Effect of respiratory phase on three-dimensional quantitative parameters of pulmonary subsolid nodules in low-dose computed tomography screening for lung cancer
Highlight box
Key findings
• In this study, we have found that the respiratory phase affects the three-dimensional quantitative parameters of pulmonary subsolid nodules (SSNs) in low-dose computed tomography (LDCT) screening, with varying impacts on different types of pulmonary SSNs.
What is known and what is new?
• Previous studies have shown that the degree of lung inflation, reflecting the change of lung volume, could affect the volume and density of pulmonary nodules.
• Our study found that the respiratory phase had the greatest effect on the volume of pulmonary SSNs and the least effect on the long axis diameter in LDCT screening. Most quantitative parameters of pure ground-glass nodules (pGGNs) were more susceptible to respiratory phase than those of part-solid nodules (PSNs).
What is the implication, and what should change now?
• During follow-up, LDCT scan in different respiratory phases may interfere with the judgment of the growth of pulmonary SSNs. The influence of respiratory phase should be fully considered when comparing the changes of SSNs volume in follow-up of LDCT scan.
Introduction
Because of the spread of low-dose computed tomography (LDCT) lung screening programmes, the number of subsolid nodules (SSNs) detected have increased dramatically. Pathologically, SSNs may be caused by partial interstitial thickening with inflammation, fibrosis, and tumor hyperplasia (1,2). The SSNs include pure ground-glass nodules (pGGNs) and part-solid nodules (PSNs) (3), most of which are early-stage lung adenocarcinomas (4-6). Most SSNs are inert; therefore, follow-up computed tomography (CT) scans are required to assess any changes. Size and density are the most crucial indicators in the follow-up CT to verify if there is no change, decrease, or growth in SSNs, which would dictate subsequent management, in accordance with the Fleischner Society guideline for SSNs (7). In general, the measurement of size and density is conducted during apnea following the acquisition of CT images after full inspiration. However, the degrees of lung inflation on follow-up chest CT scans may vary due to several factors, i.e., different respiratory phases, disease progression, and so on.
A previous study has shown that the degree of lung inflation, reflecting the change of lung volume, could affect the volume and density of pulmonary nodules (8). The size and volume of nonsolid nodules decrease when lung volume decreases during the expiratory phase, suggesting that the nonsolid nodule’s nature is readily altered by lung inflation, which may be connected to its pathological foundation (9). Therefore, we hypothesized that respiratory phase would affect the quantitative evaluation of the SSNs. Furthermore, if the effect is large enough and the patient has poor breath holding, it may affect the evaluation of pulmonary nodule growth through two follow-up CT scans. This hypothesis holds great significance in the management of SSNs, particularly with regard to their volume and density. Furthermore, a previous study has shown that pulmonary nodules exhibit reduced volumes and diameters on LDCT scans compared to standard-dose CT scans (10). Therefore, in LDCT screening, the effect of respiratory phase on the parameters of pulmonary SSNs is worthy of attention and exploration. Reviewing the literature, we could not find any papers on whether the changes in pulmonary SSNs caused by the respiratory phase will affect the judgment of growth during the follow-up of LDCT screening. The purpose of this study was to investigate the impact of respiratory phase on three-dimensional (3D) quantitative parameters of pulmonary SSNs in LDCT screening, examine how respiratory phase affects 3D quantitative parameters in different types of SSNs, and to further explore the impact of respiratory phase change on the judgment of pulmonary nodules growth. This information could affect the SSNs management in LDCT follow-up. We present this article in accordance with the TRIPOD reporting checklist (available at https://jtd.amegroups.com/article/view/10.21037/jtd-24-1440/rc).
Methods
Subject selection
This study retrospectively collected subjects who underwent the Netherlands-China Big-3 screening (NELCIN-B3, including early lung cancer, chronic obstructive pulmonary disease and cardiovascular disease) in our hospital, Second Affiliated Hospital of PLA Naval Medical University, between August 2018 and July 2021 (11). The study was conducted in accordance with the Declaration of Helsinki (as revised in 2013). The study was approved by the institutional review board of Second Affiliated Hospital of PLA Naval Medical University, Shanghai, China (No. 2018SL028), and the study was registered in the Chinese Clinical Trials Registry (http://www.chictr.org.cn/index.aspx; ChiCTR2000035283). All the individual participants igned written informed consent for participating in this study. The inclusion criteria for SSNs were as follows: (I) subjects received non-contrast enhanced paired inspiratory and expiratory chest CT scanning with low-dose; (II) chest CT reconstructed with slice thickness of 1 mm; (III) solitary or multiple lung nodule(s) manifesting as SSN on inspiratory CT imaging; (IV) no lung conditions that impact lung density, such as chronic obstructive pulmonary disease, any pattern of interstitial pneumonia, consolidation, and emphysema (emphysema index <6%). A total of 3,667 subjects underwent paired inspiratory and expiratory LDCT screening during aforementioned period. Among these subjects, the following cases were excluded: 1,543 with the long axis diameter of SSNs <6 mm; 150 with marked respiratory motion artifact of CT images or the boundary of nodules are blurred; 623 who exhibited solid/calcified nodules; 588 with any pattern of pneumonia and emphysema affecting mean lung density (MLD); 62 with thoracic deformity (pectus excavatum, pectus carinatum, etc.); 207 with pleural effusion; 264 with history of lung needle biopsy or surgery prior to CT scan. After rigorous screening, a total of 230 subjects with 255 SSNs were included in this study. The patient inclusion procedure is shown in Figure 1.
