Asthma-derived genetic signature predicts lung cancer prognosis: a multi-scale omics investigation
Highlight box
Key findings
• Global Burden of Disease data revealed positive correlation between asthma and lung cancer incidence, while Mendelian randomization suggested a protective effect of asthma against lung cancer.
• A prognostic model incorporating four asthma-related genes (CD69, KIF18A, HN1L, and KLF10) effectively stratified lung cancer patients and correlated with immune features.
What is known and what is new?
• Epidemiological studies have shown inconsistent results regarding the relationship between asthma and lung cancer risk.
• This study revealed complex asthma-lung cancer relationship through multi-omics analysis, developed novel prognostic model integrating disease features and immune characteristics.
What is the implication, and what should change now?
• The asthma-related gene signature could serve as a new tool for lung cancer prognosis and help identify candidates for immunotherapy, suggesting need for molecular profile-based personalized strategies.
Introduction
Pulmonary malignancies represent a leading cause of cancer-related mortality worldwide (1), significantly impacting life expectancy (2). Despite recent advancements in targeted and immune-based therapies, the overall prognosis for lung cancer patients remains poor, with survival rates failing to show substantial improvement (3). Therefore, it is crucial to delve into the pathogenesis of lung cancer and identify effective prognostic markers to improve patient outcomes.
Recent scientific investigations have focused on the potential link between chronic respiratory inflammation and lung malignancies. Asthma, a widespread inflammatory airway condition affecting millions worldwide, has become a key subject in this research landscape (4). The scientific community proposes two contrasting theories to elucidate the complex interplay between asthma and pulmonary malignancies. One perspective posits that chronic airway inflammation may compromise lung integrity, potentially elevating cancer risk (1,5,6). Conversely, an alternative hypothesis suggests that the heightened immune activity characteristic of asthma might enhance the body’s capacity to detect and eliminate neoplastic cells (7-9). These divergent viewpoints underscore the nuanced nature of the asthma-lung cancer relationship, highlighting the need for further investigation.
Systematic reviews have shown that asthma (but not non-asthmatic atopy) is associated with an increased risk of lung cancer, indicating that asthma itself may possess unique physiological or immunological characteristics that could be related to the development of lung cancer (10-12). Several cohort studies based on different populations further support this notion, demonstrating that asthma patients have a higher risk of developing lung cancer compared to non-asthmatic individuals, and this association is prevalent across different genders and races/ethnicities (13,14). Moreover, research has found that the level of asthma control also influences the risk of lung cancer, with poorly controlled asthma patients having a higher risk (15).
Although most studies support a positive correlation between asthma and an increased risk of lung cancer, a few studies have yielded contradictory conclusions (8,16). This suggests that the relationship between asthma and lung cancer may be influenced by other factors, such as smoking status and asthma-chronic obstructive pulmonary disease overlap (ACO) (17,18). Thus, further studies are needed to clarify the causal relationship and potential mechanisms.
Based on the available evidence, this study aims to thoroughly investigate the relationship between asthma and lung cancer through the following approaches: (I) analyzing the epidemiological association between the two using the Global Burden of Disease (GBD) database; (II) assessing the causal relationship between them using Mendelian randomization (MR); (III) constructing a lung cancer prognostic model based on the established relationship and transcriptomic data, and validating the effectiveness of the prognostic model through a series of bioinformatic methods, including bulk RNA and single-cell RNA sequencing (scRNA-seq) data analysis. Epidemiological and MR analyses provide population-level associations and causal evidence, while transcriptomic gene analysis explores shared molecular mechanisms and validates these findings at the molecular level, offering deeper insights into the biological basis of the asthma-lung cancer relationship. By integrating evidence from epidemiology, genetics, and transcriptomics, this study is expected to provide new insights into the relationship between asthma and lung cancer, offering valuable information for the prevention and treatment of lung cancer. We present this article in accordance with the STROBE-MR reporting checklist (available at https://jtd.amegroups.com/article/view/10.21037/jtd-24-1697/rc).
Methods
Source data acquisition
The incidence data for asthma and lung cancer used in this study were obtained from the 2019 GBD study database. As there was no separate data for lung cancer in the database, we used the incidence data for “Tracheal, bronchus, and lung cancer” as a substitute. The GBD 2019 study covered 204 countries and territories and assessed the health loss caused by hundreds of diseases, injuries, and risk factors from 1990 to 2019. The database is managed and publicly released by the Institute for Health Metrics and Evaluation located in Seattle, USA. Researchers can query and download relevant data online through the GBD Results tool visualization platform (http://ghdx.healthdata.org/gbd-results-tool).
To explore the potential causal link between asthma and pulmonary malignancies, we employed MR methodology. Our analysis utilized genome-wide association study (GWAS) data sourced from the European Bioinformatics Institute’s GWAS Catalog (https://www.ebi.ac.uk/gwas/). The asthma dataset (GCST90014325) encompassed over 400,000 participants, including 56,167 affected individuals. For lung cancer, we referenced the GCST004748 dataset, which comprised 85,716 subjects, with 29,266 diagnosed cases. This MR approach aimed to elucidate the nature of the relationship between these two respiratory conditions.
The bulk RNA data for asthma were obtained from the GSE165934 dataset in the Gene Expression Omnibus (GEO) database (https://www.ncbi.nlm.nih.gov/geo/), which included peripheral blood mononuclear cell (PBMC) samples from 9 healthy controls and 10 bronchial asthma patients. PBMC samples were selected as they represent systemic immune responses and are commonly used to study inflammatory and immunological mechanisms associated with asthma. Our study leveraged lung cancer transcriptome data from The Cancer Genome Atlas (TCGA) (https://www.cancer.gov/ccg/research/genome-sequencing/tcga), a comprehensive multi-omics initiative. We accessed RNA sequencing profiles via the UCSC Xena platform (https://xenabrowser.net/datapages/), focusing on cases with complete survival information. The final dataset encompassed 1,016 tumor samples and 59 adjacent normal tissues, offering a rich resource for exploring the molecular intricacies of pulmonary malignancies. To improve the reliability of our findings, we used a validation dataset to verify the prognostic model we constructed. Specifically, the validation dataset was obtained from GSE30219 in the GEO database, which included 293 lung cancer tissue samples and 14 adjacent normal tissue samples. To investigate the tumor cell heterogeneity in lung cancer tissues, we performed single-cell sequencing analysis using data from GSE198099 in the GEO database, which included single-cell sequencing data from 2 tumor tissues and 2 adjacent normal tissues. The study was conducted in accordance with the Declaration of Helsinki (as revised in 2013).
GBD data processing and analysis
In this study, we obtained incidence data for asthma and tracheal, bronchus, and lung cancer from 1990 to 2019 across various countries and regions from the GBD 2019 study. The GBD database provides estimates stratified by sex (male and female) and across 23 distinct age groups, ranging from early infancy (0–6 days) to older adults (95+ years). Although the dataset does not explicitly differentiate between childhood-onset and adult-onset asthma, we assumed that the majority of cases in this analysis represent adult-onset asthma, given the inclusion of age-standardized data and the higher incidence of asthma in older populations. Age-standardized rates per 100,000 population were computed by the direct method to the GBD population standard, ensuring comparability across populations with different age structures.
We chose to use age-standardized incidence rates (ASR) because they directly reflect disease risk, are more sensitive to changes in risk factors, and allow for unbiased comparisons across populations and over time by controlling for demographic differences. ASR is particularly suitable for investigating associations at a global scale, as it minimizes the impact of age and population structure variations, facilitating cross-regional and temporal comparisons.
To investigate the relationship between asthma and lung cancer, we conducted a Spearman correlation analysis using ASR. This approach allows us to assess the strength and direction of the association between the two diseases while controlling for potential confounding effects of age and population structure differences across countries and regions. However, it is important to note that physiological factors such as body mass index (BMI) or smoking history, which may influence the relationship between asthma and lung cancer, were not included in this dataset and represent a limitation of our study.
MR-based causal estimation
We conducted a MR analysis to examine the potential causal link between asthma and lung cancer susceptibility. The TwoSampleMR package (version 0.5.7) facilitated our investigation. In selecting instrumental variables (IVs), we applied a stringent significance threshold (P<5e−08) to identify robust genetic markers. To ensure independence among these IVs, we implemented linkage disequilibrium clumping with an r2 threshold of 0.01. The final step involved harmonizing the genetic data across asthma and lung cancer datasets, aligning effect alleles while excluding palindromic single nucleotide polymorphisms (SNPs).
