An integrated analysis of scRNA-seq and RNA-seq data revealed metastasis-related regulators as prognostic indicators in lung adenocarcinoma
Highlight box
Key findings
• This study identified the risk factors associated with lung adenocarcinoma (LUAD) prognosis and micro-metastasis in lymph nodes.
What is known, and what is new?
• Lymph node metastases mostly disseminate from primary lung tumors, retaining the majority of driver factors.
• Single-cell RNA sequencing and RNA sequencing data were downloaded from public databases, and the genes critical to the prognosis of LUAD were successfully identified.
What is the implication, and what should change now?
• Through a bioinformatics analysis, we identified biomarkers that can serve as prognostic model for LUAD. Our findings could help overcome challenges in LUAD treatment.
Introduction
Cancer is a major global public health concern. It is estimated that there will be 2,001,140 new cancer cases and 611,720 cancer-related deaths in the United States in 2024 (1). The incidence of lung cancer has been decreasing annually since 2006 (2), and it is no longer the leading cancer type in terms of expected new cases; however, it remains the primary cause of estimated cancer-related deaths.
Despite rapid advancements in precision oncology, the 5-year survival rates of advanced-stage and stage I non-small cell lung cancer (NSCLC) patients are reported to be 15% and 83%, respectively (3). Notably, even in stage-I NSCLC patients who have undergone complete tumor resection, the incidence of postoperative recurrence or metastasis remains high at 21.7% (4). This may be due to the presence of early lymph node micro-metastasis (5). Lung adenocarcinoma (LUAD) is currently the most prevalent subtype of NSCLC. Recently, a growing number of studies have examined lymph node micro-metastasis in LUAD. The detection of lymph node micro-metastasis at an early stage has substantial clinical significance, as it represents a pivotal approach for enhancing the 5-year survival rate of LUAD patients.
With the rapid advancement of cancer genomics, bulk RNA-seq (i.e., large-scale transcriptome sequencing) has gradually become a primary tool in transcriptomics research. However, compared to conventional transcriptome sequencing, scRNA-seq has limitations in RNA coverage and sequencing depth. In contrast, bulk RNA sequencing reflects the overall gene transcription profile of a sample, offering higher RNA coverage and sequencing depth, making it a high-precision approach for gene expression analysis. Therefore, integrating scRNA-seq and bulk RNA-seq allows for complementary advantages. An increasing number of genes are being explored by integrating the sequencing results of both technologies, and a number of genes have been identified as effective therapeutic targets for LUAD. Li et al. conducted a systematic and comprehensive investigation of The Cancer Genome Atlas (TCGA) RNA-seq data to identify candidate long non-coding RNAs (lncRNAs) for LUAD prognosis (6). The study of Li et al. delved into the lncRNA-associated competitive endogenous RNA (ceRNA) network in LUAD to identify potential diagnostic and prognostic biomarkers. Su et al. conducted a comprehensive analysis of the lncRNA expression profile from RNA-seq data and identified MIR22HG as a potential novel diagnostic and prognostic biomarker and a cancer treatment target (7).
The advent of single-cell sequencing has extended our understanding of the heterogeneity of cellular transcriptomes, and has enabled the more comprehensive exploration of the distribution of gene expression. Single-cell sequencing equips researchers with the ability to develop more personalized treatment strategies, and has potential implications for cancer diagnosis and treatment resistance. For example, Pang et al.’s integrative analyses of scRNA-seq data identified the temporal regulatory networks of NSCLC, and revealed their pivotal role in the prognosis of patients (8). Further, Li et al. provided novel insights into the tumor heterogeneity of NSCLC via scRNA-seq, revealing a prevalent mixed lineage subpopulation of cancer cells with shared characteristics (9). Building on these findings, many studies have leveraged the combination of both approaches to identify potential biomarkers, enhancing the precision of the patient outcomes.
In this study, we used the scRNA-seq and bulk RNA-seq data of LUAD patients to perform systematic bioinformatics analyses to establish a prognostic model, and we then validated the ability of the model to stratify risk using other external cohorts. We also examined how core genes facilitate the development of LUAD. In summary, this study sought to leverage a combination of bulk RNA-seq and scRNA-seq data to identify factors that have a significant effect on the diagnosis and survival of patients with LUAD. We present this article in accordance with the TRIPOD reporting checklist (available at https://jtd.amegroups.com/article/view/10.21037/jtd-2025-482/rc).
Methods
Datasets source and screen
This study was conducted in accordance with the Declaration of Helsinki and its subsequent amendments. TCGA-LUAD patient gene expression RNA-seq data, patient data, and mutation information were downloaded from TCGA database (http://gdc.cancer.gov) (10). A total of 585 LUAD samples were obtained, comprising 59 normal cases and 526 tumor cases. After excluding samples with incomplete patient data and survival information, a cohort of 513 LUAD patients was included in this study. The GSE68465 dataset was downloaded from the National Center for Biotechnology Information (NCBI) Gene Expression Omnibus (GEO) database (RRID:SCR_005012) (http://www.ncbi.nlm.nih.gov/geo/) (11), which comprised 443 patient samples with prognostic information. The sequencing platform used for this dataset was the GPL96 (HG-U133A) Affymetrix Human Genome U133A Array. After downloading the corresponding messenger RNA (mRNA) probe expression matrix file and the annotation file for the respective sequencing platform, each probe was converted to its corresponding gene symbol, and the probes that did not match any gene symbols were removed. This process resulted in the gene expression matrix that was used for the subsequent analysis. Simultaneously, the scRNA-seq data were selected from the GSE131907 dataset in the GEO database. Next, 10 normal lymph node samples (GSM3827147, GSM3827148, GSM3827149, GSM3827150, GSM3827151, GSM3827152, GSM3827153, GSM3827154, GSM3827155, and GSM3827156) and seven metastatic lymph node samples (GSM3827140, GSM3827141, GSM3827142, GSM3827143, GSM3827144, GSM3827145, and GSM3827146) were chosen. Quality control, batch effect removal, and data integration were performed on these samples. The sequencing platform used was GPL16791 [Illumina HiSeq 2500 (Homo sapiens)].
