Tumor peripheral bronchial signature predicts spread through air spaces in resected invasive mucinous adenocarcinoma of the lung
Highlight box
Key findings
• Tumor peripheral bronchial signature (TPBS) score was identified as an independent predicting factor for spread through air spaces (STAS) status.
• The nomogram, utilizing radiological features and TPBS score, demonstrated a high diagnostic efficiency in predicting the STAS status.
What is known and what is new?
• A high incidence of STAS has been reported in invasive mucinous adenocarcinoma (IMA) of lung.
• In this study, TPBS was defined as indicators to describe bronchial morphological changes and bronchial distribution characteristics around the tumor in the preoperative computed tomography images. The value of TPBS was examined in predicting STAS in patients with resected IMA.
What is the implication, and what should change now?
• This study is the first to utilize TPBS combined with radiological factors in predicting the STAS status in patients with IMA, aiming to provide appropriate individualized therapy for these patients.
Introduction
Spread through air spaces (STAS) is defined as tumor nests within air spaces in the lung parenchyma at a distance of at least 0.5 mm from the edge of the main tumor (1), which has been identified as a potential invasive pattern in lung adenocarcinoma (ADC) (2). Several studies have proved that sub-lobar resection may be comparable to lobectomy in terms of clinical outcomes and could be a viable surgical option for patients with early-stage lung cancer (3,4). However, other research has suggested that limited resection in patients with STAS-positive tumors carries a higher risk of recurrence and poorer survival (5,6). Therefore, it is essential for thoracic surgeons to conduct effective preoperative or intraoperative evaluation of the STAS status, aiming to determine the most appropriate surgical approach.
Invasive mucinous adenocarcinoma (IMA), previously known as mucinous bronchoalveolar carcinoma, is a subtype of lung ADC and accounts for 2–5% of all ADC cases (7,8). Pathologically, IMA is characterized by tall columnar cells with abundant cytoplasmic mucin and goblet cells (9). Furthermore, typical immunohistochemical results of IMA include the positive expression of hepatocyte nuclear factor 4 alpha, cytokeratin 20, and cytokeratin 7, as well as the deletion of napsin A and thyroid transcription factor (10,11). Genetically, the high frequency of KRAS mutations (≥50%) is exhibited in IMAs (11,12). Regarding prognosis, there is a scarcity of survival data due to its low incidence, and the few existing studies exhibit inconsistent findings. According to some studies, IMA was associated with a poorer prognosis compared to non-mucinous ADC (2,13,14). In contrast, other studies have indicated that no significant difference in prognosis was shown between patients with IMA and non-mucinous ADC (15). A recent study conducted by Lee et al. found that patients with IMA showed a higher incidence of STAS (72.3%) (16), compared with patients suffering from non-mucinous ADC (approximately 14.8–47.6%) (17-19), and other non-small cell lung cancer (NSCLC) (20,21). Moreover, STAS has been identified as an independent poor prognostic predictor for overall survival (OS) rate in IMA patients (16). Despite the high frequency of STAS in patients with IMA, there is limited study available on predicting STAS status in these patients. Previous studies mainly focused on radiological tumor features to predict STAS status in NSCLC (22,23). However, the relationship between bronchus and tumor was rarely mentioned in relation to STAS status. Kaku et al. observed scattered STAS tumor cells in the bronchioles, which formed floating cancer cell clusters or single cells. The cancer cells in the bronchioles grew in a micropapillary fashion on the mucosa surface, exhibited a discontinuous skipping distribution, and spread into the alveolar spaces (24). This study suggested that if more bronchioles are distributed around the tumor, more surrounding alveoli tumor cells will spread into. Additionally, it has been reported that bronchus truncation sign, cavity/cystic airspace and air bronchogram were more frequently occurred in NSCLC patients with positive STAS (22,23,25). Therefore, it might be feasible to predict STAS by analysis of bronchial morphological changes and bronchial distribution characteristics around the tumor in the preoperative computed tomography (CT) images. Tumor peripheral bronchial signature (TPBS) was defined as indicators to describe bronchial morphological changes and bronchial distribution characteristics around the tumor in the preoperative CT images. Then, these TPBS indicators were included to construct a complex predictor in this study. This study is the first to utilize TPBS combined with radiological factors in predicting the STAS status in patients with IMA, aiming to provide appropriate individualized therapy for these patients. We present this article in accordance with the TRIPOD reporting checklist (available at https://jtd.amegroups.com/article/view/10.21037/jtd-2024-2036/rc).
Methods
Patient population
Between February 2017 and October 2021, a total of 135 patients from The First Affiliated Hospital of Ningbo University (Hospital 1) were included in this study as a training cohort and used for internal validation. Furthermore, 66 patients from the Ningbo Medical Center Lihuili Hospital (Hospital 2) were enrolled as the external validation cohort. The detailed patient inclusion procedure is shown in Figure 1. This study was approved by the Ethics Committee of The First Affiliated Hospital of Ningbo University (No. 2024-194RS). Ningbo Medical Center Lihuili Hospital was informed and agreed with the study. Given the retrospective design of this investigation, the necessity for obtaining informed consent was exempted. The study was conducted in accordance with the Declaration of Helsinki (as revised in 2013).