For each IVs, we calculated the F-statistic using the formula F = R2/(1–R2) * (n − k − 1)/k, where R2 = 2 * MAF * (1-MAF) * Beta2, n is the sample size, k is the number of IVs, and MAF is the minor allele frequency. IVs with an F-statistic less than 10 were excluded to mitigate weak instrument bias.
The MR analysis was conducted based on the following key assumptions:
- Relevance assumption: the chosen genetic variants (IVs) exhibit a robust and significant association with the exposure variable (asthma).
- Independence assumption: the genetic variants (IVs) do not correlate with any confounding variables influencing the exposure-outcome relationship.
- Exclusion restriction assumption: the genetic variants (IVs) influence the outcome (lung cancer risk) solely through their impact on the exposure (asthma), without alternative pathways.
Multiple MR methods were employed to assess the potential causal relationship between asthma and lung cancer, including inverse-variance weighted (IVW), weighted median, weighted mode, MR-Egger regression, and simple mode. The primary analysis method was IVW, with a P value threshold of <0.05 considered statistically significant. Given our stringent criteria for IV selection (P<5e−08) and the exclusion of SNPs linked to lung cancer or related phenotypes, we opted against applying multiple testing corrections.
To evaluate the robustness of the analysis results, we performed sensitivity analyses. First, we tested for heterogeneity among the genetic IVs using Cochran’s Q statistic. A P value <0.05 indicated significant heterogeneity. Additionally, we assessed horizontal pleiotropy of the genetic IVs using the intercept term of the MR-Egger regression, with a P value <0.05 suggesting significant pleiotropy.
Bulk RNA-seq data: integrated processing and analytical workflow
In this study, we used limma (version 3.56.2) for differential expression analysis of asthma bulk RNA sequencing data, and DESeq2 (version 1.40.2) for lung cancer bulk RNA sequencing data. The choice of different packages was based on their suitability for the specific data types and their strengths in handling continuous (limma) and discrete count (DESeq2) data. We focused on genes with a Benjamini-Hochberg-adjusted P value <0.01 and a log2 fold change >1. Due to the high number of genes analyzed concurrently, we implemented a stringent adjusted P value cutoff of 0.01 to mitigate false positive results. We focused on genes demonstrating robust evidence of differential expression. This approach ensures that the identified genes are highly likely to be biologically significant and minimizes the risk of pursuing false leads. After identifying the differentially expressed genes (DEGs) in asthma and lung cancer, we took the intersection of these gene sets to determine the candidate genes of interest. This step allowed us to focus on genes that were consistently differentially expressed in both conditions, increasing the likelihood of their involvement in the underlying biological processes connecting asthma and lung cancer. Finally, we performed Spearman correlation analysis to assess the relationships between the candidate genes. By employing this approach, we aimed to uncover potential co-expression patterns and functional relationships among the identified genes, which could provide valuable insights into the shared molecular mechanisms between asthma and lung cancer.
Biological pathways and protein-protein interaction (PPI) network
To elucidate the biological roles and pathways linked to our candidate gene set, we conducted Gene Ontology (GO) and Kyoto Encyclopedia of Genes and Genomes (KEGG) enrichment analyses. These were performed using R packages clusterProfiler (v4.8.2) and org.Hs.eg.db (v3.17.0). For GO analysis, background gene sets were derived from org.Hs.eg.db, while KEGG analysis utilized sets from the KEGG database. We employed the Benjamini-Hochberg method to adjust for multiple comparisons, considering an adjusted P value <0.05 as the threshold for statistical significance. To examine the interrelationships among candidate genes, we developed a PPI network using STRING (https://string-db.org/). This approach enhanced our understanding of gene interactions and their functional implications.
Development and validation of the related prognostic model
We constructed a prognostic model using the candidate genes identified from the intersection of DEGs in asthma and lung cancer. First, we performed least absolute shrinkage and selection operator (LASSO)-penalized Cox regression analysis to select the most informative genes for predicting overall survival (OS). The optimal penalty parameter (λ) was determined through 10-fold cross-validation. The genes with non-zero coefficients at the optimal λ were considered as the final prognostic genes. Next, we fitted a multivariate Cox proportional hazards model using the selected prognostic genes. The risk score for each patient was calculated as the sum of the product of the expression level of each gene and its corresponding coefficient from the Cox model. Patients were then divided into high-risk and low-risk groups based on the median risk score. After constructing the prognostic model, we employed various methods to evaluate its performance and clinical utility. Kaplan-Meier survival curves were plotted to compare the OS between the high-risk and low-risk groups, and the significance of the difference was assessed using the log-rank test. We also generated risk curves to visualize the distribution of risk scores and the survival status of patients in the training cohort. To further assess the independence of the prognostic model, we performed multivariate Cox regression analysis by incorporating the risk score and clinical variables such as age and tumor stage. Moreover, we constructed a nomogram based on the multivariate Cox analysis results to predict the 1-, 3-, and 5-year OS probabilities. The nomogram is a graphical tool that integrates multiple prognostic factors to provide personalized survival predictions. Calibration curves were plotted to assess the consistency between the nomogram-predicted probabilities and the observed probabilities, indicating the reliability of the nomogram.
To enhance the robustness of our findings, we validated the prognostic model using an independent dataset, GSE30219, which included 293 lung cancer tissues and 14 adjacent normal tissues. The risk scores for patients in the validation cohort were calculated using the same formula derived from the training cohort. Patients were then divided into low-risk and high-risk groups based on the median risk score. Kaplan-Meier survival analysis were performed to evaluate the predictive value of the model in the validation cohort.
Immune infiltration analysis and evaluation of ESTIMATEscore and immune checkpoint gene
To investigate the relationship between the risk score and the tumor immune microenvironment, we employed the CIBERSORT algorithm to quantify the proportions of immune cells based on gene expression data. The ESTIMATE algorithm was used to calculate the stromal and immune scores, predicting tumor purity based on the infiltration of stromal and immune cells (19). Spearman correlation analysis was performed to assess the associations between the risk score and immune cell infiltration, ESTIMATE scores, and immune checkpoint gene expression. The Wilcoxon rank-sum test was used to compare the differences in immune cell infiltration between the high-risk and low-risk groups. A P value less than 0.05 was considered statistically significant. Our approach provides a comprehensive evaluation of the relationship between the risk score and the tumor immune microenvironment, highlighting potential immunological mechanisms underlying the differences in survival outcomes between risk groups.
Gene set enrichment analysis (GESA) was conducted between high- and low-risk groups
We performed GSEA to identify differentially enriched pathways between the high-risk and low-risk groups. The Benjamini-Hochberg method was used for multiple testing correction, and pathways with an adjusted P value <0.05 were considered statistically significant. This approach aims to uncover the key biological pathways differentially regulated between the risk groups, providing insights into the underlying molecular mechanisms driving the differences in prognosis.
Comprehensive scRNA-seq workflow
For our single-cell sequencing analysis, we utilized Seurat (v4.3.0.1) to preprocess data and implement quality control measures. Our criteria stipulated that each gene must be detected in at least 3 cells, while each cell should express no fewer than 300 genes. We also excluded cells with over 10% mitochondrial gene content or above 3% hemoglobin gene content. Post-quality control, we normalized the data and identified the top 2,000 variable genes for further examination. Dimensionality reduction and clustering were achieved through principal component analysis. We then applied Seurat’s FindClusters function, which leverages a shared nearest neighbor modularity optimization-based algorithm for clustering. With the resolution parameter set at 0.5, we identified 22 distinct clusters. These were subsequently visualized using uniform manifold approximation and projection (UMAP).
We evaluated gene overexpression in specific cell types through differential expression analysis, employing the Wilcoxon rank-sum test. This approach compared gene expression levels between individual cell types and all others collectively. Cell type-specific enrichment was characterized by significant gene overexpression in one cell type relative to the remaining cell population. To address multiple testing concerns, we applied the Bonferroni correction to the Wilcoxon rank-sum test P values. Genes were deemed enriched in a particular cell type when exhibiting a log2 fold change exceeding 0.5 and an adjusted P value below 0.05.
