Predicting the clinical prognosis of non-small cell lung cancer patients by predicting ALOX5 expression: a radiomics model
Highlight box
Key findings
• A comprehensive prediction model, based on Contrast-enhanced computed tomography radiomics and arachidonic acid 5-lipoxygenase (ALOX5) expression, can effectively predict clinical prognosis in patients with non-small cell lung cancer (NSCLC).
What is known and what is new?
• ALOX5 can be used as a predictive biomarker for cancer.
• ALOX5 is combined with radiomics to predict the prognosis of NSCLC.
What is the implication, and what should change now?
• Further clinical validation is needed.
Introduction
Lung cancer is the leading cause of cancer-related deaths worldwide. The projected number of deaths due to lung cancer is expected to range between 1.1 and 1.6 million for men, and between 1.2 and 1.8 million for women by 2040 (1). The most common type of lung cancer is non-small cell lung cancer (NSCLC), with a dismal prognosis in patients who are in the late or final stages. Approximately 6% of patients survive for five years (2). In 2020, NSCLC had the highest mortality rate, with 1.8 million deaths, primarily as a result of late diagnosis (3). NSCLC includes lung adenocarcinoma (LUAD) and lung squamous cell carcinoma (LSCC). To accurately identify the histological subtypes of patients, invasive procedures such as bronchoscopy, transbronchial needle aspiration, transthoracic fine needle aspiration (4), and liquid biopsy technology (5) are required. However, these methods can lead to complications, such as bleeding and pneumothorax.
Arachidonic acid 5-lipoxygenase (ALOX5) is a member of the lipoxygenase (LOX) family. In mammalian cells, six members of the ALOX family are believed to selectively regulate ferroptosis (6). ALOX5 is closely linked to tumors; Melstrom et al. (7) found that inhibiting 5-LOX in an in vivo colon cancer xenograft model suppressed tumor growth. A study by Tang et al. (8) indicated that the overexpression of ALOX5 activates gastric cancer cells. Zhou et al. (9) reported that ALOX5 mediates the growth and migration of breast cancer cells. Currently, the detection of ALOX5 expression levels is limited to peripheral blood cytokines, fresh tissue samples, or paraffin tissue samples. These methods face challenges in sample collection and are influenced by the operator and antibodies. Therefore, the search for a novel, inexpensive, non-invasive biomarker for the diagnosis of NSCLC is crucial.
The initial screening of patients with NSCLC is typically conducted using radiological methods (10). Radiomics is a high-throughput “image sequencing” technique with the capacity and parameters to record various different images (11,12). The advantages of radiomics include its non-invasive, inexpensive, and efficient nature. D’Arnese et al. (13) calculated the radiomics features of patients’ positron emission tomography/computed tomography (PET/CT) scans and employed machine learning algorithms to characterize cancer. Le et al. (14) used PET/CT to create a machine learning-based model that can correctly predict KRAS and EGFR mutations in patients with NSCLC.
We hypothesize that the molecular characteristics driving different tumor subtypes are also reflected in their imaging features. To date, no research has investigated radiomics models for predicting the expression levels of ALOX5. Our study makes a ground-breaking suggestion to use contrast-enhanced computed tomography (CECT) radiomics technology to non-invasively forecast the expression of ALOX5 messenger RNA (mRNA) in lung cancer tissues. To investigate the potential molecular pathways driving ALOX5 expression and its association with the immunological microenvironment, we also combine bioinformatics analysis. We present this article in accordance with the TRIPOD reporting checklist (available at https://jtd.amegroups.com/article/view/10.21037/jtd-24-1596/rc).