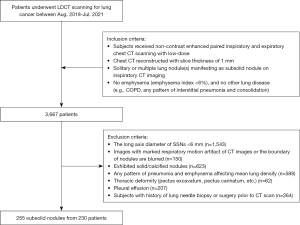
CT scanning
A 256-slice CT scanner (Brilliance-iCT, Philips Healthcare, Amsterdam, The Netherlands) was used to scan all the subjects at the conclusion of inspiration and expiration. Prior to CT scanning, breath-hold training was conducted. After complete inspiration or expiration, all the subjects were instructed to maintain apnea as long as possible. Three to five minutes separated the two scans were performed with an interval of 3–5 min (time to read the inspiratory images). Non-contrast enhanced imaging was performed from the thoracic inlet to the middle portion of the kidneys. The following imaging parameters were used for inspiratory and expiratory CT scanning: tube energy 120 kV, Z-axial and 3D automatic tube current modulation, DoseRight collimator (Philips Healthcare) was on and reduced dose level 3, pitch 0.915, collimation 128×0.625 mm, rotation 0.5 s, slice thickness 1 mm, slice interval 1 mm, matrix of 512×512, high-resolution algorithm, and iDose4 iterative reconstruction.
Imaging analysis
All SSN segmentations were performed manually using ITK-SNAP software by a radiologist who had 4-year experience in thoracic CT (Radiologist 1). The 3D volume of interest (VOI) of SSN was obtained by delineating its margin with the lung window setting (window width 1,500 HU, window level −500 HU). By using public PyRadiomics software (https://pyradiomics.readthedocs.io/en/latest/index.html), a widely used tool that complies to the image biomarker standardisation initiative (IBSI), the quantitative parameters of SSN were obtained from VOI of all nodules. Long axis diameter (the longest measurement of the nodule in any plane), short axis diameter (the line perpendicular to long axis diameter through the center), surface area, volume and density of SSNs were automatically produced and recorded. The average of long axial and short axial diameter was used to determine the mean diameter of SSNs. We selected 30 cases of SSNs to evaluate the reproducibility of intra-observer and inter-observer segmentation by thoracic radiologists with 4 years (Radiologist 1) and 8 years (Radiologist 2) of experience. Radiologist 1 performed two separate segmentations with a one-month interval, and Radiologist 2 only segmented 30 cases of SSNs once. The two radiologists were blinded to each other’s segmentation. The first segmentation by Radiologist 1 was used for further analysis. The ratio of expiratory parameter to inspiratory was computed and characterized as parameter(E-I)/I = [(parameterexpiratory − parameterinspiratory)/parameterinspiratory] based on the changes in the abovementioned parameters.
Lung quantitative parameters were analyzed with commercial software (A-VIEW, Suhai Alderi Information Technology Ltd., Dubai, UAE). After importing inspiratory and expiratory images into the software, lung volume and lung density were generated automatically. Because lung density and volume are affected by respiratory phase, which may affect the quantitative parameters of SSNs, lung density and volume were analyzed.
SSNs grouping
The SSNs were grouped according to the inspiratory CT images. Based on the guidelines of Fleischner Society (7), SSNs were divided into two groups according to the presence or absence of solid components: PSNs and pGGNs. The clinical common threshold for lung nodule size evaluation and the T descriptor of 9th edition tumor-node-metastasis (TNM) stage of non-small cell lung cancer (NSCLC) (12-15) were used to divide SSNs into two groups (≤10 and >10 mm) based on the long axis diameter. Based on the location of nodules, the nodules were divided into two groups, upper lobe (upper lobe of both lungs and middle lobe of right lung) and lower lobe.
In order to investigate whether changes in quantitative parameters of nodules caused by respiratory movement would affect the judgment of nodule growth of follow-up, according to the criteria of nodule growth in the follow-up guidelines, 255 SSNs were divided into a group with change of nodule that met the growth criteria of follow-up (changed group) and a group with change of nodule that did not meet the growth criteria (unchanged group). Changed group (representing growth) of SSNs was judged on the basis of CT scans by using the following criteria (7,12,16-18): (I) the mean diameter of nodules increased by 2 mm or more between the inspiratory phase and the expiratory phase CT; (II) the solid component of nodules increased by 2 mm or more between the inspiratory and expiratory CT; (III) there were new solid components in nodules on expiratory phase CT compared with inspiratory CT. The other nodules that did not meet the above conditions were classified as the unchanged group (Figure 2). The above two radiologists separately grouped the nodules according to CT images, and when the judgment was different, the decision was made by another senior radiologist (with 20 years of experience in thoracic CT).