We initiated cell type annotation using the SingleR package (v2.2.0), leveraging the Human Primary Cell Atlas as our reference database. This preliminary classification was subsequently refined by analyzing the expression patterns of established cell type-specific marker genes within each cluster. Clusters exhibiting comparable marker gene profiles were consolidated, ultimately yielding 13 distinct cell types. To ensure precise characterization of cellular subpopulations, we manually fine-tuned and validated the final annotation based on marker gene expression patterns.
Monocle3-based cellular differentiation analysis
We explored the developmental trajectories of CD8+ T cells and Dendritic cells using pseudotime analysis, implemented through the Monocle3 R package (v1.3.3). Our analysis focused on a subset of the Seurat object, comprising only cells from relevant clusters, which we then transformed into a Monocle3 cell_data_set object. To infer pseudotime trajectories, we applied the learn_graph function. We visualized the resulting differentiation paths using UMAP plots, generated via the plot_cells function.
Inference and analysis of cell-cell communication
To investigate the cell-cell communication in the tumor microenvironment, we performed an analysis using the CellChat (version 1.6.1) R package. We then selected the “Secreted Signaling” subset of the CellChatDB.human database to focus on specific aspects of cell-cell interactions.
Statistical analysis
Statistical analyses were performed using R software (version 4.3.1). Spearman correlation analysis was used to assess relationships between variables. Differences between groups were compared using the Wilcoxon rank-sum test. Survival analysis was conducted using Kaplan-Meier method with log-rank test and Cox proportional hazards regression. For differential expression analysis, P<0.01 with Benjamini-Hochberg correction was considered significant. For other analyses, two-sided P<0.05 was considered statistically significant unless otherwise specified.
Results
Global incidence trends and correlation between asthma and lung cancer
The global incident cases of asthma slightly increased from 32.16 million [95% uncertainty interval (UI): 25.75–40.51 million] in 1990 to 36.98 million (95% UI: 29.60–45.93 million) in 2019, representing a 15.0% increase. However, the ASR decreased by 13.1%, from 580.09 (95% UI: 474.68–715.04) per 100,000 population in 1990 to 504.28 (95% UI: 400.64–633.26) per 100,000 population in 2019 (Figure 1A). Tracheal, bronchus, and lung cancer exhibited a similar trend in incidence. The total incident cases increased from 1.12 million (95% UI: 1.08–1.18 million) in 1990 to 2.26 million (95% UI: 2.07–2.45 million) in 2019, representing a 101.8% increase. However, the ASR decreased by 2.6%, from 28.39 (95% UI: 27.18–29.67) per 100,000 population in 1990 to 27.66 (95% UI: 25.28–29.99) per 100,000 population in 2019 (Figure 1B).
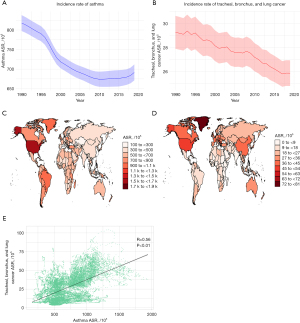
Moreover, the distribution of ASR for asthma and tracheal, bronchus, and lung cancer in 2019 was similar at the national level. Regions with high ASR of asthma also exhibited higher ASR of tracheal, bronchus, and lung cancer (Figure 1C,1D). We further conducted a correlation analysis between asthma and tracheal, bronchus, and lung cancer based on the ASR across various regions and time points. The results demonstrated a strong correlation between the ASR of asthma and tracheal, bronchus, and lung cancer (R=0.56, P<0.01; Figure 1E).
In summary, although the global incident cases of both asthma and tracheal, bronchus, and lung cancer increased from 1990 to 2019, the ASR decreased during the same period. The geographical distribution of these two diseases showed similarities, with regions having high ASR of asthma also exhibiting higher ASR of tracheal, bronchus, and lung cancer. This correlation suggests a potential relationship between the two conditions, although the observational nature of this analysis limits our ability to infer causality.
MR analysis reveals a potential causal relationship between asthma and lung cancer risk
To investigate the potential causal relationship between asthma and lung cancer risk, we performed a MR analysis using GWAS data of asthma as the exposure and GWAS data of lung cancer as the outcome. For each IVs, we calculated the F-statistic and excluded IVs with an F-statistic less than 10 to ensure the strength of the genetic instruments and avoid weak instrument bias (Table S1). The results of the MR analysis showed a potential causal relationship between asthma and lung cancer [IVW: OR: 0.89, 95% confidence interval (CI): 0.84–0.95, P=4.0088e−04; Figure 2A]. Interestingly, this finding contrasts with the positive association observed in the correlation analysis, suggesting that the relationship between asthma and lung cancer may be more complex than initially assumed.
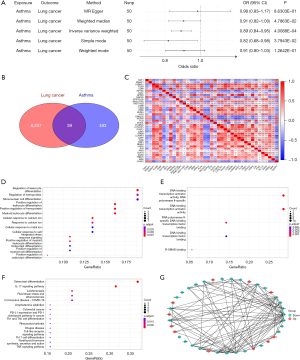
To assess the robustness of the analysis results, we first performed an MR-Egger regression to investigate horizontal pleiotropy. The results indicated that pleiotropy was unlikely to bias the causal relationship (P=0.23). Subsequently, we conducted Cochran’s Q test, which yielded P values greater than 0.05 (Q-value for IVW test: 47.63, P=0.53; Q-value for MR-Egger test: 46.18, P=0.55), suggesting an absence of heterogeneity among the SNPs.
In summary, our MR analysis, using GWAS data of asthma as the exposure and GWAS data of lung cancer as the outcome, revealed a potential causal relationship between asthma and lung cancer risk. The robustness of the results was supported by the MR-Egger regression, which ruled out significant horizontal pleiotropy, and Cochran’s Q test, which indicated no heterogeneity among the SNPs used as IVs. However, the inverse relationship identified through MR contrasts with the observational correlation analysis, indicating that the epidemiological association might not be driven by a direct causal pathway. These findings highlight the complexity of the asthma-lung cancer relationship and underscore the need for further studies that integrate demographic, environmental, and genetic data to disentangle these conflicting observations.
Identification of asthma-related DEG in lung cancer
Based on the epidemiological evidence of the association between asthma and lung cancer and the potential causal relationship revealed by MR, we sought to identify dysregulated genes in both diseases using bulk RNA sequencing. First, we analyzed the GSE165934 dataset, which included 10 patients with bronchial asthma and 9 healthy controls, and identified 532 DEGs (table available at https://cdn.amegroups.cn/static/public/jtd-24-1697-1.xlsx). Next, we performed differential expression analysis on the TCGA Lung Cancer cohort, excluding patients without survival data. We included 59 adjacent normal tissues and 1,016 tumor tissues in the study and identified 6,246 DEGs (table available at https://cdn.amegroups.cn/static/public/jtd-24-1697-2.xlsx). By comparing the DEGs from both analyses, we identified 39 genes that were differentially expressed in both asthma and lung cancer (Figure 2B). These genes represent potential molecular links between the two diseases and may play crucial roles in the development and progression of lung cancer in patients with asthma.
To further characterize the relationships among these 39 asthma-associated DEGs in lung cancer, we performed Spearman correlation analysis. The results revealed strong correlations among the genes (Figure 2C), suggesting that they may be involved in common biological pathways or regulatory networks. This finding highlights the importance of these genes in the context of lung cancer and their potential utility as biomarkers or therapeutic targets.
Functional characterization and PPI network
To investigate the potential functions of the 39 asthma-associated DEGs in lung cancer, we performed GO and KEGG enrichment analyses. The GO enrichment results revealed that these 39 genes were primarily enriched in biological processes (BP) such as leukocyte differentiation, regulation of hematopoiesis, response to calcium ion, and osteoclast differentiation (Figure 2D). The enrichment of these processes suggests that there may be critical regulatory mechanisms in lung cancer cells related to cell differentiation and ion response. The main enriched molecular functions (MF) involved DNA binding and transcriptional regulation, specifically including RNA polymerase II-specific DNA-binding transcription activator activity, general DNA-binding transcription activator activity, and binding to RNA polymerase II-specific DNA-binding transcription factors (Figure 2E). The enrichment of these functions indicates a significant enhancement of transcriptional regulatory activities. No statistically significant pathways were found in the cellular component (CC) category.