Methods
Data and image sources
Medical imaging data were sourced from the NSCLC Radiogenomics dataset of The Cancer Imaging Archive (TCIA) database (NSCLC Radiogenomics-TCIA Public Access-Cancer Imaging Archive Wiki). Transcriptome sequencing data, along with clinical and follow-up data, were downloaded from the Gene Expression Omnibus (GEO) database (https://www.ncbi.nlm.nih.gov/geo/query/acc.cgi?acc=GSE103584). The cutoff value was determined by using the “survminer” R program. Figure 1 outlines the research process for patients with NSCLC. In addition, we downloaded the transcriptome sequencing data of the LUAD dataset from The Cancer Genome Atlas (TCGA) (https://portal.gdc.cancer.gov), and the bioinformatics analysis was consistent with that of NSCLC dataset. The study was conducted in accordance with the Declaration of Helsinki (as revised in 2013).
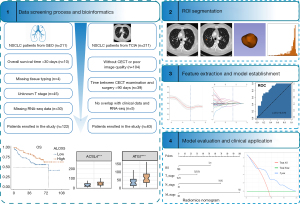
Survival analysis
To demonstrate the differences in survival rates between several groups, the Kaplan-Meier (KM) survival curve was used. The effect of ALOX5 (groups with high and low expressions) on patient prognosis was evaluated across the various subgroups of each covariate using exploratory subgroup analysis and univariate Cox regression. The main variable, ALOX5, was used to measure the differences in the ferroptosis-related genes (15) between the high- and low-expression subgroups. The Wilcoxon test was used to assess these differences. The results are presented in a boxplot graph.
Correlation analysis
The link between ALOX5 and the clinical traits of the malignancies was examined using Spearman’s rank correlation coefficient. Wilcoxon test was used to analyze the difference of ferroptosis-related genes among the different ALOX5 groups. NSCLC sample gene expression matrices were submitted to the CIBERSORTx database (https://cibersortx.stanford.edu/). The infiltration of immune cells in each sample was then calculated. Using the “corrplot” R package, we investigated the link between the expression of ALOX5 and the level of immune cell infiltration.
Screening and model construction of radiomics features
We used the R package “survminer” to determine the cutoff value for ALOX5 expression levels, separating the patients into groups with high- and low-expression. Data were collected from GEO and TCIA image databases. The radiomics feature values were standardized, with 107 features extracted using the pyradiomics package. Feature selection was performed using repeated least absolute shrinkage and selection operator (LASSO) screening. Utilizing the R package glmnet, we conducted 1,000 LASSO regression feature screenings and selected features that appeared more than 800 times during these 1,000 screenings. Employing the stats package’s glm function and R’s caret package, we established binary classification models to predict ALOX5 gene expression, using both logistic regression (LR) and Support Vector Machine (SVM) algorithms. Two doctors defined regions of interest contours, from which radiomics characteristics were extracted. The consistency of these contours was evaluated using the intraclass correlation coefficient (ICC). A random number table was used to select 20 samples at random after a single physician outlined all the cases. A second doctor then outlined these samples to extract radiomics features to check for consistency. The regions of interest contours was decided blinded to clinical information and based on consensus. These two doctors were blinded to both predictor variables and patient outcomes.
Radiomics model evaluation and consistency assessment
We assessed the efficacy of the radiomics model using a 5-fold internal cross-validation. By depicting the decision curve analysis (DCA) curve, the clinical benefit level of the radiomics prediction model was demonstrated. The likelihood of predicting gene expression levels using the radiomics score (RS) was compared using the Wilcoxon test. This comparison aimed to discern differences between high- and low- expression groups. Additionally, the model’s area under the curve (AUC) values before and after cross-validation were compared using the DeLong test.
TCIA-GEO clinical intersection samples and survival analysis
The probability value (RS) from the radiomics model, which had a higher AUC value, was integrated with the clinical data to obtain NSCLC patient data that included the RS. We calculated the cutoff value for RS (dichotomous variables), thereby dividing the patients into high- and low-expression groups.
Construction and evaluation of the Cox regression model
Using the Akaike Information Criterion (AIC) as the criterion, feature selection was performed on the included variables. A model was then constructed by selecting the variables with the smallest AIC values. Nomograms were created using the Cox regression analysis for the 1-, 3-, and 5-year survival probability. Additionally, we developed a time-dependent receiver operating characteristic (ROC) curve, a model calibration chart, and DCA curve.