Statistical analysis
We performed statistical analyses with SPSS 26.0 software (SPSS Inc.). Intraclass correlation coefficient (ICC) was used to evaluate the inter-observer and intra-observer agreement of quantitative parameters of SSNs. The Kolmogorov-Smirnov test was used to determine whether the distributions were normal. The data were expressed in mean ± standard deviation or median [interquartile range (IQR)]. In terms of measurement data that were normally distributed, paired-samples t-test or independent-samples t-test was used; otherwise, the Wilcoxon signed-rank test, Mann-Whitney U test or Kruskal-Wallis H test were utilized to assess the statistics. Two-sided P value <0.05 was considered to be statistically significant. Univariate logistic regression was used to analyze the possible factors related to the change of SSNs, and select significant factors (P<0.1). The selected parameters were imported into multivariate logistic regression using the stepwise regression method and a prediction model for the change of subsolid pulmonary nodules with respiratory phase was established. Receiver operator characteristic (ROC) curve was used to evaluate the predictive diagnostic efficiency of the model. The optimized threshold (cutoff value) was determined through Youden index, followed by the calculation of the sensitivity and specificity.
Results
There were 211 patients with solitary SSNs, 15 with two SSNs, two with three SSNs, and one with four SSNs. The inter-observer agreement of CT quantitative parameters was 0.856–0.964, and the intra-observer agreement was 0.839–0.965. The 3D quantitative parameters of SSNs obtained by manual segmentation method have high repeatability and stability.
Comparison of CT quantitative parameters of lung and SSNs between inspiratory and expiratory CT
The data in Table 1 revealed that there were significant differences in parameters of lung and SSNs between inspiratory and expiratory CT. Moreover, lung volume, long axis diameter, short axis diameter, mean diameter, surface area and volume were significantly higher in the inspiratory phase than those in the expiratory phase (P<0.001). Conversely, the lung density and the density of SSNs were significantly higher in the expiratory phase than those in the inspiratory phase (P<0.001).
Table 1
Parameters | Inspiratory CT | Expiratory CT | P |
---|---|---|---|
Lung | |||
Volume (cm3) | 4,100.67 (1,189.01) | 2,494.82 (941.11) | <0.001 |
Density (HU) | −836.29 (31.32) | −742.75 (45.14) | <0.001 |
SSNs | |||
Long axis (mm) | 8.39 (4.04) | 7.84 (3.65) | <0.001 |
Short axis (mm) | 7.02 (2.85) | 6.61 (2.53) | <0.001 |
Mean diameter (mm) | 7.80 (3.18) | 7.21 (3.03) | <0.001 |
Surface area (mm2) | 215.58 (200.98) | 180.40 (174.50) | <0.001 |
Volume (mm3) | 222.00 (289.00) | 177.00 (236.00) | <0.001 |
Density (HU) | −675.10 (115.49) | −587.35 (178.10) | <0.001 |
Data are expressed as median (interquartile range). Wilcoxon signed-ranks test was used. CT, computed tomography; LDCT, low-dose computed tomography; HU, Hounsfield unit; SSNs, subsolid nodules.
Change ratios of quantitative parameters between inspiratory and expiratory CT
The change ratios of quantitative parameters in expiratory CT relative to inspiratory CT are summarized in Table 2. Compared with the inspiratory phase, lung volume decreased by 38.75% (IQR, 17.05%), and lung density increased by 10.51%±4.91% in the expiratory phase. There are statistical differences in the change ratio between lung volume and lung density, as well as among different quantitative parameters of SSNs (P<0.001). Besides, the change ratio of the long axis diameter was the lowest (−7.14%±11.25%) and the change ratio of the volume was the highest (−20.21%±23.15%) in SSN parameters. In both lung and SSNs, the volume(E-I)/I was significantly higher than the density(E-I)/I. Mean diameter and surface area of SSN on expiratory CT was reduced by 7.67% (12.01%) and 15.52%±18.63%, respectively, while the density was increased by 12.08% (13.34%).
Table 2
Parameter(E-I)/I | Range (%) | Value (%) | P |
---|---|---|---|
Lung | <0.001** | ||
Volume | −61.81 to −0.03 | −38.75 (17.05) | |
Density | −22.76 to 1.49 | −10.51±4.91 | |
SSNs | <0.001* | ||
Long axis | −50.50 to 36.70 | −7.14±11.25 | |
Short axis | −45.84 to 49.28 | −7.71±12.28 | |
Mean diameter | −38.15 to 26.74 | −7.67 (12.01) | |
Surface area | −73.25 to 44.79 | −15.52±18.63 | |
Volume | −86.49 to 48.39 | −20.21±23.15 | |
Density | −69.96 to 6.46 | −12.08 (13.34) |
Data are expressed as mean ± standard deviation or median (interquartile range). Parameter(E-I)/I = [(parameterexpiratory − parameterinspiratory)/parameterinspiratory]. *, Kruskal-Wallis H test; **, Mann-Whitney U test. LDCT, low-dose computed tomography; SSNs, subsolid nodules.