The KEGG enrichment analysis revealed significant enrichment of several key biological pathways, including osteoclast differentiation, IL-17 signaling pathway, atherosclerosis, coronavirus disease 2019 (COVID-19), and programmed death-ligand 1 (PD-L1) expression and programmed death-1 (PD-1) checkpoint pathway in cancer (Figure 2F). These results suggest the activation of these pathways and provide important information for understanding disease mechanisms and potential therapeutic targets.
Finally, we constructed a PPI network for the 39 asthma-associated DEGs in lung cancer. The results showed significant interactions among these 39 genes (Figure 2G).
Construction and validation of a prognostic model based on asthma-associated DEGs in lung cancer
To evaluate the effectiveness of a prognostic model based on the 39 asthma-associated DEGs in lung cancer, we employed the LASSO regression method for analysis. Ultimately, the four most valuable key genes (CD69, KIF18A, HN1L, and KLF10) were selected for model construction (Figure 3A). A multivariate Cox proportional hazards model was fitted using these four key genes. The risk score for each patient was calculated as the sum of the expression levels of each gene multiplied by their corresponding coefficients from the Cox model. Patients were then divided into high-risk and low-risk groups based on the median risk score. The high-risk score was associated with higher expression levels of KIF18A, HN1L, and KLF10, and lower expression of CD69 (Figure 3B). Compared to the low-risk group, the high-risk group had a significantly lower survival rate (Figure 3C). Furthermore, multivariate COX regression analysis revealed that the riskScore was an independent risk factor for lung cancer patients [hazard ratio (HR) 1.712, 95% CI: 1.198–2.447, P=0.003; Figure 3D].
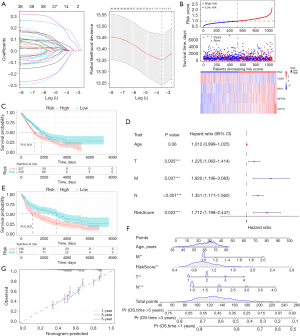
To validate the prognostic model, we applied it to the GSE30219 dataset (Figure 3E), which demonstrated the robust performance of the model constructed using TCGA data. Subsequently, we integrated the riskScore, age, and Tumor-Node-Metastasis (TNM) staging to construct a nomogram for lung cancer patients (Figure 3F). The nomogram exhibited good performance in predicting the 1-, 3-, and 5-year OS of lung cancer patients (Figure 3G).
In conclusion, we successfully constructed and validated a prognostic model based on four key asthma-associated DEGs in lung cancer. The model demonstrated strong predictive performance and identified the riskScore as an independent risk factor for lung cancer patients. The integration of the riskScore with clinical variables in a nomogram further enhanced the model’s predictive capabilities. These findings highlight the potential of utilizing asthma-associated genes in developing prognostic tools for lung cancer patients and may guide personalized treatment strategies.
Assessing the effectiveness of the prognostic model through immune cell correlation and checkpoint gene analysis
Spearman correlation analysis revealed associations between immune cells and the four key genes as well as the riskScore (Figure 4A). Notably, significant differences were observed between the high-risk and low-risk groups in terms of B cells memory, macrophages M0, macrophages M1, mast cells resting, mast cells activated, monocytes, neutrophils, natural killer (NK) cells resting, plasma cells, and T cells CD8 (Figure 4B). The ESTIMATEScore showed a negative correlation with the riskScore (R=−0.25, P<0.001; Figure 4C). Furthermore, the riskScore was significantly correlated with immune checkpoint genes (Table 1), indicating that the riskScore could serve as a prognostic marker for lung cancer patients.
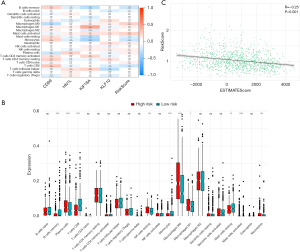
Table 1
Gene | riskScore Cor | P value |
---|---|---|
LAG3 | −0.1222 | 6.91E−05 |
CTLA-4 | −0.22008 | 4.88E−13 |
CD47 | −0.09446 | 0.002 |
TIM-3 | −0.20074 | 4.72E−11 |
TIGIT | −0.2194 | 5.77E−13 |
HAVCR2 | −0.20074 | 4.72E−11 |
DNAM-1 | −0.26281 | 3.99E−18 |
Cor, the value of Pearson’s correlation.
The significant differences in immune cell populations between the high-risk and low-risk groups suggest that the prognostic model effectively stratifies patients based on their immune profiles. The negative correlation between the ESTIMATEScore and the riskScore further supports the model’s ability to capture the immune landscape of lung cancer patients. The strong correlations between the riskScore and immune checkpoint genes highlight the potential of the model to identify patients who may benefit from immunotherapy.
These findings provide additional evidence for the effectiveness of the prognostic model based on asthma-associated DEGs in lung cancer. The model not only stratifies patients into high-risk and low-risk groups but also reflects the underlying immune characteristics of these patients. The associations with immune checkpoint genes further emphasize the clinical relevance of the model and its potential to guide personalized treatment decisions, particularly in the context of immunotherapy.
In conclusion, the analysis of immune cell correlations and checkpoint gene associations strengthens the validity and utility of the prognostic model. The model’s ability to capture immune-related differences between high-risk and low-risk patients underscores its potential as a valuable tool for risk stratification and treatment planning in lung cancer patients. Further research is warranted to explore the mechanistic links between the identified key genes, immune cell populations, and checkpoint genes, as well as to validate the model’s performance in independent cohorts.
GSEA conducted between high-risk and low-risk groups
To determine the pathways enriched in different risk groups, we performed GSEA encompassing BP, MF, CC, and KEGG pathways. In the BP category, processes such as epidermis development and keratinocyte differentiation were significantly enriched (Figure 5A), suggesting their potential involvement in the progression and prognosis of lung cancer. MF analysis revealed significant enrichment of functions like ATP-dependent DNA activity and immunoreceptor activity (Figure 5B), indicating the importance of these molecular mechanisms in the pathogenesis of lung cancer. Within the CC category, chromosome regions showed significant enrichment (Figure 5C), implying the role of chromosomal alterations in lung cancer development.
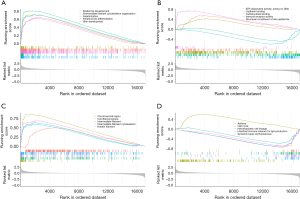
Notably, in the KEGG pathway analysis, the asthma pathway exhibited significant enrichment (Figure 5D). This finding suggests that the expression patterns of asthma-related genes may have a crucial impact on risk assessment and prognostic prediction in lung cancer patients. It highlights the potential key role of asthma in the pathological process of lung cancer, a discovery that holds great significance for the prognostic model constructed based on asthma gene sets. The enrichment of the asthma pathway in the high-risk group supports the validity and effectiveness of our prognostic model, which incorporates asthma-related genes as predictive factors.
Collectively, these enrichment analysis results provide valuable insights into the potential key biological pathways and functions involved in lung cancer development. By identifying the significant enrichment of processes such as epidermis development, keratinocyte differentiation, ATP-dependent DNA activity, immunoreceptor activity, and the asthma pathway, our study sheds light on the molecular mechanisms underlying lung cancer progression and risk stratification. These findings not only validate the effectiveness of our prognostic model but also offer a deeper understanding of the complex interplay between asthma and lung cancer, paving the way for further research and potential therapeutic interventions targeting these pathways.
