Single-cell analysis and machine learning-based integration develop an immune-responsive signature of antigen-presenting cancer-associated fibroblasts in lung adenocarcinoma
Highlight box
Key findings
• We identified a novel antigen-presenting CAFs (apCAFs) subset associated with immune checkpoint inhibitors (ICIs) response.
What is known and what is new?
• Previous studies have focused primarily on CAF subpopulations that contribute to immune resistance and impaired responses to immunotherapy. Distinct CAF subsets have been characterized in various cancer types, often being associated with unfavorable outcomes.
• This study uncovers a previously unrecognized subset of macrophage-like apCAFs in lung adenocarcinoma (LUAD) that is linked to increased efficacy of ICIs. It highlights the importance of these apCAFs in the tumor microenvironment, showing that their presence can positively influence immune responses and treatment outcomes.
What is the implication, and what should change now?
• The findings highlight the critical role of macrophage-like apCAFs in boosting ICIs efficacy, indicating their potential as therapeutic targets.
• Clinical practices should integrate this immune response signature for better patient stratification in immunotherapy. Future research should focus on leveraging these apCAFs to enhance treatment responses in LUAD, shifting attention towards CAF subsets that positively influence immune responses.
Introduction
Background
Lung adenocarcinoma (LUAD) as the most common histological subtype of lung cancer induces more than one million deaths per years (1). Given the significant mortality rates associated with LUAD, identifying novel and more effective prognostic biomarkers is essential. This advancement will enhance personalized medicine approaches and potentially extend patient survival. Clinically, immunotherapies, such as programmed cell death protein 1 (PD-1) and programmed death-ligand 1 (PD-L1) monoclonal antibodies, represent a new breakthrough for anticancer treatment and have been the first-line treatment for advanced LUAD (2-4). However, like other therapies, immunotherapies show tremendous efficacy, but these responses only occur in a subset of patients (5,6), highlighting the urgent need to explore cellular signature and precise component for circumventing drug resistance in LUAD.
The tumor microenvironment (TME) significantly influences the outcomes of immunotherapeutic treatments for cancers (7). Cancer-associated fibroblasts (CAFs) are prevailing stromal cells in the TME of LUAD. Recent studies have shown that CAFs are highly molecularly heterogeneous, with distinct subsets performing different roles in tumor pathogenesis (8,9). Typical examples in pancreatic cancer or breast cancer include αSMA-high myofibroblastic CAFs (myCAFs) characterized by extracellular matrix (ECM) signatures and primarily located adjacent to cancer cells, αSMA-low inflammatory CAFs (iCAFs) expressing high levels of cytokines and chemokines and located more distal to cancer cells, and antigen-presenting CAFs (apCAFs) characterized by high expression of major histocompatibility complex (MHC) class II and CD74, significantly modulating tumor immunity (10-12). CAFs engage with tumor cells and immune cells through the secretion of cytokines and vesicles, actively participate in the remodeling of the ECM, and ultimately influence the immune response (13-15). Recent evidence suggests that CAFs can affect the tumor immune microenvironment (16) profiling and regulate therapeutic response to immune checkpoint inhibitors (ICIs) (17). CAFs are often viewed as cells that weaken the immune response, primarily due to their ability to inhibit CD8+ T cells and natural killer (NK) cells, while also promoting the activation of myeloid-derived suppressor cells (MDSCs) within the TME (18-20). However, the specific CAFs subsets within LUAD that favor therapeutic response to immunotherapies remain to be defined.
In our study, we employed the scissors algorithm, which can establish correlations between the phenotypes extracted from bulk RNA sequencing (RNA-seq) and the cell subpopulations associated with these phenotypes from single-cell data, to integrate a single-cell RNA sequencing (scRNA-seq) dataset (21) with bulk RNA-seq immunotherapy cohort (GSE126044) (22), identifying a subset of apCAFs that respond most favourably to immunotherapy. This group of cells not only expresses the conserved markers of apCAFs, such as CD74 and MHC class II (MHCII) molecules, but also co-stimulatory molecules like CD86. Furthermore, we utilized 101 machine learning algorithms to construct a robust signature that can predict patient survival and the efficacy of immunotherapy.
Rationale and knowledge gap
While existing research predominantly explores CAFs that hinder immune responses to therapies, there remains a significant knowledge gap regarding CAF subsets that enhance ICIs efficacy. This lack of focus on positively contributing CAF populations, particularly in LUAD, limits the potential for developing effective treatment strategies that leverage the TME to improve patient outcomes.
Objective
The objective of this study is to identify and characterize apCAF subsets associated with favorable ICIs responses in LUAD. By integrating bulk and scRNA-seq data, we aim to develop a robust machine learning-driven immune response signature to predict ICIs efficacy, thereby facilitating better patient stratification and informing targeted therapeutic approaches. We present this article in accordance with the TRIPOD reporting checklist (available at https://jtd.amegroups.com/article/view/10.21037/jtd-2024-2015/rc).
Methods
Multiplexed immunohistochemistry (IHC) and quantitative analysis
We collected samples of pathologically diagnosed lung cancer from Shanghai Chest Hospital, Shanghai Jiao Tong University School of Medicine. The clinical characteristics of the collected tumor samples used in multiplexed IHC are detailed in Table S1.
In multiplexed IHC, CD45, PanCK, CD68, CD86, and HLA-DR were defined. In brief, slide sections from formalin-fixed paraffin-embedded (FFPE) blocks underwent deparaffinization in xylene followed by rehydration using ethanol. Microwave-induced antigen retrieval was performed in ER1 antigen retrieval solution (AR9961-CN; Leica, Wetzlar, Germany) for 20 minutes at 100 ℃. Endogenous peroxidase activity was quenched using 3% H2O2 for 10 minutes. Non-specific binding sites were blocked using a blocking solution provided by WiSee Biotechnology (A10015-100; Shanghai, China) for an additional 10 minutes.
Primary antibodies were incubated in a moist chamber at room temperature for 60 minutes, followed by incubation with the secondary antibody (Leica Rabbit Anti-Rabbit Poly-HRP) for 10 minutes using the Leica DS9800 staining system. Slides were then incubated with the fluorescent dye Novo-Light TSA 620 (WiSee Biotechnology, Shanghai, China) at room temperature for 10 minutes for color development. The following primary antibodies were used: CD45 (1:100), PanCK (1:100), CD68 (1:100), CD86 (1:100), HLA-DR (1:300).