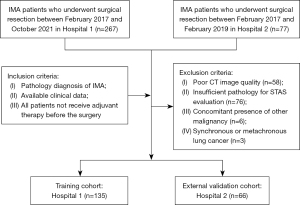
The inclusion criteria are as follows: patients with (I) pathology diagnosis of IMA; (II) complete clinical data; (III) all patients not receive adjuvant therapy before the surgery.
The exclusion criteria are as follows: patients with (I) poor CT image quality; (II) insufficient pathology for STAS evaluation; (III) concomitant presence of other malignancy; and (IV) synchronous or metachronous lung cancer.
Clinical data and pathological data collection
Patient data, including clinical characteristic (gender, age and smoking status, surgical type, lymph nodal sampling and lymph node invasion), pathological type and STAS Status were collected.
Radiologic analysis
Two board-certified thoracic radiologists independently reviewed preoperative three-dimensional CT images. For the quantitative analysis, the final data were derived from the average measurements provided by two independent radiologists. In cases involving qualitative indicators, any discrepancies or disagreements between the radiologists were thoroughly discussed until a mutual consensus was achieved. Firstly, normal radiologic features, such as clinical T classification, density type, lobulation, consolidation-tumor ratio (CTR), lobulated margin, spiculated margin, pleural indentation and combined pneumonia, were collected. Subsequently, TPBS included several indicators to describe bronchial morphological changes and bronchial distribution characteristics around the tumor in the three-dimensional CT images. We mainly measure TPBS in the coronal plane and then use the sagittal and frontal planes to check for neglected small bronchi. TPBS was assessed: (I) bronchial morphological changes were described by cavity/cystic airspace, bronchus truncation sign and air bronchogram sign; (II) bronchial distribution characteristics was assessed: The relative location of the bronchiole and tumor was described by number of bronchioles within the safety surgical margin (NOBWSSM), the closest distance from tumor to the bronchiole (CDFTB), NOBWSSM/tumor diameter (TD) and CDFTB/TD; The relative location of the main bronchus and tumor was described by the distance from tumor to the mediastinum (DFTM), lung width (LW), DFTM/LW, DFTM/TD and LW/TD. The definitions of various TPBS were described in Table S1, and TPBS was shown in Figure 2.
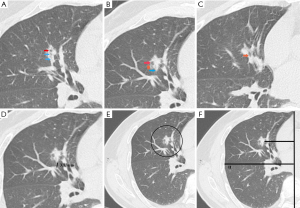
Histopathologic analysis
The pathological diagnosis for each patient included in this study was determined by a highly experienced lung pathologist. STAS was defined as tumor nests within air spaces in the lung parenchyma at a distance of at least 0.5 mm from the edge of the main tumor.
Statistical analysis
The statistical analyses for this study were conducted using R statistical software, version 4.3.2. Categorical data were presented as the number of cases and analyzed using the χ2 test. For measurement data that were expressed as the mean [standard deviation (SD)], the Mann-Whitney U test was employed. The least absolute shrinkage and selection operator (LASSO) were applied to select TPBS that had the strongest relevance with positive STAS and constructed the formula for TPBS score. Independent predictors of STAS were selected by univariate and multivariate logistic regression analyses conducted on the clinical data, radiologic features and TPBS score in the training cohort. Subsequently, an independent prediction model was developed using these predictors, and a corresponding nomogram that was verified by bootstrap analyses with 500 resamples was constructed. The discriminative performance of the prediction models in identifying STAS was assessed in both the training and validation cohorts. This evaluation was conducted using the receiver operating characteristic (ROC) curve analysis, along with the calculation of the area under the curve (AUC) value, which serves as a quantitative measure of the model’s predictive accuracy. Calibration curves and the Hosmer-Lemeshow test were used to assess the goodness-of-fit of the prediction model, and decision curve analysis (DCA) was employed to evaluate the clinical utility of the nomogram. Kaplan-Meier survival analysis was performed to calculate difference in prognosis. All reported P values were two-tailed, and a P value of <0.05 was considered statistically significant.
Results
Clinicopathological characteristics of the study cohorts
As indicated in Table 1, a total of 201 patients with IMA were enrolled in this study, among which 85 patients were STAS-positive and 116 patients were STAS-negative. The analysis revealed statistically significant differences in several variables, including clinical T classification, density type, CTR, lobulated margin, spiculated margin, combined pneumonia, air bronchogram sign, bronchus truncation sign, NOBWSSM, DFTM/LW in the both cohorts. However, age, lymph nodal sampling, pleural indentation, cavity/cystic airspace, and DFTM demonstrated statistically significant differences exclusively within the training cohort.