Single-cell RNA sequencing reveals cell type-specific enrichment and developmental trajectories of key genes
To investigate the expression patterns of the four key genes in specific cell types, we performed scRNA-seq analysis. We obtained 27,365 cells from two tumor tissues and two adjacent normal tissues from the GSE198099 dataset. A total of 22 clusters were identified and visualized using UMAP (Figure 6A). Further annotation of these cell clusters was conducted using SingleR and manual annotation based on marker genes, leading to the identification of 13 cell types (Figure 6B). Among the four key genes, two genes (CD69 and KLF10) exhibited cell type-specific enrichment. In adjacent normal tissues, CD69 was specifically enriched in B cells (cluster 8), CD4+ T cells (cluster 6), CD8+ T cells (clusters 0, 1, and 13), mast cells (cluster 17), and NK cells (cluster 2). KLF10 was specifically enriched in endothelial cells (cluster 14), fibroblasts (cluster 16), and type II alveolar cells (cluster 15) (Figure 6C,6D). In tumor tissues, CD69 was specifically enriched in B cells (cluster 8), CD8+ T cells (clusters 0 and 1), mast cells (cluster 17), and NK cells (cluster 2). KLF10 was specifically enriched in dendritic cells (clusters 11 and 21), endothelial cells (cluster 14), Type II alveolar cells (cluster 15), and macrophages (cluster 9) (Figure 6E,6F).
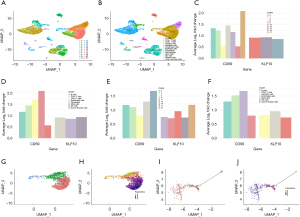
To uncover the heterogeneity of cell states during disease progression, we performed pseudotime analysis on the two main cell populations: CD8+ T cells and dendritic cells. Monocle 3 revealed the developmental trajectories of CD8+ T cells from cluster 0 to cluster 1 and finally to cluster 13 (Figure 6G,6H) and dendritic cells from cluster 11 to cluster 21 (Figure 6I,6J).
Notably, the trajectory analysis revealed differential expression patterns of the key genes. Low expression of CD69 was associated with high risk scores. As CD8+ T cells progressed from cluster 0 to cluster 1 and finally to cluster 13, the specific expression of CD69 decreased. Moreover, cluster 13 in tumor tissues did not exhibit specific expression of CD69. Conversely, high expression of KLF10 was associated with high risk scores. As Dendritic cells progressed from cluster 11 to cluster 21, the specific expression of KLF10 increased. These findings further support our previously constructed prognostic model.
In conclusion, our scRNA-seq analysis identified cell type-specific enrichment of CD69 and KLF10 in various cells. The developmental trajectories of CD8+ T cells and Dendritic cells revealed differential expression patterns of these key genes, with lower CD69 expression and higher KLF10 expression associated with higher risk scores. These results provide additional validation for our prognostic model and offer insights into the cell type-specific roles of these genes in lung cancer progression.
CellChat analysis reveals coordinated cellular interactions and signaling pathways in lung cancer
To determine the potential interactions between cells, we performed CellChat analysis. Cell communication mainly consists of three parts: secreted signaling, ECM-receptor, and cell-cell contact (Figure 7A). We then selected secreted signaling for further analysis, and the results showed close interactions among various cell types, except for ciliated cells (Figure 7B). Our analysis revealed that the MIF signaling pathway is very likely to play a role in lung cancer (Figure 7C). In this signaling pathway, the main interactions include epithelial cells and fibroblasts as senders, and B cells, dendritic cells, and macrophages as receivers (Figure 7D).
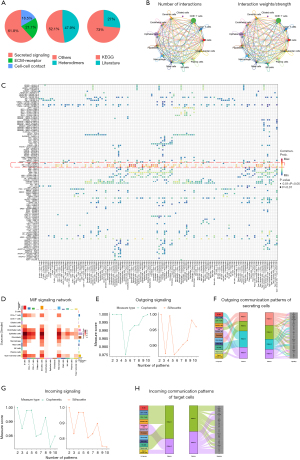
To understand how multiple cell populations and signaling pathways coordinate their functions in lung cancer, we divided the outgoing communication into four modules (Figure 7E). Epithelial cells and fibroblasts belong to pattern4, and the MIF signaling pathway also belongs to pattern4 (Figure 7F). The incoming communication was divided into two modules (Figure 7G), with B cells, dendritic cells, and macrophages belonging to pattern1, and the MIF signaling pathway also belonging to pattern1 (Figure 7H). This further validates the coordinated actions of epithelial cells and fibroblasts as senders and B cells, dendritic cells, and macrophages as receivers, based on the consideration of all signaling pathways (not just the MIF signaling pathway).
Notably, the MIF, MK, PTN, CXCL, and CHEMERIN signaling pathways belong to the same module in both incoming and outgoing communication, suggesting that these five signaling pathways are likely to coordinate their functions.
Integrating these findings with the background information on the potential relationships between the signaling pathways and the four genes (CD69, KIF18A, HN1L, and KLF10) used in the prognostic model, we can infer that the coordinated actions of these signaling pathways and cellular interactions may contribute to the effectiveness of the prognostic model. The MIF and CXCL pathways, in particular, have been linked to CD69 expression and function, suggesting their potential role in the prognostic value of the model.
Furthermore, the coordination of MIF, MK, PTN, CXCL, and CHEMERIN signaling pathways in both incoming and outgoing communication implies that these pathways may work together to regulate the tumor microenvironment and influence the prognostic value of the four-gene model. Although direct interactions between these signaling pathways and the four genes may not be fully elucidated, their coordinated actions in the complex network of cellular interactions and signaling pathways within the tumor microenvironment support the validity and effectiveness of the prognostic model.
In conclusion, the CellChat analysis reveals coordinated cellular interactions and signaling pathways in lung cancer, with the MIF signaling pathway and the coordinated actions of epithelial cells, fibroblasts, B cells, dendritic cells, and macrophages playing prominent roles. The coordination of MIF, MK, PTN, CXCL, and CHEMERIN signaling pathways in both incoming and outgoing communication suggests their potential involvement in regulating the tumor microenvironment and contributing to the effectiveness of the four-gene prognostic model. These findings provide further support for the validity of the prognostic model and highlight the importance of considering the complex interplay between cellular interactions and signaling pathways in understanding lung cancer prognosis.
Discussion
Growing evidence suggests that allergic diseases are associated with the incidence of multiple cancers. MR studies support the protective effect of allergic diseases on esophageal cancer, colorectal cancer, and possibly gastric cancer (20), but did not find a causal impact on breast and prostate cancer risk (21). A systematic review further indicates that allergies are associated with reduced risk of brain cancer, pancreatic cancer, melanoma, lymphatic and hematopoietic system cancers, colorectal cancer, female urogenital system cancers, and overall cancer risk (10). While a previous study also explored the asthma-lung cancer relationship through meta-analysis and MR (11), our study extends these findings by focusing on the molecular mechanisms and developing a prognostic model. Our study uniquely integrates multi-omics approaches to explore the molecular mechanisms underlying this association. In this study, we used two distinct approaches, integrating epidemiological data from the GBD database and causal inference from MR analysis, to explore the relationship between asthma and lung cancer. Importantly, these methods yielded conflicting results, highlighting the complexity of this relationship. GBD data demonstrated a significant positive correlation (R=0.56, P<0.01) between the incidence of asthma and lung cancer, suggesting a potential association at the epidemiological level, which is consistent with some previous observational studies indicating that asthma patients may face a higher risk of lung cancer (10,13,14). However, our MR analysis supported that asthma may reduce the risk of lung cancer (IVW: OR: 0.89, 95% CI: 0.84–0.95, P=4.0088e−04). This contradiction underscores the importance of carefully interpreting observational associations and using complementary methods to assess causal relationships.
Several factors may contribute to this discrepancy: (I) unmeasured confounding factors. The positive correlation between asthma and lung cancer found in observational studies may be influenced by unmeasured confounding factors, such as common environmental exposures or lifestyle factors. For instance, both asthma and lung cancer incidence may be elevated in populations exposed to high levels of pollution, creating a spurious correlation. MR analysis, by using genetic variants as IVs, can better control for these confounding factors, thus providing more reliable evidence regarding causal relationships (22,23). (II) Reverse causality. The relationship between asthma and lung cancer may be affected by reverse causality, where lung cancer and its treatment may influence the occurrence or control of asthma (15). This may lead observational studies to overestimate the impact of asthma on lung cancer risk, while MR analysis, by using genetic variants IVs, can avoid the influence of reverse causality. Moreover, there may be biological heterogeneity in the relationship between asthma and lung cancer. (III) Heterogeneity in asthma phenotypes. Asthma is a complex disease, and different types or phenotypes of asthma may have different relationships with lung cancer (24,25). For example, some types of asthma may be primarily allergen-driven, while others may be related to infection or airway remodeling. The inflammatory or immune response pathways involved in different types of asthma may have different impacts on the occurrence of lung cancer (26). These divergent mechanisms may lead to conflicting results when generalized across all asthma cases, emphasizing the need to analyze specific asthma subtypes. (IV) Bias in observational studies. Reporting bias and selection bias may also distort the true associations in observational studies. For example, populations with better healthcare access might report both asthma and early-stage lung cancer more frequently, artificially inflating the correlation. In summary, while the GBD data suggest a positive correlation between asthma and lung cancer at the epidemiological level, our MR analysis provides evidence for a protective effect of asthma, likely mediated by immune or inflammatory mechanisms. These findings highlight the complexity of this relationship and underscore the need for further research to explore the underlying biological mechanisms and the role of different asthma phenotypes in lung cancer risk. A deeper understanding of this association could offer new insights into the prevention and treatment of lung cancer, particularly for asthma patients.