Detection and imaging of the slides were accomplished using the Pannoramic 3DHISTECH system (3DHISTECH, Budapest, Hungary), while HALO® software (Indica Labs, California, USA) was employed for batch analysis to calculate the count of positively stained cells bearing either a single marker or combination (23).
The study was conducted in accordance with the Declaration of Helsinki and its subsequent amendments. The study and the use of lung cancer tissue for this study was approved by the Institutional Review Board at the Shanghai Lung Tumor Clinical Medical Center, Shanghai Chest Hospital (Approval No. KS(Y)23072). Prior to the involvement in the study, informed consent was obtained from all participants.
Data acquisition
scRNA-seq data from four distinct lung cancer datasets were obtained from Gene Expression Omnibus (GEO) GSE131907 (21), alongside a Code Ocean capsule (https://doi.org/10.2433/CO.0121060.v1) (24). A scRNA-seq dataset (25) for NSCLC neoadjuvant PD-1 blockade combined with chemotherapy was acquired from GEO (GSE207422). Another single-cell cohort is derived from Zilionis et al. 2019 (26). We acquired survival data from lung bulk RNA-seq datasets available on the The Cancer Genome Atlas (TCGA) through the Genomic Data Commons (GDC) portal and GEO databases: TCGA-LUAD (27), GSE31210 (28), GSE72094 (29), GSE68465 (30), GSE8894 (31), GSE30219 (32) and GSE50081 (33). Another independent LUAD in East Asians datasets (34) with survival information was downloaded from the European Genomephenome Archive (EGA, http://www.ebi.ac.uk/ega/) using accession codes EGAD00001004421 and EGAD00001004422.
To validate the prediction of immunotherapy efficacy, two cohorts undergoing immunotherapeutic treatments were employed: GSE126044 were non-small cell lung cancer (NSCLC) patients who underwent therapy with anti-PD-1 (22). Advanced NSCLC patients, pertaining to the study GSE135222, received treatment utilizing anti-PD-1/PD-L1 (35). To validate the prediction of chemotherapy efficacy, three breast cancer datasets have been analyzed: GSE45255 (36), GSE3494 (37) and GSE1456 (38).
Single-cell data preprocessing
Fastq format sequences from high-throughput sequencing were processed using Cell Ranger (v7.0.1) for quality assessment and alignment to the reference genome (human: GRCh38). Data analysis was conducted using the Seurat R package (v4.3.0) (39). In a quality control step, cells were flagged as low-quality and excluded if they exhibited fewer than 300 or more than 7,000 detected genes, unique molecular identifier (UMI) counts exceeding 100,000, mitochondrial gene fractions surpassing 10%, or erythrocyte gene fractions exceeding 3%. Mitochondrial fractions were computed using Seurat’s Percentage FeatureSet function with pattern=“λMT-”. The same preprocessing pipeline was applied to all publicly available scRNA-seq datasets used in our study.
Dimension reduction, clustering and annotation analysis
The gene expression matrix underwent normalization using the “NormalizeData” function, followed by the identification of 3,000 highly variable genes (HVGs) using the “FindVariableFeatures” function. Batch effects were mitigated using the “Harmony” function. Principal component analysis (PCA) was employed to reduce dimensionality. The top 15 principal components were determined using the “ElbowPlot” function. Cell clustering was performed using the “FindNeighbors” and “FindClusters” functions with resolution specified by the “Clustree” algorithm (40). Clusters were visualized in a two-dimensional plot using the “RunUMAP” functions. Characteristic genes for each cell subgroup were identified using the “FindAllMarkers” function in Seurat, and cell types were defined based on marker genes using the SingleR package (v2.0.0) and literature references (marker genes shown in Table S2). This processing pipeline was also applied to all publicly available scRNA-seq datasets used in our study.
“Scissor” algorithm
Scissor (v2.0.0) was employed to blend phenotype-linked bulk expression data with single-cell data. Initially, it gauged the resemblance between each single cell and bulk sample, followed by refining a regression model on the correlation matrix alongside sample phenotype to pinpoint pertinent subpopulations. Additional insights can be gleaned from the referenced literature (41).
Gene set scoring
For scoring gene sets in scRNA-seq data, we employed the UCell package (v2.3.1) (42). Similarly, for bulk RNA-seq data, we utilized single-sample Gene Set Enrichment Analysis (ssGSEA) from the Gene Set Variation Analysis (GSVA) package (v1.46.0) (43) to compute signature scores for each sample. Supplementary tables contain all gene sets used for signature scoring.
Differential-expression analysis
In the analysis of the GSE131907 dataset (21), we used the Mast algorithm in “FindAllMarkers” function in Seurat to identify genes specific to CAF-C3 and CAF-C14. We defined an immunotherapy nonresponsive signature gene set with 21 genes from differential genes with log2fold change (FC) exceeding 0.6 and adjusted P below 0.01 (listed in Table S3). Similarly, we defined an immunotherapy-responsive signature gene set with 17 genes from differential genes exhibiting log2FC below −1.3 and adjusted P values under 0.01 (listed in Table S3).
To discern genes differentially expressed between clusters, we employed Seurat’s “FindAllMarkers” function (v4.3.0) with parameters logfc.threshold =0.25 and only.pos = T. Utilizing the non-parametric Wilcoxon rank-sum test, we obtained P values for comparisons and adjusted them using Bonferroni correction across all genes. We selected the top 20 markers as signature gene sets for CAF clusters in GSE131907 (21) detailed in Table S4.
Gene ontology (GO) enrichment analysis
We conducted GO enrichment analysis using the clusterProfiler package (v4.6.0) (44). Subsequently, representative pathways were visualized using the ggplot2 package (v3.4.1).
Gene set enrichment analysis (GSEA)
We downloaded Hallmark, GO, and Kyoto Encyclopedia of Genes and Genomes (KEGG) gene sets from The Molecular Signatures Database (45) (MSigDB, http://software.broadinstitute.org/gsea/msigdb/). Subsequently, we conducted GSEA for differentially expressed genes (DEGs) using the clusterProfiler package (v4.6.0) and the GSEABase toolkit (v1.60.0) in R, employing default settings (46,47). Significantly enriched pathways were identified based on criteria including P<0.05, P.adjust <0.25, and NES >1.