Statistical analysis
The standard deviation and mean were displayed for each clinical quantitative indicator. To determine whether the differences between each indicator and outcomes were significant, the independent sample t-test was used to determine whether the quantitative data followed a normal distribution. Otherwise, Mann-Whitney U test was utilized. The log-rank test was used to determine the significance of the survival rate differences between groups. The link between the expression of ALOX5 and the other factors was investigated using a likelihood ratio test. The Hosmer-Lemeshow goodness-of-fit test was used to assess the calibration of the radiomics prediction model. A 95% confidence interval and a P value lower than 0.05 were regarded as statistically significant. R program was utilized to run all statistical analyses (version 4.1.0).
Results
Patient characteristics
Overall, 122 patients were chosen from the GEO database for subsequent bioinformatics analysis, and 63 patients were selected from the TCIA database for radiomics analysis. To increase the credibility of the study, 320 patients were chosen from the TCGA-LUAD database for subsequent bioinformatics analysis, both datasets were validated simultaneously. There were two groups of patients with 122 NSCLC included in the survival analysis: one group with high ALOX5 expression of (n=56), while the other had low expression (n=66), based on a cutoff value of 3.5234. Table 1 displays the clinical data for patients with NSCLC. There was no statistically significant difference in the age distribution between the groups with high- and low-expression levels of ALOX5 (P=0.62). The clinical information of the LUAD dataset is presented in Table S1.
Table 1
Variables | Total (n=122) | Low (n=66) | High (n=56) | P |
---|---|---|---|---|
Age | 0.62 | |||
<66 years | 44 [36] | 22 [33] | 22 [39] | |
≥66 years | 78 [64] | 44 [67] | 34 [61] | |
Gender | >0.99 | |||
Female | 33 [27] | 18 [27] | 15 [27] | |
Male | 89 [73] | 48 [73] | 41 [73] | |
Histology | 0.002 | |||
Adenocarcinoma | 92 [75] | 42 [64] | 50 [89] | |
Squamous cell carcinoma | 30 [25] | 24 [36] | 6 [11] | |
Smoking status | 0.28 | |||
Nonsmoker | 20 [16] | 13 [20] | 7 [12] | |
Current | 24 [20] | 15 [23] | 9 [16] | |
Former | 78 [64] | 38 [58] | 40 [71] | |
T stage | 0.24 | |||
Tis/T1 | 56 [46] | 34 [52] | 22 [39] | |
T2/T3/T4 | 66 [54] | 32 [48] | 34 [61] | |
N stage | 0.66 | |||
N0 | 97 [80] | 51 [77] | 46 [82] | |
N1/N2 | 25 [20] | 15 [23] | 10 [18] | |
M stage | 0.66 | |||
M0 | 117 [96] | 64 [97] | 53 [95] | |
M1 | 5 [4] | 2 [3] | 3 [5] | |
KRAS mutation status | 0.042 | |||
Mutant | 23 [19] | 8 [12] | 15 [27] | |
Unknown | 27 [22] | 19 [29] | 8 [14] | |
Wildtype | 72 [59] | 39 [59] | 33 [59] | |
EGFR mutation status | 0.22 | |||
Mutant | 18 [15] | 8 [12] | 10 [18] | |
Unknown | 28 [23] | 19 [29] | 9 [16] | |
Wildtype | 76 [62] | 39 [59] | 37 [66] | |
Radiotherapy | 0.60 | |||
No | 108 [89] | 57 [86] | 51 [91] | |
Yes | 14 [11] | 9 [14] | 5 [9] | |
Chemotherapy | 0.42 | |||
No | 86 [70] | 44 [67] | 42 [75] | |
Yes | 36 [30] | 22 [33] | 14 [25] |
Data are presented as n [%].
