Development and validation of a nomogram for predicting pulmonary embolism in patients with pulmonary tuberculosis
Highlight box
Key findings
• In our study, the area under the receiver operating characteristic curve of the development cohort and validation cohort in the prediction model were 0.903 and 0.821. The calibration curves for the two cohorts showed that the probabilities predicted by the model were very close to the actual probabilities. This indicates that nomogram has significant clinical applications.
What is known and what is new?
• The clinical symptoms of pulmonary embolism (PE) are usually similar to those of respiratory diseases, which makes early clinical diagnosis difficult.
• Our predictive models can better predict the risk of PE in pulmonary tuberculosis (PTB) patients and aid in early clinical identification.
What is the implication, and what should change now?
• The clinical presentation of PTB is clinically difficult to identify early with PE, the developed nomogram performed well in both the predictive development cohort and the validation cohort. Patients with high model scores are more likely to develop PE, and early identification and timely intervention are prognostically helpful.
IntroductionOther Section
Pulmonary tuberculosis (PTB) is still an infectious disease that seriously endangers human health and it is also a major public health problem of global concern. PTB is an infectious condition caused by Mycobacterium tuberculosis (MTB) that affects the lungs and causes a severe cough, fever, and chest pain (1,2). Pulmonary embolism (PE) is a syndrome of clinical pathophysiology caused by the obstruction of the pulmonary artery and its branches due to exogenous or endogenous embolus dislodgment, resulting in the obstruction of pulmonary circulation (3). PE has no typical symptoms in the early stages. However, symptoms such as weakness, cough, chest pain, cold sweat, and dyspnea develop with disease progression (4). Due to the complexity and ambiguity of the clinical signs and symptoms, some symptoms of PE are similar to those of PTB and can be difficult to distinguish clinically. When the two are combined, the misdiagnosis rate is high, and the diagnosis is easily missed (5).
Many studies have investigated the risk factors for the formation of PE (6,7). Currently, clinical screening for PE is based on the Wells criteria and the revised Geneva score. The Wells criteria and Geneva score are clinical prediction tools commonly used to assess the probability of PE in patients presenting with symptoms suggestive of the condition (8,9). The Wells criteria assign points based on clinical features and risk factors, categorizing patients into low, moderate, or high probability of PE. Similarly, the Geneva score, available in original and revised forms, uses a scoring system derived from clinical variables to estimate PE likelihood. These tools guide clinicians in deciding the necessity of further diagnostic testing. When patients with PTB are comorbid with PE, it is easy to miss the diagnosis of PE. It is difficult for clinicians to accurately assess the probability of PE in patients with PTB based on clinical data. In addition, there are few studies on combined PE in PTB, and most of the available studies are case reports (10-12).
Therefore, we retrospectively analyzed clinical data from patients with PTB to construct and externally validate a predictive model for early identification of the risk of PE in patients with PTB. We hope that the results of this study will benefit clinical practice and improve patient prognosis. We present this article in accordance with the TRIPOD reporting checklist (available at https://jtd.amegroups.com/article/view/10.21037/jtd-24-1627/rc).
MethodsOther Section
Study design
The sample size for this study was estimated based on the event-per-variable (EPV) rule, which is widely used in regression analyses to ensure model stability and reliability. According to this guideline, we included 694 patients from Anhui Chest Hospital as a developmental cohort, including 84 tuberculosis patients with combined PE from September 2019 to December 2023. The validation cohort included 236 tuberculosis patients admitted to Fuyang Infectious Disease Clinical College of Anhui Medical University from May 2021 to July 2023, of whom 19 were complicated with PE. We conducted a retrospective analysis based on the clinical data of these two groups of patients. The data cutoff for the study was February 1, 2023. We developed a predictive model of PE in PTB patients based on a developmental cohort. Visualization of the model was done using diagrams. External validation was performed using data sets from another center.
The study was conducted in accordance with the Declaration of Helsinki (as revised in 2013). The study was approved by Ethics Committee of Anhui Chest Hospital (No. KJ2030-39) and Ethics Committee of Fuyang Infectious Disease Clinical College of Anhui Medical University (No. 20230814015). Informed consent was taken from all the patients.