Cell Type Identification by Estimating Relative Subsets of RNA Transcripts (CIBERSORT)
We utilized R to perform CIBERSORT (48), estimating the abundance of 22 immune cell subsets in each sample. Input the expression matrix of bulk RNA-seq and LM22.txt, which records the gene expression profiles of 22 immune cell types, including 7 types of T cells, naïve and memory B cells, plasma cells, NK cells, and myeloid cells.
xCell
Immune infiltration analysis was conducted using the xCell package (v1.1.0) (49). This package employs a deconvolution method to perform cell-type enrichment analysis on bulk transcriptome samples based on gene expression data of 64 immune and stromal cell types, generating enrichment scores corresponding to each of the 64 cell types for each sample.
Estimation of STromal and Immune cells in MAlignant Tumor tissues using Expression data (ESTIMATE)
Immune score, tumor purity, stromal score and ESTIMATE score were evaluated using the ESTIMATE package (v1.0.13) (50).
Machine learning-based integrative analysis
In order to create a highly accurate and stable ICI-responsive signature from CAF-C14, we combined 10 different machine learning algorithms with 101 algorithm variations. The integrative algorithms included random survival forest (RSF), elastic network (Enet), Lasso, Ridge, stepwise Cox, CoxBoost, partial least squares regression for Cox (plsRcox), supervised principal components (SuperPC), generalised boosted regression modelling (GBM), and survival support vector machine (survival-SVM). The process for generating the signature was carried out as follows: (I) incorporate the DEGs (listed in Table S5) from CAF-C3 and CAF-C14 exhibiting log2FC below −1 and adjusted P values under 0.01 into the univariate cox regression; (II) prognostic genes were identified through univariate Cox regression analysis in the TCGA-LUAD cohort (listed in Table S6); (III) using the leave-one-out cross-validation (LOOCV) approach, we applied 101 algorithm combinations to the prognostic genes to develop predictive models in the TCGA-LUAD cohort (27); (IV) all models were evaluated in five validation datasets [GSE31210 (28), GSE72094 (29), GSE68465 (30), GSE30219 (32) and GSE50081 (33)]; (V) the Harrell’s concordance index (C-index) was computed for each model across all validation datasets, with the model exhibiting the highest average C-index deemed the most optimal. C-index listed in Table S7.
Survival analysis
We conducted survival analyses to assess the clinical significance of various signatures including immunotherapy nonresponsive, immunotherapy responsive and risk scores from machine learning-based integrative analysis. Bulk RNA samples were processed as follows: gene expression values were converted to Fragments Per Kilobase Million (FPKM) and subjected to log-transformation. Z-scores were subsequently computed for each gene across all samples. We utilized ssGSEA from the GSVA package (v1.46.0) (43) to compute signature scores for each sample. Subsequently, based on the cutoff values of the signature scores determined using the survminer package (v0.4.9), samples were divided into two groups. We employed the Kaplan-Meier method in survival package (v3.3-1) to estimate survival curves for both patient groups and assessed statistical significance using the log-rank test.
Statistical analyses
Statistical evaluations were undertaken with GraphPad Prism 9.0 software and R software (v4.2.0).
Results
Single-cell analysis identified heterogeneous subpopulations of CAFs in LUAD
We analyzed a large public scRNA-seq dataset of LUAD (the Kim et al. cohort, n=58) (21) and focused on primary LUAD samples (n=15), excluding those from metastatic sites. Through dimensionality reduction and clustering techniques, we identified 19 distinct clusters (Figure 1A). These clusters were annotated according to the expression of specific marker genes, categorizing them into T cells, NK cells, B cells, myeloid cells, mast cells, fibroblasts, endothelial cells, and epithelial cells (Figure 1B,1C and Table S2).
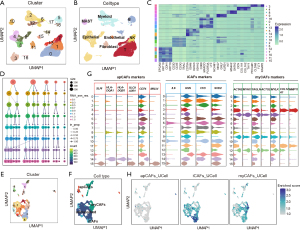
We then focused on exploring the cellular heterogeneity among CAF subpopulations (n=1,813). Using the “Clustree” algorithm (40) to determine the ideal number of CAF clusters (Figure 1D), we employed an unsupervised graph-based clustering approach that revealed 16 distinct subclusters, which were visualized using the Uniform Manifold Approximation and Projection (UMAP) algorithm (Figure 1E). Afterward, we applied the gene signatures corresponding to myCAFs, iCAFs, and apCAFs to Kim et al. cohort (Table S2). This analysis enabled us to categorize three clusters (C6, C10, C13) within the iCAFs subgroup, five clusters (C3, C4, C9, C11, C12) as myCAFs, and three clusters (C8, C14, C15) as apCAFs (Figure 1F,1G). The remaining clusters (C0, C1, C2, C5, C7) were identified as unclassified CAFs. Additionally, we also utilized the UCell algorithm (42), an R package for evaluating gene signature enrichment in scRNA-seq data, to verify the accuracy of our annotations (Figure 1H, Table S3).
Identifying CAF subsets that predict immunotherapy response
To identify the key CAF subsets correlated with the ICIs response, we employed Scissor (41), a robust algorithm that allows us to establish correlations between the phenotypes extracted from bulk RNA-seq data and the cell subpopulations associated with these phenotypes from single-cell data.
Based on bulk RNA-seq data from GSE126044 NSCLC ICI therapy dataset (22), we identified 36 CAF cells as Scissor+ cells, linked to favorable response to ICIs (termed Scissor_R cells thereafter), and 63 CAF cells associated with non-response to ICIs (termed Scissor_NR cells thereafter) (Figure 2A). Strikingly, the Scissor_R cells were predominantly localized within the CAF-C14 subset, indicating that this subset is closely associated with effective responses to treatment. In contrast, Scissor_NR cells were primarily found within the CAF-C3 subset, suggesting that CAF-C3 may play a critical role in mediating resistance to ICIs (Figure 2B).