High ALOX5 predicts better overall survival (OS)
In NSCLC, the findings showed that the tumors expressed significantly lower ALOX5 than normal tissues (P<0.001) (Figure 2A). The same results were observed in LUAD (Figure S1A). According to the KM curve, high ALOX5 expression was generally associated with improved OS, with a P value of 0.06 (Figure 2B). Low ALOX5 expression predicts lower OS in patients with LUAD (Figure S1B). OS was protected by high ALOX5 expression in univariate analysis [hazard ratio (HR) =0.541, 95% confidence interval (CI): 0.282–1.036, P=0.06]. After adjusting for multiple factors, both high ALOX5 expression (HR =0.453, 95% CI: 0.213–0.963, P=0.04) and chemotherapy (HR =0.216, 95% CI: 0.071–0.653, P=0.007) emerged as statistically significant protective factors against OS (Figure 2C). We also observed similar results in LUAD (Figure S1C).
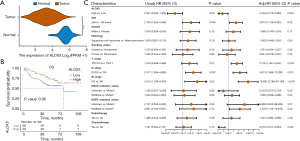
In subgroup analysis, for the subgroups aged <66 and ≥66 years, elevated ALOX5 levels provided OS with protection, and the interaction test had a P value of 0.97. We conclude that the effect of ALOX5 on OS is consistent across different age groups (Figure S2A). In the ALOX5-overexpression group, the ferroptosis-related genes, ACSL4 and ATG7, were expressed at considerably higher levels (P<0.001), while the expression of ACSL3, TP53, SAT1, GPX4, and other genes did not reveal any evident differences between the two groups (Figure 3A). Additionally, we observed similar results in LUAD (Figure S2B).
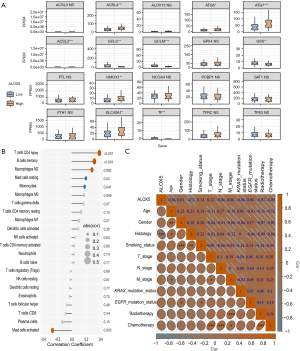
ALOX5 is associated with the degree of immune cell infiltration
We also examined the infiltration of immune cells in NSCLC and discovered that ALOX5 and the amount of T cell CD4 naive, memory B cells, macrophages M2, and resting mast cells infiltration were strongly positively associated (Figure 3B). The correlation heatmap indicated a negative correlation (P<0.001) between the ALOX5 expression and histology in NSCLC (Figure 3C).
Screening of radiomics features and establishment of models
Using a cutoff value of 3.5234 for ALOX5 expression levels, patients were divided into groups based on their expression levels. The results of the LASSO regression feature screening are displayed in Figure 4A,4B. The first eight features shown in Figure 4C were selected as the radiomics features for this screening. Table S2 provides the regression coefficients for these features in the LR model. The importance of the selected features in the LR model is illustrated in Figure 4D, while the importance in the SVM model is presented in Figure S3A.
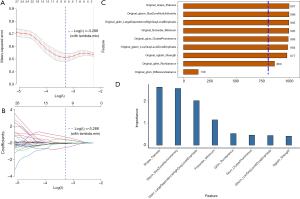
All of the radiomics features in the model had ICCs that were higher than 0.9, suggesting suitable consistency among these radiomics features (Table 2). Since the AUC score for the LR model was better than that for the SVM model, the predicted value from the LR model was chosen for subsequent analyses.
Table 2
Features | x |
---|---|
Original_shape_Flatness | 0.900690004 |
Original_glszm_SizeZoneNonUniformity | 0.958312035 |
Original_firstorder_Minimum | 0.982934911 |
Original_gldm_LargeDependenceHighGrayLevelEmphasis | 0.972177477 |
Original_glcm_ClusterProminence | 0.966206556 |
Original_glszm_LowGrayLevelZoneEmphasis | 0.900690799 |
Original_ngtdm_Strength | 0.94230887 |
Original_glrlm_RunVariance | 0.912802954 |
ICC, intraclass correlation coefficient.