Inclusion and exclusion criteria
Our inclusion criteria were patients diagnosed with PE by computed tomography pulmonary angiography (CTPA) and hospitalized. The diagnosis of PTB patients is based on clinical signs and symptoms, chest radiographs, sputum and/or bronchoalveolar lavage fluid MTB culture, acid-fast bacillus microscopy, and anti-tuberculosis treatment effect (13).
Exclusion criteria were: (I) the patients had more clinical data loss. (II) The suspected PE patients were not clearly diagnosed.
Data collection
Clinical data were collected, including comorbidities such as chronic obstructive pulmonary disease (COPD), diabetes, hypertension, and tumors. Clinical symptoms such as chest pain, cough, dyspnea, and hemoptysis; and laboratory test data, including platelet count, fibrinogen, D-dimer levels.
Statistical analysis
All statistical analyses were performed using R (4.1.2). Continuous variables were expressed as mean ± standard deviation and categorical variables were presented as numbers and percentages. Initially, the Kolmogorov-Smirnov test was used to check whether the variables were normally distributed. Subsequently, the Chi-squared test, Fisher’s exact test, Student’s t-test, and the Mann-Whitney U test were used for data analysis, as appropriate. Univariate and multivariate regression analyses were used to investigate risk factors in patients with PTB combined with PE.
Additionally, a nomogram was established to predict the probability of PE based on independent risk factors screened from the developmental cohort. In the development and validation cohorts, the performance of the prediction model was evaluated using the area under the receiver operating characteristic (ROC) curve (AUC), calibration curve analysis, and decision curve analysis (DCA). Statistical significance was set at P<0.05.
ResultsOther Section
Basic characteristics of patients
A total of 990 patients were included according to the study design. Among these patients, 60 were excluded, as they did not meet the study criteria. Ultimately, 930 patients were included in this analysis (Figure 1). The clinical data for the development and validation cohorts are described in Table 1 (14). The developmental cohort consisted of 694 patients with PTB, including 501 males (72.19%) and 193 females (27.81%), with an average age of 54.36±17.47 years. A total of 236 patients with tuberculosis were included in the validation cohort, including 181 males (76.69%) and 55 females (23.31%), with an average age of 58.75±19.04 years. Based on imaging results, 610 people in the development cohort had no detectable PE and 84 had detectable PE, with a positive rate of 12.1%. In the validation cohort 217 were without PE and 19 were examined for PE, with a positive rate of 8.05%.
Table 1
Clinical characteristics | Development cohort (n=694) | Validation cohort (n=236) | P value |
---|---|---|---|
Age (years) | 54.37±17.48 | 58.75±19.04 | 0.001 |
Sex | 0.18 | ||
Male | 501 (72.19) | 181 (76.69) | |
Female | 193 (27.81) | 55 (23.31) | |
Smoking | 0.47 | ||
No | 378 (54.47) | 135 (57.20) | |
Yes | 316 (45.53) | 101 (42.80) | |
COPD | 0.002 | ||
No | 546 (78.67) | 207 (87.71) | |
Yes | 148 (21.33) | 29 (12.29) | |
Hypertension | 0.040 | ||
No | 600 (86.46) | 191 (80.93) | |
Yes | 94 (13.54) | 45 (19.07) | |
Tumor | 0.20 | ||
No | 656 (94.52) | 228 (96.61) | |
Yes | 38 (5.48) | 8 (3.39) | |
Diabetes | 0.52 | ||
No | 597 (86.02) | 199 (84.32) | |
Yes | 97 (13.98) | 37 (15.68) | |
Cough | <0.001 | ||
No | 179 (25.79) | 26 (11.02) | |
Yes | 515 (74.21) | 210 (88.98) | |
Chest pain | 0.46 | ||
No | 640 (92.22) | 214 (90.68) | |
Yes | 54 (7.78) | 22 (9.32) | |
Hemoptysis | 0.058 | ||
No | 605 (87.18) | 194 (82.20) | |
Yes | 89 (12.82) | 42 (17.80) | |
Dyspnea | 0.24 | ||
No | 538 (77.52) | 174 (73.73) | |
Yes | 156 (22.48) | 62 (26.27) | |
Bedridden | 0.88 | ||
No | 669 (96.40) | 227 (96.19) | |
Yes | 25 (3.60) | 9 (3.81) | |
Surgery | 0.97 | ||
No | 682 (98.27) | 232 (98.31) | |
Yes | 12 (1.73) | 4 (1.69) | |
Hemoglobin (g/L) | 119.96±19.21 | 113.96±25.76 | <0.001 |
Platelet count (×109/L) | 239.10±92.23 | 272.83±108.08 | <0.001 |
Fibrinogen (g/L) | 3.83±1.42 | 4.38±1.31 | <0.001 |
D-dimer (mg/L) | 0.51±1.36 | 1.88±2.73 | <0.001 |
Pulmonary embolism | 0.09 | ||
No | 610 (87.90) | 217 (91.95) | |
Yes | 84 (12.10) | 19 (8.05) |
The table is adapted from Kong et al. (14). Reuse with exemptions from licenses of European Journal of Medical Research under the journal’s copyright policy CC BY-NC 4.0. Data are presented as mean ± standard deviation or n (%). COPD, chronic obstructive pulmonary disease.