Table 1
Characteristic | Training set (n=135) | External validation set (n=66) | ||||||
---|---|---|---|---|---|---|---|---|
STAS (−) (N=80) | STAS (+) (N=55) | P value | STAS (−) (N=36) | STAS (+) (N=30) | P value | |||
Clinical characteristic | ||||||||
Age (years), mean (SD) | 61.29 (12.81) | 66.25 (9.62) | 0.02 | 61.97 (9.07) | 63.33 (11.71) | 0.60 | ||
Gender | 0.92 | 0.61 | ||||||
Female | 49 | 35 | 19 | 13 | ||||
Male | 31 | 20 | 17 | 17 | ||||
Smoking status | 0.63 | 0.44 | ||||||
Non-smoker | 52 | 33 | 24 | 18 | ||||
Ever | 28 | 22 | 12 | 12 | ||||
Surgery type, n (%) | 0.09 | 0.052 | ||||||
Lobectomy | 41 (51.2) | 38 (69.1) | 25 (69.4) | 27 (90.0) | ||||
Segmentectomy | 5 (6.2) | 1 (1.8) | 2 (5.6) | 2 (6.7) | ||||
Wedge resection | 34 (42.5) | 16 (29.1) | 9 (25.0) | 1 (3.3) | ||||
Lymph nodal sampling, mean (SD) | 8.38 (5.03) | 10.60 (7.18) | 0.04 | 7.97 (5.29) | 9.78 (6.10) | 0.19 | ||
Lymph node invasion | 0.18 | 0.29 | ||||||
Negative | 79 | 51 | 34 | 25 | ||||
Positive | 1 | 4 | 2 | 5 | ||||
Radiologic features | ||||||||
T classification, n (%) | <0.001 | <0.001 | ||||||
T1a | 47 (58.8) | 3 (5.5) | 16 (44.4) | 2 (6.7) | ||||
T1b | 26 (32.5) | 25 (45.5) | 16 (44.4) | 9 (30.0) | ||||
T1c | 7 (8.8) | 14 (25.5) | 3 (8.3) | 7 (23.3) | ||||
T2a | 0 (0.0) | 8 (14.5) | 0 (0.0) | 8 (26.7) | ||||
T2b | 0 (0.0) | 2 (3.6) | 1 (2.8) | 0 (0.0) | ||||
T3 | 0 (0.0) | 3 (5.5) | 0 (0.0) | 4 (13.3) | ||||
Density type | <0.001 | 0.003 | ||||||
Solid | 37 | 48 | 14 | 18 | ||||
mGGN | 43 | 7 | 22 | 12 | ||||
Lobulation n, (%) | 0.37 | 0.38 | ||||||
RUL | 17 (21.2) | 11 (20.0) | 3 (8.3) | 6 (20.0) | ||||
RML | 5 (6.2) | 5 (9.1) | 5 (13.9) | 1 (3.3) | ||||
RLL | 31 (38.8) | 17 (30.9) | 17 (47.2) | 12 (40.0) | ||||
LUL | 11 (13.8) | 4 (7.3) | 4 (11.1) | 5 (16.7) | ||||
LLL | 16 (20.0) | 18 (32.7) | 7 (19.4) | 6 (20.0) | ||||
CTR, mean (SD) | 0.64 (0.45) | 0.98 (0.08) | <0.001 | 0.70 (0.25) | 0.83 (0.22) | 0.03 | ||
Lobulated margin | <0.001 | <0.001 | ||||||
Absent | 66 | 23 | 28 | 10 | ||||
Present | 14 | 32 | 8 | 20 | ||||
Spiculated margin | 0.002 | 0.03 | ||||||
Absent | 43 | 14 | 20 | 11 | ||||
Present | 37 | 41 | 16 | 19 | ||||
Pleural indentation | 0.002 | 0.87 | ||||||
Absent | 67 | 32 | 21 | 16 | ||||
Present | 13 | 23 | 15 | 14 | ||||
Combined pneumonia | 0.03 | <0.001 | ||||||
Absent | 77 | 46 | 33 | 22 | ||||
Present | 3 | 9 | 3 | 8 | ||||
TPBS | ||||||||
Air bronchogram sign | <0.001 | <0.001 | ||||||
Absent (<3) | 73 | 30 | 32 | 11 | ||||
Present (≥3) | 7 | 25 | 4 | 19 | ||||
Bronchus truncation sign | <0.001 | 0.007 | ||||||
Absent | 64 | 18 | 25 | 10 | ||||
Present | 16 | 37 | 11 | 20 | ||||
Cavity/cystic airspace | 0.02 | 0.11 | ||||||
Absent | 69 | 37 | 26 | 15 | ||||
Present | 11 | 18 | 10 | 15 | ||||
NOBWSSM, mean (SD) | 1.24 (1.30) | 3.07 (2.21) | <0.001 | 2.31 (2.71) | 4.63 (1.96) | 0.03 | ||
CDFTB (mm), mean (SD) | 6.59 (7.02) | 3.52 (4.43) | 0.27 | 5.88 (7.45) | 3.13 (4.15) | 0.37 | ||
DFTM (mm), mean (SD) | 72.54 (25.29) | 55.76 (24.03) | <0.001 | 67.84 (21.27) | 60.43 (22.68) | 0.07 | ||
LW (mm), mean (SD) | 114.76 (16.09) | 112.67 (16.11) | 0.46 | 110.31 (9.50) | 109.40 (10.46) | 0.71 |
CDFTB, closest distance from tumor to the bronchiole; CTR, consolidation tumor ratio; DFTM, distance from tumor to the mediastinum; LW, lung width; LLL, left lower lobe; LUL, left upper lobe; mGGN, mixed ground-glass nodule; NOBWSSM, number of bronchioles within the safety surgical margin; RLL, right lower lobe; RML, right middle lobe; RUL, right upper lobe; SD, standard deviation; STAS, spread through air spaces; TPBS, tumor peripheral bronchial signature.