Radiomics model displayed good performance
The AUC value for the LR model was 0.819, as shown in the ROC curve in Figure 5A. After 5-fold internal cross-validation, the AUC was 0.783 (Figure 5B). The LR radiomics model demonstrated good predictive performance, with an AUC-precision-recall (PR) curve value of 0.782 (Figure 5C). The Hosmer-Lemeshow goodness-of-fit test for the calibration curve indicated that the radiomics prediction model showed good consistency between the predicted probability of gene overexpression and the actual value (P>0.05) (Figure 5D). DCA demonstrated the strong clinical value (Figure 5E) of the model. RS distribution in the high- and low-ALOX5 expression groups was significantly different from one another (P<0.05), and RS values were higher in the group with high ALOX5 expression (Figure 5F).
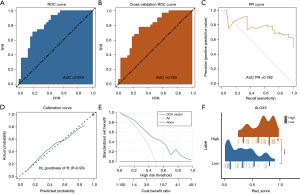
The AUC score of the SVM model was 0.810. After 5-fold internal cross-validation, the AUC was 0.763 (Figure S3B,S3C). The calibration curve is depicted in Figure S3D, and the DCA curve in Figure S3E. Figure S3F illustrates the variance in the RS distribution across the groups with high and low ALOX5 expression. Similarly, in the SVM model, the high ALOX5 expression group displayed a higher RS. Before cross-validation, the DeLong test neither found any evident differences between the two models’ AUC values (P=0.63), nor were there significant differences in AUC values after cross-validation (P=0.81).
High RS is significantly associated with improved OS
The clinical characteristics data for the 63 TCIA-GEO clinical intersection samples are presented in Table S3. The cutoff value for RS is 0.4238. Based on RS, patients were divided into two groups: a group with high expression (n=36) and low expression (n=27). Upon incorporating these groups into survival analysis, the KM curve reveals a significant correlation between high RS and improved OS (P<0.05) (Figure 6A).
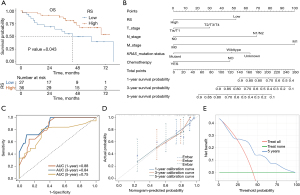
The prediction effect of the nomogram prediction model is good
Next, we utilized the GEO variables in conjunction with RS from the LR radiomics model to establish a COX nomogram prediction model (Figure 6B) and then evaluated this prediction model. The ROC curve depicted in Figure 6C demonstrates that the AUC values for the predictive ability of the model regarding patients’ OS at 1, 3, and 5 years are 0.88, 0.84, and 0.75, respectively. The calibration plot of the model indicates that the curves at various time points closely align with the diagonal, signifying low prediction error (Figure 6D). The 5-year DCA reveals that the model has high clinical utility within a threshold range of 0.25–0.9 (Figure 6E).
Discussion
This study innovatively proposes to use CT radiomics technology to non-destructively predict the mRNA expression of ALOX5 in NSCLC tissues, which has certain guiding significance for the judgment of prognosis and the selection of diagnosis and treatment methods. First, we analyzed and found that ALOX5 expression was closely related to NSCLC prognosis. Functional enrichment and immune cell infiltration analyses were performed to explore the molecular function of ALOX5 in NSCLC. We also used the LUAD dataset to validate the same analysis. Different datasets and different pathological subtypes showed similar conclusions: ALOX5 expression was closely related to prognosis, which makes our study more compelling. The radiomics phenotype showed a significant correlation with NSCLC’s OS. We extracted radiomics features from CT data and constructed a radiomics model using LR for predicting ALOX5 expression and then tested the model’s performance. The association between RS and ALOX5 expression was examined in the final step, and conducted survival analysis using the radiomics model. In the actual clinical diagnosis and treatment process, we usually use invasive tissue sampling, so in recent years, the research focus of prognostic markers has mostly incorporated the characteristics of “non-invasive”. The greatest advantage of radiomics prediction model is that it is non-invasive. The use of radiomics model to predict mRNA expression and prognosis of patients can bring inspiration for clinical application.