Table 2 (14) shows the differences between the non-PE group (n=610) and the PE group (n=84) in the developmental cohort. The difference in clinical characteristics between the two groups was statistically significant: age (52.86±17.17 vs. 65.31±15.84 years, P<0.001), smoking (43.11% vs. 63.10%, P<0.001), tumor (3.93% vs. 16.67%, P<0.001), chest pain (6.23% vs. 19.05%, P<0.001), dyspnea (15.57% vs. 72.62%, P<0.001), surgery (0.98% vs. 7.14% P<0.001) and D-dimer (0.29±0.51 vs. 2.11±3.24 mg/L, P<0.001).
Table 2
Clinical characteristics | Non-PTE (n=610) | PTE (n=84) | P value |
---|---|---|---|
Age | 52.86±17.17 | 65.31±15.84 | <0.001 |
Sex | 0.03 | ||
Male | 432 (70.82) | 69 (82.14) | |
Female | 178 (29.18) | 15 (17.86) | |
Smoking | <0.001 | ||
No | 347 (56.89) | 31 (36.90) | |
Yes | 263 (43.11) | 53 (63.10) | |
COPD | 0.08 | ||
No | 486 (79.67) | 60 (71.43) | |
Yes | 124 (20.33) | 24 (28.57) | |
Hypertension | 0.003 | ||
No | 536 (87.87) | 64 (76.19) | |
Yes | 74 (12.13) | 20 (23.81) | |
Tumor | <0.001 | ||
No | 586 (96.07) | 70 (83.33) | |
Yes | 24 (3.93) | 14 (16.67) | |
Diabetes | 0.56 | ||
No | 523 (85.74) | 74 (88.10) | |
Yes | 87 (14.26) | 10 (11.90) | |
Cough | 0.13 | ||
No | 163 (26.72) | 16 (19.05) | |
Yes | 447 (73.28) | 68 (80.95) | |
Chest pain | <0.001 | ||
No | 572 (93.77) | 68 (80.95) | |
Yes | 38 (6.23) | 16 (19.05) | |
Hemoptysis | 0.14 | ||
No | 536 (87.87) | 69 (82.14) | |
Yes | 74 (12.13) | 15 (17.86) | |
Dyspnea | <0.001 | ||
No | 515 (84.43) | 23 (27.38) | |
Yes | 95 (15.57) | 61 (72.62) | |
Bedridden | 0.06 | ||
No | 591 (96.89) | 78 (92.86) | |
Yes | 19 (3.11) | 6 (7.14) | |
Surgery | <0.001 | ||
No | 604 (99.02) | 78 (92.86) | |
Yes | 6 (0.98) | 6 (7.14) | |
Hemoglobin (g/L) | 120.37±18.94 | 115.65±24.52 | 0.07 |
Platelet count (×109/L) | 240.22±93.13 | 230.93±85.49 | 0.39 |
Fibrinogen (g/L) | 3.78±1.40 | 4.17±1.58 | 0.02 |
D-dimer (mg/L) | 0.29±0.51 | 2.11±3.24 | <0.001 |
The table is adapted from Kong et al. (14). Reuse with exemptions from licenses of European Journal of Medical Research under the journal’s copyright policy CC BY-NC 4.0. Data are presented as mean ± standard deviation or n (%). COPD, chronic obstructive pulmonary disease.