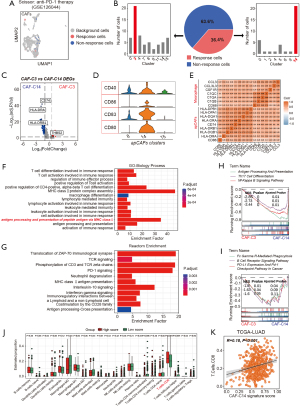
Furthermore, we performed a comparative analysis of the transcriptional profiles of CAF-C3 and CAF-C14 to identify their distinct gene signatures. Genes significantly upregulated in CAF-C3 were designated as the ICIs-non-responsive signature, whereas those significantly upregulated in CAF-C14 were classified as the ICIs-responsive signature (Table S3). Specifically, CAF-C14 exhibited a predominant upregulation of genes associated with MHCII pathways (HLA-DPB1, HLA-DPA1, HLA-DRA, HLA-DQA1, HLA-DQB1, HLA-DRB1 and CD74) (Figure 2C). THBS2 was identified as the second highest-ranked gene of CAF-C3. Our recent studies highlighted the role of THBS2+ CAFs in promoting tumor aggressiveness in early-stage LUAD, shaping a suppressive immune microenvironment, and predicting resistance to ICIs (51). In our previous research (51), we extensively investigated the specific functional characteristics of THBS2+ CAFs (CAF-C3). However, limited information has been elucidated regarding CAFs associated with favorable responses to immunotherapy. Therefore, this study focuses on CAF-C14, which has demonstrated a strong association with positive immunotherapeutic outcomes. Molecular characterization analysis revealed that the top 5 biomarkers in CAF-C14 include CD74 and MHC-II-related genes: HLA-DRA, HLA-DRB1, TYROBP and HLA-DPB1, compared with CAF-C3 (Figure 2C). The high expression levels of CD74 and MHC-II genes in CAF-C14 are reminiscent of apCAFs, which have been recently identified in breast and pancreatic cancer (10-12).
Notably, a previous study has described that “anti-presenting CAFs” express MHCII and CD74, while not classic costimulatory molecules (CD86, CD83, CD80, CD40) in pancreatic cancer (11). In contrast, our analysis in LUAD showed that CAF-C14 express co-stimulatory molecules (Figure 2D). Moreover, CAF-C14 had high expression of macrophage markers (C1QA, C1QB, C1QC, CD68, CD86). Further, there is a strong correlation between the expression levels of macrophage markers and MCH-II molecules (Figure 2E), suggesting a “macrophage-like” status of CAF-C14. Therefore, we termed this cell population macrophage-like apCAFs.
GO analysis revealed that CAF-C14 was mainly enriched for activation of immune response and antigen processing, presentation via MHCII compared with CAF-C3 (Figure 2F). The Reactome enrichment analyses of CAF-C14 DEGs were predominantly involved in the immune response signals, including phosphorylation of CD3 and TCR zeta chains and PD-1 signaling (Figure 2G). Additionally, GSEA showed that CAF-C14 cells had higher enrichment for antigen processing and presentation, B cell receptor signaling pathway, and PD-L1 expression and PD-1 checkpoint pathways (Figure 2H,2I). Among various immune cell compositions analyzed, we observed a significantly higher proportion of CD8+ T cells in patients with elevated CAF-C14 signature scores compared to those with lower scores (Figure 2J). Furthermore, the infiltration of CD8+ T cells displayed a notable positive correlation with the signature derived from the CAF-C14 (Figure 2K). Together, analysis suggests that CAF-C14 may play a role in recruiting, expanding, and activating CD8+ T cells. This observation indicates a potential link between the presence of CAF-C14 and favorable responses to ICIs.
CAF-C14 is associated with good immunotherapy response
Next, survival analysis in multiple independent ICIs datasets confirmed that CAF-C14-derived signature (associated with ICIs responsive signature; Table S3) predicted better response or longer survival after ICI therapy (35,52-54) (Figure 3A; Figure S1A-S1C). Using the median gene signature score of CAF-C14 cells and CAF-C3 cells (associated with ICIs non-responsive or responsive signature; Table S3) as a cutoff, patients were stratified into four biomarker subgroups. Interestingly, we observed that CAF-C14Lo/CAF-C3Hi defined a subgroup with the worst prognosis, while CAF-C14Hi/CAF-C3Lo was associated with high overall survival (OS) after immunotherapy in one clinical cohort (54) (Figure S1D).
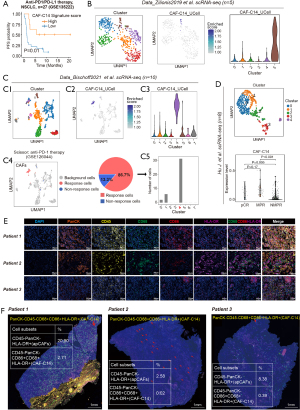
The existence of CAF-C14 has been validated in the scRNA-seq cohort by Zilionis et al. (26). We performed a clustering analysis on 447 CAFs, which resulted in the discovery of five unique CAF subpopulations (Figure 3B). By utilizing the gene signature from the top 20 markers of CAF-C14 (shown in Table S4), our analysis with feature plots and violin plots demonstrated that Cluster 5 corresponded to CAF-C14 (Figure 3B). The presence of CAF-C14 has also been confirmed in the Bischoff et al. scRNA-seq cohort (24), involving 10 individuals diagnosed with early-stage LUAD. We conducted UMAP analysis of 672 CAFs to identify nine distinct CAF subpopulations (Figure 3C). We employed the gene signature (Table S4) consisting of the top 20 markers CAF-C14, feature plots in combination with violin plots revealed that Cluster 4 signified CAF-C14 (Figure 3C). Similar with Kim et al. cohort, integrated with bulk RNA-seq data from GSE126044 NSCLC ICI therapy dataset (22), we identified 39 CAF cells as Scissor+ cells, linked to response to ICIs (termed Scissor_R cells thereafter), and 6 CAF cells associated with non-response to ICIs (termed Scissor_NR cells thereafter) (Figure 3C). Strikingly, the Scissor_R cells predominantly localized within Cluster 4, signifying that this subset was primarily associated with effective responses (Figure 3C).
Additionally, these findings were also confirmed in the neoadjuvant ICI treatment setting. Based on a previously published single-cell dataset (25) from two pre-treatment and six post-treatment LUAD patients, including major pathologic response (MPR; n=1), non-MPR (NMPR; n=6) and pathologic complete response (pCR; n=1). To assess CAFs diversity after ICI therapy, we clustered CAFs into five subclusters (Figure 3D). Using the CAF-C14 signatures (Table S3) to score CAF cells in this new scRNA-seq dataset, CAF cells from pCR and MPR sources exhibited higher CAF-C14 scores (Figure 3D). Together, our analysis identified CAF-C14 subsets that were associated with good response to ICI therapy.