We analyzed 122 samples from the GEO database and found low ALOX5 expression in tumor tissues. High ALOX5 expression was identified as a protective factor against OS. A study by Wei et al. (16) suggests that ALOX5 is an immune-related gene potentially involved in lung cancer development. Another study by Miao et al. (17) indicated that ALOX5 is a differentially expressed gene related to ferroptosis in patients with LUSC, and it is closely related to its prognosis. According to Zhao et al. (18), normal lung tissue expresses ALOX5 at higher levels than NSCLC tissues and impacts the prognosis of patients with NSCLC, consistent with our findings. Li et al. (19) found that ALOX5 knockdown inhibits gefitinib resistance in NSCLC cells by inhibiting autophagy, potentially explaining the molecular action of ALOX5. A recent study (20) found that prognosis and immune cells in the tumor microenvironment are closely related. A positive link between ALOX5 and immune cells that infiltrate tumors was also revealed in our study. Bruni et al. (21) summarized numerous studies and found that longer lifespan is linked to cytotoxic T cells, memory T cells, Th1 cells, follicular helper T cells (TFH cells), and B cells. However, current research primarily focuses on the correlation between immune cells and tumor prognosis. A comprehensive evaluation involving multiple cells is still required.
The concept of was first introduced by the Dutch scholar Lambin’s team in 2012 (22). In our study, we extracted enhanced chest CT images from patients with NSCLC and used the LASSO algorithm to filter out the optimal feature subset. We employed a LR algorithm for the prediction model, achieving good accuracy. The calibration curve also indicated that the probabilities predicted by our radiomics model were consistent with the true values. There was a noticeable distinction in the distribution between high and low RS gene groups, confirming the efficacy of the model in distinguishing between high and low gene expression.
This study ultimately identified eight significant radiomics features; original_Shape_Flatness ranked the highest in importance, representing the original flatness of lesion morphology in patients with NSCLC. This could be connected to the lower flatness of lesion structures in patients with lung cancer. Among the eight prominent features, six were second- or higher-order texture features, emphasizing their critical role in reflecting expressions. Notably, feature extraction in this study relied on contrast-enhanced CT images, which when compared with standard plain CT scans, can more accurately capture valuable information such as the lesion’s edge, tumor blood supply, and internal structure.
The nomograms indicated that if a patient with T1N0M0 stage, KRAS mutation, or no chemotherapy had a score of approximately 47.5, they were at low risk, with a 5-year OS greater than 0.9. The model could intuitively predict outcomes. Zhou et al. (23) found a significant correlation between 12 CT radiological features and Ki-67 in lung cancer but did not establish a predictive model. Tian et al. (24) conducted deep learning on the imaging data of 939 patients with stage IV NSCLC and revealed that the clinical effects of immunotherapy can be predicted by radiomics, which can also predict high programmed cell death ligand 1 (PD-L1) expression in NSCLC. All these studies underscore the clinical potential of radiomics. Radiomics is a non-invasive, dynamic, and quantitative technique to reflect tumor characteristics. Our radiomics-based model exhibited good efficiency in predicting ALOX5 expression and could be valuable in guiding clinical prognosis prediction. Compared with traditional methods, our model reduces invasive procedures and simplifies the actual clinical workflow. For patients, non-invasive examinations are more easily accepted, and image data acquisition is more convenient.
There are some limitations in this study. First, we used a public database, and variations in data and images can be significant. Second, most cases included in the study were adenocarcinomas, which could introduce bias in data. Third, manually outlining the volume of interest (VOI) could lead to human bias. Although manual delineation of VOI is currently the most accurate among various lesion segmentation methods, it is still subject to some extent of subjective influence. Chemotherapy and immunotherapy are also important treatments for lung cancer. In the next part of our study, we will further analyze the significance of radiomics model in guiding the decision-making of chemotherapy and immunotherapy for lung cancer patients. There are many deep learning models, and recent advancements in deep learning, such as convolutional neural networks (CNNs) or transformer-based architectures, have shown significant improvements in processing and analyzing medical imaging data. Comparing different deep learning models and finally selecting the best model is also our next research direction.