We summarized the results of univariate and multivariate regression analyses for the developmental cohort in Table 3, which provides a detailed summary of odds ratio (OR) and 95% confidence interval (CI). A univariate regression analysis was conducted from the 17 variables included and 10 variables were selected. These variables include: age, smoking, hemoglobin, fibrinogen, D-dimer, hypertension, tumor, chest pain, dyspnea and surgery. Multivariate regression analysis of the above variables showed that age (OR =1.027; 95% CI: 1.006–1.048; P=0.01), D-dimer (OR =2.873; 95% CI: 1.932–4.272; P<0.001), tumor (OR =3.843; 95% CI: 1.285–11.499; P=0.02), chest pain (OR =3.434; 95% CI: 1.415–8.338; P=0.006), dyspnea (OR =9.261; 95% CI: 4.936–17.378; P<0.001), surgery (OR =6.455; 95% CI: 1.330–31.320; P=0.03) were independent risk factors for PE in PTB patients.
Table 3
Variables | Univariable logistic regression analysis | Multivariate logistic regression analysis | |||
---|---|---|---|---|---|
OR (95% CI) | P value | OR (95% CI) | P value | ||
Sex | 0.528 (0.294, 0.947) | 0.03 | 1.222 (0.511, 2.921) | 0.65 | |
Age | 1.052 (1.034, 1.071) | <0.001 | 1.027 (1.006, 1.048) | 0.01 | |
Smoking | 2.256 (1.408, 3.614) | <0.001 | 1.439 (0.697, 2.967) | 0.32 | |
Hemoglobin | 0.988 (0.978, 1.000) | 0.04 | 0.996 (0.981, 1.010) | 0.57 | |
Platelet count | 0.999 (0.996, 1.001) | 0.39 | – | – | |
Fibrinogen | 1.199 (1.029, 1.399) | 0.02 | 0.924 (0.739, 1.155) | 0.49 | |
D-dimer | 4.051 (2.880, 5.699) | <0.001 | 2.873 (1.932, 4.272) | <0.001 | |
COPD | 1.568 (0.939, 2.618) | 0.09 | – | – | |
Hypertension | 2.264 (1.296, 3.954) | 0.004 | 0.926 (0.402, 2.135) | 0.86 | |
Tumor | 4.883 (2.415, 9.875) | <0.001 | 3.843 (1.285, 11.499) | 0.02 | |
Diabetes | 0.812 (0.404, 1.633) | 0.56 | – | – | |
Cough | 1.550 (0.874, 2.749) | 0.13 | – | – | |
Chest pain | 3.542 (1.875, 6.690) | <0.001 | 3.434 (1.415, 8.338) | 0.006 | |
Hemoptysis | 1.575 (0.856, 2.895) | 0.14 | – | – | |
Dyspnea | 14.378 (8.487, 24.356) | <0.001 | 9.261 (4.936, 17.378) | <0.001 | |
Bedridden | 2.393 (0.927, 6.173) | 0.07 | – | – | |
Surgery | 7.744 (2.437, 24.601) | <0.001 | 6.455 (1.330, 31.320) | 0.02 |
CI, confidence interval; COPD, chronic obstructive pulmonary disease; OR, odds ratio.
Development of the nomogram
The multivariate regression analysis results suggested that age, D-dimer, tumor, chest pain, dyspnea and surgery were independent risk factors for PE in PTB patients. We constructed a nomogram to predict the probability of PE in PTB patients based on the six variables that were assessed (Figure 2A). Each value on the horizontal axis corresponds to a distinct relative score, and the sum of all indicator scores represents the probability of developing a risk of PE. Clinical factors correspond to a specific point. By drawing a vertical line upward from this point on the axis, and locating the sum of the points on the total point axis, the sum indicates the predicted probability of embolism.
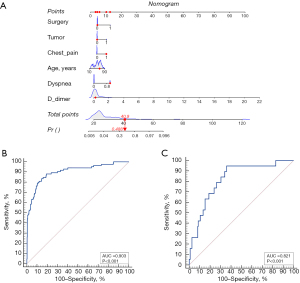
Evaluation of the predictive model
A total of 236 PTB patients from another hospital were included in the external test of the prediction model. Figure 2B,2C show the ROC curve of the development cohorts and validation cohorts and the AUC of the prediction model is 0.903 and 0.821 (P<0.05). Figure 3A,3B show the calibration curves for the development and validation cohorts, respectively, which demonstrate that the predicted probabilities of the model are very close to the actual probabilities. Figure 3C shows the DCA curves for both cohorts with significant net gains for most threshold probabilities.