Construction of TPBS score
Based on LASSO, 12 TPBS were integrated to predict STAS (Figure 3A). Tuning parameter lambda was selected using tenfold cross-validation via minimum criteria to find the optimal model via cross-validation (Figure 3B, λ=0.0295). The optimal model included bronchus truncation sign, air bronchogram sign, cavity/cystic airspace, NOBWSSM, CDFTB/TD and DFTM. Formula for TPBS score: TPBS score = 0.072 × NOBWSSM + 0.732 × air bronchogram sign + 0.727 × bronchus truncation sign + 0.549 × cavity/cystic airspace − 0.330 × CDFTB/TD − 0.012 × TDFM.
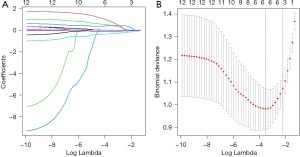
Univariate and multivariate logistic regression analysis
In the training cohort, univariable analysis revealed statistically significant differences in TPBS score between the STAS-positive and the STAS-negative groups (Table 2). The optimal combination of variables identified through multivariate logistic regression analysis included clinical T classification, spiculated margin, combined pneumonia, CTR and TPBS score, among which clinical T classification [odds ratio (OR): 2.61] and TPBS score (OR: 1.99) were identified as independent risk factors to predict the STAS status (Table 2).
Table 2
Characteristic | Univariable analysis | Multivariable analysis | |||
---|---|---|---|---|---|
OR (95% CI) | P value | OR (95% CI) | P value | ||
Age (>50 years) | 1.03 (0.98, 1.08) | 0.32 | – | – | |
Gender (male) | 1.20 (0.40, 3.58) | 0.71 | – | – | |
Smoking status (present) | 1.24 (0.42, 2.45) | 0.84 | – | – | |
T classification (> T1) | 1.86 (0.76, 4.72) | 0.002 | 2.61 (1.37, 5.62) | 0.007 | |
Lobulated margin (present) | 0.97 (0.26, 3.39) | 0.94 | – | – | |
Spiculated margin (present) | 2.57 (0.80, 9.07) | 0.12 | 2.80 (0.93, 9.12) | 0.07 | |
Pleural indentation (present) | 1.73 (0.56, 5.35) | 0.27 | – | – | |
Combined pneumonia (present) | 3.89 (0.58, 32.9) | 0.22 | 5.21 (0.94, 40.1) | 0.08 | |
CTR (continuous) | 2.04 (1.06, 2.48) | 0.11 | 1.95 (1.03, 2.78) | 0.12 | |
TPBS score (continuous) | 1.81 (1.04, 3.31) | 0.02 | 1.99 (1.17, 3.57) | 0.03 |
CI, confidence interval; CTR, consolidation tumor ratio; OR, odds ratio; TPBS, tumor peripheral bronchial signature.
Model development and evaluation
Based on the optimal combination of variables identified through multivariate logistic regression analysis, three distinct models were developed: (I) a TPBS model comprising TPBS score; (II) a basic model comprising clinical T classification, spiculated margin, combined pneumonia and CTR; and (III) a hybrid model, including of all the above variables. Subsequently, the predictive diagnostic performance was compared across different models. The findings demonstrated that the AUC values across the two cohorts were 0.838 and 0.744 for the TPBS model, 0.877 and 0.806 for the basic model, and 0.904 and 0.831 for the hybrid model, respectively. The predictive efficacy and the ROC curves are shown in Table 3 and Figure 4, respectively. A nomogram was subsequently constructed utilizing the regression coefficients derived from the variables identified through multivariate logistic regression analysis, as depicted in Figure 5. The regression equation was ln (P/1−P) = −5.76 + 0.96 × T classification + 1.03 × Spiculated margin + 1.65 × Combined pneumonia + 2.97 × CTR + 0.688 × TPBS score, where P denotes the probability of predicting the occurrence of STAS. The Hosmer-Lemeshow test was employed to validate the adequacy of the combined model, demonstrating a satisfactory fit for both cohorts (P=0.34 and 0.58, respectively). Furthermore, calibration curves were generated through bootstrapping for 500 repetitions, which demonstrated that the predicted probabilities of the hybrid model closely matched the actual probabilities in both cohorts (Figure 6A,6B; training cohort: corrected C-index: 0.891, corrected brier index =0.134; external validation cohort: corrected C-index: 0.809, corrected brier index =0.182). The DCA revealed that the hybrid model exhibited superior clinical utility compared to the basic and TPBS models in the two cohorts (Figure 7A,7B).
Table 3
Model | Cohort | AUC (95% CI) | Sensitivity | Specificity |
---|---|---|---|---|
TPBS model | Training | 0.838 (0.770, 0.905) | 0.782 | 0.812 |
External validation | 0.744 (0.620, 0.869) | 0.667 | 0.778 | |
Basic model | Training | 0.877 (0.823, 0.931) | 0.927 | 0.725 |
External validation | 0.806 (0.692, 0.919) | 0.767 | 0.806 | |
Hybrid model | Training | 0.904 (0.853, 0.955) | 0.909 | 0.787 |
External validation | 0.831 (0.727, 0.934) | 0.767 | 0.861 |
AUC, area under the curve; CI, confidence interval; TPBS, tumor peripheral bronchial signature.