Conclusions
According to the study findings, the prognosis for NSCLC is highly associated with the degree of ALOX5 expression. A comprehensive prediction model, based on CECT radiomics and clinical feature indicators, can effectively predict ALOX5 expression level in patients with NSCLC. This offers a new approach for the clinical evaluation of patient prognosis.
Acknowledgments
We thank Editage (https://app.editage.com) for editing the English language of this manuscript.
Footnote
Reporting Checklist: The authors have completed the TRIPOD reporting checklist. Available at https://jtd.amegroups.com/article/view/10.21037/jtd-24-1596/rc
Peer Review File: Available at https://jtd.amegroups.com/article/view/10.21037/jtd-24-1596/prf
Funding: This work was supported by
Conflicts of Interest: All authors have completed the ICMJE uniform disclosure form (available at https://jtd.amegroups.com/article/view/10.21037/jtd-24-1596/coif). The authors have no conflicts of interest to declare.
Ethical Statement: The authors are accountable for all aspects of the work in ensuring that questions related to the accuracy or integrity of any part of the work are appropriately investigated and resolved. The data in this investigation were acquired from publicly available databases, therefore, the study was exempt from ethical review. The study was conducted in accordance with the Declaration of Helsinki (as revised in 2013).
Open Access Statement: This is an Open Access article distributed in accordance with the Creative Commons Attribution-NonCommercial-NoDerivs 4.0 International License (CC BY-NC-ND 4.0), which permits the non-commercial replication and distribution of the article with the strict proviso that no changes or edits are made and the original work is properly cited (including links to both the formal publication through the relevant DOI and the license). See: https://creativecommons.org/licenses/by-nc-nd/4.0/.
References
- Wéber A, Morgan E, Vignat J, et al. Lung cancer mortality in the wake of the changing smoking epidemic: a descriptive study of the global burden in 2020 and 2040. BMJ Open 2023;13:e065303. [Crossref] [PubMed]
- Latimer KM, Mott TF. Lung cancer: diagnosis, treatment principles, and screening. Am Fam Physician 2015;91:250-6.
- Restrepo JC, Dueñas D, Corredor Z, et al. Advances in Genomic Data and Biomarkers: Revolutionizing NSCLC Diagnosis and Treatment. Cancers (Basel) 2023;15:3474. [Crossref] [PubMed]
- Planchard D, Popat S, Kerr K, et al. Metastatic non-small cell lung cancer: ESMO Clinical Practice Guidelines for diagnosis, treatment and follow-up. Ann Oncol 2018;29:iv192-237. [Crossref] [PubMed]
- Guibert N, Pradines A, Favre G, et al. Current and future applications of liquid biopsy in nonsmall cell lung cancer from early to advanced stages. Eur Respir Rev 2020;29:190052. [Crossref] [PubMed]
- Chen X, Kang R, Kroemer G, et al. Targeting ferroptosis in pancreatic cancer: a double-edged sword. Trends Cancer 2021;7:891-901. [Crossref] [PubMed]
- Melstrom LG, Bentrem DJ, Salabat MR, et al. Overexpression of 5-lipoxygenase in colon polyps and cancer and the effect of 5-LOX inhibitors in vitro and in a murine model. Clin Cancer Res 2008;14:6525-30. [Crossref] [PubMed]
- Tang J, Zhang C, Lin J, et al. ALOX5-5-HETE promotes gastric cancer growth and alleviates chemotherapy toxicity via MEK/ERK activation. Cancer Med 2021;10:5246-55. [Crossref] [PubMed]