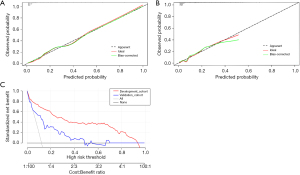
DiscussionOther Section
In our study, PTB complicated with PE was associated with tumors, D-dimer levels, chest pain, age, surgery and dyspnea. Furthermore, multivariate regression analysis demonstrated that these clinical variables were independent risk factors for PTB complicated with PE. The tumor is an independent risk factor for PTB combined with PE. A study by Park et al. found that the occurrence of PE in a significant proportion of patients with malignant tumors is one of the leading causes of death (15). It is also estimated that 20–30% of thromboembolic cases are associated with malignancy (16). Additionally, the incidence of cancer-associated thrombosis has increased over time (17). In a meta-analysis, Reynolds et al. found that the incidence of PE in patients with malignancies ranged from 0.13% to 8.65%, which is significantly higher than the incidence of PE in the general population (18). Similarly, in the present study, tumors were seen as an independent risk factor for PTB complicated with PE, and patients with PTB combined with tumors had a higher risk of PE.
During the breakdown of cross-linked fibronectin, a D-dimer is formed (19). The D-dimer test is a standard, quick, easy, and low-cost laboratory test. When elevated, this serves as a sensitive indicator of acute intravascular thrombosis (20-23). Some studies have shown that each unit increase in D-dimer levels in patients with TB, the risk of complicated PE increases several-fold (24,25). The possibility of PE should be considered in PTB patients if the D-dimer level is several times higher than that before during dynamic follow-up.
Furthermore, dyspnea is a common clinical manifestation of PE, with an overall prevalence of 32.1% reported in a study of patients in the emergency department presenting with dyspnea (26). Additionally, a study on acute PE in Thailand showed that the most common symptom was dyspnea (27). Our study also shows that patients with PTB who present with dyspnea should be screened for possible combined PE.
A related study by Heit et al. showed that age is an independent risk factor for PE, with the risk increasing approximately 1-fold every 10 years with increasing age (28). White found that the prevalence of PE is low in adolescents and high in the elderly population. Its pathogenesis may be closely related to aging, increased blood viscosity, and thrombosis due to vascular endothelial damage (29). In a multicenter retrospective study, PE was examined in 30% of patients presenting for chest pain, and PE was diagnosed in nearly 3% of patients (30). This also suggests that patients with chest pain should be alert to the possibility of PE in clinical diagnosis and treatment. Moulder et al. found that operative time was closely related to embolization (31). Some scholars have pointed out that the risk of embolism after malignant tumor surgery is as high as 8.4% (32). In surgical patients, in addition to various types of surgery, intraoperative anaesthesia, and postoperative bed rest, also predispose to thrombosis.
In recent years, the nomogram has been widely used as a new predictive model for the diagnosis of various diseases. It improves the ability of medical professionals, the effectiveness of further treatment measures and supports them in making informed medical decisions (33). In this study, age, chest pain, tumor, D-miner, surgery and dyspnea were screened by multivariate analysis, and a nomogram was constructed. We also conducted external validation, the AUC of the prediction model is 0.821, which shows that the model has good predictive value.
There are some limitations in this study. First, the sample size was relatively small, and the statistical power was reduced. Second, only two hospitals were included in this study, and a multicenter study could be conducted in the course of the follow-up study. Third, follow-up bias cannot be avoided because of the retrospective nature of this study. For the results to be more valuable, large-sample, multicenter prospective cohort studies are needed to confirm our findings.
ConclusionsOther Section
We developed a nomogram to predict the incidence of PE in patients with PTB using six common clinical variables, including age, chest pain, tumors, D-dimer levels, surgery and dyspnea. Our model was evaluated by calibration curve, ROC curve analysis and DCA. This model was shown to be clinically valuable for clarifying the diagnosis and ensuring early initiation of treatment in patients with PTB combined with PE.
AcknowledgmentsOther Section
We thank the anonymous reviewers for constructive comments. We gratefully acknowledge Dr. Shen Ya from the Clinical College of Infectious Diseases, Fuyang, Anhui Medical University, for providing the validation cohort data.