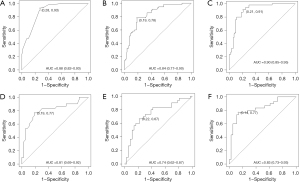
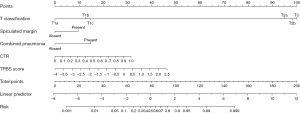
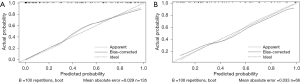
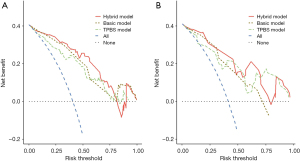
Correlation between prognosis and TPBS score
ROC curves of the TPBS models were employed to determine the cut-off value in the training group. Patients in both cohorts were then categorized into low and high-risk groups. The Kaplan-Meier survival analysis revealed statistically significant differences between the tumor peripheral bronchial signature-high risk (TPBS-H) and tumor peripheral bronchial signature-low risk (TPBS-L) groups in terms of disease-free survival (DFS) (Figure 8A, training cohort, P=0.007). Although the differences were not statistically significant (Figure 8B, P=0.059), the external validation cohort exhibited a similar prognostic trend. Survival curves for DFS across the four subtypes are presented in Figure 8C,8D. Even though significant differences in DFS were not observed in the two cohorts (training cohort: P=0.051; external cohort: P=0.08), patients with STAS (+) + TPBS-H had the worst prognosis.
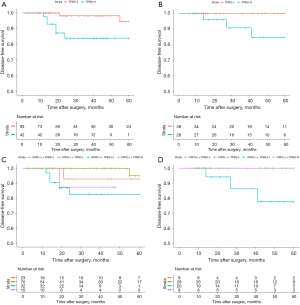
Discussion
Consistent with the results of other studies (16,26,27), our findings have illustrated that patients with IMA show a high incidence of STAS (42.3%). The higher occurrence of STAS in IMA may come from pneumatic spread with mucus (28). Mucinous tumors produce abundant extracellular mucin and often contain viable detached neoplastic cells within the intra alveolar mucus. These neoplastic foci spread via floating in pools of abundant extracellular mucin that replace air spaces. This may be the cause of tumor peripheral bronchial changes in the IMA patients with positive STAS. Moreover, it has been recently reported that aberrant mucin expression, including MUC2, MUC5AC and MUC6, has been found in IMA patients (29). In terms of causality regarding higher STAS incidence, MUC5AC in IMA could alter cell polarity and migration of cancer cells. Mucin proteins, whether functioning independently or through interactions with different receptors, have the potential to mediate cellular signaling (29,30). STAS is widely recognized as a significant risk factor associated with postoperative tumor recurrence and metastasis in patients diagnosed with early-stage lung cancer, and it has been shown that lobectomy could result in better clinical outcomes compared to sublobectomy (5,6,31). Therefore, accurately predicting the STAS status prior to surgery holds significant clinical importance, as it plays a crucial role in guiding the selection of the most appropriate surgical approach. This study identified TPBS score as an independent risk factor to predict the STAS status in IMA patients. Based on the optimal combination of variables, including clinical T classification, spiculated margin, combined pneumonia, CTR and TPBS score, selected through multivariate logistic regression analysis, the hybrid model was developed. This model exhibited the highest diagnostic efficacy among the models tested. The AUC values in the training cohort and external validation cohorts were 0.904 and 0.831, respectively. The sensitivity was 90.9% and 76.7%, while the specificity was 78.7% and 86.1%, in the training and external validation cohorts, respectively.
In the present study, it was found that clinical T classification, spiculated margin, combined pneumonia, CTR and TPBS score were the best predictors included, which is consistent with previous literature reports (32,33) and suggests a potential link between STAS-positivity and the underlying pathological mechanism of the tumor. Several studies have shown an increased risk of STAS with larger tumor size (19,34,35). Tumors larger than 2 cm are associated with a greater than 38% probability of STAS (35). Our study proved that T2a tumors exhibited significantly higher risks of STAS compared to T1c tumors in the prediction nomogram.
Spiculation is commonly linked to the infiltration of tumor cells into nearby blood vessels and lymphatic vessels, which indicates the potentially aggressive nature of lung cancer (36). In our study, it was observed that STAS tended to be associated with combined pneumonia. Tumor-infiltrating neutrophils are abundant in ADC and linked to poorer outcomes in patients with ADC (37). Previous research indicated that tumor-infiltrating neutrophils may play a role in the release of mitogenic and scattering factors that facilitate cancer cell migration. Additionally, they have been implicated in inducing detachment of tumor cells and promoting tumor shedding (38,39). These mechanisms are also potentially linked to tumor detachment and progression. Further molecular studies are required on tumor-host interactions to identify the underlying biological mechanisms of STAS.
Solid components generally constitute the more aggressive segment of the tumor (40). Prior research has indicated a positive association between CTR and STAS (15,41-43) and higher CTR values have been linked to more aggressive tumors, an increased likelihood of a positive STAS status, and a poorer patient prognosis (44). In our study, a higher incidence of STAS was observed in cases with a higher proportion of solid components shown on CT scans, which is consistent with previous research.