Subgroup comparison between different types of SSNs
The results of subgroup comparison are presented in Tables S1-S3. The long axis diameter(E-I)/I of nodules showed no statistical difference between PSNs and pGGNs (P=0.16). However, there were significant differences in the short axis diameter(E-I)/I, mean diameter(E-I)/I, surface area(E-I)/I, volume(E-I)/I, and density(E-I)/I between PSNs and pGGNs (all P<0.05). The parameters(E-I)/I in pGGNs were greater than that in PSNs, except for the density(E-I)/I. Additionally, there were no significant differences in all parameters(E-I)/I between nodules with diameter ≤10 mm and diameter >10 mm (all P>0.05). A statistical difference was observed in the density(E-I)/I of SSNs between the upper and lower lobes, and the lower lobe has a higher density(E-I)/I of SSNs than the upper lobe (P<0.001). No significant differences were found in the change ratios of other quantitative parameters between the upper and lower lobes (all P>0.05).
Comparison of quantitative parameters between the changed group and unchanged group
After the respiration phase changed, 34 pGGNs and 34 PSNs demonstrated growth according to the follow-up criteria (changed group: 68 cases). Conversely, 179 pGGNs and 8 PSNs did not meet the growth criteria (unchanged group: 187 cases).
As shown in Table 3, the volume(E-I)/I of lung was significantly different between the two groups (P=0.03), while lung volume, lung density on inspiratory CT, and the density(E-I)/I of lung were not statistically different. Regarding the nodule parameters, there were significant differences in all the quantitative parameters of SSNs on inspiratory CT (all P<0.001), the long axis diameter(E-I)/I (P=0.002) and the density(E-I)/I (P<0.001) between the two groups. The long axis diameter, short axis diameter, mean diameter, surface area, volume and density of SSNs on inspiratory CT in the changed group were greater than those in unchanged group.
Table 3
Parameter | Changed group | Unchanged group | P |
---|---|---|---|
Lung | |||
Inspiratory CT | |||
Volume (cm3) | 4,150.35 (1,006.23) | 4,101.99 (1,186.00) | 0.46** |
Density (HU) | −829.83 (35.55) | −837.52 (27.52) | 0.20** |
Parameter(E-I)/I | |||
Volume (%) | 43.22 (16.27) | 38.65 (18.72) | 0.03** |
Density (%) | 12.53 (7.89) | 10.58 (4.94) | 0.050** |
SSNs | |||
Inspiratory CT | |||
Long axis (mm) | 11.79 (8.55) | 7.94 (2.12) | <0.001** |
Short axis (mm) | 9.84 (6.04) | 6.70 (1.96) | <0.001** |
Mean diameter (mm) | 10.96 (6.68) | 7.26 (1.95) | <0.001** |
Surface area (mm2) | 425.29 (564.46) | 189.53 (109.35) | <0.001** |
Volume (mm3) | 524.50 (1,015.00) | 186.00 (164.00) | <0.001** |
Density (HU) | −580.10 (98.60) | −701.87 (91.60) | <0.001** |
Parameter(E-I)/I | |||
Long axis (%) | −10.76±12.81 | −4.20±10.46 | 0.002* |
Short axis (%) | −9.63 (14.84) | −6.18 (12.24) | 0.63** |
Mean diameter (%) | −9.22 (13.41) | −5.26 (8.76) | 0.08** |
Surface area (%) | −14.81 (25.64) | −12.61 (17.58) | 0.68** |
Volume (%) | −23.13±27.86 | −16.12±22.53 | 0.29* |
Density (%) | −23.26 (14.93) | −10.03 (9.67) | <0.001** |
Data are expressed as mean ± standard deviation or median (interquartile range). Parameter(E-I)/I = [(parameterexpiratory − parameterinspiratory)/parameterinspiratory]; *, independent-sample t-test; **, Mann-Whitney U test. CT, computed tomography; HU, Hounsfield unit; SSNs, subsolid nodules.
Logistic regression analysis of 3D quantitative parameters
Since in the most lung cancer screening studies, as well as in routine clinical scenarios, only chest CT scans in the inspiratory phase are performed on subjects or patients, we only included the quantitative parameters in the inspiratory phase for logistic regression analysis. Logistic regression analysis results are shown in Table 4. The univariate logistic regression results showed that the lung density and all the quantitative parameters of SSNs on inspiratory CT were candidate predictors for whether SNNs change with respiratory phase (P<0.1). The selected parameters were imported into the multivariate logistic regression analysis. The results showed that the lung density on inspiratory CT, as well as the long axis diameter, short axis diameter, surface area and density of SSNs on inspiratory CT, were independent indicators of whether SSNs changed with respiratory phase. The area under the ROC curve was 0.911, with a sensitivity of 94.1% and a specificity of 70.6% (Figure 3).