Analysis of scRNA-seq data, cell type identification, and selection of core marker genes
A single-cell atlas modeling approach was used to depict the expression and distribution of the model genes. First, the 17 samples contained in the GEO dataset GSE131907 underwent quality control, batch effect removal, and data integration using the R package Seurat (version: 4.0.5, https://cran.r-project.org/web/packages/Seurat/index.html) (12). The SingleR package (version 2.4.0, https://bioconductor.org/packages/release/bioc/html/SingleR.html) (13) and CellMarker database were employed for the cell type annotation. The FindAllMarkers function from the Seurat package (RRID:SCR_007322) was used (setting parameters min.pct = 0.2 and only.pos = TRUE) to identify the core marker genes for each cell type in both the normal and metastatic lymph node samples.
Single-sample gene set enrichment analysis (ssGSEA) scores and functional enrichment analysis of the marker genes
Based on the significantly distinct marker genes for every cellular subtype in the single-cell data, ssGSEA scores were calculated for every cellular subtype in TCGA-LUAD dataset using the ssGSEA method (https://gsea-msigdb.github.io/ssGSEA-gpmodule/v10/) (14). A Wilcoxon analysis was conducted to assess the score differences in each cell type between the LUAD samples without lymph node metastasis (N0) and those with lymph node metastasis (N1, N2, and N3). The cells with significantly different scores (P<0.05) between the normal and LUAD groups were recorded as the core cells. The clusterProfiler (RRID:SCR_016884) package (15) in R software was used to perform the Gene Ontology (GO) and Kyoto Encyclopedia of Genes and Genomes (KEGG) (RRID:SCR_012773) functional enrichment analyses of the marker genes of each cell type.
Pseudo-temporal and cell-cell communication analyses
A pseudo-temporal analysis involves predicting cellular changes over time by constructing the trajectories of cellular changes. Monocle2 (RRID:SCR_016339) (version 2.26, http://cole-trapnell-lab.github.io/monocle-release/docs/#introduction) (16) is a logically clear and reasonable algorithm for pseudo-temporal analysis. Its trajectory inference based on dimensionality reduction plots conforms closely to natural patterns, providing an aesthetically pleasing and seamless connection to both preceding and subsequent analyses. Cell-cell communication analysis helps us to understand the interactions between cells, decipher cellular communication networks, examine the interactions among various cell types during development, explore the tumor immune microenvironment, and identify potential therapeutic targets for diseases. CellChat (an open-source R package, https://github.com/sqjin/CellChat, version 1.6.1) (17) was used to analyze the role of the model genes in cell communication.
Discovery and pathway enrichment analyses (PEAs) of the differentially expressed genes (DEGs) in LUAD
Based on the tumor vs. normal comparison, a differential analysis was performed to compare the patient and normal groups in TCGA-LUAD using the limma package (RRID:SCR_010943) in R (18). This analysis calculated the P value and log fold change (FC) for every gene. Additionally, the Benjamini and Hochberg method was employed for multiple comparison adjustment, resulting in adjusted P values (Padj values). The evaluation was conducted at two levels to determine both the differential FC and significance. The criteria for differential expression were set as follows: a P value <0.05, and a |logFC| >1 (indicating a fold change of at least 2). Based on the DEGs acquired in the preceding stage, the “clusterProfiler” (RRID:SCR_016884) package in the R software (15) was used to conduct the functional enrichment analysis using the GO data, and the PEA using the KEGG data.
Weighted gene co-expression network analysis (WGCNA)
A WGCNA was performed using TCGA-LUAD data to identify the disease-related module genes. The R package WGCNA (version 1.72-1; https://cran.r-project.org/web/packages/WGCNA/) (19) was employed, using the disease status as the phenotype for the WGCNA. The disease-related module genes were then screened, and the correlation between the module eigengenes and LUAD was analyzed using the Pearson correlation coefficient.
Identification of significant prognostic candidate genes
A set of candidate genes comprising the intersecting core cell marker genes, LUAD-related genes from the WGCNA, and DEGs were used for the subsequent analysis. These candidate genes were then used for further investigations. The clinical expression data of the 513 patients were randomly divided into a training set (359 cases) and a validation set (154 cases) at a ratio of 7:3. For the training set of 359 samples, based on the expression levels of the identified candidate genes, a single-factor Cox regression analysis was performed using the R survival package (version 2.41-1; http://bioconductor.org/packages/survivalr/). A significance threshold with a P value <0.05 was applied to filter out the genes that were significantly associated with survival prognosis at the expression level, and these genes were selected for further analysis.
Building and validating the prognostic model
The variables with P values <0.05 from the previous step were incorporated into the survival regression analysis using the least absolute shrinkage and selection operator (LASSO) algorithm from the glmnet (RRID:SCR_015505) package (20). A 10-fold cross-validation was employed to analyze the key prognostic genes, and subsequently, a score formula was constructed through stepwise Cox regression analysis using the R package survminer (version 0.4.9; https://cran.rstudio.com/web/packages/survminer/index.html). The stepwise Cox regression analysis established the risk-score formula based on the regression coefficients of individual genes and the expression levels of the model genes. The formula was expressed as follows:
(gene expression denotes the gene expression value and β denotes the corresponding LASSO regression coefficient).