FootnoteOther Section
Reporting Checklist: The authors have completed the TRIPOD reporting checklist. Available at https://jtd.amegroups.com/article/view/10.21037/jtd-24-1627/rc
Data Sharing Statement: Available at https://jtd.amegroups.com/article/view/10.21037/jtd-24-1627/dss
Peer Review File: Available at https://jtd.amegroups.com/article/view/10.21037/jtd-24-1627/prf
Funding: None.
Conflicts of Interest: All authors have completed the ICMJE uniform disclosure form (available at https://jtd.amegroups.com/article/view/10.21037/jtd-24-1627/coif). The authors have no conflicts of interest to declare.
Ethical Statement: The authors are accountable for all aspects of the work in ensuring that questions related to the accuracy or integrity of any part of the work are appropriately investigated and resolved. The study was conducted in accordance with the Declaration of Helsinki (as revised in 2013). The study was approved by Ethics Committee of Anhui Chest Hospital (No. KJ2030-39) and Ethics Committee of Fuyang Infectious Disease Clinical College of Anhui Medical University (No. 20230814015). Informed consent was taken from all the patients.
Open Access Statement: This is an Open Access article distributed in accordance with the Creative Commons Attribution-NonCommercial-NoDerivs 4.0 International License (CC BY-NC-ND 4.0), which permits the non-commercial replication and distribution of the article with the strict proviso that no changes or edits are made and the original work is properly cited (including links to both the formal publication through the relevant DOI and the license). See: https://creativecommons.org/licenses/by-nc-nd/4.0/.
ReferencesOther Section
- Harding E. WHO global progress report on tuberculosis elimination. Lancet Respir Med 2020;8:19. [Crossref] [PubMed]
- Miggiano R, Rizzi M, Ferraris DM. Mycobacterium tuberculosis Pathogenesis, Infection Prevention and Treatment. Pathogens 2020;9:385. [Crossref] [PubMed]
- Essien EO, Rali P, Mathai SC. Pulmonary Embolism. Med Clin North Am 2019;103:549-64. [Crossref] [PubMed]
- Goldhaber SZ. Pulmonary embolism. Lancet 2004;363:1295-305. [Crossref] [PubMed]
- Dakhlia S, Iqbal P, Abubakar M, et al. A case of concomitant pulmonary embolism and pulmonary tuberculosis in the era of COVID 19, a matter of cautious approach. Clin Case Rep 2021;9:e04071. [Crossref] [PubMed]
- Zhang Z, Tang L, Hu Y. Progress in the research on venous thromboembolism. J Huazhong Univ Sci Technolog Med Sci 2017;37:811-5. [Crossref] [PubMed]
- Yan C, Wang X, Su H, et al. Recent Progress in Research on the Pathogenesis of Pulmonary Thromboembolism: An Old Story with New Perspectives. Biomed Res Int 2017;2017:6516791. [Crossref] [PubMed]
- van Es N, Kraaijpoel N, Klok FA, et al. The original and simplified Wells rules and age-adjusted D-dimer testing to rule out pulmonary embolism: an individual patient data meta-analysis. J Thromb Haemost 2017;15:678-84. [Crossref] [PubMed]
- Klok FA, Mos IC, Nijkeuter M, et al. Simplification of the revised Geneva score for assessing clinical probability of pulmonary embolism. Arch Intern Med 2008;168:2131-6. [Crossref] [PubMed]
- Huang L, Yin C, Gu X, et al. Severe pulmonary tuberculosis complicated with insidious pulmonary thromboembolism: a case report and literature review. J Thromb Thrombolysis 2020;49:644-50. [Crossref] [PubMed]
- Kwas H, Habibech S, Zendah I, et al. Pulmonary embolism and tuberculosis. Asian Cardiovasc Thorac Ann 2014;22:487-90. [Crossref] [PubMed]
- Chen L, Liu C, Liang T, et al. Pulmonary embolism following the third thoracic tuberculosis surgery: A case report and literature review. J Clin Lab Anal 2022;36:e24256. [Crossref] [PubMed]