Asthma is driven by both genetic predispositions and environmental factors, such as allergens, air pollution, and smoking, which interact through epigenetic mechanisms. Epigenetic modifications, including DNA methylation, histone modifications, and non-coding RNAs, play a critical role in mediating these interactions. For example, DNA methylation changes in genes regulating Th2 cytokines, such as IL-4 and IL-13, can amplify allergic inflammation in asthma, while environmental exposures like smoking can alter the epigenetic landscape, contributing to chronic inflammation and airway remodeling. These epigenetic processes may underlie some of the shared immune and inflammatory pathways observed in our study, such as the IL-17 signaling pathway, providing further insight into the biological connection between asthma and lung cancer.
Based on the epidemiological association between asthma and lung cancer and their causal relationship in MR analysis, we conducted bulk RNA analysis. We identified 39 asthma-related DEGs in lung cancer from 1,016 lung cancer tissues and 59 paracancerous tissues. The PBMC samples used for asthma were chosen because they reflect systemic immune responses associated with asthma, whereas lung tissue samples from lung cancer patients provide localized tumor microenvironment data. Together, these sample types offer complementary insights into the systemic and local interactions between asthma and lung cancer. The use of PBMC samples for asthma and lung tissue samples for lung cancer introduces inherent differences in sample types, which may influence the comparability of the identified DEGs. However, these shared pathways suggest a potential systemic link between asthma and lung cancer. In lung cancer, these 39 genes showed good correlation and interaction, indicating that they may be involved in common biological pathways and regulatory networks. We noted that these genes were mainly enriched in key regulatory mechanisms related to cell differentiation and ion response, and the involved DNA binding and transcriptional regulation reflected significantly enhanced transcriptional regulatory activity. Notably, these genes were enriched in the IL-17 signaling pathway and PD-L1 expression and PD-1 checkpoint pathway in cancer. The IL-17 signaling pathway in asthma mainly promotes airway inflammation and increases airway remodeling, thus exacerbating the pathological process of asthma (27-29). This deepens the understanding of the association between asthma and lung cancer. The PD-L1 expression and PD-1 checkpoint pathway in cancer suggests that these genes may be related to immunotherapy and may be beneficial for predicting the efficacy of immunotherapy. This finding highlights a shared immune-related mechanism between asthma and lung cancer, offering insights into the potential overlap in their pathophysiological processes.
Despite the differences in sample sources, the prognostic model based on four asthma-related genes (CD69, KIF18A, HN1L, KLF10) effectively stratified lung cancer patients. These four genes were identified as having significant prognostic value through a rigorous screening process. Using LASSO regression analysis, we selected these genes from the 39 asthma-related DEGs identified in lung cancer, and their prognostic significance was further confirmed by multivariate Cox proportional hazards analysis. High-risk scores derived from the model were characterized by higher expression levels of KIF18A, HN1L, and KLF10, and lower expression of CD69, which were significantly associated with reduced survival in lung cancer patients. Multivariate Cox regression further established the riskScore as an independent prognostic factor, highlighting the robust prognostic value of these four genes. Several studies have demonstrated the role of these four genes in lung cancer. CD69 may contribute to the retention of T cells within the tumor tissue and may influence their exhaustion state (30-32). This characteristic may impact lung cancer prognosis, as some studies suggest that the presence of CD69+ immune cells in tumors such as lung cancer may be associated with a favorable prognosis (33-35). KIF18A is upregulated in lung cancer, and its overexpression is associated with poor patient prognosis (36). This overexpression is associated with higher tumor stage and may affect tumor growth and metastasis (37). HN1L overexpression promotes malignant proliferation of non-small cell lung cancer cells (38). Additionally, studies have shown that KLF10 is associated with poor prognosis in lung squamous cell carcinoma (39-41). The present study also supports these research results, indicating that the prognostic model consisting of the four key genes observed in lung cancer has the potential to be a good prognostic marker. Integrating riskScore, age, and TNM staging, we constructed nomograms and multivariate Cox analysis as well as survival analysis for lung cancer patients, further confirming the effectiveness of our prognostic model. Moreover, our prognostic model was also validated in GSE30219, further confirming its effectiveness.
Through the analysis of immune cell correlation and immune checkpoint gene correlation, we found that the model can effectively capture immune-related differences between high-risk and low-risk patients. The significant associations between the riskScore and various immune cell populations, such as CD8+ T cells, macrophages, and memory B cells, suggest that the model reflects the immune microenvironment of lung cancer. This indicates that high-risk patients may exhibit distinct immune profiles, which could be linked to tumor progression or immune suppression. Significant differences in multiple immune cell populations between the high-risk and low-risk groups indicate that the model can stratify patients according to their immune profiles, which is important for guiding personalized treatment decisions. Immunotherapy, characterized by its ability to target immune checkpoints such as PD-1 and PD-L1, has become a cornerstone in cancer treatment. Evaluation criteria for immunotherapy efficacy include biomarker expression (e.g., PD-L1 levels), tumor-infiltrating lymphocyte (TIL) presence, and clinical outcomes such as progression-free survival (PFS) and OS. In our study, the strong correlation between riskScore and immune checkpoint genes suggests that the prognostic model could serve as a useful tool for identifying lung cancer patients who may benefit from immune checkpoint blockade therapies, providing a new dimension for precision medicine. Furthermore, the correlation between riskScore and immune checkpoint genes highlights the potential of the model to identify patients who may benefit from immunotherapy. This finding is highly consistent with the current research hotspots in tumor immunotherapy (42,43), indicating that the prognostic model can not only predict patients’ survival risk but also provide a reference for selecting appropriate immunotherapy candidates. Additionally, while immunotherapy can significantly improve survival in cancer patients, its effects on asthma remain complex. Immune checkpoint inhibitors can exacerbate asthma symptoms by amplifying immune activation, particularly in Th2-dominated conditions. On the other hand, these therapies may also modulate inflammatory pathways, such as the IL-17 pathway identified in our study, potentially influencing asthma outcomes. This dual effect underscores the importance of closely monitoring asthma patients undergoing immunotherapy to mitigate adverse effects. At the same time, the negative correlation between ESTIMATEScore and riskScore further confirms the model’s ability to reflect the immune landscape of lung cancer patients. Moreover, GSEA results showed that the asthma pathway was significantly enriched in the high-risk group, indicating that the expression patterns of asthma-related genes may have an important impact on risk assessment and prognostic prediction in lung cancer patients, highlighting the potential key role of asthma in the pathogenesis of lung cancer and further supporting the effectiveness of our prognostic model. In summary, these results emphasize the potential of the prognostic model based on asthma-related genes as a powerful tool for risk stratification and treatment planning in lung cancer patients, providing an important theoretical basis for the clinical application of the model. Future studies should further explore the interplay between immunotherapy and asthma to optimize treatment strategies for lung cancer patients with coexisting asthma.