Using this equation, the risk-score values for every sample in the training set (359 cases), internal validation set (154 cases), and external validation set (GSE68465) were computed. The samples were then divided into high- and low-risk groups based on the median risk score, and the R survival package was used to assess the correlation between the grouping and the actual survival prognosis information using Kaplan-Meier curves. The “survROC” package was used to plot the receiver operating characteristic (ROC) curves to evaluate the performance of the risk-score model in predicting the overall survival (OS) of LUAD patients at 1, 2, 3, 4, and 5 years.
Clinical characteristic analysis of subtypes
Integrating TCGA clinical information data, the samples were categorized into subtypes based on their clinical information, including their age (>60 and ≤60 years), gender, M stage, T stage, tumor microenvironment, and OS. In every subtype, the LUAD specimens were subdivided into two risk sets (high risk and low risk). The allocation of the clinical-pathological features among the subtypes was examined by the Kruskal-Wallis test or Wilcoxon rank-sum test. To gain a deeper understanding of the correlation between the clinical-pathological features and survival rates, a stratified analysis was conducted of the clinical factors for the two groups.
Cell culture
The Cell Bank of the Chinese Academy of Sciences (Shanghai, China) provided the LUAD cell lines (A549) and normal lung epithelial cell line (BEAS-2B) used in the study. F-12 K medium containing 10% fetal bovine serum (FBS) was used to culture the A549 cells. A BEGMTM BulletKitTM (Lonza, GA, USA) was used to culture the BEAS-2B cells. The mycoplasma contamination of these two cells was checked using the short tandem repeat method.
Plasmid construction and transfection
For overexpression, the full-length coding sequence was cloned into a plasmid with Clover Destabilized Hairpin (pCDH) vector. The transfection was performed using Lipofectamine 3000 (Invitrogen, Thermofisher, L3000001, USA) in accordance with the manufacturer’s instructions.
RNA extraction and quantitative real-time polymerase chain reaction (qRT-PCR)
The total RNA was extracted from the cultured cells using TRIzol reagent (Invitrogen, Thermofisher, 15596026CN, USA) in accordance with the manufacturer’s instructions. In the following step, RNA was reverse transcribed into complementary DNA using HiScript® II Q RT SuperMix (+gDNA wiper) (Vazyme, Nanjing, China). The Quantitative Real-time polymerase chain reaction(qRT-PCR) was carried out with Hieff UNICON®qPCR Synergetic Binding Reagent Green MasterMix (Yeasen, 11200ES03, China) using a QuantStudioTM Dx Real-Time PCR Instrument (Applied Biosystems, Thermofisher, QuantStudio 6, USA). The results were normalized to glyceraldehyde-3-phosphate dehydrogenase (GAPDH). The primers for the qRT-PCR are listed in Table S1.
Cell counting kit 8 (CCK-8) assay
The cells were plated into 96-well plates (1,500 cells per well). The CCK-8 detection kit (C0039, Beyotime, Shanghai, China) was used to detect cell proliferation. CCK-8 solution (10 µL) was added at 0, 1, 2, 3, and 4 days for 2 hours; absorbance was detected at 450 nm using a spectrophotometer.
Invasion assay
For the Transwell invasion assay, the upper chamber was coated with Matrigel before the cells were added. Next, the 1.5×104 cells in the 0.1-mL serum-free medium were transferred to the upper chamber, and medium with 10% FBS was added to the lower chamber. All the cells were incubated for 24 hours. The cells were fixed with 4% paraformaldehyde and stained with 0.5% crystal violet. The upper chamber was cleaned with a cotton swab. The upper chamber was observed under a microscope, and the cells were counted. All the derived cell invasion data were normalized to that of cell proliferation at 24 hours.
Statistical analysis
All statistical analyses were conducted using R software (v3.6.3). Correlation matrices were generated based on Pearson or Spearman correlation coefficients. Comparisons between two groups were assessed using the Wilcoxon test. Survival analysis was performed using Kaplan-Meier curves, and differences were evaluated with the log-rank test. A P value of less than 0.05 was considered statistically significant.
Results
Identification of LUAD metastatic lymph node cell subtypes
First, we filtered out the unqualified cells and obtained the qualified core cells from 10 normal lymph node samples and seven metastatic lymph node samples for the subsequent analysis (Figure 1A). A principal component analysis (PCA) was conducted on the specimens, and all the cells were found to be dispersed, consistent with logical expectations (Figure 1B), indicating effective sample integration without batch effects. We identified 2,000 highly variable genes after performing an analysis of variance (Figure 1C). Further, in the PCA, we also selected the top 20 principal components (PCs) with P values <0.05 for further analysis (Figure 1D,1E). Subsequently, the uniform manifold approximation and projection (UMAP) algorithm was employed to classify the core cells into 11 distinct cell clusters (Figure 1F). Using the “singleR” package and the CellMarker database (21), we identified marker genes to annotate different clusters, which resulted in the following 11 cell clusters: macrophages, monocytes, dendritic cells (DCs), cluster of differentiation 8-positive effector memory T (CD8+ Tem) cells, natural killer (NK) cells, CD4-positive central memory T (CD4+_central_memory) cells, epithelial cells, memory B cells, plasma cells, naive B cells, and megakaryocyte-erythroid progenitor (MEP) cells (Figure 1G). The expression of the key signature genes for every cell subtype was observed using a bubble plot (Figure 1H).