- Li HM, Huang Q, Tang F, et al. Altered NCF2, NOX2 mRNA Expression Levels in Peripheral Blood Mononuclear Cells of Pulmonary Tuberculosis Patients. Int J Gen Med 2021;14:9203-9. [Crossref] [PubMed]
- Kong H, Li Y, Shen Y, et al. Predicting the risk of pulmonary embolism in patients with tuberculosis using machine learning algorithms. Eur J Med Res 2024;29:618. [Crossref] [PubMed]
- Park DY, An S, Kashoor I, et al. In-hospital prognosis of malignancy-related pulmonary embolism: an analysis of the national inpatient sample 2016-2018. J Thromb Thrombolysis 2022;54:630-8. [Crossref] [PubMed]
- Timp JF, Braekkan SK, Versteeg HH, et al. Epidemiology of cancer-associated venous thrombosis. Blood 2013;122:1712-23. [Crossref] [PubMed]
- Mahajan A, Brunson A, Adesina O, et al. The incidence of cancer-associated thrombosis is increasing over time. Blood Adv 2022;6:307-20. [Crossref] [PubMed]
- Reynolds MW, Shibata A, Zhao S, et al. Impact of clinical trial design and execution-related factors on incidence of thromboembolic events in cancer patients: a systematic review and meta-analysis. Curr Med Res Opin 2008;24:497-505. [Crossref] [PubMed]
- Nagy Z, Horváth O, Kádas J, et al. D-dimer as a potential prognostic marker. Pathol Oncol Res 2012;18:669-74. [Crossref] [PubMed]
- van Belle A, Büller HR, Huisman MV, et al. Effectiveness of managing suspected pulmonary embolism using an algorithm combining clinical probability, D-dimer testing, and computed tomography. JAMA 2006;295:172-9. [Crossref] [PubMed]
- Crawford F, Andras A, Welch K, et al. D-dimer test for excluding the diagnosis of pulmonary embolism. Cochrane Database Syst Rev 2016;2016:CD010864. [Crossref] [PubMed]
- Robson SC, White NW, Aronson I, et al. Acute-phase response and the hypercoagulable state in pulmonary tuberculosis. Br J Haematol 1996;93:943-9. [Crossref] [PubMed]
- Turken O, Kunter E, Sezer M, et al. Hemostatic changes in active pulmonary tuberculosis. Int J Tuberc Lung Dis 2002;6:927-32.
- Kearon C, de Wit K, Parpia S, et al. Diagnosis of Pulmonary Embolism with d-Dimer Adjusted to Clinical Probability. N Engl J Med 2019;381:2125-34. [Crossref] [PubMed]
- Li X, Qin Y, Ye W, et al. Diagnostic performance of D-dimer in predicting pulmonary embolism in tuberculous pleural effusion patients. BMC Pulm Med 2021;21:177. [Crossref] [PubMed]
- Prandoni P, Lensing AWA, Prins MH, et al. Prevalence of pulmonary embolism among patients with recent onset of dyspnea on exertion. A cross-sectional study. J Thromb Haemost 2023;21:68-75. [Crossref] [PubMed]
- Sompradeekul S, Ittimakin S. Clinical characteristics and outcome of thai patients with acute pulmonary embolism. J Med Assoc Thai 2007;90:59-67.
- Heit JA, Spencer FA, White RH. The epidemiology of venous thromboembolism. J Thromb Thrombolysis 2016;41:3-14. [Crossref] [PubMed]
- White RH. The epidemiology of venous thromboembolism. Circulation 2003;107:I4-8. [Crossref] [PubMed]
- Lefevre-Scelles A, Jeanmaire P, Freund Y, et al. Investigation of pulmonary embolism in patients with chest pain in the emergency department: a retrospective multicenter study. Eur J Emerg Med 2020;27:357-61. [Crossref] [PubMed]
- Moulder JK, Moore KJ, Strassle PD, et al. Effect of length of surgery on the incidence of venous thromboembolism after benign hysterectomy. Am J Obstet Gynecol 2021;224:364.e1-7. [Crossref] [PubMed]
- Lyman GH, Culakova E, Poniewierski MS, et al. Morbidity, mortality and costs associated with venous thromboembolism in hospitalized patients with cancer. Thromb Res 2018;164:S112-8. [Crossref] [PubMed]
- Yang Y, Liang S, Geng J, et al. Development of a nomogram to predict 30-day mortality of patients with sepsis-associated encephalopathy: a retrospective cohort study. J Intensive Care 2020;8:45. [Crossref] [PubMed]