Table 4
Parameters on inspiratory CT |
Univariate logistic regression | Multivariate logistic regression | |||||||
---|---|---|---|---|---|---|---|---|---|
β | OR value | 95% CI | P | β | OR value | 95% CI | P | ||
Lung | |||||||||
Volume | 0.000 | 1.000 | 1.000–1.000 | 0.39 | |||||
Density | 0.008 | 1.008 | 0.999–1.017 | 0.10 | –0.020 | 0.980 | 0.965–0.995 | 0.009 | |
SSNs | |||||||||
Long axis | 0.285 | 1.330 | 1.213–1.458 | <0.001 | 0.599 | 1.820 | 1.273–2.601 | 0.003 | |
Short axis | 0.395 | 1.483 | 1.308–1.680 | <0.001 | 0.600 | 1.822 | 1.138–2.918 | 0.009 | |
Mean diameter | 0.345 | 1.412 | 1.265–1.577 | <0.001 | |||||
Surface area | 0.003 | 1.003 | 1.002–1.004 | <0.001 | –0.013 | 0.987 | 0.977–0.997 | 0.02 | |
Volume | 0.001 | 1.001 | 1.001–1.002 | <0.001 | 0.002 | 1.002 | 0.999–1.005 | 0.15 | |
Density | 0.015 | 1.015 | 1.011–1.019 | <0.001 | 0.015 | 1.015 | 1.009–1.020 | <0.001 |
3D, three-dimensional; CI, confidence interval; CT, computed tomography; OR, odds ratio; SSNs, subsolid nodules.
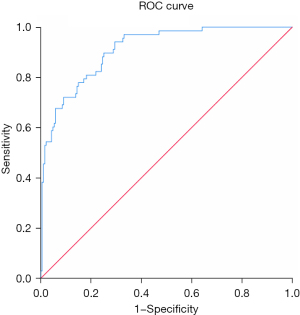
Discussion
Significant differences were found in the quantitative parameters of SSNs between the inspiratory CT and the expiratory CT. The change ratio in the long axis diameter of SSNs was the lowest, while the change ratio of the volume was the highest. The changes in the quantitative parameters were associated with different density types of SSNs, and conversely, they remained independent of nodule size. All the quantitative parameters of SSNs on inspiratory CT in the changed group were greater than those in the unchanged group. In the present study, lung density, long axis diameter, short axis diameter, surface area and density of SSNs on inspiratory CT were independent indicators to predict whether SSN changes with respiratory phase.
There were significant differences in lung density and lung volume between inspiratory and expiratory CT in this study. A previous study has confirmed that blood significantly influences lung density/weight in pigs (19). When compared with bloodless conditions in pigs, CT scans obtained in vivo consistently overestimate lung density due to the inclusion of pulmonary blood. When the lungs inflate (inspiratory phase), the small blood vessels of the lungs are compressed, causing blood draw from the pulmonary circulatory system (20). At the same time, the alveoli expand during inspiration, and increased air in the alveoli reduces the lung density. In addition, the ribs and sternum are raised during inhalation, while the lower margin of the ribs is deflected outwards. This action increases the anterior-to-posterior and the left-to-right diameters of the thoracic cavity. The diaphragmatic muscles move downward, thereby increasing the vertical diameter of the thoracic cavity. As a result, when inhaling, the chest expands and the volume of the lungs increases. Conversely, during exhalation, the thoracic cavity and lung volume decrease due to the retraction force of lung (21). In this study, the volume of the lungs increased and the density of the lungs decreased from the end of exhalation to the end of inhalation, which is consistent with normal physiological processes and similar to previously reported results (9).
According to the guidelines for managing pulmonary nodules, size is one of the most critical factors in determining management strategies. In addition, Qi et al. included 316 pulmonary SSNs and showed significant differences between non-invasive adenocarcinomas and invasive adenocarcinomas regarding mean diameter and volume (22). In this study, there were statistical differences in the diameter and volume of SSNs in different respiratory phases. The diameter and volume of SSNs decreased significantly in the expiratory phase, indicating that pulmonary SSNs were susceptible to the influence of lung inflation status. The pathological basis of SSN includes alveolar gas reduction, cell number increase, alveolar epithelial cell proliferation, alveolar septum thickening, and partial fluid filling in the terminal air sac (23,24). Additionally, the alveoli do not completely collapse, making SSN more susceptible to deformation from external forces such as pulling of adjacent lung tissue, leading to changes in size and volume. Change in respiratory phase leads to variations in quantitative parameters of pulmonary nodules, which may impact the assessment of nodule growth during follow-up and the calculation of volume doubling time (VDT). This has significant implications for the accurate management and treatment of pulmonary nodules. We found that the change ratio of the long axis diameter was the smallest, and there was no statistical difference observed in the long axis diameter(E-I)/I of SSNs among different density types, different sizes and different locations. Therefore, the long axis diameter showed a good stability to the change of respiratory phase and can be recommended as the best observation index in the follow-up of pulmonary SSNs.
