. Eur J Cardiovasc Nurs 2021;20:485-92. [Crossref] [PubMed]
- Khan MS, Sreenivasan J, Lateef N, et al. Trends in 30- and 90-Day Readmission Rates for Heart Failure. Circ Heart Fail 2021;14:e008335. [Crossref] [PubMed]
- Wei D, Sun Y, Chen R, et al. The Charlson comorbidity index and short-term readmission in patients with heart failure: A retrospective cohort study. Medicine (Baltimore) 2023;102:e32953. [Crossref] [PubMed]
- Sheng S, Xu FQ, Zhang YH, et al. Charlson Comorbidity Index is correlated with all-cause readmission within six months in patients with heart failure: a retrospective cohort study in China. BMC Cardiovasc Disord 2023;23:111. [Crossref] [PubMed]
- Setter NW, Peres ML, de Almeida BMM, et al. Charlson comorbidity index scores and in-hospital prognosis of patients with severe acute respiratory infections. Intern Med J 2020;50:691-7. [Crossref] [PubMed]
- Kubo Y, Tanaka K, Yamasaki M, et al. Influences of the Charlson Comorbidity Index and Nutrition Status on Prognosis After Esophageal Cancer Surgery. Ann Surg Oncol 2021;28:7173-82. [Crossref] [PubMed]
- Nauffal V, Tanawuttiwat T, Zhang Y, et al. Predictors of mortality, LVAD implant, or heart transplant in primary prevention cardiac resynchronization therapy recipients: The HF-CRT score. Heart Rhythm 2015;12:2387-94. [Crossref] [PubMed]
- He W, Jia J, Chen J, et al. Comparison of prognostic value of red cell distribution width and NT-proBNP for short-term clinical outcomes in acute heart failure patients. Int Heart J 2014;55:58-64. [Crossref] [PubMed]
- Tan BY, Gu JY, Wei HY, et al. Electronic medical record-based model to predict the risk of 90-day readmission for patients with heart failure. BMC Med Inform Decis Mak 2019;19:193. [Crossref] [PubMed]
- Ji X, Ke W. Red blood cell distribution width and all-cause mortality in congestive heart failure patients: a retrospective cohort study based on the Mimic-III database. Front Cardiovasc Med 2023;10:1126718. [Crossref] [PubMed]