Importantly, the presence of macrophage-like apCAFs was confirmed in human LUAD tissue, as multiplexed immunohistochemistry (mIHC) analysis by co-staining epithelial (PanCK), hematopoietic (CD45), macrophage (CD68), costimulatory molecule (CD86) and MHCII (HLA-DR) markers showed that (Figure 3E; Figure S1E) the percentage of “macro-like apCAFs” (PanCK−CD45−CD68+CD86+HLA−DR+) in three randomly selected LUAD samples was 2.71% 0.02% and 0.39% of the entire cells, respectively (Figure 3F).
A predictive model based on the macrophage-like apCAFs (CAF-C14) assesses survival and responses to ICIs therapy
Utilizing the expression data from 58 ICIs responsive genes derived from the DEGs between CAF-C3 and CAF-C14 (refer to Table S5), a univariate Cox regression analysis was conducted in the TCGA-LUAD dataset, resulting in the identification of 16 genes with prognostic significance (Figure 4A; Table S6). The 16 identified genes underwent a machine learning-driven integrative approach to construct an immune-related signature. Using the TCGA-LUAD dataset, we applied a LOOCV framework to evaluate 101 different prediction models and subsequently determined the C-index for each model across all validation sets (Figure 4B; Table S7). Notably, the best-performing model emerged from the integration of Lasso with SuperPC, as well as CoxBoost combined with SuperPC, achieving the highest average C-index of 0.59. This hybrid model also attained the top C-index across all validation datasets (Figure 4B). The optimal λ in the Lasso regression was determined when the partial likelihood deviance reached its lowest point, as assessed through the LOOCV approach (Figure 4C). Four genes (HLA-DMA, ADH1B, CLEC7A and CXCR4) with nonzero coefficients from the Lasso regression were analyzed using SuperPC. Following this, we derived a risk score for each patient using the integrated Lasso and SuperPC model. Based on the optimal cut-off value established by the survminer package, patients were divided into low-risk and high-risk groups. According to Figure 4D-4I and Figure S2A-S2G, patients identified as high-risk had significantly reduced OS, progression-free survival (PFS), disease-specific survival (DSS) or disease-free survival (DFS) compared to their low-risk counterparts in both the TCGA-LUAD training dataset, seven validation datasets (all P<0.05). Nevertheless, the results from the multivariate Cox regression analysis showed that the risk score derived from macrophage-like apCAFs was statistically significant in only 5 of the 8 studied cohorts (Figure S2A-S2G). The receiver operating characteristic (ROC) analysis evaluated the ability of the risk score based on macrophage-like apCAFs to discriminate among outcomes. In the TCGA-LUAD dataset, the areas under the curve (AUCs) were recorded at 0.640 for 1 year, 0.550 for 3 years, and 0.590 for 5 years. In the GSE68465 cohort, the values were 0.60 across the first two years and 0.59 for the fifth. For GSE72904, the AUCs were 0.62, 0.66, and 0.66 for 1, 3, and 5 years respectively. The GSE13210 dataset showed values of 0.73 for 1 year, 0.71 for 3 years, and 0.61 for 5 years. In GSE30219, the AUCs were 0.63, 0.60, and 0.59, while for GSE50018, they were 0.53, 0.57, and 0.59 (Figure 4J). We evaluated the predictive effectiveness of the machine-learning based risk signature in an immunotherapy dataset, where Kaplan-Meier analysis showed that patients in the high-risk group had poor OS (Figure 4K). Also, patients in high-risk group have worse outcomes with chemotherapy (Figure S2H-S2K). Therefore, the machine-learning based risk signature derived from CAF-C14 demonstrated a notable level of predictive capability.
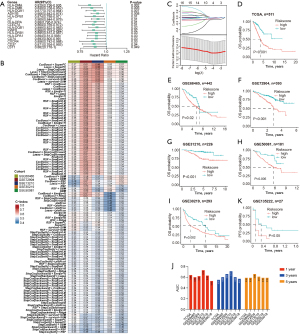
Immune-related differences based on CAF-C14 in LUAD
Recognizing the variations in the tumor immune microenvironment between the low-risk and high-risk subgroups, we conducted an extensive analysis of immune-related differences across multiple cohorts. Our differential analysis of immune cell infiltration in both groups revealed that the high-risk subgroup had significantly lower scores for CD8+ T cells and ImmuneScore, as well as elevated tumor purity scores (Figure 5A-5G). Subsequently, in the GSE135222 cohort, we divided patients according to their risk scores, and consistent with our prior findings, those in the high-risk category exhibited comparable results (Figure 5H). In addition, populations in the TCGA-LUAD dataset classified as low-risk exhibited enhanced antigen-presenting abilities and increased levels of co-stimulatory molecules (Figure 5I). Moreover, histological examination through hematoxylin and eosin staining revealed a widespread distribution of active diffuse lymphocytes in low-risk LUAD patients, in contrast to the concentrated focal lymphocyte presence primarily observed in high-risk patients (Figure 5J).
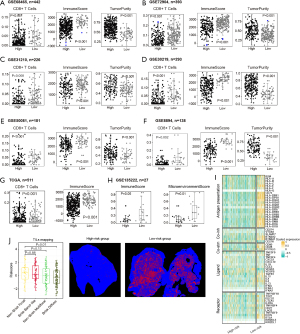
Discussion
Key findings
CAFs have been widely studied and play a critical role in immunotherapy resistance (15,55-57). Emerging evidence has highlighted the heterogeneity of CAFs, which might become a tumor immune barrier in the TME. However, the specific CAF subsets underlying the resistance to immunotherapy are poorly understood. In this study, by integrating scRNA-seq, bulk RNA-seq datasets and mIHC, we proposed one CAFs subset that named “macro-like apCAFs”, which might be instrumental for clinical patient stratification and optimization of the therapy strategies.