In this study, the volume of SSNs showed the most variation with respiratory phase and was relatively unstable. Some scholars have explored the factors affecting the variability of nodule volume between different CT scans. As Moser et al.’s research showed, the change ratio of nodule volume between the end of inspiration and the end of exhalation was 7.5% (25). However, Bartlett et al.’s study found that after two chest CT examinations in the same day, the variation ratio of nodule volume reached 15.5% (8). In contrast, the results of this study showed the change ratio in nodule volume of 20.21%, which was higher than the results of the two studies above. This may be because the nodules included in their study were solid nodules and semi-automatic segmentation was adopted, and solid nodules were less susceptible to variation in respiration. In the present study, SSNs were included and manually segmented by radiologists. Fan et al.’s previous preliminary study compared the difference of the volume in pGGN between paired inspiratory and expiratory CT, and the results showed that the change ratio of nodule volume was 19.8% (9), which was close to 20.21% in this study. It is speculated that ground glass component in the nodules is more susceptible to the degree of lung inflation than solid component.
The changes in most quantitative parameters (size parameters) of pGGNs were greater than that of PSNs in this study. This may be attributed to the presence of solid components in PSNs. Pathologically, pGGNs are not associated with alveolar structure destruction and have more internal gas content, which can be expelled during exhalation. On the other hand, solid components in PSNs result from infiltration, scar formation, alveolar collapse, and interstitial hyperplasia of lung adenocarcinoma (23,26-28), there is no air can be expelled on exhalation. In addition, the cell density of pGGN is lower than that of PSN, and it is more likely to deform with changes in lung inflation. We found that the changes in all quantitative parameters were independent of nodule size (≤10 and >10 mm). This suggests that a 10-mm threshold may be unnecessary for SSNs identified during screening. Previous studies have indicated that the change ratio of density was greater in the lower lobe compared to the upper lobe on dual respiratory phase imaging (29-31). Consistent with these findings, it has been shown that the density of SSNs in the lower lobe was susceptible to respiratory phase in our study.
A recent study has shown that peritumoral parenchyma has important biological characteristics in terms of cell migration, inflammatory response and vascularization, which is of great value for clinical evaluation of lesion invasion behavior (32). Such peritumoral microenvironment leads to a close relationship between the edge of invasive pulmonary nodules and the surrounding pulmonary parenchyma. Therefore, we speculated that during respiratory movement, the pulmonary parenchyma around the nodule generates traction on the nodule, resulting in changes in the nodule. In addition, a previous study used the morphological changes of lung tumors during respiration to predict their histological subtypes (33), indicating that the morphological changes of lung nodules between the dual respiration phases are related to the pathological properties of nodules. Therefore, we speculated that the changes in the quantitative parameters of SSNs due to respiration could reflect the pathological properties of SSNs on the basis of reflecting the morphological changes of nodules. We found the pulmonary SSNs with larger long axis diameter, larger short axis diameter, higher density and smaller surface area were more susceptible to respiratory phase. We will explore the relationship between the change ratio in quantitative parameters of SSNs during respiration and pathological types in the future.
There are some limitations to this study. First, this is a retrospective study and this may cause a selection bias. Second, we only evaluated the end-inspiration and end-expiration time points in our study, and there was a lack of research on the influence of different respiratory stages on quantitative parameters of lung nodules. Four-dimensional (4D) CT is a combination of conventional CT images and time to form dynamic images, which can dynamically observe the position, shape and size of tumors during respiratory. And it is widely used in radiotherapy (34). In future studies, 4D respiratory gating CT technique should be recommended to obtain accurate results for changes in quantitative parameters at any time point in the respiratory cycle. Third, the changed group and unchanged group were divided according to the changes in diameter and solid components of SSNs, and the change in volume was not taken as one of the criteria for grouping, which may lead to deviations in grouping. However, the grouping criteria in this study were based on the reference of other scholars’ research and practical methods in clinical work. Finally, a radiologist with four years of expertise manually segmented the data to determine the quantitative parameters of the SSNs; however, a portion of nodules were repeatedly segmented by another radiologist with eight years of experience and the consensus on segmentation was good by two radiologists. We will consider using semi-automatic or fully automatic segmentation in the future to improve repeatability and stability.
Conclusions
In conclusion, respiratory phase had the greatest effect on the volume of pulmonary SSNs and the least effect on the long axis diameter. Therefore, the influence of respiratory phase should be fully considered when comparing the changes of SSNs volume in the follow-up of LDCT scan. Most quantitative parameters of pGGNs were more susceptible to respiratory phase than those of PSNs. During follow-up, LDCT scan in different respiratory phases may interfere with the judgment of the growth of pulmonary SSNs.
Acknowledgments
We thank the investigators and participants at the investigative sites for their support during the conduct of the study.