We further explored the expression patterns of the four key genes in the prognostic model in specific cell types through scRNA-seq analysis. The results showed that CD69 and KLF10 exhibited cell-type-specific enrichment in different cell types. Notably, pseudotime analysis highlighted that cell populations initially enriched with CD69 and KLF10 underwent developmental shifts into states associated with higher prognostic risk scores, indicating that dynamic changes in gene expression correspond to disease progression. This provides additional validation for our previously constructed prognostic model and offers insights into the cell-type-specific roles of these genes in lung cancer progression. Furthermore, we determined potential cell-cell interactions through CellChat analysis. The results suggest that the MIF signaling pathway may play an important role in lung cancer, with epithelial cells and fibroblasts as signal senders and B cells, dendritic cells, and macrophages as signal receivers. MIF acts as an immune regulator in the tumor microenvironment, inducing the formation of a tumor-suppressive immune environment (44). Notably, the MIF, MK, PTN, CXCL, and CHEMERIN signaling pathways belong to the same module in both outgoing and incoming communication, indicating that these five signaling pathways may coordinate their functions. Integrating these findings with background information on the potential relationships between signaling pathways and the four genes (CD69, KIF18A, HN1L, and KLF10) used in the prognostic model, we can infer that the coordination of these signaling pathways and cell-cell interactions may contribute to the effectiveness of the prognostic model. In particular, the MIF and CXCL pathways have been associated with CD69 expression and function, suggesting their potential role in the prognostic value of the model (45-53). These findings further support the validity of the prognostic model and highlight the importance of considering complex interactions between cell-cell interactions and signaling pathways in understanding lung cancer prognosis.
Despite these promising findings, we recognize the inherent challenges associated with bulk RNA sequencing analysis of lung cancer tissues. One significant limitation lies in the complexity of lung pathology, particularly in advanced stages of the disease, where tumor regions often coexist with atelectasis and pneumonitis. This heterogeneity may confound the identification of DEGs specifically associated with lung cancer progression. Such complexity raises the difficulty of distinguishing whether observed gene expression changes are the “cause” or the “result” of the disease. To address this, we integrated single-cell RNA sequencing (scRNA-seq) data to validate the cell-type-specific expression of key genes in the prognostic model, which allowed us to partially mitigate the confounding effects of tissue complexity and enhance the reliability of our findings.
Despite the comprehensive analysis and significant findings, there are some limitations in this study. First, although we integrated multiple levels of evidence, including epidemiological data, genetic data, and transcriptomic data, further experimental validation is required to confirm the causal relationships and molecular mechanisms identified in this study. Second, the prognostic model was constructed and validated based on publicly available datasets, and its clinical application needs to be further evaluated in prospective cohorts. Third, the functional roles and interactions of the key genes and signaling pathways identified in this study warrant further investigation to elucidate their precise mechanisms in lung cancer pathogenesis and prognosis.
Conclusions
In conclusion, our study provides a comprehensive analysis of the relationship between asthma and lung cancer, utilizing epidemiological data, MR analysis, and transcriptomic data. We constructed a robust prognostic model based on asthma-related DEGs in lung cancer, which showed good performance in risk stratification and prognostic prediction. The model also demonstrated potential in capturing immune-related differences and guiding immunotherapy decisions. The scRNA-seq analysis further validated the cell-type-specific expression patterns of key genes and revealed potential cell-cell interactions and signaling pathways that may contribute to the effectiveness of the prognostic model. In addition to its implications for lung cancer, our study provides new insights into asthma by identifying asthma-related genes, such as CD69, KIF18A, HN1L, and KLF10, which may serve as potential biomarkers for early diagnosis and disease evaluation. The enrichment of asthma-related pathways, such as the IL-17 signaling pathway, highlights their relevance in assessing disease severity and progression. These findings offer a novel basis for integrating immune and transcriptomic markers into personalized strategies for asthma diagnosis and prognosis. These findings enhance our understanding of the complex relationship between asthma and lung cancer and provide valuable insights for the development of personalized prevention and treatment strategies for lung cancer patients with asthma.
Acknowledgments
None.
Footnote
Reporting Checklist: The authors have completed the STROBE-MR reporting checklist. Available at https://jtd.amegroups.com/article/view/10.21037/jtd-24-1697/rc
Peer Review File: Available at https://jtd.amegroups.com/article/view/10.21037/jtd-24-1697/prf
Funding: This work was supported by
Conflicts of Interest: All authors have completed the ICMJE uniform disclosure form (available at https://jtd.amegroups.com/article/view/10.21037/jtd-24-1697/coif). The authors have no conflicts of interest to declare.
Ethical Statement: The authors are accountable for all aspects of the work in ensuring that questions related to the accuracy or integrity of any part of the work are appropriately investigated and resolved. The study was conducted in accordance with the Declaration of Helsinki (as revised in 2013).
Open Access Statement: This is an Open Access article distributed in accordance with the Creative Commons Attribution-NonCommercial-NoDerivs 4.0 International License (CC BY-NC-ND 4.0), which permits the non-commercial replication and distribution of the article with the strict proviso that no changes or edits are made and the original work is properly cited (including links to both the formal publication through the relevant DOI and the license). See: https://creativecommons.org/licenses/by-nc-nd/4.0/.
References
- Sung H, Ferlay J, Siegel RL, et al. Global Cancer Statistics 2020: GLOBOCAN Estimates of Incidence and Mortality Worldwide for 36 Cancers in 185 Countries. CA Cancer J Clin 2021;71:209-49. [Crossref] [PubMed]
- Murray CJ, Atkinson C, Bhalla K, et al. The state of US health, 1990-2010: burden of diseases, injuries, and risk factors. JAMA 2013;310:591-608. [Crossref] [PubMed]
- Nasim F, Sabath BF, Eapen GA. Lung Cancer. Med Clin North Am 2019;103:463-73. [Crossref] [PubMed]
- Papi A, Brightling C, Pedersen SE, et al. Asthma. Lancet 2018;391:783-800. [Crossref] [PubMed]
- He MM, Lo CH, Wang K, et al. Immune-Mediated Diseases Associated With Cancer Risks. JAMA Oncol 2022;8:209-19. [Crossref] [PubMed]
- Azad N, Rojanasakul Y, Vallyathan V. Inflammation and lung cancer: roles of reactive oxygen/nitrogen species. J Toxicol Environ Health B Crit Rev 2008;11:1-15. [Crossref] [PubMed]
- Allegra J, Lipton A, Harvey H, et al. Decreased prevalence of immediate hypersensitivity (atopy) in a cancer population. Cancer Res 1976;36:3225-6.
- El-Zein M, Parent ME, Siemiatycki J, et al. History of allergic diseases and lung cancer risk. Ann Allergy Asthma Immunol 2014;112:230-6. [Crossref] [PubMed]
- Turner MC, Chen Y, Krewski D, et al. An overview of the association between allergy and cancer. Int J Cancer 2006;118:3124-32. [Crossref] [PubMed]
- Karim AF, Westenberg LEH, Eurelings LEM, et al. The association between allergic diseases and cancer: a systematic review of the literature. Neth J Med 2019;77:42-66.