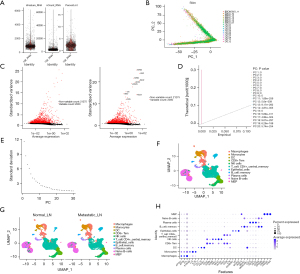
ssGSEA scores and the PEA
ssGSEA scores were calculated for every cell type in TCGA-LUAD dataset using the ssGSEA method. A Wilcoxon analysis was conducted to examine the differences in the ssGSEA scores between the samples with no lymph node metastasis (N0) and the samples with lymph node metastasis (N1, N2, and N3) for each cell type. As Figure 2A,2B show, there were significant differences in the ssGSEA scores for the DCs (P=0.02), epithelial cells (P=0.001), macrophages (P=0.03), MEP cells (P=0.03), and plasma cells (P=0.004) between the groups without lymph node metastasis and those with lymph node metastasis. Therefore, these five cell types were selected as the core cells for the subsequent analysis.
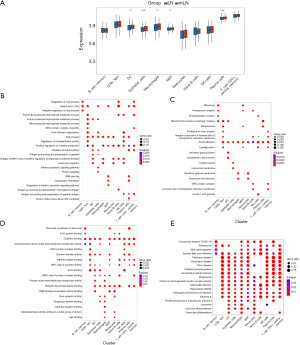
We also conducted GO and KEGG PEAs of the core marker genes of each cell type, including the core cells (Figure 2A). We found that, except for epithelial cells and memory B cells, the marker genes of the cell subtypes were related to the positive regulation of cytokine production (Figure 2B). The results indicate that apart from memory B cells, other cell-type marker genes were linked to focal adhesion (Figure 2C). In addition, all the cell-type marker genes were connected to the cadherin binding, with the sole exception of macrophages (Figure 2D). In addition, the marker genes of B cells were connected to the viral carcinogenesis. The marker genes of endothelial cells were linked to chemical carcinogenesis (Figure 2E).
In accordance with the described methods, a pseudo-temporal analysis was conducted, and the Monocle 2 algorithm was used to examine the interactions between the cells. The results suggest that the cells in the LUAD metastatic lymph node samples underwent differentiation in three distinct directions over time (Figure 3A). The trajectory analysis revealed that subpopulations of T cells and B cells exhibited dissimilar differentiation patterns. Notably, one branch differentiated predominantly into mononuclear cells, while another branch differentiated predominantly into epithelial cells (Figure 3B).
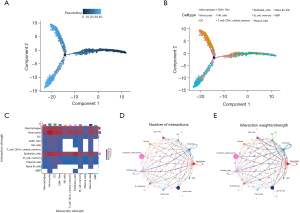
According to the heatmap of the ligand-receptor pair quantities, the epithelial cells, macrophages, and monocytes exhibited stronger interactions with other cells (Figure 3C). Specifically, the frequency and strength of the interactions between monocytes and macrophages, monocytes and DCs, as well as monocytes and CD8+ Tem cells, were high (Figure 3D,3E).
Detection and functional enrichment analysis of the DEGs in the bulk RNA-seq data
In total, 1,994 DEGs were identified in TCGA-LUAD cohort (which comprised 59 normal tissue samples and 513 LUAD samples), of which 1,089 genes were upregulated and 905 genes were downregulated (Figure 4A,4B). The GO analysis revealed that the DEGs were mainly enriched in functions related to humoral immune response, phagocytosis, cell recognition, and other immune-related processes (Figure 4C). The KEGG enrichment analysis results indicated that the DEGs were enriched in pathways such as the cytokine-cytokine receptor interaction, cell cycle, cell adhesion molecules, and phagosomes (Figure 4D).
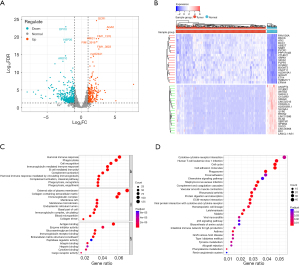
Identification of LUAD-related core gene modules
To establish a link between the clinical information and key genes, a WGCNA was conducted. In the establishment of the transcript-transcript association networks, the soft-thresholding power β was set to 11 when the scale-free topology fitting index reached 0.9 (Figure 5A). The dynamic tree-cutting algorithm was employed to divide the modules and construct a network diagram, resulting in 10 modules in total (excluding the gray module) (Figure 5B). A bivariate analysis between the sample traits and the modules was performed. Of these 11 modules, three (i.e., the blue, turquoise, and magenta modules) exhibited a strong relationship with the disease (r≥0.4, P<0.05) (Figure 5C). Therefore, the genes in these three modules were selected for further analysis (blue: 802 genes; turquoise: 929 genes; magenta: 132 genes; total: 1,863 genes) (Figure 5D-5F).
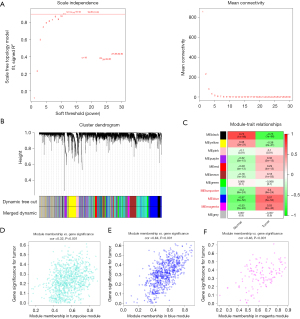
Development and confirmation of the predictive model
Using the intersection of the marker genes contained in the five core cells from the above single-cell data and the LUAD-related genes from the WGCNA, as well as the DEGs, the acquired genes were characterized as the candidate genes. As Figure 6A shows, a total of 145 candidate genes were obtained.