Strengths and limitations
Enhancing treatment effectiveness and identifying reliable biomarkers are significant challenges in immunotherapy. Despite the breakthrough of ICI therapy in advanced NSCLC, many patients remain unresponsive to this treatment (58-61). The heterogeneity of CAFs plays an important role in establishing an immunosuppressive TME and limiting the efficacy of immunotherapy strategies (10,62,63). It remains to be determined which CAF subtypes are responsible for this in LUAD. In pancreatic ductal adenocarcinoma and breast cancer, apCAFs, characterized by the expression of MHCII proteins, assume an immunomodulatory role (11,64). Notably, MHCII-expressing CAFs lack the co-stimulatory molecules typically found in professional antigen-presenting cells (APCs) (11,64), which are essential for initiating the clonal proliferation of CD4 T cells (65). This suggests that apCAFs could function as decoy receptors, impeding optimal T cell responses. However, in human NSCLC, antigen-presenting fibroblasts seem to actively promote rather than suppress MHCII immunity (66). Interestingly, our study described a new population of apCAFs that not only expressed CD74 and MHC class II but also exhibited co-stimulatory molecules (CD80, CD86, CD83 and CD40) along with macrophage markers. We termed this subcluster “Macro-like apCAFs” and confirmed their presence in LUAD patient samples. This finding underscores the intricate immunomodulatory functions within the TME. Targeting apCAFs or modulating their activity could potentially enhance the effectiveness of immunotherapy by alleviating the inhibitory signals they might transmit to T cells. However, this study has several limitations. It primarily relies on existing RNA-seq data, which may not fully reflect the dynamic TME in vivo. Additionally, there is a lack of experimental validation for the predictive signature in larger and more diverse patient populations.
Comparison with similar researches
This study contrasts with previous research by identifying a distinct subset of apCAFs linked to favorable ICIs responses in LUAD. While earlier studies focused on CAFs that impair immune responses, we emphasize the therapeutic potential of macrophage-like apCAFs.
Explanations of findings
In summary, our research on CAFs reveals their role in immune therapy responses, highlighting the importance of understanding CAF heterogeneity in stratifying LUAD patients. Additionally, we constructed a risk scoring model that can predict patient efficacy and survival. These findings provide valuable insights that can guide the future development of more precise and effective cancer therapies.
Implications and actions needed
The findings highlight the importance of targeting macrophage-like apCAFs to enhance ICIs efficacy and improve patient outcomes in LUAD. Future actions include validating our immune response signature in clinical settings and exploring therapeutic strategies that modulate the TME to harness the benefits of this CAFs subset.
Conclusions
In conclusion, our study underscores the critical role of CAFs in influencing the efficacy of immunotherapy in LUAD. By identifying and characterizing the novel “macro-like apCAFs”, which possess both antigen-presenting and co-stimulatory properties, we have shed light on the complex interplay within the TME that affects immune responses. Understanding the heterogeneity of CAFs is essential for delineating the mechanisms behind immunotherapy resistance and for developing targeted approaches to enhance treatment outcomes. Furthermore, our risk scoring model offers a promising tool for predicting patient responses and survival chances, paving the way for more personalized cancer treatment strategies. Collectively, these insights not only advance our knowledge of CAF biology but also hold potential for improving therapeutic interventions in LUAD, ultimately contributing to better patient management and outcomes in the realm of cancer immunotherapy.
Acknowledgments
None.
Footnote
Reporting Checklist: The authors have completed the TRIPOD reporting checklist. Available at https://jtd.amegroups.com/article/view/10.21037/jtd-2024-2015/rc
Data Sharing Statement: Available at https://jtd.amegroups.com/article/view/10.21037/jtd-2024-2015/dss
Peer Review File: Available at https://jtd.amegroups.com/article/view/10.21037/jtd-2024-2015/prf
Funding: This work was supported by
Conflicts of Interest: All authors have completed the ICMJE uniform disclosure form (available at https://jtd.amegroups.com/article/view/10.21037/jtd-2024-2015/coif). The authors have no conflicts of interest to declare.
Ethical Statement: The authors are accountable for all aspects of the work in ensuring that questions related to the accuracy or integrity of any part of the work are appropriately investigated and resolved. The study was conducted in accordance with the Declaration of Helsinki and its subsequent amendments. The study and the use of lung cancer tissue for this study was approved by the Institutional Review Board at the Shanghai Lung Tumor Clinical Medical Center, Shanghai Chest Hospital (Approval No. KS(Y)23072). Prior to the involvement in the study, informed consent was obtained from all participants.
Open Access Statement: This is an Open Access article distributed in accordance with the Creative Commons Attribution-NonCommercial-NoDerivs 4.0 International License (CC BY-NC-ND 4.0), which permits the non-commercial replication and distribution of the article with the strict proviso that no changes or edits are made and the original work is properly cited (including links to both the formal publication through the relevant DOI and the license). See: https://creativecommons.org/licenses/by-nc-nd/4.0/.
References
- Siegel RL, Giaquinto AN, Jemal A. Cancer statistics, 2024. CA Cancer J Clin 2024;74:12-49. [Crossref] [PubMed]
- Grigg C, Rizvi NA. PD-L1 biomarker testing for non-small cell lung cancer: truth or fiction? J Immunother Cancer 2016;4:48. [Crossref] [PubMed]