- Huang Q, Huang Y, Xu S, et al. Association of asthma and lung cancer risk: A pool of cohort studies and Mendelian randomization analysis. Medicine (Baltimore) 2024;103:e35060. [Crossref] [PubMed]
- García Sanz MT, González Barcala FJ, Alvarez Dobaño JM, et al. Asthma and risk of lung cancer. Clin Transl Oncol 2011;13:728-30. [Crossref] [PubMed]
- Kantor ED, Hsu M, Du M, et al. Allergies and Asthma in Relation to Cancer Risk. Cancer Epidemiol Biomarkers Prev 2019;28:1395-403. [Crossref] [PubMed]
- Boffetta P, Ye W, Boman G, et al. Lung cancer risk in a population-based cohort of patients hospitalized for asthma in Sweden. Eur Respir J 2002;19:127-33. [Crossref] [PubMed]
- Jiang L, Sun YQ, Langhammer A, et al. Asthma and asthma symptom control in relation to incidence of lung cancer in the HUNT study. Sci Rep 2021;11:4539. [Crossref] [PubMed]
- Rahman HH, Niemann D, Munson-McGee SH. Association between asthma, chronic bronchitis, emphysema, chronic obstructive pulmonary disease, and lung cancer in the US population. Environ Sci Pollut Res Int 2023;30:20147-58. [Crossref] [PubMed]
- Charokopos A, Braman SS, Brown SAW, et al. Lung Cancer Risk among Patients with Asthma-Chronic Obstructive Pulmonary Disease Overlap. Ann Am Thorac Soc 2021;18:1894-900. [Crossref] [PubMed]
- Brown DW, Young KE, Anda RF, et al. Asthma and risk of death from lung cancer: NHANES II Mortality Study. J Asthma 2005;42:597-600. [Crossref] [PubMed]
- Yoshihara K, Shahmoradgoli M, Martínez E, et al. Inferring tumour purity and stromal and immune cell admixture from expression data. Nat Commun 2013;4:2612. [Crossref] [PubMed]
- Yuan S, Vithayathil M, Kar S, et al. Assessing the protective role of allergic disease in gastrointestinal tract cancers using Mendelian randomization analysis. Allergy 2021;76:1559-62. [Crossref] [PubMed]
- Jiang X, Dimou NL, Zhu Z, et al. Allergy, asthma, and the risk of breast and prostate cancer: a Mendelian randomization study. Cancer Causes Control 2020;31:273-82. [Crossref] [PubMed]
- Sekula P, Del Greco M F, Pattaro C, et al. Mendelian Randomization as an Approach to Assess Causality Using Observational Data. J Am Soc Nephrol 2016;27:3253-65. [Crossref] [PubMed]
- Bowden J, Holmes MV. Meta-analysis and Mendelian randomization: A review. Res Synth Methods 2019;10:486-96. [Crossref] [PubMed]
- Chung KF, Dixey P, Abubakar-Waziri H, et al. Characteristics, phenotypes, mechanisms and management of severe asthma. Chin Med J (Engl) 2022;135:1141-55. [Crossref] [PubMed]
- Miller RL, Grayson MH, Strothman K. Advances in asthma: New understandings of asthma's natural history, risk factors, underlying mechanisms, and clinical management. J Allergy Clin Immunol 2021;148:1430-41. [Crossref] [PubMed]
- Peebles RS Jr, Aronica MA. Proinflammatory Pathways in the Pathogenesis of Asthma. Clin Chest Med 2019;40:29-50. [Crossref] [PubMed]
- Ritzmann F, Lunding LP, Bals R, et al. IL-17 Cytokines and Chronic Lung Diseases. Cells 2022;11:2132. [Crossref] [PubMed]
- Ji T, Li H. T-helper cells and their cytokines in pathogenesis and treatment of asthma. Front Immunol 2023;14:1149203. [Crossref] [PubMed]
- Habib N, Pasha MA, Tang DD. Current Understanding of Asthma Pathogenesis and Biomarkers. Cells 2022;11:2764. [Crossref] [PubMed]
- Wang Y, Sun Z, Du X, et al. Increased death and exhaustion of CD69(high) T cells and NK cells are associated with PD-1 antibody application in the in vitro co-culture system. PeerJ 2023;11:e15374. [Crossref] [PubMed]
- Mita Y, Kimura MY, Hayashizaki K, et al. Crucial role of CD69 in anti-tumor immunity through regulating the exhaustion of tumor-infiltrating T cells. Int Immunol 2018;30:559-67. [Crossref] [PubMed]
- Li Y, Gu Y, Yang P, et al. CD69 is a Promising Immunotherapy and Prognosis Prediction Target in Cancer. Immunotargets Ther 2024;13:1-14. [Crossref] [PubMed]
- Hu ZW, Sun W, Wen YH, et al. CD69 and SBK1 as potential predictors of responses to PD-1/PD-L1 blockade cancer immunotherapy in lung cancer and melanoma. Front Immunol 2022;13:952059. [Crossref] [PubMed]
- Koyama-Nasu R, Wang Y, Hasegawa I, et al. The cellular and molecular basis of CD69 function in anti-tumor immunity. Int Immunol 2022;34:555-61. [Crossref] [PubMed]
- Wu ZX, Da TT, Huang C, et al. CD69(+)CD103(+)CD8(+) tissue-resident memory T cells possess stronger anti-tumor activity and predict better prognosis in colorectal cancer. Cell Commun Signal 2024;22:608. [Crossref] [PubMed]
- Zhong Y, Jiang L, Lin H, et al. Overexpression of KIF18A promotes cell proliferation, inhibits apoptosis, and independently predicts unfavorable prognosis in lung adenocarcinoma. IUBMB Life 2019;71:942-55. [Crossref] [PubMed]
- Chen FT, Zhong FK. Kinesin Family Member 18A (KIF18A) Contributes to the Proliferation, Migration, and Invasion of Lung Adenocarcinoma Cells In Vitro and In Vivo. Dis Markers 2019;2019:6383685. [Crossref] [PubMed]
- Li L, Zeng TT, Zhang BZ, et al. Overexpression of HN1L promotes cell malignant proliferation in non-small cell lung cancer. Cancer Biol Ther 2017;18:904-15. [Crossref] [PubMed]
- Lai X, Fu G, Du H, et al. Identification of a cancer-associated fibroblast classifier for predicting prognosis and therapeutic response in lung squamous cell carcinoma. Medicine (Baltimore) 2023;102:e35005. [Crossref] [PubMed]
- Feng X, Liu X, Xiang J, et al. Exosomal ITGB6 from dormant lung adenocarcinoma cells activates cancer-associated fibroblasts by KLF10 positive feedback loop and the TGF-β pathway. Transl Lung Cancer Res 2023;12:2520-37. [Crossref] [PubMed]
- Zhang Y, Xue Q, Pan G, et al. Integrated Analysis of Genome-Wide Copy Number Alterations and Gene Expression Profiling of Lung Cancer in Xuanwei, China. PLoS One 2017;12:e0169098. [Crossref] [PubMed]
- Memon D, Schoenfeld AJ, Ye D, et al. Clinical and molecular features of acquired resistance to immunotherapy in non-small cell lung cancer. Cancer Cell 2024;42:209-224.e9. [Crossref] [PubMed]
- Nabet BY, Hamidi H, Lee MC, et al. Immune heterogeneity in small-cell lung cancer and vulnerability to immune checkpoint blockade. Cancer Cell 2024;42:429-443.e4. [Crossref] [PubMed]
- Calandra T, Bucala R. Macrophage Migration Inhibitory Factor (MIF): A Glucocorticoid Counter-Regulator within the Immune System. Crit Rev Immunol 2017;37:359-70. [Crossref] [PubMed]
- Domingues PH, Teodósio C, Otero Á, et al. Association between inflammatory infiltrates and isolated monosomy 22/del(22q) in meningiomas. PLoS One 2013;8:e74798. [Crossref] [PubMed]
- Nakajima H, Takagi H, Horiguchi N, et al. Lack of macrophage migration inhibitory factor protects mice against concanavalin A-induced liver injury. Liver Int 2006;26:346-51. [Crossref] [PubMed]
- Reome JB, Hylind JC, Dutton RW, et al. Type 1 and type 2 tumor infiltrating effector cell subpopulations in progressive breast cancer. Clin Immunol 2004;111:69-81. [Crossref] [PubMed]
- Takagi D, Iwabuchi K, Maeda M, et al. Natural killer T cells ameliorate antibody-induced arthritis in macrophage migration inhibitory factor transgenic mice. Int J Mol Med 2006;18:829-36.
- LaBelle JL, Truitt RL. Characterization of a murine NKT cell tumor previously described as an acute myelogenous leukemia. Leuk Lymphoma 2002;43:1637-44. [Crossref] [PubMed]
- Xu X, Ye L, Zhang Q, et al. Group-2 Innate Lymphoid Cells Promote HCC Progression Through CXCL2-Neutrophil-Induced Immunosuppression. Hepatology 2021;74:2526-43. [Crossref] [PubMed]
- Radulovic K, Rossini V, Manta C, et al. The early activation marker CD69 regulates the expression of chemokines and CD4 T cell accumulation in intestine. PLoS One 2013;8:e65413. [Crossref] [PubMed]
- Wang F, Zhang G, Xu T, et al. High and selective cytotoxicity of ex vivo expanded allogeneic human natural killer cells from peripheral blood against bladder cancer: implications for natural killer cell instillation after transurethral resection of bladder tumor. J Exp Clin Cancer Res 2024;43:24. [Crossref] [PubMed]
- Lee YS, Park GS, Ko SH, et al. Lactobacillus paracasei ATG-E1 improves particulate matter 10 plus diesel exhaust particles (PM(10)D)-induced airway inflammation by regulating immune responses. Front Microbiol 2023;14:1145546. [Crossref] [PubMed]