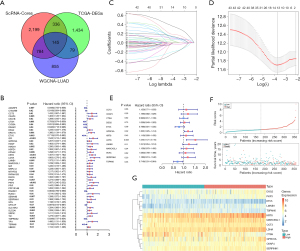
To assess whether the 145 candidate genes could serve as prognostic biomarkers for LUAD, a univariate Cox proportional hazards model was constructed of the previously identified genes. A total of 48 feature genes associated with prognosis were identified (Figure 6B). These genes were identified through the LASSO regression, and the findings are shown in Figure 6C. Subsequently, an optimized gene combination was obtained using a Cox regression algorithm to construct the Cox model (Figure 6C,6D). The results revealed a final set of 13 model genes (i.e., CCT3, CKAP4, CTSH, DSG2, ETV5, GPRC5A, KRT8, LDHA, LMNB1, MARCKSL1, PKP3, SERPINH1, and TSPAN6) (Figure 6E). Risk score = 0.11520548 × CCT3 + 0.18112067 × CKAP4 − 0.00997156 × CTSH + 0.03517401 × DSG2 − 0.23767106 × ETV5 + 0.18523307 × GPRC5A + 0.10402744 × KRT8 + 0.43195223 × LDHA + 0.21564394 × LMNB1 + 0.12106006 × MARCKSL1 + 0.079441205 × PKP3 + 0.17047390 × SERPINH1 − 0.334711202 × TSPAN6.
As described in the methods section above, the risk score of every individual was estimated. TCGA training, validation, and GEO validation set samples were then classified into the following two groups: the high-risk group (comprising those with a value above the average value); and the low-risk group (comprising those with a value less than or equal to the average value) (Figure 6F,6G). A survival analysis of the high- and low-risk groups was performed, as shown in Figure 7A, indicating a significant difference in patient survival between the high- and low-risk groups (P<0.05). The model performed well as assessed by the Kaplan-Meier curves using the survival package in R. The Kaplan-Meier analysis revealed that patients with high-risk scores had significantly lower OS than those with low-risk scores. The ROC curves were generated with survROC to evaluate the area under the curve (AUC) values for 1 to 5 years survival in LUAD individuals (Figure 7B). Additionally, the effectiveness of the predictive model was validated using the GSE68465 dataset and the validation set (Figure 7C-7F). For clinical validity, we also present the outcome data using Kaplan-Meier curves for stage-I disease (Figure S1A,S1B). The results indicated that the prognostic model constructed with the selected feature genes performed well. The internal and external validation sets showed a significant difference in the survival analysis (P<0.05). The AUC values for the 1- to 5-year ROC curves were all >0.6.
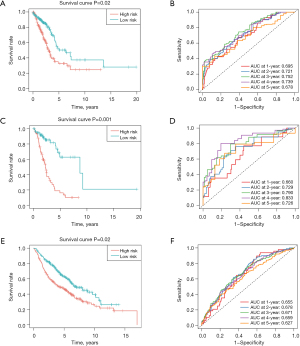
Analysis of the relationship between the risk scores and clinical features
We integrated TCGA clinical information data, and then employed the Kruskal-Wallis test or Wilcoxon rank-sum test to analyze the distribution differences of risk scores among the different clinical factors (i.e., age, gender, stage, and pathological stage) and different subtypes (Table 1). A heatmap of the clinical factors and risk scores is shown in Figure S2A. A stratified analysis of the clinical features revealed significant differences in the survival rates between the high- and low-risk groups in terms of pathological T and N stage, and overall stage (Figure S2B-S2G). Therefore, our prognostic model had good predictive value. To investigate the prognostic implications of clinical and pathological features in conjunction with the risk model, clinical and pathological factors were included in the Cox independent prognosis analysis to assess the contribution of each variable to patient survival. The findings indicated that both risk score and stage acted as independent prognostic factors for patients with LUAD (Figure S3A). Finally, the predicted results were visualized in a nomogram (Figure S3B). The C-index was calculated to be 0.71. Based on the predictive model, a calibration curve was generated, and a slope closer to 1 indicated more accurate predictions (Figure S3C). Taken together, our findings indicate that the risk score is an independent predictor. The nomograph demonstrates a high prognostic value for forecasting OS of LUAD individuals.
Table 1
Variables | Low (N=180), n (%) | High (N=179), n (%) | Total (N=359), n (%) | P value |
---|---|---|---|---|
Pathologic M | 0.32 | |||
M0 | 122 (67.80) | 124 (69.10) | 246 (68.45) | |
M1 | 7 (3.95) | 13 (7.30) | 20 (5.63) | |
M2 | 51 (28.25) | 42 (23.60) | 93 (25.92) | |
Pathologic N | 0.007** | |||
N0 | 135 (74.86) | 107 (59.78) | 242 (67.32) | |
N1 | 25 (13.97) | 39 (21.79) | 64 (17.88) | |
N2 | 14 (7.82) | 30 (16.76) | 44 (12.29) | |
N3 | 0 (0.00) | 1 (0.56) | 1 (0.28) | |
NX | 6 (3.35) | 2 (1.12) | 8 (2.23) | |
Pathologic T | 0.045* | |||
T1 | 72 (40.00) | 46 (25.70) | 118 (32.87) | |
T2 | 85 (47.22) | 103 (57.54) | 188 (52.37) | |
T3 | 17 (9.44) | 18 (10.06) | 35 (9.75) | |
T4 | 5 (2.78) | 11 (6.15) | 16 (4.46) | |
TX | 1 (0.56) | 1 (0.56) | 2 (0.56) | |
Age (years) | 0.06 | |||
≤60 | 47 (26.14) | 62 (34.83) | 109 (30.51) | |
>60 | 133 (73.86) | 117 (65.17) | 250 (69.49) | |
Gender | 0.23 | |||
Male | 78 (43.33) | 90 (50.28) | 168 (46.80) | |
Female | 102 (56.67) | 89 (49.72) | 191 (53.20) | |
Stage | <0.001*** | |||
Stage I | 116 (64.37) | 84 (46.93) | 200 (55.71) | |
Stage II | 40 (22.41) | 43 (24.02) | 83 (23.12) | |
Stage III | 17 (9.20) | 39 (21.79) | 56 (15.60) | |
Stage IV | 7 (4.02) | 13 (7.26) | 20 (5.57) |
*, P<0.05; **, P<0.01; ***, P<0.001.