Footnote
Reporting Checklist: The authors have completed the TRIPOD reporting checklist. Available at https://jtd.amegroups.com/article/view/10.21037/jtd-24-1440/rc
Data Sharing Statement: Available at https://jtd.amegroups.com/article/view/10.21037/jtd-24-1440/dss
Peer Review File: Available at https://jtd.amegroups.com/article/view/10.21037/jtd-24-1440/prf
Funding: This work was supported by
Conflicts of Interest: All authors have completed the ICMJE uniform disclosure form (available at https://jtd.amegroups.com/article/view/10.21037/jtd-24-1440/coif). W.T. reports receiving funding from National Natural Science Foundation of China and Shanghai Sailing Program to this manuscript. L.F. reports receiving funding from the National Natural Science Foundation of China, the program of Science and Technology Commission of Shanghai Municipality, National Key R&D Program of China, Construction of CT Standardized Database for Chronic Obstructive Pulmonary Disease, and Clinical Innovation Project of Shanghai Changzheng Hospital to this manuscript. S.L. reports receiving funding from Special Project for Promoting High-Quality Development of Industries in Shanghai, 2022–2023 (Artificial Intelligence Topic) to this manuscript. The other authors have no conflicts of interest to declare.
Ethical Statement: The authors are accountable for all aspects of the work in ensuring that questions related to the accuracy or integrity of any part of the work are appropriately investigated and resolved. The study was conducted in accordance with the Declaration of Helsinki (as revised in 2013). The study was approved by the institutional review board of Second Affiliated Hospital of PLA Naval Medical University (No. 2018SL028). Written informed consent was obtained from all individual participants.
Open Access Statement: This is an Open Access article distributed in accordance with the Creative Commons Attribution-NonCommercial-NoDerivs 4.0 International License (CC BY-NC-ND 4.0), which permits the non-commercial replication and distribution of the article with the strict proviso that no changes or edits are made and the original work is properly cited (including links to both the formal publication through the relevant DOI and the license). See: https://creativecommons.org/licenses/by-nc-nd/4.0/.
References
- Yanagawa M, Johkoh T, Noguchi M, et al. Radiological prediction of tumor invasiveness of lung adenocarcinoma on thin-section CT. Medicine (Baltimore) 2017;96:e6331. [Crossref] [PubMed]
- Chen H, Kim AW, Hsin M, et al. The 2023 American Association for Thoracic Surgery (AATS) Expert Consensus Document: Management of subsolid lung nodules. J Thorac Cardiovasc Surg 2024;168:631-647.e11. [Crossref] [PubMed]
- Bankier AA, MacMahon H, Colby T, et al. Fleischner Society: Glossary of Terms for Thoracic Imaging. Radiology 2024;310:e232558. [Crossref] [PubMed]
- Wu FZ, Huang YL, Wu CC, et al. Assessment of Selection Criteria for Low-Dose Lung Screening CT Among Asian Ethnic Groups in Taiwan: From Mass Screening to Specific Risk-Based Screening for Non-Smoker Lung Cancer. Clin Lung Cancer 2016;17:e45-56. [Crossref] [PubMed]
- Lin KF, Wu HF, Huang WC, et al. Propensity score analysis of lung cancer risk in a population with high prevalence of non-smoking related lung cancer. BMC Pulm Med 2017;17:120. [Crossref] [PubMed]
- Chen PA, Huang EP, Shih LY, et al. Qualitative CT Criterion for Subsolid Nodule Subclassification: Improving Interobserver Agreement and Pathologic Correlation in the Adenocarcinoma Spectrum. Acad Radiol 2018;25:1439-45. [Crossref] [PubMed]
- MacMahon H, Naidich DP, Goo JM, et al. Guidelines for Management of Incidental Pulmonary Nodules Detected on CT Images: From the Fleischner Society 2017. Radiology 2017;284:228-43. [Crossref] [PubMed]
- Bartlett EC, Kemp SV, Rawal B, et al. Defining growth in small pulmonary nodules using volumetry: results from a “coffee-break” CT study and implications for current nodule management guidelines. Eur Radiol 2022;32:1912-20. [Crossref] [PubMed]
- Fan L, Li Q, Tu W, et al. Changes in quantitative parameters of pulmonary nonsolid nodule induced by lung inflation according to paired inspiratory and expiratory computed tomography imaging. Eur Radiol 2019;29:4333-40. [Crossref] [PubMed]
- Gheysens G, De Wever W, Cockmartin L, et al. Detection of pulmonary nodules with scoutless fixed-dose ultra-low-dose CT: a prospective study. Eur Radiol 2022;32:4437-45. [Crossref] [PubMed]
- Du Y, Li Q, Sidorenkov G, et al. Computed Tomography Screening for Early Lung Cancer, COPD and Cardiovascular Disease in Shanghai: Rationale and Design of a Population-based Comparative Study. Acad Radiol 2021;28:36-45. [Crossref] [PubMed]
- Kishi K, Homma S, Kurosaki A, et al. Small lung tumors with the size of 1cm or less in diameter: clinical, radiological, and histopathological characteristics. Lung Cancer 2004;44:43-51. [Crossref] [PubMed]
- Rami-Porta R, Nishimura KK, Giroux DJ, et al. The International Association for the Study of Lung Cancer Lung Cancer Staging Project: Proposals for Revision of the TNM Stage Groups in the Forthcoming (Ninth) Edition of the TNM Classification for Lung Cancer. J Thorac Oncol 2024;19:1007-27. [Crossref] [PubMed]
- Liu J, Qi L, Wang Y, et al. Diagnostic performance of a deep learning-based method in differentiating malignant from benign subcentimeter (≤10 mm) solid pulmonary nodules. J Thorac Dis 2023;15:5475-84. [Crossref] [PubMed]
- Cui SL, Qi LL, Liu JN, et al. A prediction model based on computed tomography characteristics for identifying malignant from benign sub-centimeter solid pulmonary nodules. J Thorac Dis 2024;16:4238-49. [Crossref] [PubMed]
- Cho J, Kim ES, Kim SJ, et al. Long-Term Follow-up of Small Pulmonary Ground-Glass Nodules Stable for 3 Years: Implications of the Proper Follow-up Period and Risk Factors for Subsequent Growth. J Thorac Oncol 2016;11:1453-9. [Crossref] [PubMed]
- Lee JH, Lim WH, Hong JH, et al. Growth and Clinical Impact of 6-mm or Larger Subsolid Nodules after 5 Years of Stability at Chest CT. Radiology 2020;295:448-55. [Crossref] [PubMed]
- Kim BG, Nam H, Hwang I, et al. The Growth of Screening-Detected Pure Ground-Glass Nodules Following 10 Years of Stability. Chest 2024; Epub ahead of print. [Crossref]
- Protti A, Iapichino GE, Milesi M, et al. Validation of computed tomography for measuring lung weight. Intensive Care Med Exp 2014;2:31. [Crossref] [PubMed]
- Miller M. Pulmonary Physiology and Pathophysiology, An Integrated, Case-Based Approach. Chest 2004;126:1393.