- Zhou X, Jiang Y, Li Q, et al. Aberrant ALOX5 Activation Correlates with HER2 Status and Mediates Breast Cancer Biological Activities through Multiple Mechanisms. Biomed Res Int 2020;2020:1703531. [Crossref] [PubMed]
- Wood DE, Kazerooni EA, Baum SL, et al. Lung Cancer Screening, Version 3.2018, NCCN Clinical Practice Guidelines in Oncology. J Natl Compr Canc Netw 2018;16:412-41. [Crossref] [PubMed]
- Coroller TP, Grossmann P, Hou Y, et al. CT-based radiomic signature predicts distant metastasis in lung adenocarcinoma. Radiother Oncol 2015;114:345-50. [Crossref] [PubMed]
- Forghani R, Savadjiev P, Chatterjee A, et al. Radiomics and Artificial Intelligence for Biomarker and Prediction Model Development in Oncology. Comput Struct Biotechnol J 2019;17:995-1008. [Crossref] [PubMed]
- D'Arnese E, Donato GWD, Sozzo ED, et al. On the Automation of Radiomics-Based Identification and Characterization of NSCLC. IEEE J Biomed Health Inform 2022;26:2670-9. [Crossref] [PubMed]
- Le NQK, Kha QH, Nguyen VH, et al. Machine Learning-Based Radiomics Signatures for EGFR and KRAS Mutations Prediction in Non-Small-Cell Lung Cancer. Int J Mol Sci 2021;22:9254. [Crossref] [PubMed]
- Tang B, Yan R, Zhu J, et al. Integrative analysis of the molecular mechanisms, immunological features and immunotherapy response of ferroptosis regulators across 33 cancer types. Int J Biol Sci 2022;18:180-98. [Crossref] [PubMed]
- Wei X, Wang C, Feng H, et al. Effects of ALOX5, IL6R and SFTPD gene polymorphisms on the risk of lung cancer: A case-control study in China. Int Immunopharmacol 2020;79:106155. [Crossref] [PubMed]
- Miao TW, Yang DQ, Chen FY, et al. A ferroptosis-related gene signature for overall survival prediction and immune infiltration in lung squamous cell carcinoma. Biosci Rep 2022;42:BSR20212835. [Crossref] [PubMed]
- Zhao Q, Sun Z, Pan Y, et al. Role of ALOX5 in non-small cell lung cancer: A potential therapeutic target associated with immune cell infiltration. Zhong Nan Da Xue Xue Bao Yi Xue Ban 2023;48:311-22. [Crossref] [PubMed]
- Li W, Wang H, Yang Y, et al. Integrative Analysis of Proteome and Ubiquitylome Reveals Unique Features of Lysosomal and Endocytic Pathways in Gefitinib-Resistant Non-Small Cell Lung Cancer Cells. Proteomics 2018;18:e1700388. [Crossref] [PubMed]
- Backman M, Strell C, Lindberg A, et al. Spatial immunophenotyping of the tumour microenvironment in non-small cell lung cancer. Eur J Cancer 2023;185:40-52. [Crossref] [PubMed]
- Bruni D, Angell HK, Galon J. The immune contexture and Immunoscore in cancer prognosis and therapeutic efficacy. Nat Rev Cancer 2020;20:662-80. [Crossref] [PubMed]
- Lambin P, Rios-Velazquez E, Leijenaar R, et al. Radiomics: extracting more information from medical images using advanced feature analysis. Eur J Cancer 2012;48:441-6. [Crossref] [PubMed]
- Zhou B, Xu J, Tian Y, et al. Correlation between radiomic features based on contrast-enhanced computed tomography images and Ki-67 proliferation index in lung cancer: A preliminary study. Thorac Cancer 2018;9:1235-40. [Crossref] [PubMed]
- Tian P, He B, Mu W, et al. Assessing PD-L1 expression in non-small cell lung cancer and predicting responses to immune checkpoint inhibitors using deep learning on computed tomography images. Theranostics 2021;11:2098-107. [Crossref] [PubMed]