- Martinez P, Peters S, Stammers T, et al. Immunotherapy for the First-Line Treatment of Patients with Metastatic Non-Small Cell Lung Cancer. Clin Cancer Res 2019;25:2691-8. [Crossref] [PubMed]
- Ribas A, Wolchok JD. Cancer immunotherapy using checkpoint blockade. Science 2018;359:1350-5. [Crossref] [PubMed]
- Yang F, Wang Y, Tang L, et al. Efficacy of immune checkpoint inhibitors in non-small cell lung cancer: A systematic review and meta-analysis. Front Oncol 2022;12:955440. [Crossref] [PubMed]
- O'Donnell JS, Teng MWL, Smyth MJ. Cancer immunoediting and resistance to T cell-based immunotherapy. Nat Rev Clin Oncol 2019;16:151-67. [Crossref] [PubMed]
- Pei L, Liu Y, Liu L, et al. Roles of cancer-associated fibroblasts (CAFs) in anti- PD-1/PD-L1 immunotherapy for solid cancers. Mol Cancer 2023;22:29. [Crossref] [PubMed]
- Chhabra Y, Weeraratna AT. Fibroblasts in cancer: Unity in heterogeneity. Cell 2023;186:1580-609. [Crossref] [PubMed]
- Lavie D, Ben-Shmuel A, Erez N, et al. Cancer-associated fibroblasts in the single-cell era. Nat Cancer 2022;3:793-807. [Crossref] [PubMed]
- Davidson S, Coles M, Thomas T, et al. Fibroblasts as immune regulators in infection, inflammation and cancer. Nat Rev Immunol 2021;21:704-17. [Crossref] [PubMed]
- Öhlund D, Handly-Santana A, Biffi G, et al. Distinct populations of inflammatory fibroblasts and myofibroblasts in pancreatic cancer. J Exp Med 2017;214:579-96. [Crossref] [PubMed]
- Elyada E, Bolisetty M, Laise P, et al. Cross-Species Single-Cell Analysis of Pancreatic Ductal Adenocarcinoma Reveals Antigen-Presenting Cancer-Associated Fibroblasts. Cancer Discov 2019;9:1102-23. [Crossref] [PubMed]
- Ping Q, Yan R, Cheng X, et al. Cancer-associated fibroblasts: overview, progress, challenges, and directions. Cancer Gene Ther 2021;28:984-99. [Crossref] [PubMed]
- Bu L, Baba H, Yoshida N, et al. Biological heterogeneity and versatility of cancer-associated fibroblasts in the tumor microenvironment. Oncogene 2019;38:4887-901. [Crossref] [PubMed]
- Öhlund D, Elyada E, Tuveson D. Fibroblast heterogeneity in the cancer wound. J Exp Med 2014;211:1503-23. [Crossref] [PubMed]
- Sikkema L, Ramírez-Suástegui C, Strobl DC, et al. An integrated cell atlas of the lung in health and disease. Nat Med 2023;29:1563-77. [Crossref] [PubMed]
- An Y, Liu F, Chen Y, et al. Crosstalk between cancer-associated fibroblasts and immune cells in cancer. J Cell Mol Med 2020;24:13-24. [Crossref] [PubMed]
- Barrett R, Puré E. Cancer-associated fibroblasts: key determinants of tumor immunity and immunotherapy. Curr Opin Immunol 2020;64:80-7. [Crossref] [PubMed]
- Xiang H, Ramil CP, Hai J, et al. Cancer-Associated Fibroblasts Promote Immunosuppression by Inducing ROS-Generating Monocytic MDSCs in Lung Squamous Cell Carcinoma. Cancer Immunol Res 2020;8:436-50. [Crossref] [PubMed]
- Zhang H, Yue X, Chen Z, et al. Define cancer-associated fibroblasts (CAFs) in the tumor microenvironment: new opportunities in cancer immunotherapy and advances in clinical trials. Mol Cancer 2023;22:159. [Crossref] [PubMed]
- Kim N, Kim HK, Lee K, et al. Single-cell RNA sequencing demonstrates the molecular and cellular reprogramming of metastatic lung adenocarcinoma. Nat Commun 2020;11:2285. [Crossref] [PubMed]
- Cho JW, Hong MH, Ha SJ, et al. Genome-wide identification of differentially methylated promoters and enhancers associated with response to anti-PD-1 therapy in non-small cell lung cancer. Exp Mol Med 2020;52:1550-63. [Crossref] [PubMed]
- Yang H, Sun B, Ma W, et al. Multi-scale characterization of tumor-draining lymph nodes in resectable lung cancer treated with neoadjuvant immune checkpoint inhibitors. EBioMedicine 2022;84:104265. [Crossref] [PubMed]
- Bischoff P, Trinks A, Obermayer B, et al. Single-cell RNA sequencing reveals distinct tumor microenvironmental patterns in lung adenocarcinoma. Oncogene 2021;40:6748-58. [Crossref] [PubMed]
- Hu J, Zhang L, Xia H, et al. Tumor microenvironment remodeling after neoadjuvant immunotherapy in non-small cell lung cancer revealed by single-cell RNA sequencing. Genome Med 2023;15:14. [Crossref] [PubMed]
- Zilionis R, Engblom C, Pfirschke C, et al. Single-Cell Transcriptomics of Human and Mouse Lung Cancers Reveals Conserved Myeloid Populations across Individuals and Species. Immunity 2019;50:1317-1334.e10. [Crossref] [PubMed]
- Comprehensive molecular profiling of lung adenocarcinoma. Nature 2014;511:543-50. [Crossref] [PubMed]
- Okayama H, Kohno T, Ishii Y, et al. Identification of genes upregulated in ALK-positive and EGFR/KRAS/ALK-negative lung adenocarcinomas. Cancer Res 2012;72:100-11. [Crossref] [PubMed]
- Schabath MB, Welsh EA, Fulp WJ, et al. Differential association of STK11 and TP53 with KRAS mutation-associated gene expression, proliferation and immune surveillance in lung adenocarcinoma. Oncogene 2016;35:3209-16. [Crossref] [PubMed]
- Director's Challenge Consortium for the Molecular Classification of Lung Adenocarcinoma. Gene expression-based survival prediction in lung adenocarcinoma: a multi-site, blinded validation study. Nat Med 2008;14:822-7. [Crossref] [PubMed]
- Lee ES, Son DS, Kim SH, et al. Prediction of recurrence-free survival in postoperative non-small cell lung cancer patients by using an integrated model of clinical information and gene expression. Clin Cancer Res 2008;14:7397-404. [Crossref] [PubMed]
- Rousseaux S, Debernardi A, Jacquiau B, et al. Ectopic activation of germline and placental genes identifies aggressive metastasis-prone lung cancers. Sci Transl Med 2013;5:186ra66. [Crossref] [PubMed]
- Der SD, Sykes J, Pintilie M, et al. Validation of a histology-independent prognostic gene signature for early-stage, non-small-cell lung cancer including stage IA patients. J Thorac Oncol 2014;9:59-64. [Crossref] [PubMed]
- Chen J, Yang H, Teo ASM, et al. Genomic landscape of lung adenocarcinoma in East Asians. Nat Genet 2020;52:177-86. [Crossref] [PubMed]
- Jung H, Kim HS, Kim JY, et al. DNA methylation loss promotes immune evasion of tumours with high mutation and copy number load. Nat Commun 2019;10:4278. [Crossref] [PubMed]