Based on LASSO regression, a complex predictor, called TPBS score, was constructed using TPBS. These signatures were designed to describe bronchial morphological changes and bronchial distribution characteristics around the tumor in the preoperative CT images. Malignant indicators in invasive lung ADC, such as cavitation, bronchus truncation sign, and air bronchogram sign showed statistical significance between the STAS-positive and STAS-negative groups, reported by de Margerie-Mellon (41) and Chen et al. (45), which may be attributed to the ability of tumor cells to detach from the primary lesion, disseminate through the airway, and proliferate along the adjacent alveolar wall. This process ultimately leads to the obstruction of the terminal bronchioles and the subsequent manifestation of an air bronchogram (22). However, the existing literature on this topic is limited, indicating that these indicators may serve as independent predictors of the STAS status. In this study, for the first time the relationship between TPBS and STAS statue was deeply explored, thus constructing relevant prediction models. In comparison to traditional deep learning prediction models based on CT images, this model constructed predictors through a multidimensional description of TPBS, which significantly enhanced the interpretability of the model.
Our study is limited by its retrospective nature. A total of 134 of 344 (39%) lung cancer patients who could have been qualified to be included in the study were excluded due to missing records of their STAS status or CT images. The difference in DFS was only observed in the training cohort rather than the external validation cohort. This discrepancy may be due to the fact that 79% of the external validation cohort underwent lobectomy and the external validation cohort had a smaller sample size.
To further investigate the potential relationship between TPBS score and STAS, survival analysis of sub-group in both cohorts was conducted. Although a significant difference was not observed in two cohorts, STAS-positive with TPBS-H may show poorer prognosis than STAS-positive with TPBS-L. Uruga et al. (46) defined a high STAS burden as ≥5 single cells or clusters of STAS groups in airspaces beyond the main tumor edge. High STAS burden showed worse DFS and OS compared with both low STAS (<5 single cells or clusters of STAS groups) or no STAS in resected early-stage lung ADCs (46), which may suggest a hidden association between TPBS-H and high STAS burden. However, this speculation requires further verification in a large sample cohort with detailed assessment of STAS burden. Due to the lack of OS data, our study did not take the impact of TPBS on overall mortality of IMA into account. The IMA was selected as the study subject because it exhibits a higher degree of tumor invasion and a higher frequency of STAS, compared to most non-mucinous ADC. Moreover, pneumatic spread with mucus is more likely to cause bronchial changes around the tumor. In the future, it will be necessary to verify whether TPBS score can predict the state of STAS in non-mucinous ADC.
Conclusions
TPBS score was identified as independent predicting factors for STAS status. The nomogram, using radiological features and TPBS score, exhibits a high level of diagnostic accuracy in predicting the STAS status among patients with resected IMA. Most importantly, this tool may help develop personalized preoperative or intraoperative treatment strategies.
Acknowledgments
None.
Footnote
Reporting Checklist: The authors have completed the TRIPOD reporting checklist. Available at https://jtd.amegroups.com/article/view/10.21037/jtd-2024-2036/rc
Data Sharing Statement: Available at https://jtd.amegroups.com/article/view/10.21037/jtd-2024-2036/dss
Peer Review File: Available at https://jtd.amegroups.com/article/view/10.21037/jtd-2024-2036/prf
Funding: This study was supported by grant from
Conflicts of Interest: All authors have completed the ICMJE uniform disclosure form (available at https://jtd.amegroups.com/article/view/10.21037/jtd-2024-2036/coif). The authors have no conflicts of interest to declare.
Ethical Statement: The authors are accountable for all aspects of the work in ensuring that questions related to the accuracy or integrity of any part of the work are appropriately investigated and resolved. This study was approved by the Ethics Committee of The First Affiliated Hospital of Ningbo University (No. 2024-194RS). Ningbo Medical Center Lihuili Hospital was informed and agreed with the study. Given the retrospective design of this investigation, the necessity for obtaining informed consent was exempted. The study was conducted in accordance with the Declaration of Helsinki (as revised in 2013).
Open Access Statement: This is an Open Access article distributed in accordance with the Creative Commons Attribution-NonCommercial-NoDerivs 4.0 International License (CC BY-NC-ND 4.0), which permits the non-commercial replication and distribution of the article with the strict proviso that no changes or edits are made and the original work is properly cited (including links to both the formal publication through the relevant DOI and the license). See: https://creativecommons.org/licenses/by-nc-nd/4.0/.