- Schwartzstein RM, Parker MJ. Respiratory Physiology: A Clinical Approach. Lippincott Williams & Wilkins; 2015.
- Qi L, Lu W, Yang L, et al. Qualitative and quantitative imaging features of pulmonary subsolid nodules: differentiating invasive adenocarcinoma from minimally invasive adenocarcinoma and preinvasive lesions. J Thorac Dis 2019;11:4835-46. [Crossref] [PubMed]
- Succony L, Rassl DM, Barker AP, et al. Adenocarcinoma spectrum lesions of the lung: Detection, pathology and treatment strategies. Cancer Treat Rev 2021;99:102237. [Crossref] [PubMed]
- Travis WD, Garg K, Franklin WA, et al. Evolving concepts in the pathology and computed tomography imaging of lung adenocarcinoma and bronchioloalveolar carcinoma. J Clin Oncol 2005;23:3279-87. [Crossref] [PubMed]
- Moser JB, Mak SM, McNulty WH, et al. The influence of inspiratory effort and emphysema on pulmonary nodule volumetry reproducibility. Clin Radiol 2017;72:925-9. [Crossref] [PubMed]
- Koike H, Ashizawa K, Tsutsui S, et al. Differentiation Between Heterogeneous GGN and Part-Solid Nodule Using 2 D Grayscale Histogram Analysis of Thin-Section CT Image. Clin Lung Cancer 2023;24:541-50. [Crossref] [PubMed]
- Wang Y, Lyu D, Zhou T, et al. Multivariate analysis based on the maximum standard unit value of (18)F-fluorodeoxyglucose positron emission tomography/computed tomography and computed tomography features for preoperative predicting of visceral pleural invasion in patients with subpleural clinical stage IA peripheral lung adenocarcinoma. Diagn Interv Radiol 2023;29:379-89. [Crossref] [PubMed]
- Wu S, Fan X, Li X, et al. Clinical and non-contrast computed tomography characteristics and disease development in patients with benign pulmonary subsolid nodules with a solid component ≤ 5 mm. Insights Imaging 2024;15:6. [Crossref] [PubMed]
- Furukawa BS, Pastis NJ, Tanner NT, et al. Comparing Pulmonary Nodule Location During Electromagnetic Bronchoscopy With Predicted Location on the Basis of Two Virtual Airway Maps at Different Phases of Respiration. Chest 2018;153:181-6. [Crossref] [PubMed]
- Chen A, Pastis N, Furukawa B, et al. The effect of respiratory motion on pulmonary nodule location during electromagnetic navigation bronchoscopy. Chest 2015;147:1275-81. [Crossref] [PubMed]
- Shen G, Wang YJ, Sheng HG, et al. Double CT imaging can measure the respiratory movement of small pulmonary tumors during stereotactic ablative radiotherapy. J Thorac Dis 2012;4:131-40. [Crossref] [PubMed]
- Kim N, Kim HK, Lee K, et al. Single-cell RNA sequencing demonstrates the molecular and cellular reprogramming of metastatic lung adenocarcinoma. Nat Commun 2020;11:2285. [Crossref] [PubMed]
- Lao Y, David J, Mirhadi A, et al. Discriminating lung adenocarcinoma from lung squamous cell carcinoma using respiration-induced tumor shape changes. Phys Med Biol 2018;63:215027. [Crossref] [PubMed]
- Gong YJ, Li YK, Zhou R, et al. A novel approach for estimating lung tumor motion based on dynamic features in 4D-CT. Comput Med Imaging Graph 2024;115:102385. [Crossref] [PubMed]