Identifying the roles of two specific genes in this cluster
To further investigate the roles of the regulated genes identified in this cluster, we conducted qRT-PCR to measure their expression levels in the A549 cell line (Figure 8A). The expression levels of genes SERPINH1 (P=0.14), TSPAN6 (P=0.93), and MARCKSL1 (P=0.88) were not statistically significant. The remaining genes showed differential expression. These genes have been recognized as biomarkers in various tumor types; however, the functional roles of KRT8 and CTSH in LUAD remain poorly understood. Therefore, we constructed overexpression plasmids for these two genes to perform an experimental validation (Figure 8B). Through a comprehensive functional validation, we found that KRT8 significantly promoted cell proliferation, while CTSH exhibited the opposite effect (Figure 8C). The transwell experiments further showed that KRT8 significantly enhanced tumor cell invasion, while CTSH exhibited the opposite effect, suggesting their potential role in tumor cell invasiveness (Figure 8D). Collectively, these findings provide strong evidence of the malignant nature of these aggressive tumor cells and highlight the potential prognostic value.
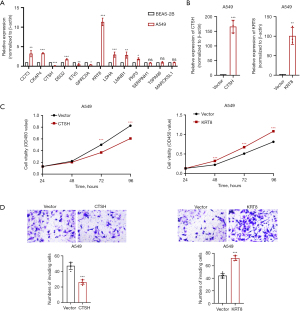
Discussion
LUAD is one of the most prevalent malignancies globally (1). The incidence of LUAD continues to increase in numerous countries, and the mortality rate of LUAD remains high (1). Despite extensive endeavors in recent times aimed at addressing LUAD, prognostic evaluation remains constrained by the tumor’s heterogeneity and invasive nature (22). From a therapeutic perspective, stage IIIA–N2 NSCLC exhibits significant heterogeneity, meaning that patients with lymph node metastasis at the same stage may have vastly different situations, requiring considerable differences in treatment approaches (23,24). Chang et al. performed sequencing to detect the mutation status of epidermal growth factor receptor (EGFR) in the primary tumors and lymph node metastases of 56 NSCLC patients, and found that the heterogeneity of the EGFR mutations in primary tumors and lymph node metastases reached 28.6% (16/56), indicating varying degrees of heterogeneity of the EGFR gene in primary tumors and metastatic lesions in NSCLC (25). The mutation heterogeneity between different lesions in the same patient may lead to differential treatment responses. Therefore, novel biomarkers urgently need to be identified to facilitate the development of personalized treatments and enhance patient prognosis. Unlike bulk RNA-seq, which examines the average gene expression levels, scRNA-seq has become a valuable tool for dissecting transcriptional profiles to delineate cell subpopulations and elucidate specific biomarkers and heterogeneity among diverse cell types in various cancers, such as LUAD. Thus, our investigation integrated bulk RNA-seq and scRNA-seq to establish a risk model. This model was shown to have outstanding prognostic capabilities and high accuracy in predicting the immunotherapy response in LUAD.
In the initial step, we found 11 cell clusters from the scRNA-seq data of normal lymph nodes and metastatic lymph nodes, comprising macrophages, monocytes, DCs, CD8+ Tem cells, NK cells, CD4+_central_memory cells, epithelial cells, memory B cells, plasma cells, naive B cells, and MEP cells. Among these, epithelial cells, macrophages, and monocytes exhibited strong interactions with other cells, and the expression levels of the former two were generally upregulated in the lymph node metastasis samples. This heterogeneity and interaction of 11 cell clusters with the tumor microenvironment are crucial in tumorigenesis and therapy resistance, which also indicates predictive potential of the risk score for lymph node metastasis. In the second step, we obtained the DEGs from TCGA, and the GO/KEGG analysis revealed enrichment in pathways such as the cytokine-cytokine receptor interaction, cell cycle, cell adhesion molecules, and phagosomes, which may contribute to the progression and metastasis of LUAD. A study has shown that genes related to cytokine interactions primarily regulate immune responses in LUAD (26). Additionally, the activation of the cell cycle and cell adhesion molecule signaling pathways is vital in initiating and advancing LUAD. Further, we identified three modules highly correlated with the disease using a WGCNA. By intersecting the gene sets mentioned above, we identified 145 candidate genes to enhance the stability of the biomarkers.
Next, a prognostic model consisting of 13 genes was established using a univariate Cox regression analysis and the LASSO algorithm. These genes included CCT3, CKAP4, CTSH, DSG2, ETV5, GPRC5A, KRT8, LDHA, LMNB1, MARCKSL1, PKP3, SERPINH1, and TSPAN6. The results of the ROC curve analysis showed that this model was able to accurately predict patient prognosis. A key advantage of our model is that it is the first to integrate single-cell sequencing data from lymph node metastases, a feature that distinguishes it from many existing models and underscores its uniqueness. By incorporating data on lymph node metastases, our model enhances predictive accuracy. Unlike other signatures, this signature was derived from the integration of diverse datasets and algorithms, and validated by both intrinsic and extrinsic validation sets, which were distinct from the training set. The AUC values ranged from 0.627 to 0.833, indicating high reliability and relevance. The survival differences between the high- and low-risk groups differed a little for the various datasets. However, this did not affect the overall effectiveness of the model. This may be related to the sample size, sequencing platforms, and individual patient differences.