References
- Shimomura M, Miyagawa-Hayashino A, Omatsu I, et al. Spread through air spaces is a powerful prognostic predictor in patients with completely resected pathological stage I lung adenocarcinoma. Lung Cancer 2022;174:165-71. [Crossref] [PubMed]
- Travis WD, Brambilla E, Nicholson AG, et al. The 2015 World Health Organization Classification of Lung Tumors: Impact of Genetic, Clinical and Radiologic Advances Since the 2004 Classification. J Thorac Oncol 2015;10:1243-60. [Crossref] [PubMed]
- Cao C, Gupta S, Chandrakumar D, et al. Meta-analysis of intentional sublobar resections versus lobectomy for early stage non-small cell lung cancer. Ann Cardiothorac Surg 2014;3:134-41. [PubMed]
- Chiang XH, Hsu HH, Hsieh MS, et al. Propensity-Matched Analysis Comparing Survival After Sublobar Resection and Lobectomy for cT1N0 Lung Adenocarcinoma. Ann Surg Oncol 2020;27:703-15. [Crossref] [PubMed]
- Shiono S, Endo M, Suzuki K, et al. Spread Through Air Spaces Is a Prognostic Factor in Sublobar Resection of Non-Small Cell Lung Cancer. Ann Thorac Surg 2018;106:354-60. [Crossref] [PubMed]
- Ren Y, Xie H, Dai C, et al. Prognostic Impact of Tumor Spread Through Air Spaces in Sublobar Resection for 1A Lung Adenocarcinoma Patients. Ann Surg Oncol 2019;26:1901-8. [Crossref] [PubMed]
- Warth A, Muley T, Meister M, et al. The novel histologic International Association for the Study of Lung Cancer/American Thoracic Society/European Respiratory Society classification system of lung adenocarcinoma is a stage-independent predictor of survival. J Clin Oncol 2012;30:1438-46. [Crossref] [PubMed]
- Yoshizawa A, Sumiyoshi S, Sonobe M, et al. Validation of the IASLC/ATS/ERS lung adenocarcinoma classification for prognosis and association with EGFR and KRAS gene mutations: analysis of 440 Japanese patients. J Thorac Oncol 2013;8:52-61. [Crossref] [PubMed]
- Yousem SA, Beasley MB. Bronchioloalveolar carcinoma: a review of current concepts and evolving issues. Arch Pathol Lab Med 2007;131:1027-32. [Crossref] [PubMed]
- Travis WD, Brambilla E, Riely GJ. New pathologic classification of lung cancer: relevance for clinical practice and clinical trials. J Clin Oncol 2013;31:992-1001. [Crossref] [PubMed]
- Sugano M, Nagasaka T, Sasaki E, et al. HNF4α as a marker for invasive mucinous adenocarcinoma of the lung. Am J Surg Pathol 2013;37:211-8. [Crossref] [PubMed]
- Tsuta K, Kawago M, Inoue E, et al. The utility of the proposed IASLC/ATS/ERS lung adenocarcinoma subtypes for disease prognosis and correlation of driver gene alterations. Lung Cancer 2013;81:371-6. [Crossref] [PubMed]
- Wislez M, Antoine M, Baudrin L, et al. Non-mucinous and mucinous subtypes of adenocarcinoma with bronchioloalveolar carcinoma features differ by biomarker expression and in the response to gefitinib. Lung Cancer 2010;68:185-91. [Crossref] [PubMed]
- Casali C, Rossi G, Marchioni A, et al. A single institution-based retrospective study of surgically treated bronchioloalveolar adenocarcinoma of the lung: clinicopathologic analysis, molecular features, and possible pitfalls in routine practice. J Thorac Oncol 2010;5:830-6. [Crossref] [PubMed]
- Shim HS, Kenudson M, Zheng Z, et al. Unique Genetic and Survival Characteristics of Invasive Mucinous Adenocarcinoma of the Lung. J Thorac Oncol 2015;10:1156-62. [Crossref] [PubMed]
- Lee MA, Kang J, Lee HY, et al. Spread through air spaces (STAS) in invasive mucinous adenocarcinoma of the lung: Incidence, prognostic impact, and prediction based on clinicoradiologic factors. Thorac Cancer 2020;11:3145-54. [Crossref] [PubMed]
- Morales-Oyarvide V, Mino-Kenudson M. Tumor islands and spread through air spaces: Distinct patterns of invasion in lung adenocarcinoma. Pathol Int 2016;66:1-7. [Crossref] [PubMed]
- Shiono S, Yanagawa N. Spread through air spaces is a predictive factor of recurrence and a prognostic factor in stage I lung adenocarcinoma. Interact Cardiovasc Thorac Surg 2016;23:567-72. [Crossref] [PubMed]
- Dai C, Xie H, Su H, et al. Tumor Spread through Air Spaces Affects the Recurrence and Overall Survival in Patients with Lung Adenocarcinoma >2 to 3 cm. J Thorac Oncol 2017;12:1052-60. [Crossref] [PubMed]
- Lu S, Tan KS, Kadota K, et al. Spread through Air Spaces (STAS) Is an Independent Predictor of Recurrence and Lung Cancer-Specific Death in Squamous Cell Carcinoma. J Thorac Oncol 2017;12:223-34. [Crossref] [PubMed]
- Yokoyama S, Murakami T, Tao H, et al. Tumor Spread Through Air Spaces Identifies a Distinct Subgroup With Poor Prognosis in Surgically Resected Lung Pleomorphic Carcinoma. Chest 2018;154:838-47. [Crossref] [PubMed]
- Wang Y, Lyu D, Zhang D, et al. Nomogram based on clinical characteristics and radiological features for the preoperative prediction of spread through air spaces in patients with clinical stage IA non-small cell lung cancer: a multicenter study. Diagn Interv Radiol 2023;29:771-85. [Crossref] [PubMed]