- Nagalla S, Chou JW, Willingham MC, et al. Interactions between immunity, proliferation and molecular subtype in breast cancer prognosis. Genome Biol 2013;14:R34. [Crossref] [PubMed]
- Miller LD, Smeds J, George J, et al. An expression signature for p53 status in human breast cancer predicts mutation status, transcriptional effects, and patient survival. Proc Natl Acad Sci U S A 2005;102:13550-5. [Crossref] [PubMed]
- Pawitan Y, Bjöhle J, Amler L, et al. Gene expression profiling spares early breast cancer patients from adjuvant therapy: derived and validated in two population-based cohorts. Breast Cancer Res 2005;7:R953-64. [Crossref] [PubMed]
- Stuart T, Butler A, Hoffman P, et al. Comprehensive Integration of Single-Cell Data. Cell 2019;177:1888-1902.e21. [Crossref] [PubMed]
- Zappia L, Oshlack A. Clustering trees: a visualization for evaluating clusterings at multiple resolutions. Gigascience 2018;7:giy083. [Crossref] [PubMed]
- Sun D, Guan X, Moran AE, et al. Identifying phenotype-associated subpopulations by integrating bulk and single-cell sequencing data. Nat Biotechnol 2022;40:527-38. [Crossref] [PubMed]
- Andreatta M, Carmona SJ. UCell: Robust and scalable single-cell gene signature scoring. Comput Struct Biotechnol J 2021;19:3796-8. [Crossref] [PubMed]
- Hänzelmann S, Castelo R, Guinney J. GSVA: gene set variation analysis for microarray and RNA-seq data. BMC Bioinformatics 2013;14:7. [Crossref] [PubMed]
- Wu T, Hu E, Xu S, et al. clusterProfiler 4.0: A universal enrichment tool for interpreting omics data. Innovation (Camb) 2021;2:100141. [Crossref] [PubMed]
- Liberzon A, Birger C, Thorvaldsdóttir H, et al. The Molecular Signatures Database (MSigDB) hallmark gene set collection. Cell Syst 2015;1:417-25. [Crossref] [PubMed]
- Yang H, Hall SRR, Sun B, et al. NF2 and Canonical Hippo-YAP Pathway Define Distinct Tumor Subsets Characterized by Different Immune Deficiency and Treatment Implications in Human Pleural Mesothelioma. Cancers (Basel) 2021;13:1561. [Crossref] [PubMed]
- Yang H, Sun B, Xu K, et al. Pharmaco-transcriptomic correlation analysis reveals novel responsive signatures to HDAC inhibitors and identifies Dasatinib as a synergistic interactor in small-cell lung cancer. EBioMedicine 2021;69:103457. [Crossref] [PubMed]
- Newman AM, Liu CL, Green MR, et al. Robust enumeration of cell subsets from tissue expression profiles. Nat Methods 2015;12:453-7. [Crossref] [PubMed]
- Aran D, Hu Z, Butte AJ. xCell: digitally portraying the tissue cellular heterogeneity landscape. Genome Biol 2017;18:220. [Crossref] [PubMed]
- Yoshihara K, Shahmoradgoli M, Martínez E, et al. Inferring tumour purity and stromal and immune cell admixture from expression data. Nat Commun 2013;4:2612. [Crossref] [PubMed]
- Yang H, Sun B, Fan L, et al. Multi-scale integrative analyses identify THBS2(+) cancer-associated fibroblasts as a key orchestrator promoting aggressiveness in early-stage lung adenocarcinoma. Theranostics 2022;12:3104-30. [Crossref] [PubMed]
- Riaz N, Havel JJ, Makarov V, et al. Tumor and Microenvironment Evolution during Immunotherapy with Nivolumab. Cell 2017;171:934-949.e16. [Crossref] [PubMed]
- Ulloa-Montoya F, Louahed J, Dizier B, et al. Predictive gene signature in MAGE-A3 antigen-specific cancer immunotherapy. J Clin Oncol 2013;31:2388-95. [Crossref] [PubMed]
- Lauss M, Donia M, Harbst K, et al. Mutational and putative neoantigen load predict clinical benefit of adoptive T cell therapy in melanoma. Nat Commun 2017;8:1738. [Crossref] [PubMed]
- Barrett RL, Puré E. Cancer-associated fibroblasts and their influence on tumor immunity and immunotherapy. Elife 2020;9:e57243. [Crossref] [PubMed]
- Kalluri R. The biology and function of fibroblasts in cancer. Nat Rev Cancer 2016;16:582-98. [Crossref] [PubMed]
- Sahai E, Astsaturov I, Cukierman E, et al. A framework for advancing our understanding of cancer-associated fibroblasts. Nat Rev Cancer 2020;20:174-86. [Crossref] [PubMed]
- Xu K, Yang H, Ma W, et al. Neoadjuvant immunotherapy facilitates resection of surgically-challenging lung squamous cell cancer. J Thorac Dis 2021;13:6816-26. [Crossref] [PubMed]
- Xu K, Ma J, Hall SRR, et al. Battles against aberrant KEAP1-NRF2 signaling in lung cancer: intertwined metabolic and immune networks. Theranostics 2023;13:704-23. [Crossref] [PubMed]
- Yang H, Ma W, Sun B, et al. Smoking signature is superior to programmed death-ligand 1 expression in predicting pathological response to neoadjuvant immunotherapy in lung cancer patients. Transl Lung Cancer Res 2021;10:3807-22. [Crossref] [PubMed]
- Yang Z, Wang S, Yang H, et al. Treatment patterns and clinical outcomes of patients with resectable non-small cell lung cancer receiving neoadjuvant immunochemotherapy: A large-scale, multicenter, real-world study (NeoR-World). J Thorac Cardiovasc Surg 2024;168:1245-1258.e17. [Crossref] [PubMed]
- Grout JA, Sirven P, Leader AM, et al. Spatial Positioning and Matrix Programs of Cancer-Associated Fibroblasts Promote T-cell Exclusion in Human Lung Tumors. Cancer Discov 2022;12:2606-25. [Crossref] [PubMed]
- Sun J, Jiang K, Wang Y, et al. One-Pot Synthesis of Tumor-Microenvironment Responsive Degradable Nanoflower-Medicine for Multimodal Cancer Therapy with Reinvigorating Antitumor Immunity. Adv Healthc Mater 2023;12:e2302016. [Crossref] [PubMed]
- Friedman G, Levi-Galibov O, David E, et al. Cancer-associated fibroblast compositions change with breast cancer progression linking the ratio of S100A4(+) and PDPN(+) CAFs to clinical outcome. Nat Cancer 2020;1:692-708. [Crossref] [PubMed]
- Yang SY, Denning SM, Mizuno S, et al. A novel activation pathway for mature thymocytes. Costimulation of CD2 (T,p50) and CD28 (T,p44) induces autocrine interleukin 2/interleukin 2 receptor-mediated cell proliferation. J Exp Med 1988;168:1457-68. [Crossref] [PubMed]
- Kerdidani D, Aerakis E, Verrou KM, et al. Lung tumor MHCII immunity depends on in situ antigen presentation by fibroblasts. J Exp Med 2022;219:e20210815. [Crossref] [PubMed]