We also examined the association between the model and clinical-pathological features. The findings revealed a significant correlation between the risk score and patients’ lymph node metastasis and tumor T stage, suggesting that the model possesses predictive value for OS. Additionally, we found that many of the 13 signature genes were related to the tumor microenvironment. Keratin 8 (KRT8) is a major component of the intermediate filament cytoskeleton and is primarily expressed in simple epithelial tissues (27). Apart from colon cancer, KRT8 has been found to be significantly overexpressed in many cancers. An analysis of scRNA-seq data from 7,447 lung tumor cells revealed that the genes significantly associated with KRT8 (P<0.05) were involved in the p53-related pathway (28). KRT8 has also been identified as a target of cisplatin. The upregulation of KRT8 in cancer-associated fibroblasts causes cisplatin to inhibit the metastatic potential of lung cancer cells by suppressing the AKT pathway. The knockdown of CCT3 significantly inhibits proliferation and promotes apoptosis in A549 cells (29). CCT3 has shown a positive correlation with infiltrating T helper 2 cell, and a negative correlation with mast cells and immature DCs. In breast cancer, the accumulation of liver X receptor oxysterol ligands in extracellular vesicles derived from cancer cells is regulated by Tspan6. Tspan6 stimulates the chemoattractive potential of breast cancer cells for B cells (30). Additionally, qPCR was conducted to verify the expression level of these genes in the A549 cells. However, the role of CTSH and KRT8 in lung cancer has not yet been reported. Thus, we selected both of them as targets for further experiments. KRT8 promoted the proliferation and invasion of LUAD, while CTSH exhibited an inhibitory effect.
Given the cancer heterogeneity, interactions among the infiltrating immune cells, different cell populations, the tumor mutation burden, and clinical characteristics, the robustness of this investigation lies in the development and implementation of an innovative prognostic framework adept at precisely predicting OS in LUAD patients. The results of this study offer tangible support for the targeted and precise management of LUAD patients. However, this study was not without its inherent limitations, which include: (I) the relatively small size of the scRNA-seq sample, future validation should be conducted in more prospective and multi-center LUAD cohorts; (II) that the pathways that regulate the marker genes in LUAD remain unclear; and (III) further animal experiments will be necessary to elucidate the underlying mechanisms. The latter represents an avenue for future research.
Conclusions
We conducted a comprehensive bioinformatics analysis and identified key genes associated with LUAD prognosis. Among these genes, we found that KRT8 promotes the proliferation and invasion of LUAD, while CTSH exerts an inhibitory effect.
Acknowledgments
None.
Footnote
Reporting Checklist: The authors have completed the TRIPOD reporting checklist. Available at https://jtd.amegroups.com/article/view/10.21037/jtd-2025-482/rc
Data Sharing Statement: Available at https://jtd.amegroups.com/article/view/10.21037/jtd-2025-482/dss
Peer Review File: Available at https://jtd.amegroups.com/article/view/10.21037/jtd-2025-482/prf
Funding: This work was supported by
Conflicts of Interest: All authors have completed the ICMJE uniform disclosure form (available at https://jtd.amegroups.com/article/view/10.21037/jtd-2025-482/coif). The authors have no conflicts of interest to declare.
Ethical Statement: The authors are accountable for all aspects of the work in ensuring that questions related to the accuracy or integrity of any part of the work are appropriately investigated and resolved. This study was conducted in accordance with the Declaration of Helsinki and its subsequent amendments.
Open Access Statement: This is an Open Access article distributed in accordance with the Creative Commons Attribution-NonCommercial-NoDerivs 4.0 International License (CC BY-NC-ND 4.0), which permits the non-commercial replication and distribution of the article with the strict proviso that no changes or edits are made and the original work is properly cited (including links to both the formal publication through the relevant DOI and the license). See: https://creativecommons.org/licenses/by-nc-nd/4.0/.