- Gao Z, An P, Li R, et al. Development and validation of a clinic-radiological model to predict tumor spread through air spaces in stage I lung adenocarcinoma. Cancer Imaging 2024;24:25. [Crossref] [PubMed]
- Kaku S, Motoi N, Watanabe H, et al. "Real" Tumor-Spread Through Air Spaces of Lung Adenocarcinoma Presented Intrapulmonary Metastases Through Bronchiole Air Spaces: A Case Report. JTO Clin Res Rep 2022;3:100273. [Crossref] [PubMed]
- Gu Y, Zheng B, Zhao T, et al. Computed Tomography Features and Tumor Spread Through Air Spaces in Lung Adenocarcinoma: A Meta-analysis. J Thorac Imaging 2023;38:W19-29. [Crossref] [PubMed]
- Isaka T, Yokose T, Miyagi Y, et al. Detection of tumor spread through airspaces by airway secretion cytology from resected lung cancer specimens. Pathol Int 2017;67:487-94. [Crossref] [PubMed]
- Kim SK, Kim TJ, Chung MJ, et al. Lung Adenocarcinoma: CT Features Associated with Spread through Air Spaces. Radiology 2018;289:831-40. [Crossref] [PubMed]
- Gaikwad A, Souza CA, Inacio JR, et al. Aerogenous metastases: a potential game changer in the diagnosis and management of primary lung adenocarcinoma. AJR Am J Roentgenol 2014;203:W570-82. [Crossref] [PubMed]
- Lakshmanan I, Ponnusamy MP, Macha MA, et al. Mucins in lung cancer: diagnostic, prognostic, and therapeutic implications. J Thorac Oncol 2015;10:19-27. [Crossref] [PubMed]
- Geles A, Gruber-Moesenbacher U, Quehenberger F, et al. Pulmonary mucinous adenocarcinomas: architectural patterns in correlation with genetic changes, prognosis and survival. Virchows Arch 2015;467:675-86. [Crossref] [PubMed]
- Kadota K, Kushida Y, Kagawa S, et al. Limited Resection Is Associated With a Higher Risk of Locoregional Recurrence than Lobectomy in Stage I Lung Adenocarcinoma With Tumor Spread Through Air Spaces. Am J Surg Pathol 2019;43:1033-41. [Crossref] [PubMed]
- Toyokawa G, Yamada Y, Tagawa T, et al. Computed tomography features of resected lung adenocarcinomas with spread through air spaces. J Thorac Cardiovasc Surg 2018;156:1670-1676.e4. [Crossref] [PubMed]
- Qi L, Xue K, Cai Y, et al. Predictors of CT Morphologic Features to Identify Spread Through Air Spaces Preoperatively in Small-Sized Lung Adenocarcinoma. Front Oncol 2020;10:548430. [Crossref] [PubMed]
- Toyokawa G, Yamada Y, Tagawa T, et al. Significance of Spread Through Air Spaces in Resected Pathological Stage I Lung Adenocarcinoma. Ann Thorac Surg 2018;105:1655-63. [Crossref] [PubMed]
- Tasnim S, Raja S, Mukhopadhyay S, et al. Preoperative predictors of spread through air spaces in lung cancer. J Thorac Cardiovasc Surg 2024;168:660-669.e4. [Crossref] [PubMed]
- Jin C, Cao J, Cai Y, et al. A nomogram for predicting the risk of invasive pulmonary adenocarcinoma for patients with solitary peripheral subsolid nodules. J Thorac Cardiovasc Surg 2017;153:462-469.e1. [Crossref] [PubMed]
- Lin EY, Pollard JW. Role of infiltrated leucocytes in tumour growth and spread. Br J Cancer 2004;90:2053-8. [Crossref] [PubMed]
- Wislez M, Rabbe N, Marchal J, et al. Hepatocyte growth factor production by neutrophils infiltrating bronchioloalveolar subtype pulmonary adenocarcinoma: role in tumor progression and death. Cancer Res 2003;63:1405-12. [PubMed]
- Wislez M, Antoine M, Rabbe N, et al. Neutrophils promote aerogenous spread of lung adenocarcinoma with bronchioloalveolar carcinoma features. Clin Cancer Res 2007;13:3518-27. [Crossref] [PubMed]
- Travis WD, Asamura H, Bankier AA, et al. The IASLC Lung Cancer Staging Project: Proposals for Coding T Categories for Subsolid Nodules and Assessment of Tumor Size in Part-Solid Tumors in the Forthcoming Eighth Edition of the TNM Classification of Lung Cancer. J Thorac Oncol 2016;11:1204-23.
- de Margerie-Mellon C, Onken A, Heidinger BH, et al. CT Manifestations of Tumor Spread Through Airspaces in Pulmonary Adenocarcinomas Presenting as Subsolid Nodules. J Thorac Imaging 2018;33:402-8. [Crossref] [PubMed]
- Edge SB, Compton CC. The American Joint Committee on Cancer: the 7th edition of the AJCC cancer staging manual and the future of TNM. Ann Surg Oncol 2010;17:1471-4.
- Chen Y, Jiang C, Kang W, et al. Development and validation of a CT-based nomogram to predict spread through air space (STAS) in peripheral stage IA lung adenocarcinoma. Jpn J Radiol 2022;40:586-94. [Crossref] [PubMed]
- Tu W, Sun G, Fan L, et al. Radiomics signature: A potential and incremental predictor for EGFR mutation status in NSCLC patients, comparison with CT morphology. Lung Cancer 2019;132:28-35. [Crossref] [PubMed]
- Chen D, She Y, Wang T, et al. Radiomics-based prediction for tumour spread through air spaces in stage I lung adenocarcinoma using machine learning. Eur J Cardiothorac Surg 2020;58:51-8. [Crossref] [PubMed]
- Uruga H, Fujii T, Fujimori S, et al. Semiquantitative Assessment of Tumor Spread through Air Spaces (STAS) in Early-Stage Lung Adenocarcinomas. J Thorac Oncol 2017;12:1046-51. [Crossref] [PubMed]