References
- Siegel RL, Giaquinto AN, Jemal A. Cancer statistics, 2024. CA Cancer J Clin 2024;74:12-49. [Crossref] [PubMed]
- Jemal A, Ma J, Rosenberg PS, et al. Increasing lung cancer death rates among young women in southern and midwestern States. J Clin Oncol 2012;30:2739-44. [Crossref] [PubMed]
- Allemani C, Matsuda T, Di Carlo V, et al. Global surveillance of trends in cancer survival 2000-14 (CONCORD-3): analysis of individual records for 37 513 025 patients diagnosed with one of 18 cancers from 322 population-based registries in 71 countries. Lancet 2018;391:1023-75. [Crossref] [PubMed]
- Wang C, Wu Y, Shao J, et al. Clinicopathological variables influencing overall survival, recurrence and post-recurrence survival in resected stage I non-small-cell lung cancer. BMC Cancer 2020;20:150. [Crossref] [PubMed]
- Jeong JH, Kim NY, Pyo JS. Prognostic roles of lymph node micrometastasis in non-small cell lung cancer. Pathol Res Pract 2018;214:240-4. [Crossref] [PubMed]
- Li X, Li B, Ran P, et al. Identification of ceRNA network based on a RNA-seq shows prognostic lncRNA biomarkers in human lung adenocarcinoma. Oncol Lett 2018;16:5697-708. [Crossref] [PubMed]
- Su W, Feng S, Chen X, et al. Silencing of Long Noncoding RNA MIR22HG Triggers Cell Survival/Death Signaling via Oncogenes YBX1, MET, and p21 in Lung Cancer. Cancer Res 2018;78:3207-19. [Crossref] [PubMed]
- Pang J, Yu Q, Chen Y, et al. Integrating Single-cell RNA-seq to construct a Neutrophil prognostic model for predicting immune responses in non-small cell lung cancer. J Transl Med 2022;20:531. [Crossref] [PubMed]
- Li Q, Wang R, Yang Z, et al. Molecular profiling of human non-small cell lung cancer by single-cell RNA-seq. Genome Med 2022;14:87. [Crossref] [PubMed]
- Wang Z, Jensen MA, Zenklusen JC. A Practical Guide to The Cancer Genome Atlas (TCGA). Methods Mol Biol 2016;1418:111-41. [Crossref] [PubMed]
- Barrett T, Wilhite SE, Ledoux P, et al. NCBI GEO: archive for functional genomics data sets--update. Nucleic Acids Res 2013;41:D991-5. [Crossref] [PubMed]
- Hao Y, Stuart T, Kowalski MH, et al. Dictionary learning for integrative, multimodal and scalable single-cell analysis. Nat Biotechnol 2024;42:293-304. [Crossref] [PubMed]
- Aran D, Looney AP, Liu L, et al. Reference-based analysis of lung single-cell sequencing reveals a transitional profibrotic macrophage. Nat Immunol 2019;20:163-72. [Crossref] [PubMed]
- Subramanian A, Tamayo P, Mootha VK, et al. Gene set enrichment analysis: a knowledge-based approach for interpreting genome-wide expression profiles. Proc Natl Acad Sci U S A 2005;102:15545-50. [Crossref] [PubMed]
- Wu T, Hu E, Xu S, et al. clusterProfiler 4.0: A universal enrichment tool for interpreting omics data. Innovation (Camb) 2021;2:100141. [Crossref] [PubMed]
- Trapnell C, Cacchiarelli D, Grimsby J, et al. The dynamics and regulators of cell fate decisions are revealed by pseudotemporal ordering of single cells. Nat Biotechnol 2014;32:381-6. [Crossref] [PubMed]
- Jin S, Guerrero-Juarez CF, Zhang L, et al. Inference and analysis of cell-cell communication using CellChat. Nat Commun 2021;12:1088. [Crossref] [PubMed]
- Smyth GK. limma: Linear Models for Microarray Data. In: Gentleman R, Carey VJ, Huber W, et al. Bioinformatics and Computational Biology Solutions Using R and Bioconductor. New York, NY: Springer New York; 397-420.
- Langfelder P, Horvath S. WGCNA: an R package for weighted correlation network analysis. BMC Bioinformatics 2008;9:559. [Crossref] [PubMed]
- Friedman J, Hastie T, Tibshirani R. Regularization Paths for Generalized Linear Models via Coordinate Descent. J Stat Softw 2010;33:1-22.
- Zhang X, Lan Y, Xu J, et al. CellMarker: a manually curated resource of cell markers in human and mouse. Nucleic Acids Res 2019;47:D721-8. [Crossref] [PubMed]
- Wang X, Bai H, Zhang J, et al. Genetic Intratumor Heterogeneity Remodels the Immune Microenvironment and Induces Immune Evasion in Brain Metastasis of Lung Cancer. J Thorac Oncol 2024;19:252-72. [Crossref] [PubMed]
- Kang SR, Song HC, Byun BH, et al. Intratumoral Metabolic Heterogeneity for Prediction of Disease Progression After Concurrent Chemoradiotherapy in Patients with Inoperable Stage III Non-Small-Cell Lung Cancer. Nucl Med Mol Imaging 2014;48:16-25. [Crossref] [PubMed]
- Cortiula F, Reymen B, Peters S, et al. Immunotherapy in unresectable stage III non-small-cell lung cancer: state of the art and novel therapeutic approaches. Ann Oncol 2022;33:893-908. [Crossref] [PubMed]
- Chang YL, Wu CT, Shih JY, et al. Comparison of p53 and epidermal growth factor receptor gene status between primary tumors and lymph node metastases in non-small cell lung cancers. Ann Surg Oncol 2011;18:543-50. [Crossref] [PubMed]
- Han S, Jiang D, Zhang F, et al. A new immune signature for survival prediction and immune checkpoint molecules in non-small cell lung cancer. Front Oncol 2023;13:1095313. [Crossref] [PubMed]
- Fang J, Wang H, Liu Y, et al. High KRT8 expression promotes tumor progression and metastasis of gastric cancer. Cancer Sci 2017;108:178-86. [Crossref] [PubMed]
- Li X, Song Q, Guo X, et al. The Metastasis Potential Promoting Capacity of Cancer-Associated Fibroblasts Was Attenuated by Cisplatin via Modulating KRT8. Onco Targets Ther 2020;13:2711-23. [Crossref] [PubMed]
- Huang J, Hu B, Yang Y, et al. Integrated analyzes identify CCT3 as a modulator to shape immunosuppressive tumor microenvironment in lung adenocarcinoma. BMC Cancer 2023;23:241. [Crossref] [PubMed]
- Molostvov G, Gachechiladze M, Shaaban AM, et al. Tspan6 stimulates the chemoattractive potential of breast cancer cells for B cells in an EV- and LXR-dependent manner. Cell Rep 2023;42:112207. [Crossref] [PubMed]